Background: Prostate cancer (PCa) is a prevalent form of malignant tumors affecting the prostate gland and is frequently diagnosed in males in Western countries. Identifying diagnostic and prognostic biomarkers is not only important for screening drug targets but also for understanding their pathways and reducing the cost of experimental verification of PCa. The objective of this study was to identify and validate promising diagnostic and prognostic biomarkers for PCa. Methods: This study implemented a machine learning technique to evaluate the diagnostic and prognostic biomarkers of PCa using protein-protein interaction (PPI) networks. In addition, multi-database validation and literature review were performed to verify the diagnostic biomarkers. To optimize the prognosis of our results, univariate Cox regression analysis was utilized to screen survival-related genes. This study employed stepwise multivariate Cox regression analysis to develop a prognostic risk model. Finally, receiver operating characteristic analysis confirmed that these predictive biomarkers demonstrated a substantial level of sensitivity and specificity when predicting the prognostic survival of patients. Results: The hub genes were UBE2C (Ubiquitin Conjugating Enzyme E2 C), CCNB1 (Cyclin B1), TOP2A (DNA Topoisomerase II Alpha), TPX2 (TPX2 Microtubule Nucleation Factor), CENPM (Centromere Protein M), F5 (Coagulation Factor V), APOE (Apolipoprotein E), NPY (Neuropeptide Y), and TRIM36 (Tripartite Motif Containing 36). All of these hub genes were validated by multiple databases. By validation in these databases, these 10 hub genes were significantly involved in significant pathways. The risk model was constructed by a four-gene-based prognostic factor that included TOP2A, UBE2C, MYL9, and FLNA. Conclusions: The machine learning algorithm combined with PPI networks identified hub genes that can serve as diagnostic and prognostic biomarkers for PCa. This risk model will enable patients with PCa to be more accurately diagnosed and predict new drugs in clinical trials.
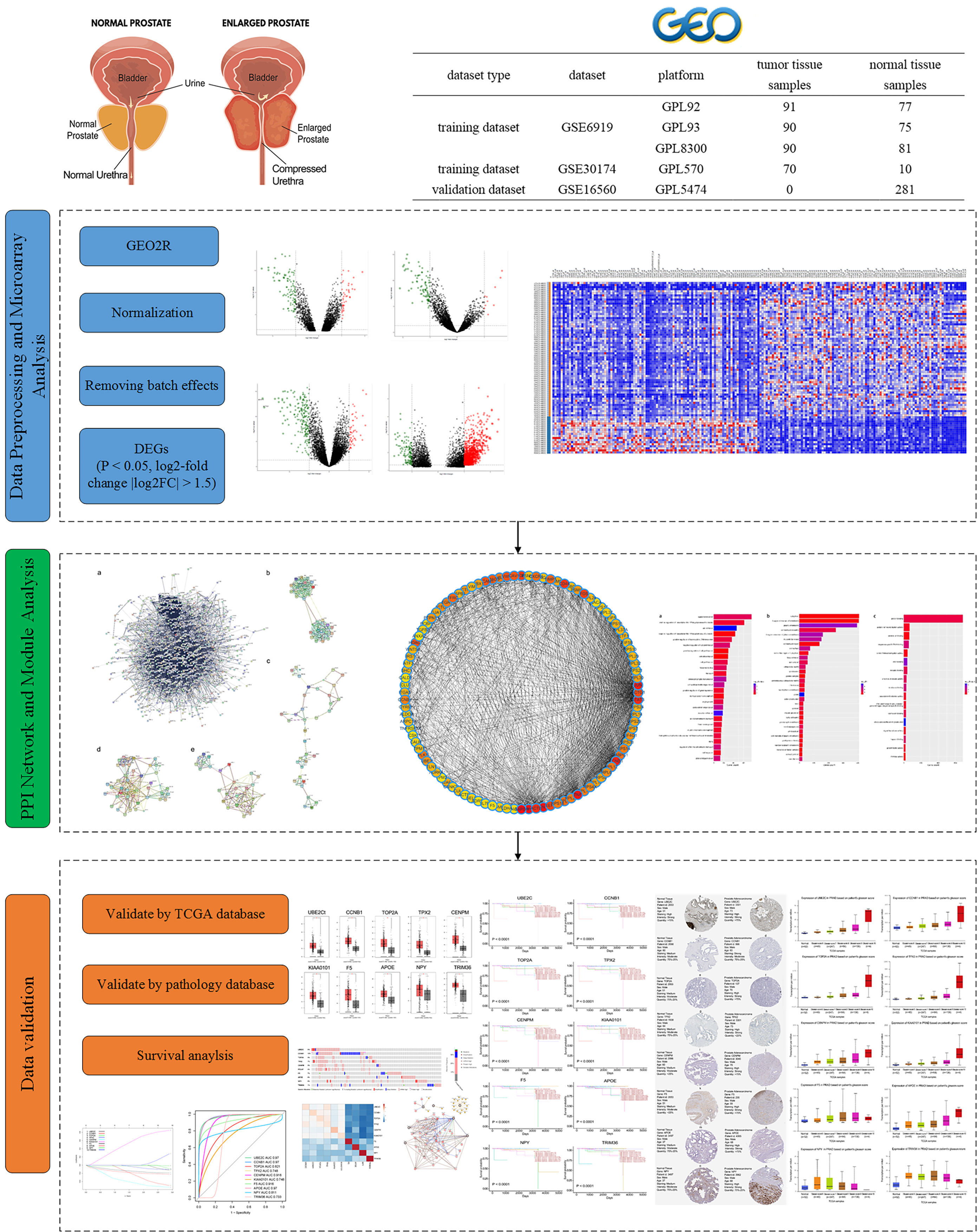