- Academic Editor
†These authors contributed equally.
Purpose: Current evidence suggests that phosphoserine aminotransferase 1 (PSAT1) is overexpressed in various tumors. Herein, we investigate the significance of PSAT1 in non-small cell lung cancer (NSCLC) and its correlation with immune infiltration. Methods: The expression profile of PSAT1 in NSCLC patients and related clinical information was obtained from the Gene Expression Omnibus (GEO) and The Cancer Genome Atlas (TCGA-NSCLC) databases. In silico and experimental validation were conducted to assess the role of PSAT1 in NSCLC. Gene set enrichment analysis (GSEA) was performed to investigate the disparities in biological functions between groups with high and low PSAT1 expression. Additionally, the biological characteristics and immune cell infiltration were compared between these two groups. We also assessed whether PSAT1 expression could predict the sensitivity of NSCLC patients to immunotherapy using the immunophenotype score (IPS) and an anti-PD-L1 immunotherapy cohort (IMvig-or210). Furthermore, the difference in drug sensitivity between PSAT1-high and PSAT1-low expression cell lines was investigated. Results: Analysis of transcriptional expression profiles using TCGA data revealed overexpression of PSAT1 in NSCLC tissues correlated with poor overall survival (OS). GSEA results showed enrichment of DNA recombination and repair, nucleotide biosynthesis, and the P53 signaling pathway in the PSAT1-high group. Experimental validation demonstrated that the knockdown of PSAT1 suppressed cell proliferation, migration, and invasion of NSCLC. Immune cell infiltration analysis revealed an immune-activated tumor microenvironment in the PSAT1-low group. It was also observed that PSAT1-low cell lines were more likely to benefit from immunotherapy and several chemotherapy drugs. Conclusions: PSAT1 has enormous potential for applications in the prediction of NSCLC patient outcomes and provides the foothold for more precise individualized treatment of this patient population.
Current evidence suggests lung cancer is the most prevalent cancer type, accounting for 1.6 million annual deaths worldwide [1]. The majority, approximately 85%, of lung cancer-related deaths are attributed to non-small cell lung cancer (NSCLC), which primarily consists of two main subtypes: lung adenocarcinoma (LUAD) and lung squamous cell carcinoma (LUSC) [2]. Although the past few years have witnessed significant inroads in NSCLC management, the prognosis for NSCLC remains dismal [3]. Therefore, identifying new prognostic markers is crucial to refining current screening approaches and improving the prognosis of this patient population.
Immune-checkpoint inhibitors (ICI), specially programmed cell death protein-1 (PD-1) inhibitors, have changed the treatment of NSCLC over the past decade, significantly improving the overall survival rate (OS) [4]. Unfortunately, not all patients will respond well to ICI treatment, and severe side effects may occur [5, 6, 7]. To date, PD-L1 alone is the only recognized prognostic biomarker for ICI treatment in NSCLC, assessed by immunohistochemistry (IHC) [8]. However, it cannot accurately predict the effect of immunotherapy since pathologists cannot consistently read the expression of PD-L1 on immune cells, even with training [9, 10, 11]. Although tumor mutation burden (TMB) has recently become another potential indicator of immunotherapy response, existing data shows its limitations [12]. These findings overlap in their assertion of the need to identify new markers to accurately predict the efficacy of immunotherapy.
It is now understood that phosphate aminotransferase 1 (PSAT1) is a rate-limiting enzyme in serine and glycine synthesis essential for malignant cell growth [13]. Previous studies have found that most human tumors overexpress PSAT1 and are associated with poor prognosis [14]. Upregulation of PSAT1 expression has been associated with the advancement of NSCLC by suppressing cyclin D1 degradation and stimulating cell proliferation [15, 16]. However, the effect of PSAT1 on the immune microenvironment in tumors and its correlation with immunotherapy response in NSCLC patients remain underexplored.
Herein, we assess PSAT1 levels in NSCLC tissues and their relationship with prognosis. Our experimental findings reveal that silencing PSAT1 suppresses the proliferation, migration, and invasion of NSCLC cell lines. Moreover, we examine the relationship between PSAT1 expression levels and immune infiltration in the tumor microenvironment (TME) of NSCLC, which reveals that PSAT1 overexpression may suppress anti-tumor immune activity. Finally, we identify the potential of PSAT1 expression as a novel biomarker to guide more precise treatment strategies involving immunotherapy and chemotherapy for this patient population.
The expression profile of PSAT1 in NSCLC patients and corresponding clinical data were collected from Gene Expression Omnibus (GEO) datasets (https://www.ncbi.nlm.nih.gov/) [17] and The Cancer Genome Atlas (TCGA-NSCLC) (https://portal.gdc.cancer.gov/) [18] datasets.
TCGA-NSCLC cohorts were examined for PSAT1 mRNA expression levels and divided into two groups (PSAT1-high and PSAT1-low group) based on the median expression. Then, the relationship between the PSAT1 expression profile and TCGA-NSCLC was analyzed. In addition, the Kaplan–Meier Plotter tool (https://kmplot.com/analysis/) [19] was applied to validate the impact of PSAT1 on the OS of NSCLC patients in three independent GEO datasets (GSE37745, GSE19188, and GSE30219).
To explore the different biological functions and pathways between the
PSAT1-high and PSAT1-low groups, Gene Ontology (GO) and Kyoto
Encyclopedia of Genes and Genomes (KEGG) enrichment analysis were conducted using
gene set enrichment analysis (GSEA) [20]. Using the threshold at p
A total of 24 paired samples consisting of tumor and normal tissue obtained from patients that underwent lung cancer surgery at Sun Yat-sen University Cancer Hospital were included in the experimental analysis. Detailed information regarding these samples can be found in Supplementary Table 1. This study was carried out following ethical guidelines and was endorsed by the Ethics Committee of Sun Yat-sen University Cancer Hospital (Approval No. YB2018-85).
The expression profiles of PSAT1 were assessed by quantitative
real-time PCR (qRT-PCR). Total RNA was isolated from cancer tissue samples and
adjacent normal tissue samples with TRIzol (TIANGEN, Beijing, China).
PrimeScript
Two human-derived NSCLC cell lines (H1299 and PC9) were used in this study,
purchased from the Xinyuan Biotech Co. Ltd. (Shanghai, China), and then frozen
and stored in liquid nitrogen. Genotype was determined by STR DNA testing and was
free of bacterial, mycoplasma, and fungal contamination. DMEM (Gibco, Grand
Island, NY, USA) containing 10% fetal bovine serum (Sigma, USA) and 5% CO
The Cell Counting Kit-8 (CCK-8) from JingXin Biological Technology in Guangzhou,
China, was utilized to measure cell proliferation. Cell suspensions of H1299 and
PC9 cells were prepared at a density of 5
For in vitro migration assays, Transwell plates with 8-µm pores
(Corning, NY, USA) were used. A total of 5
Tumor Immune Single Cell Hub (TISCH) (http://tisch.comp-genomics.org) is a database focusing on single-cell RNA sequencing in tumor microenvironments (TME) [21]. In this study, the TISCH database was used to systematically study the expression of PSAT1 in various cell types of NSCLC. This study utilized the TISCH database to analyze the expression of PSAT1 across different cell types of NSCLC.
Using the “GSVA” package, 28 immune cells were scored in GSE37745 using single set gene set enrichment analysis (ssGSEA) [22]. In addition, the “estimate” package was used to calculate the tumor purity and TME score of each patient (including the ESTIMATE score, immune score, and stromal score) [23].
We downloaded immunophenoscores (IPS) from The Cancer Immunome Atlas (TCIA, https://www.tcia.at/) database [24] for TCGA-NSCLC patients, which is an indicator of the sensitivity of NSCLC patients to immunotherapy, including anti-PD-1 and CTLA-4 therapy. In addition, we used another immunotherapy dataset to assess the predictive immunotherapy sensitivity of PSAT1, including 173 patients with advanced clear-cell renal cell carcinoma (ccRCC) who received anti-PD-1 immunotherapy (Nivolumab) [25].
To evaluate the chemotherapy sensitivity differences between cells expressing high and low levels of PSAT1, the half-maximal inhibitory concentration (IC50) of commonly used drugs was determined using the “pRRophetic” package. This package utilizes the expression matrix and drug response data from the Cancer Genome Project (CGP) plan, which includes information on 138 anticancer drugs tested against 727 cell lines [26].
The gene expression data were subjected to analysis using the Student’s
t-test. The analysis of variance (ANOVA) test was employed to detect the
expression level of PSTA1 across different stages of lung cancer. The
Kaplan–Meier method was utilized to estimate OS. Spearman correlation analysis
was employed for all correlation analyses. Data processing and plotting in this
study were conducted using GraphPad Prism 8.0 (San Diego, California, USA).
Statistical significance was determined as a p-value
Analysis of the NSCLC database from TCGA revealed a significant increase in PSAT1 expression in tumor tissues compared to adjacent normal tissues (Fig. 1A,B). These findings were further confirmed by qRT-PCR analysis using 24 paired NSCLC samples obtained from our center (Fig. 1C). In addition, the relationship between PSAT1 expression and clinical stages of NSCLC patients from TCGA was analyzed, demonstrating that PSAT1 was significantly upregulated in T2/T3, N1, and stage II-IV (Fig. 1D–F). Finally, GSE37745, GSE19188, and GSE30219 datasets verified that NSCLC patients with high expression of PSAT1 displayed inferior OS (Fig. 1G–I).
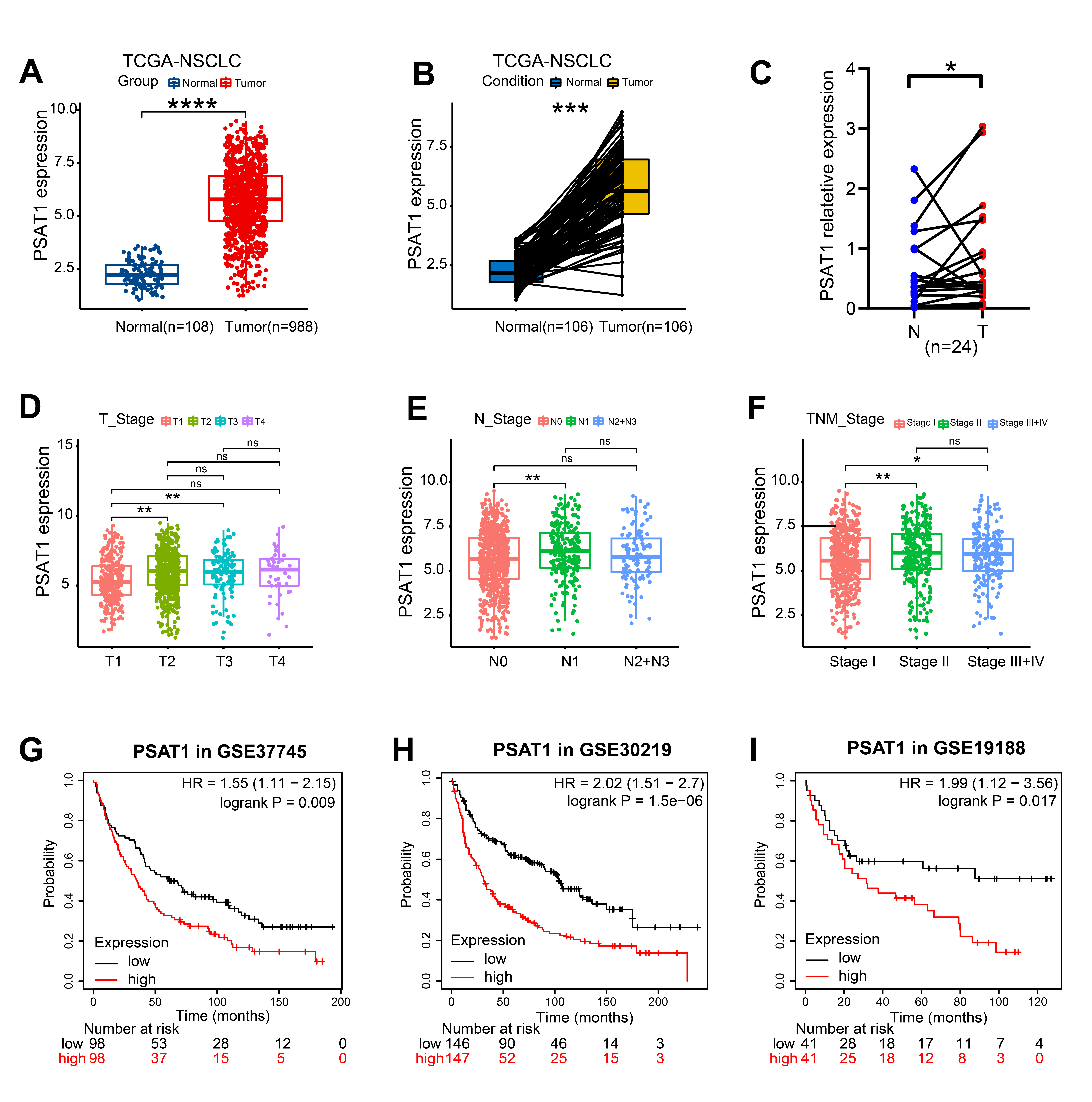
Characteristics of PSAT1 expression in NSCLC. (A)
Expression of PSAT1 in the TCGA-NSCLC cohort. (B) Expression of
PSAT1 based on the paired NSCLC data from TCGA. (C) qRT-PCR showed the
expression of PSAT1 in the tumor and adjacent normal tissue in NSCLC,
p
To investigate the potential mechanism of PSAT1 in NSCLC, GSEA was performed between PSAT1-high and PSAT1-low expression groups in 196 cases of NSCLC patients from GSE37745. GO annotation showed significant enrichment in biological processes, including DNA recombination and repair in the PSAT1-high group, whereas the immune response was significantly enriched in the PSAT1-low group (Fig. 2A,B and Supplementary Table 2). KEGG pathway analysis indicated that DNA nucleotide biosynthesis and P53 signaling pathway were enriched in the PSAT1-high group, whereas complement and coagulation cascades were enriched in the PSAT1-low group (Fig. 2C,D and Supplementary Table 3).
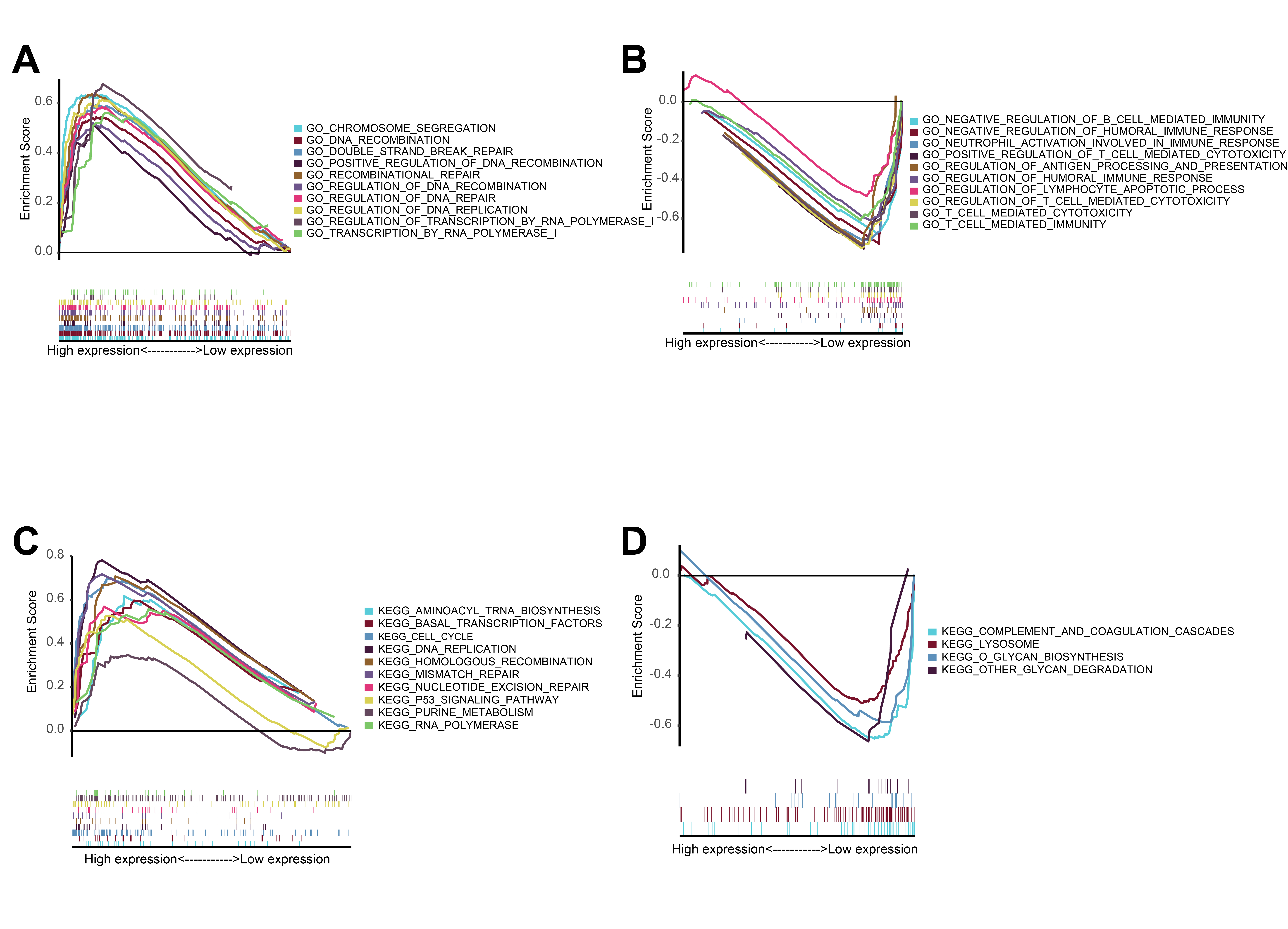
Gene set enrichment analysis between two PSAT1 subgroups. Enrichment of GO in the PSAT1-high group (A) and PSAT1-low group (B). Enrichment of KEGG in the PSAT1-high group (C) and PSAT1-low group (D). GO, Gene Ontology; KEGG, Kyoto Encyclopedia of Genes and Genomes.
In CCK8 assays, the suppression of PSAT1 resulted in significant inhibition of H1299 and PC9 cell proliferation (Fig. 3A,B). Moreover, the knockdown of PSAT1 demonstrated a significant reduction in the migration and invasion abilities of NSCLC cells (Fig. 3C–F). These findings strongly suggest that PSAT1 may promote NSCLC proliferation, migration, and invasion.
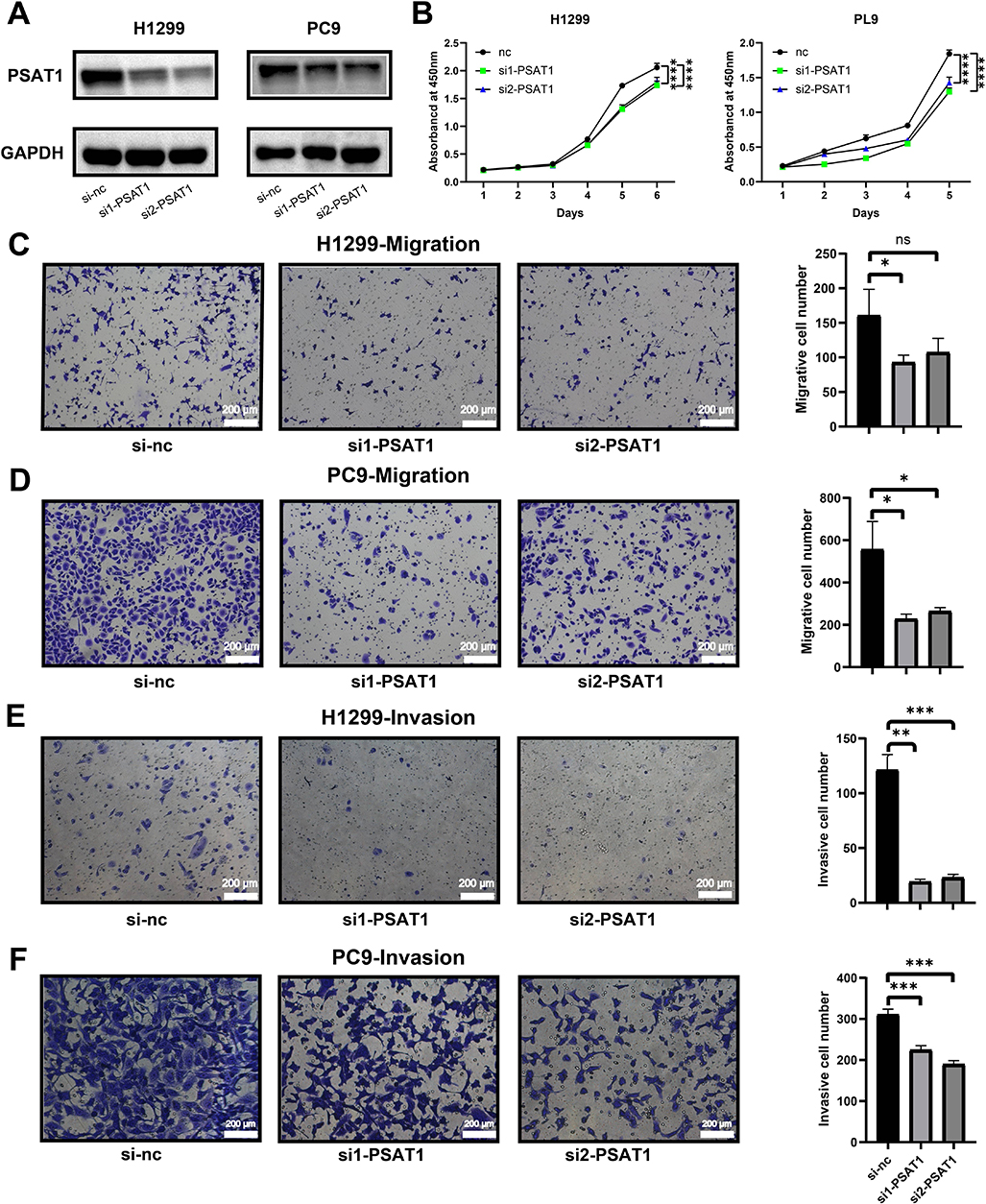
Experimental validation of the role of PSAT1
in NSCLC cells. (A) The knockdown efficiency by specific siRNA against
PSAT1 was confirmed by western blot. (B) The CCK8 assay measured the
proliferation ability of H1299 and PC9 cells after knocking down PSAT1.
H1299 and PC9 cell migration assay (C,D) and invasion assay (E,F) were performed
in control and si-PSAT1 groups. si-nc, blank control; si-PSAT1-1 and
si-PSAT1-2, knock down PSAT1 in NSCLC cells; ns, p
The expression of PSAT1 in the TME was analyzed using the dataset NSCLC_GSE148071 from the TISCH database, which revealed that PSAT1 was primarily expressed in malignant cells, with minimal expression in other cells (Fig. 4A,B).
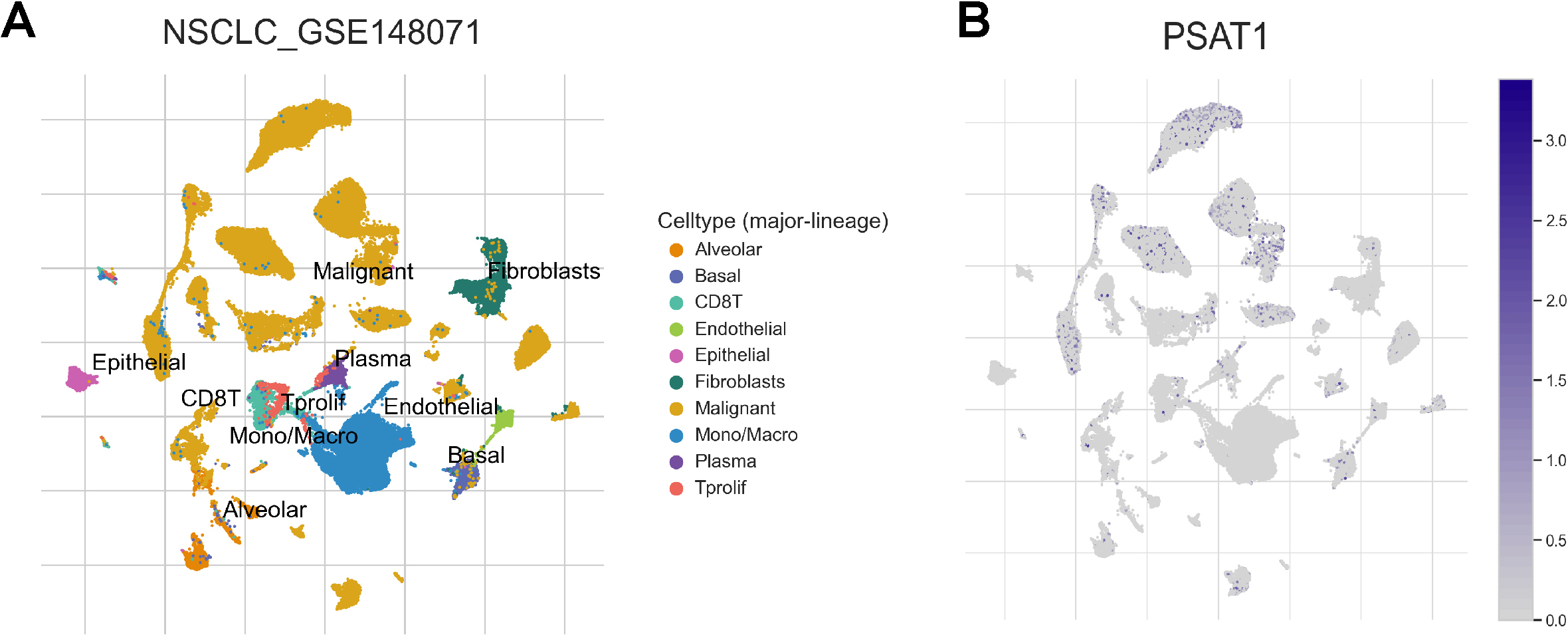
PSAT1 Expression in TME-associated cells from GSE148071. (A) Annotation of all cell types in GSE148071. (B) PSAT1 expression is primarily in malignant cells. TME, tumor microenvironments.
A total of 28 types of immune cells were assessed using ssGSEA for differences between groups with high and low PSAT1 expression. Heatmap analysis using dataset GSE37745 showed significant infiltration of 28 types of immune cells in the TME (Fig. 5A). The 21 types of immune cells exhibited significant differences in abundance between both groups (Fig. 5B). In addition, higher immune infiltration levels were observed in the PSAT1-low group. According to ESTIMATE analysis, the PSAT1-low group revealed higher immune and stromal scores, whereas the PSAT1-high group displayed higher tumor purity scores (Fig. 5C–E). Collectively, these results highlight a better prognosis for the PSAT1-low group.
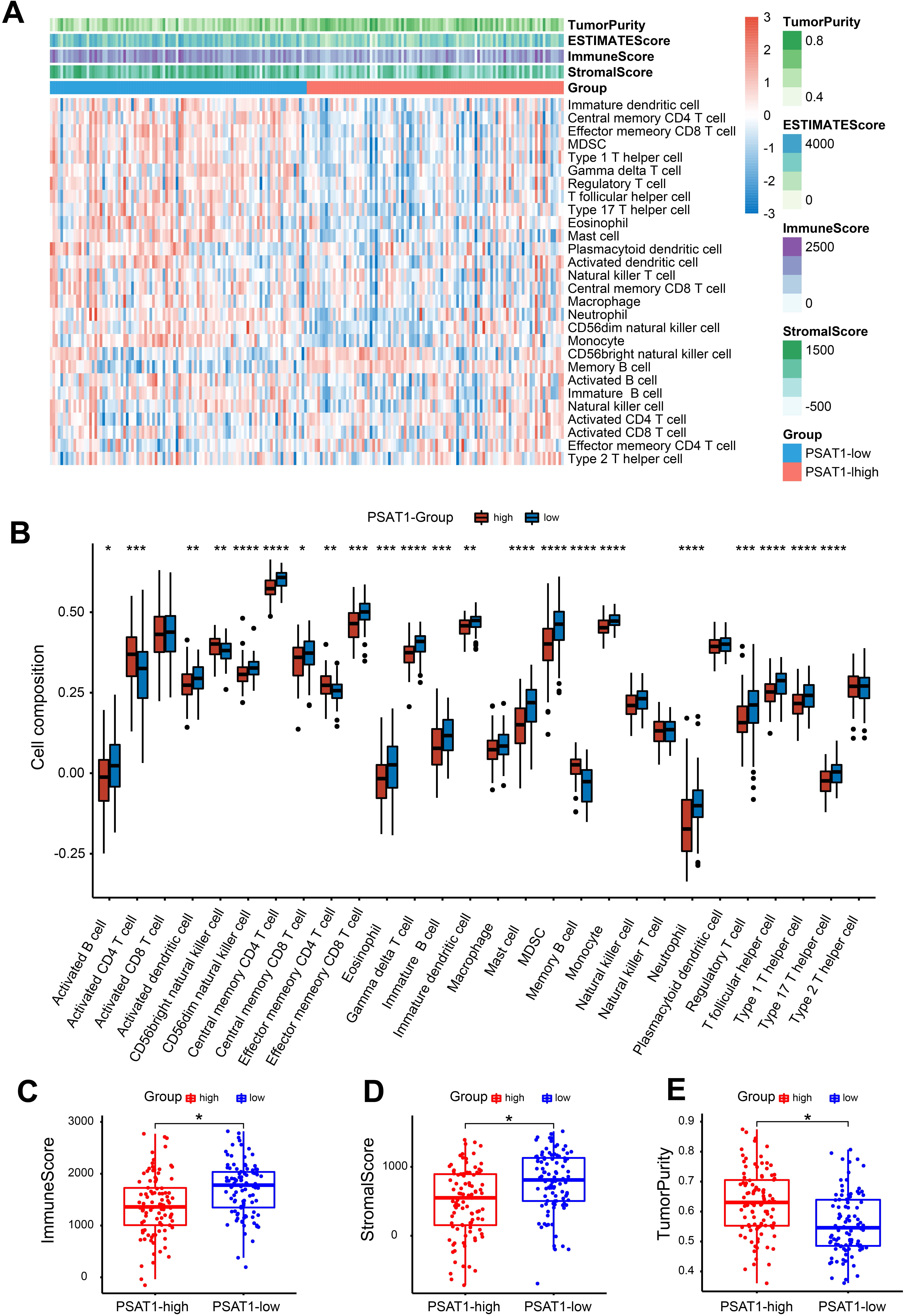
Analysis of TME cell infiltration. (A) Heatmap showing ssGSEA
scores, ESTIMATE score, immune score, stromal score, and tumor purity between
PSAT1-high and PSAT1-low group. (B) The differences in the
proportions of 28 immune cells between PSAT1-high and PSAT1-low
groups. Differences in the immune score (C), stromal score (D), and tumor purity
(E) between PSAT1-high and PSAT1-low groups. *p
The differences in the levels of 10 immune checkpoints were compared between groups with high and low PSAT1 expression to assess the possible correlation with immunotherapy. The results demonstrated that the PSAT1-high group was associated with significant upregulation of PD-L1, CD276, and PD-L2 in contrast with the PSAT1-low group (Fig. 6A). To investigate the relationship between PSAT1 expression and immunotherapy sensitivity, we recruited an anti-PD1 treatment cohort of ccRCC patients stratified into groups with high and low PSAT1 using the median expression of PSAT1 as the cutoff. Finally, the PSAT1-low group had relatively higher rates of complete or partial response (CR/PR), clinical benefit or no change (CB/NCB), and immune checkpoint blockade response or no change (ICB/NCB) than the PSAT1-high group (Fig. 6B,C). Similarly, downregulated expression of PSAT1 correlated with superior outcomes (Fig. 6D). In conclusion, it can be inferred that PSAT1 can be harnessed to predict the efficacy of immunotherapy.
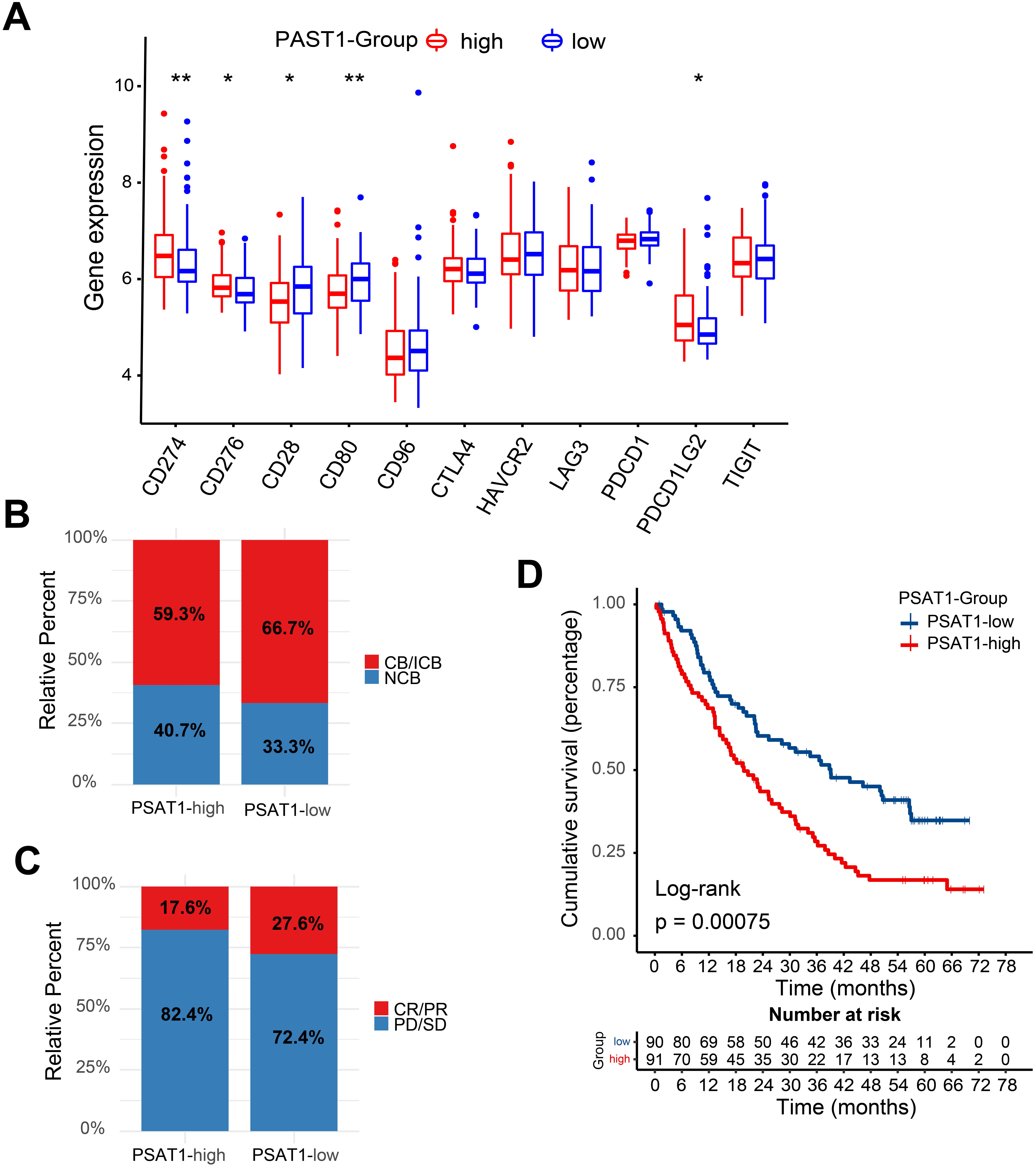
The predictive value of PSAT1 for
immunotherapy response in NSCLC patients. (A) Expression of 10 immune
checkpoints between high- and low-risk groups. (B,C) Proportions of anti-PD-L1
immunotherapy response in high and low PSAT1 score groups. (D) KM survival analysis in anti-PD-1 immunotherapy cohort of NSCLC. The
median value is represented by the line in the box. *p
Drug therapy remains the standard of care for patients with late-stage NSCLC. However, drugs are often empirically selected. In this study, we studied the relationship between the expression of PSAT1 in various cell lines and sensitivity to multiple chemotherapy drugs using CGP data. It was found that the PSAT1-low group was more sensitive to cisplatin, docetaxel, etoposide, gemcitabine, paclitaxel, vinblastine, gefitinib, and vinorelbine (Fig. 7A–H). However, the PSAT1-high group showed better sensitivity to lapatinib and erlotinib (Fig. 7I–J), suggesting that PSAT1 expression can be used to guide drug selection.
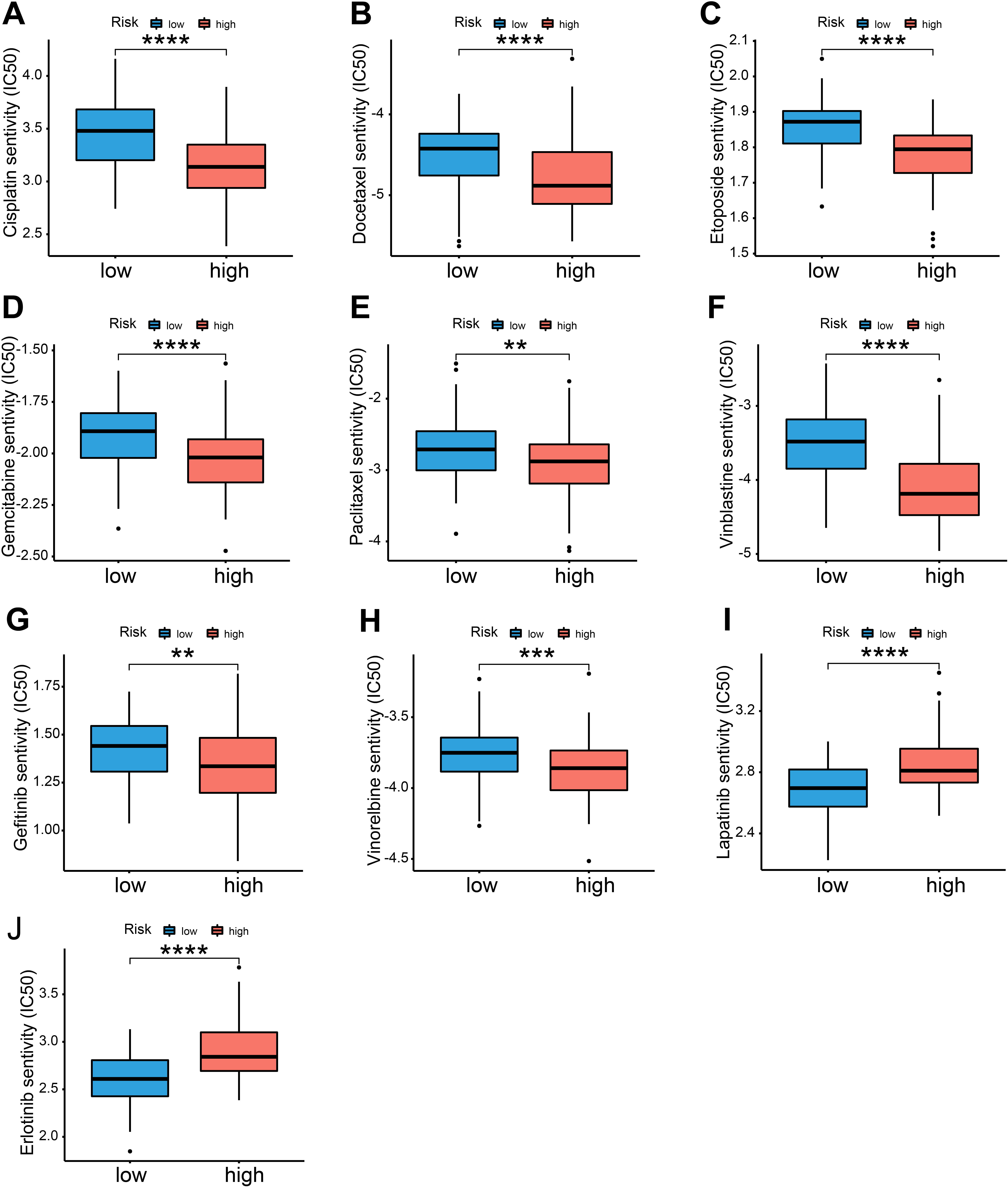
PSAT1 expression is associated with sensitivity to (A)
Cisplatin. (B) Docetaxel. (C) Etoposide. (D) Gemcitabine. (E) Paclitaxel. (F)
Vinblastine. (G) Gefitinib. (H) Vinorelbine. (I) Lapatinib. (J) Erlotinib.
**p
The crucial role of PSAT1 as a key enzyme in serine and glycine
synthesis has been well-established. Excessive activation of the serine/glycine
metabolic pathway is known to potentially promote oncogenesis by supporting cell
cycle progression [15, 27, 28]. An increasing body of evidence suggests
PSAT1 is essential in tumor progression and metastasis [29, 30].
Research by Sen et al. [31] and Zhang et al. [32] demonstrated
that inhibition of PSAT1 could promote DNA damage and apoptosis in Ewing
sarcoma (EWS) and epithelial ovarian cancer (EOC). PSAT1 has been shown
to facilitate lung adenocarcinoma progression via suppressing the
IRF1-IFN
To explore the functional significance of endogenous PSAT1 in NSCLC cells, we employed siRNAs to silence PSAT1 expression in H1299 and PC9 cells. CCK8 and Transwell assays revealed that the knockdown of PSAT1 suppressed NSCLC proliferation, migration, and invasion, consistent with the literature [15, 16], further supporting the notion that PSAT1 holds promise as a potential biomarker in NSCLC.
In addition, we found that the expression levels of immune checkpoint molecules, including PD-L1, PD-L2, and CD276, were significantly upregulated in the PSAT1-high group. However, we also found that the PSAT1-high group shows lower immune cell infiltration, with lower CR/PR, CB/NCB, and ICB/NCB rates for immunotherapy than the PSAT1-low group. This confusing result may be due to the fact that PD-1 and PD-L1 are not gold standard markers for predicting the effectiveness of immunotherapy. Our findings indicate that PSAT1 upregulation in NSCLC is associated with reduced response to immunotherapy.
Given that many NSCLC patients do not respond to targeted therapy and immunotherapy, conventional drug therapy remains the primary treatment for advanced cases. Our study also investigated the relationship between PSAT1 expression and multiple drugs commonly used in NSCLC treatment. Interestingly, we found that NSCLC patients in the PSAT1-high group exhibited increased sensitivity to drugs such as lapatinib and erlotinib. These findings suggest that PSAT1 expression may help guide treatment strategies for this patient population.
This study has several limitations that should be acknowledged. Firstly, the lack of NSCLC patients who received immunotherapy limits our ability to validate the predictive value of PSAT1 in immunotherapy response. Further prospective studies involving NSCLC patients undergoing immunotherapy are required to validate the prognostic value of PSAT1. Secondly, further in vivo, and in vitro experiments are necessary to understand better the precise mechanism through which PSAT1 modulates immune infiltration in NSCLC.
This study presents strong evidence of PSAT1 overexpression in NSCLC, correlating with an unfavorable prognosis. Moreover, our findings highlight the significant involvement of PSAT1 in immune cell infiltration, thereby suggesting its potential as a valuable indicator for predicting responses to both immunotherapy and chemotherapy.
We acknowledge TCGA and GEO database for providing their platforms and contributors for uploading their meaningful datasets.
HL, CW, WC, SM and YC contributed to the study design. HL, CW, WC, LZ, WG, MZ and ZW contributed to literature search. HL, CW, LZ, WG, MZ and ZW contributed to collecting cancer tissue and experimental validation, HL and WC wrote the article and performed data analysis. YC, SM and ZW contributed to edit, supervision and funding acquisition. All authors contributed to editorial changes in the manuscript. All authors read and approved the final manuscript. All authors have participated sufficiently in the work to take public responsibility for appropriate portions of the content and agreed to be accountable for all aspects of the work in ensuring that questions related to its accuracy or integrity.
All procedures performed in this study involving human material were in accordance with the ethical standards of the Sun Yat-sen University Cancer Center ethics committee (YB2018-85) and with the 1964 Helsinki Declaration and its later amendments or comparable ethical standards. For this type of study, formal consent is not required, as many of them were dead when the study was initiated. This article does not contain any studies with animals performed by any of the authors.
We sincerely thank Ms. Baolian Qiu for helping to edit this article.
This research was funded by the National Natural Science Foundation of China (NO.81871986 and NO.81772884).
The authors declare no conflict of interest.
Publisher’s Note: IMR Press stays neutral with regard to jurisdictional claims in published maps and institutional affiliations.