Academic Editor: Rafael Franco
Background: Machine learning techniques and magnetic resonance imaging methods have been widely used in computer-aided diagnosis and prognosis of severe brain diseases such as schizophrenia, Alzheimer, etc. Methods: In this paper, a regularized multi-task learning method for schizophrenia classification is proposed, and three MRI datasets of schizophrenia, collected from different data centers, are investigated. Firstly, slice extraction is used in image preprocessing. Then texture features of gray-level co-occurrence matrices are extracted from the above processed images. Finally, a p-norm regularized multi-task learning method is proposed to simultaneously learn the site-specific and site-shared features of the multi-site data, which can effectively discriminate schizophrenia patients from normal controls. Results: The classification error rate on 10 datasets can be reduced from 10% to 30%. Conclusions: The proposed method obtains excellent results and provides objective evidence for clinical diagnosis and treatment of schizophrenia.
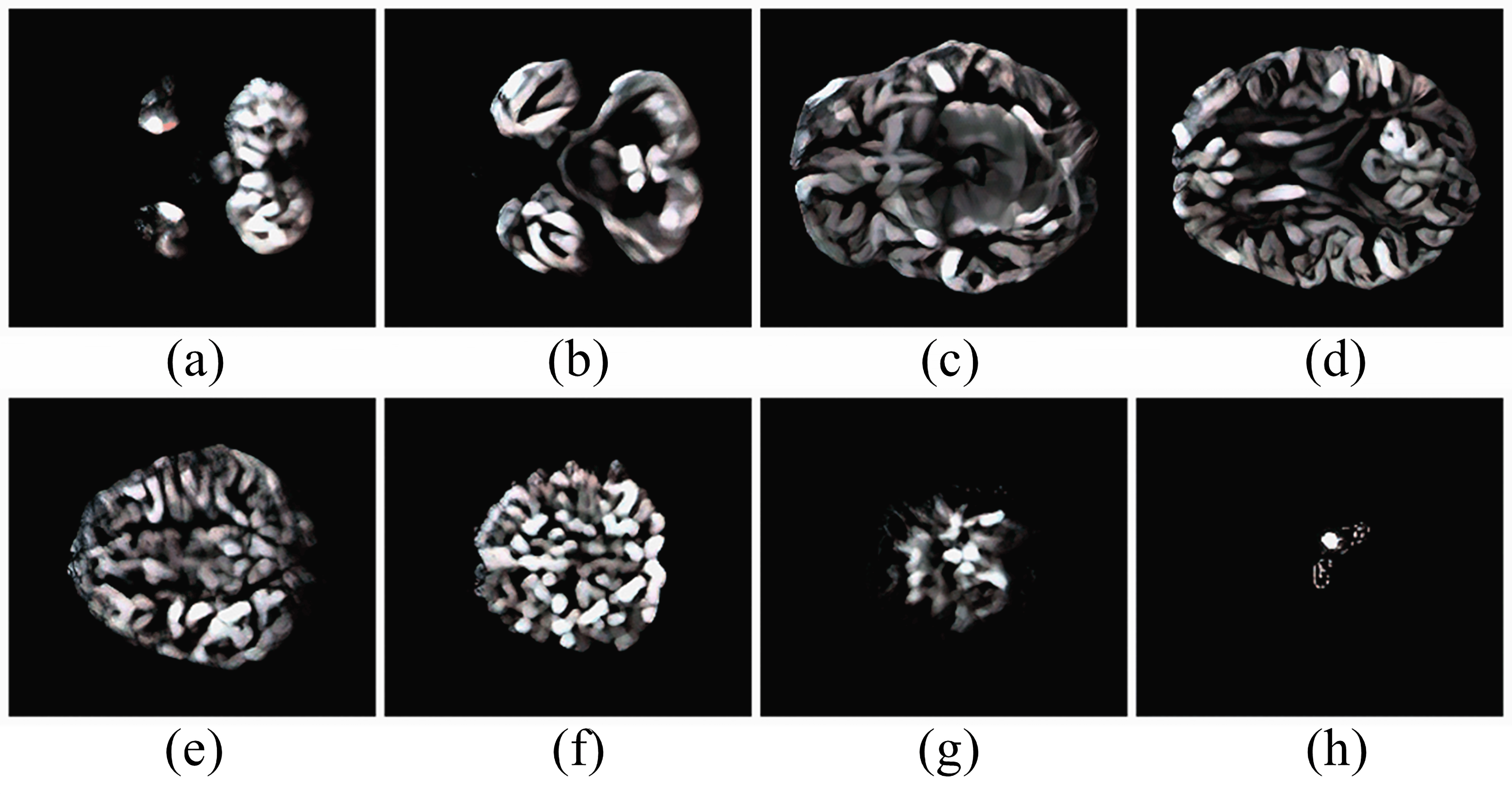