- Academic Editor
Background: Emotions are thought to be related to distinct patterns of neural oscillations, but the interactions among multi-frequency neural oscillations during different emotional states lack full exploration. Phase-amplitude coupling is a promising tool for understanding the complexity of the neurophysiological system, thereby playing a crucial role in revealing the physiological mechanisms underlying emotional electroencephalogram (EEG). However, the non-sinusoidal characteristics of EEG lead to the non-uniform distribution of phase angles, which could potentially affect the analysis of phase-amplitude coupling. Removing phase clustering bias (PCB) can uniform the distribution of phase angles, but the effect of this approach is unknown on emotional EEG phase-amplitude coupling. This study aims to explore the effect of PCB on cross-frequency phase-amplitude coupling for emotional EEG. Methods: The technique of removing PCB was implemented on a publicly accessible emotional EEG dataset to calculate debiased phase-amplitude coupling. Statistical analysis and classification were conducted to compare the difference in emotional EEG phase-amplitude coupling prior to and post the removal of PCB. Results: Emotional EEG phase-amplitude coupling values are overestimated due to PCB. Removing PCB enhances the difference in coupling strength between fear and happy emotions in the frontal lobe. Comparable emotion recognition performance was achieved with fewer features after removing PCB. Conclusions: These findings suggest that removing PCB enhances the difference in emotional EEG phase-amplitude coupling patterns and generates features that contain more emotional information. Removing PCB may be advantageous for analyzing emotional EEG phase-amplitude coupling and recognizing human emotions.
Emotion is an integrated psychophysiological state that comprises an individual’s psychological and physiological reaction to external stimuli [1]. Previous research indicates that certain regions of the brain, including dorsolateral prefrontal lobe [2], amygdala [3], and hippocampus [4], are the crucial areas responsible for emotions. Brain signals can be acquired to capture information regarding emotional states [5]. Electroencephalogram (EEG) is a non-invasive method of recording neural signals, which provides high temporal resolution and is preferred for estimating emotional states [6]. Human emotion is a complex psychological and physiological phenomenon [7], and the non-linear, non-stationary properties of EEG signals further amplify the challenge of extracting meaningful information [8]. Therefore, ascertaining the relationship between EEG pattern variations and emotional fluctuations constitutes a major research challenge within the realm of emotional EEG.
EEG signals have three fundamental attributes: phase, amplitude, and frequency [9]. In prior research, emotional EEG signals have been analyzed mainly using mean square amplitude [10], power spectrum [11], time-frequency spectrum [12], phase-locking value [13], and other methods [14]. However, there are few studies on the interactive relationship of phase and amplitude between different frequencies within single-channel emotional EEG. The interaction between neuronal oscillations at different frequencies is known as cross-frequency coupling (CFC) [15]. Some researchers have found that CFC plays a functional role in transmitting information between neurons [16], and is associated with visual [17], auditory [18], and cognitive control [19]. Recently, CFC has also been applied in studying neurological and psychiatric diseases [20], such as Parkinson’s disease [21], epilepsy [22], schizophrenia [23] and obsessive-compulsive disorder [24]. Phase-amplitude coupling is the most typical type of CFC, in which the phase of the low-frequency signal modulates the amplitude of the high-frequency signal. Canolty et al. [25] discovered that phase-amplitude coupling exists in various regions of the brain. Phase-amplitude coupling has been proposed to have a crucial functional role in neural information processing and cognition [26]. In recent studies, researchers have tried to explore the relationship between emotion and phase-amplitude coupling. Bramson et al. [27] showed that enhanced phase-amplitude coupling can reduce neural noise in the anterior prefrontal cortex and sensorimotor cortex communication, allowing for improved control over emotional action tendencies. Chan et al. [28] found that distributed theta–gamma and alpha–gamma phase-amplitude coupling reveal implicit prosody processing associated with negative emotions. Additionally, Zhang et al. [29] suggested that phase-amplitude coupling is a potential feature for emotion owing to the nested nature of brain activities. Therefore, phase-amplitude coupling may be essential in revealing the physiological mechanisms that underlie emotional EEG data, thus contributing to the facilitation of emotion recognition.
Neural signals are not idealized sinusoidal waves [30]. The commonly used analytical technique of phase-amplitude coupling ignores the phase clustering bias (PCB) generated by the non-uniform distribution of phase angles [31]. This may lead to a widespread problem, underestimation or overestimation of genuine phase-amplitude coupling [31], where emotional EEG is no exception. Removing PCB can uniform the distribution of phase angles, but the effect of this approach is unknown on emotional EEG phase-amplitude coupling. In this study, the effect is explored through statistical analysis and classification. Here, it is hypothesized that removing PCB would produce a positive effect and that debiased phase-amplitude coupling (DPAC) might be advantageous in the study of multi-frequency interaction of emotional EEG and the recognition of human emotions.
In this study, experimental data were derived from the public emotional EEG dataset SEED-IV [32]. SEED-IV is an advanced version of the original SEED, and contains 62-channel EEG signals for four emotions recorded in15 subjects. Scalp voltages were recorded using the ESI NeuroScan System (SynAmps RT, Compumedics Neuroscan Inc., Charlotte, NC, USA) at a 200 Hz sampling rate. The SEED-IV dataset used audio-visual stimuli to elicit four target emotions: neutral, sad, fear, and happy. Subjects were instructed to watch the video clips and experience the corresponding emotions during each trial. Each subject completed three separate sessions, and the stimuli for the three sessions were completely different. A session comprised a total of 24 trials, encompassing six trials per emotion. The duration of each video clip was approximately two minutes, and each trial started with a 5 s hint and ended with a 45 s self-assessment and relaxation. To facilitate data analysis, we organized the four trials related to each of the four different emotions into a block. Hence each session consisted of six blocks.
Generally, unprocessed EEG signals contain electrical noises induced by facial muscle activity and eye movements. EEG signals were preprocessed with the open-source EEGLAB toolbox [33]. First, unnecessary channels were eliminated, and channel locations were edited. The CB1 and CB2 channels were removed due to their nonconformity with the international 10–20 system. Subsequently, the reference electrode standardization technique (REST) [34], a widely recommended reference method, was applied to re-reference EEG signals to the point at infinity. An finite impulse response (FIR) filter was then utilized for bandpass filtering in the 1–70 Hz range, and a 50-Hz notch filter was employed to eliminate power-line interference. Next, independent component analysis (ICA) was executed. Finally, ICLabel [35] and ADJUST (Automatic EEG artifact Detection based on the Joint Use of Spatial and Temporal features) [36] were utilized to remove independent components associated with blinks, eye movements, and electromyography (EMG). Wavelet packet transform was used to decompose the EEG signal of each channel into six sub-bands: Delta (1–4 Hz), Theta (4–8 Hz), Alpha (8–13 Hz), Beta (13–30 Hz), Low Gamma (30–45 Hz), and High Gamma (45–70 Hz). Following this, the 60-channel EEG signals of each frequency band were segmented into 5-second time windows to calculate the phase-amplitude coupling values. The window length of 5 s was determined by considering the maximum inclusion of training samples while capturing genuine phase-amplitude coupling values effectively. A study on the segmentation of EEG signal for classification was also referenced [37]. The Supplementary Material provided further evidence that a 5-second time window was reasonable.
The mean vector length (MVL) [38] is a regular method to quantify the coupling
strength between low-frequency signal phase and high-frequency signal amplitude,
as it is more sensitive to the coupling strength than other methods [39]. For the
original EEG signal filtered into low- and high- frequency signals denoted
as
Where
PCB is calculated by averaging the complex vectors of phase angles as shown in Eqn. 2:
Driel et al. [31] introduced a modified version of the regular MVL method, which can uniform the distribution of phase angles so as to debias MVL method. The debiased mean vector length (DMVL) is calculated using Eqn. 3.
PCB needs to be linearly subtracted from each phase angle before evaluating the phase-amplitude coupling.
In this study, the phase-amplitude coupling values were calculated using the regular MVL method (hereafter referred to as PAC) and modified version of the regular MVL method (hereafter referred to as DPAC).
For each channel, the PAC and DPAC values were calculated using formulas (1) and
(3), respectively, for each 5-s time window. These values were then averaged
across the time windows within each emotion for 15 subjects. The Wilcoxon signed
rank test was employed to compare the differences among the four emotions for PAC
and DPAC, as mentioned in the main text or figure legends. The 95% confidence
level threshold was set, and false discovery rate (FDR) was used to adjust the
p-value for multiple comparisons. “*” represents p
To further assess the effect of PCB on emotional EEG phase amplitude coupling, PAC and DPAC were used as features for emotion recognition, and their performance was compared. Support Vector Machine (SVM) is a binary linear classifier that distinguishes classes by finding hyperplanes that maximize the margins between two classes [40]. The LIBSVM toolbox (https://www.csie.ntu.edu.tw/~cjlin/libsvm/) is an integrated software that implements support vector classification, and supports multi-class classification [41]. SVM with a linear kernel function and a penalty factor C = 10 was applied to emotion recognition.
When evaluating performance, it is important to note that if the EEG signal of the entire session is randomly split into K folds, both the training and test sets may contain segments from the same trial. Information could be leaked from the training set to the test set due to the correlated data distribution of the segments from the same trial. This may result in an inflated recognition performance on the test set. To evaluate the classification results more reasonably, leave-one-block-out cross-validation method was adopted in this study, where one block refers to four trials of four different emotions.
The EEG signals possess a high dimensional feature space owing to the number of channels. Some features might have a small contribution to the classification task. To mitigate the risk of overfitting and to develop a simpler classification model, the Minimal-Redundancy-Maximal-Relevance (mRMR) [42] algorithm was utilized to rank features according to their importance to the four-class classification tasks. The most important M features were sequentially selected for emotion recognition, recording the classification accuracy for each frequency band pair.
The comodulogram, which depicts the strength of phase-amplitude coupling, is typically used to visualize the interaction between different frequency bands. The horizontal axis represents the phase frequency, while the vertical axis represents the amplitude frequency, and the color corresponds to the coupling strength.
To examine the coupling strength between low-frequency phase and high-frequency amplitude across all frequency bands during different emotional states, phases were extracted from 14 sub-bands within the frequency range of 1–29 Hz (with a bandwidth of 2 Hz and a step size of 2 Hz), and amplitudes from 17 sub-bands within the frequency range of 1–69 Hz (with a bandwidth of 4 Hz and step size of 4 Hz), as determined by the related work of comodulogram [21]. PAC and DPAC were calculated separately for all frequency pairs in each channel. The average comodulograms of four emotions were obtained by averaging the PAC and DPAC across 15 subjects within each emotion. From 60 channels, 60 groups of comodulograms were obtained.
As the frontal lobe is associated with emotions [43], comodulograms of channels in the frontal lobe were averaged. This process was then repeated for DPAC. Fig. 1 depicts the coupling patterns of neutral, sad, fear, and happy emotions. Specifically, the coupling patterns of neutral and happy emotions exhibited similarities, mainly manifesting in the phase frequency range of 1–5 Hz and the amplitude frequency range of 10–30 Hz. On the other hand, the two negative emotions, sad and fear emotions, displayed similar patterns, particularly in the phase frequency range of 1–5 Hz and the amplitude frequency range of 30–69 Hz. As seen from the bottom panel of Fig. 1, the regions of colored in red, which representing the stronger coupling in corresponding frequency ranges, exhibited smaller size when compared to those in the top panel of Fig. 1. This discrepancy can be attributed to the weaker coupling strength after removing PCB.
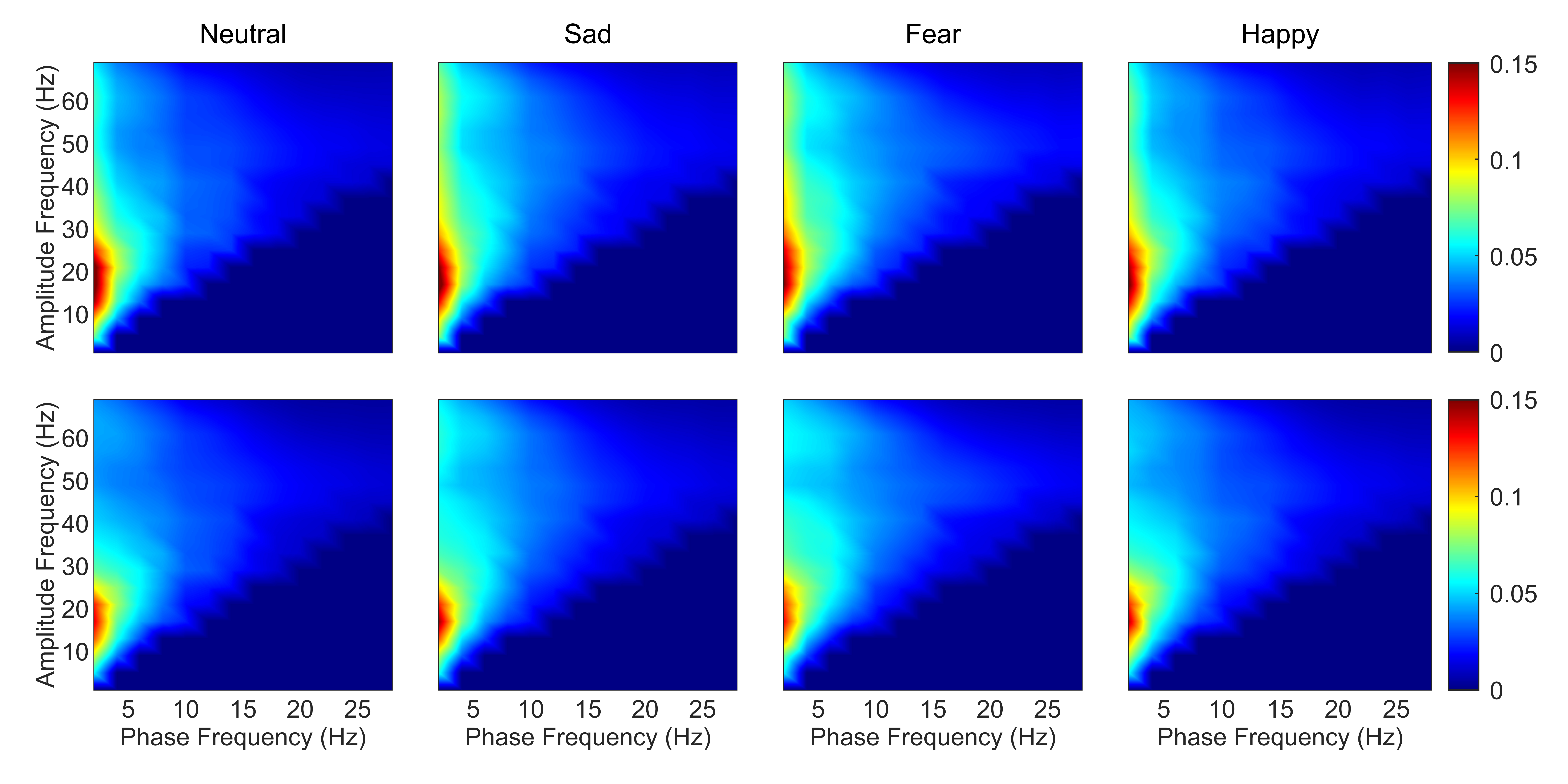
Average emotion comodulograms for channels in the frontal lobe (N = 15 subjects). The top panel shows PAC and the bottom panel shows DPAC. The title of each column gives the emotion. Color bar corresponds to the strength of coupling. Note the similar patterns between PAC and DPAC, and with removal of the phase clustering bias leading to weaker coupling strength. PAC, phase-amplitude coupling; DPAC, debiased phase-amplitude coupling.
As per the emotion comodulograms mentioned earlier, emotional EEG phase-amplitude coupling mainly occurs between six pairs of frequency band: Delta and Beta (D-B), Delta and Low Gamma (D-LG), Delta and High Gamma (D-HG), Theta and Low Gamma (T-LG), Theta and High Gamma (T-HG), and Alpha and High Gamma (A-HG). To further compare the phase-amplitude coupling of these six frequency band pairs, the PAC and DPAC were calculated for all EEG signal channels for the four emotions, and then averaged across channels. As shown in Fig. 2, DPAC and PAC had similar patterns for the four emotions in the six frequency band pairs. However, the Wilcoxon sign ranks test demonstrated that the DPAC values were significantly smaller than those of PAC (Z = 3.408, p = 0.001).
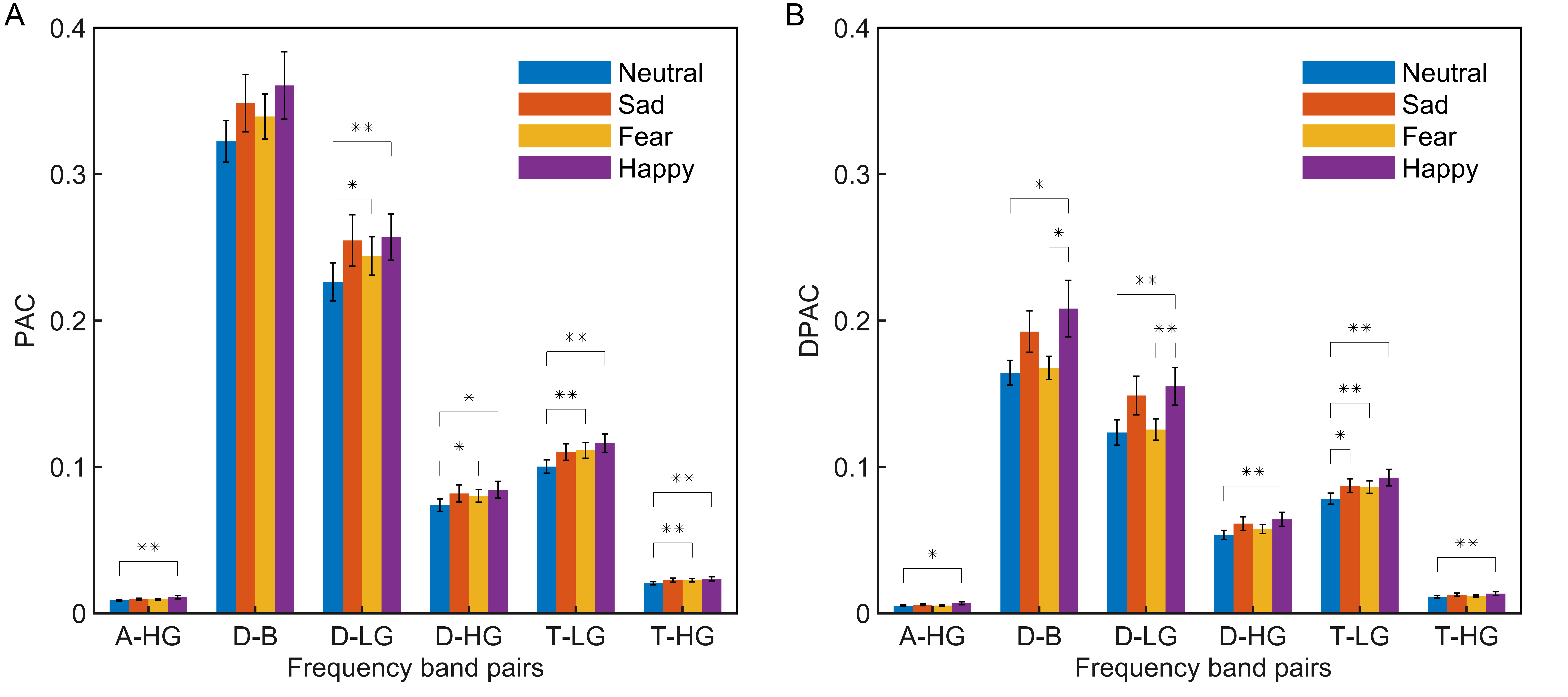
Statistical differences among emotions (neutral, blue; sad,
orange; fear, yellow; happy, purple) in the average of all channels (N =
15 subjects, **p
Furthermore, Wilcoxon signed rank tests showed that DPAC and PAC differed significantly between some emotions, as indicated by the significance markers shown in Fig. 2. In particular, after removing PCB, DPAC between fear and happy emotions (Z = 2.360, p = 0.018), as well as neutral and happy emotions (Z = 2.521, p = 0.012), in the D-B frequency band pair both showed significant differences.
As EEG signals have numerous channels the spatial distribution of phase-amplitude coupling strength on the scalp surface can be observed through brain topographic maps. With 60 EEG channels, average brain topographic maps were obtained across the 15 subjects. Figs. 3,4 present the brain topographic maps of PAC and DPAC for six frequency band pairs and four emotions, respectively. Each row denotes an emotion and each column represents a frequency band pair. PAC and DPAC are linearly mapped to the normal scale [0,1].
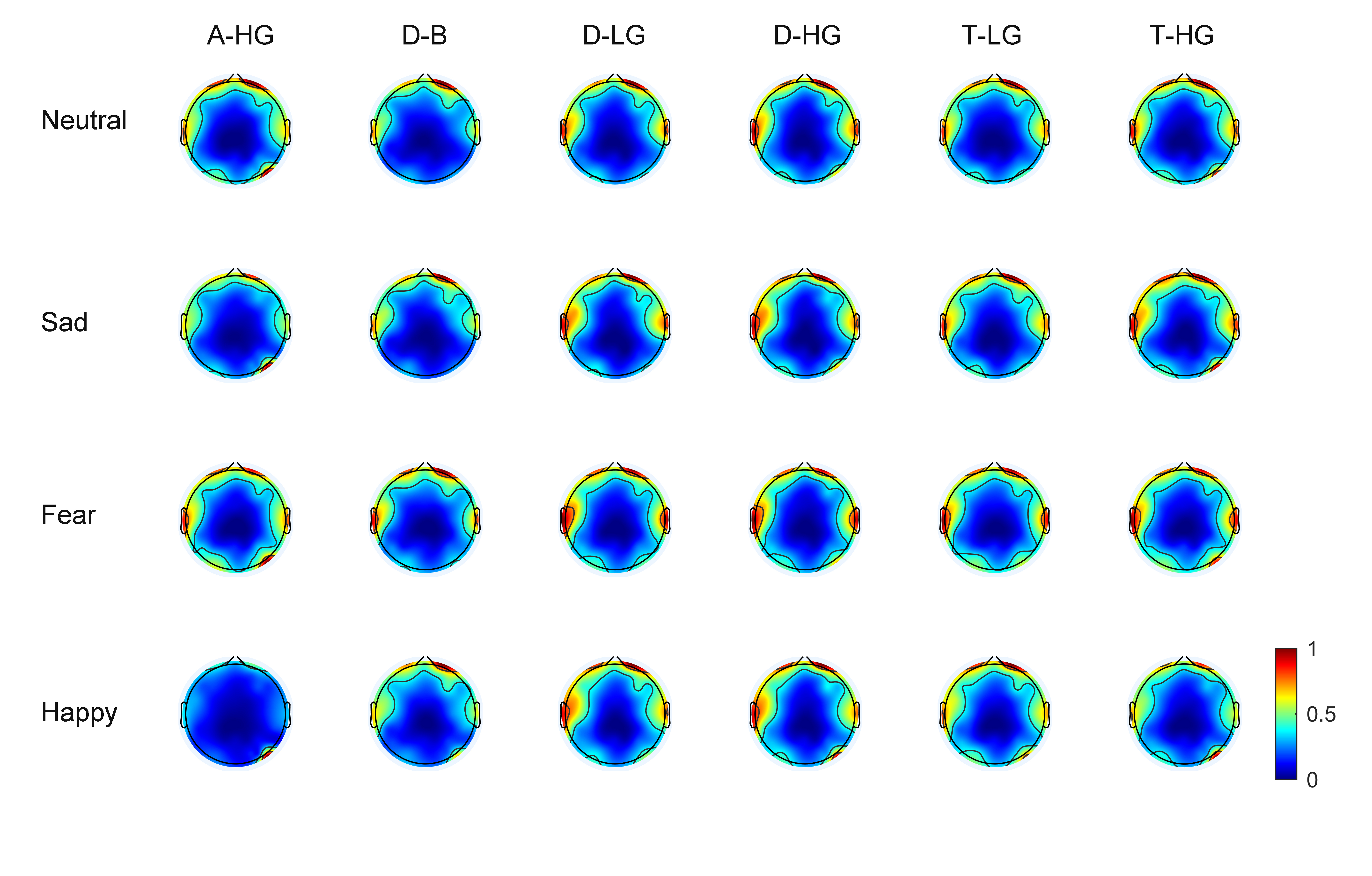
Topographic maps of PAC for 60 channels (N = 15 subjects). The color bars represent the phase-amplitude coupling value, which is linearly mapped to the normal scale [0,1].
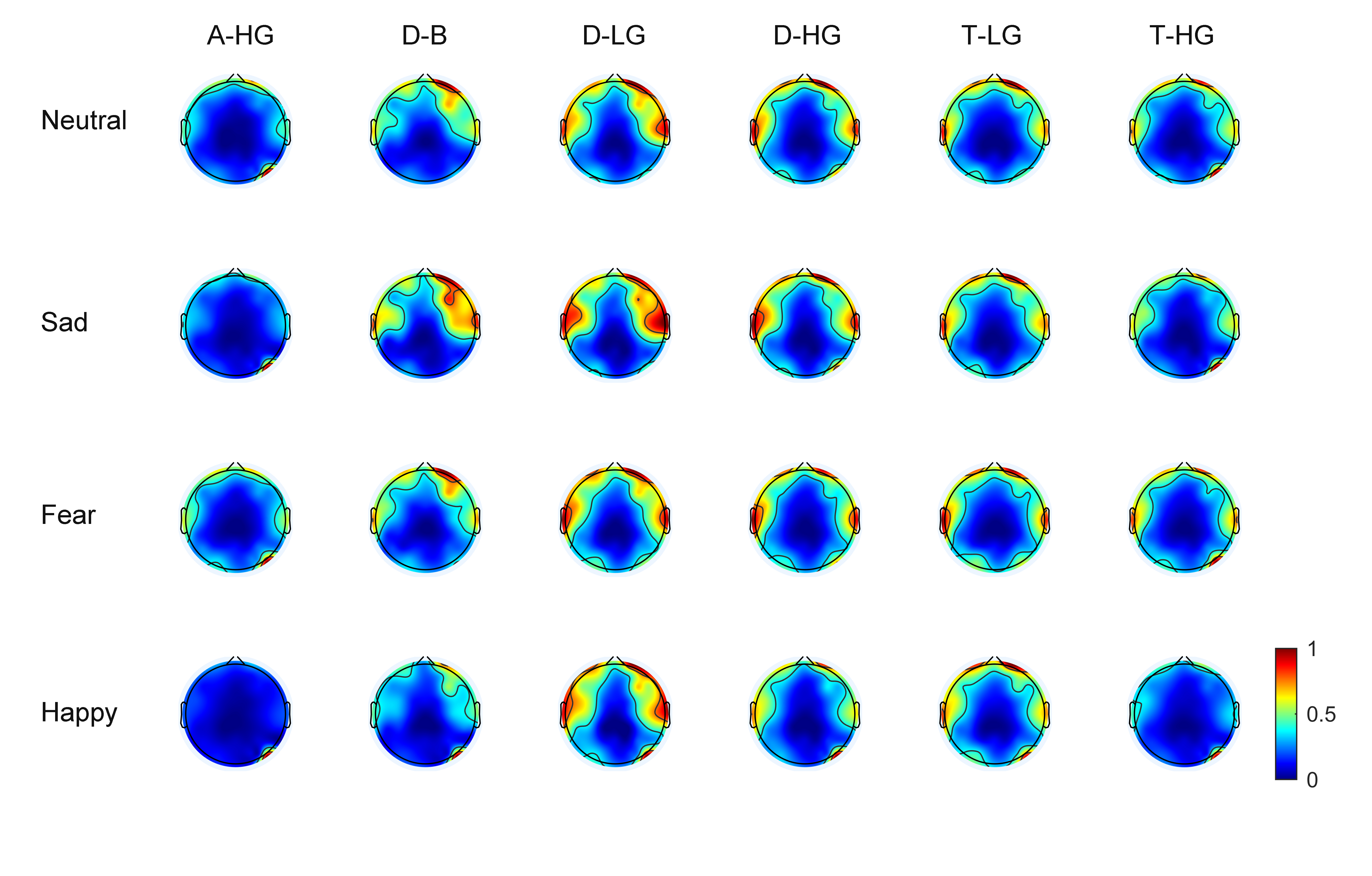
Topographic maps of DPAC for 60 channels (N = 15 subjects). The color bars represent the phase-amplitude coupling value, which is linearly mapped to the normal scale [0,1].
Figs. 3,4 reveal that PAC and DPAC are strong in both the prefrontal and temporal lobes, and also exist in the occipital lobe. The brain topographic maps of PAC and DPAC also appear to exhibit notable symmetry between the left and right hemispheres.
A comparison of Figs. 3,4 showed that the topographic maps of
phase-amplitude coupling exhibit greater differences among the four emotions and
the six frequency band pairs both prior to and post the removal of PCB. A
statistical analysis of the observed phenomena was also conducted. The difference
values of 60 channels between any two frequency band pairs were calculated under
each emotion. Similarly, the difference values of 60 channels between any two
emotions were calculated under each frequency band pair. For all these difference
values, the difference between PAC and DPAC were significant by the Wilcoxon
signed rank test (Z = 6.7359, p
Since the frontal lobe is frequently reported associated with emotional EEG in previous literature [44], PAC and DPAC of each subject’s frontal lobe channels (F8, F6, F4, F2, FZ, F1, F3, F5, F7) were averaged. Wilcoxon signed rank tests revealed the differences among the four emotions for six frequency band pairs. The significant test results are depicted in Fig. 5.
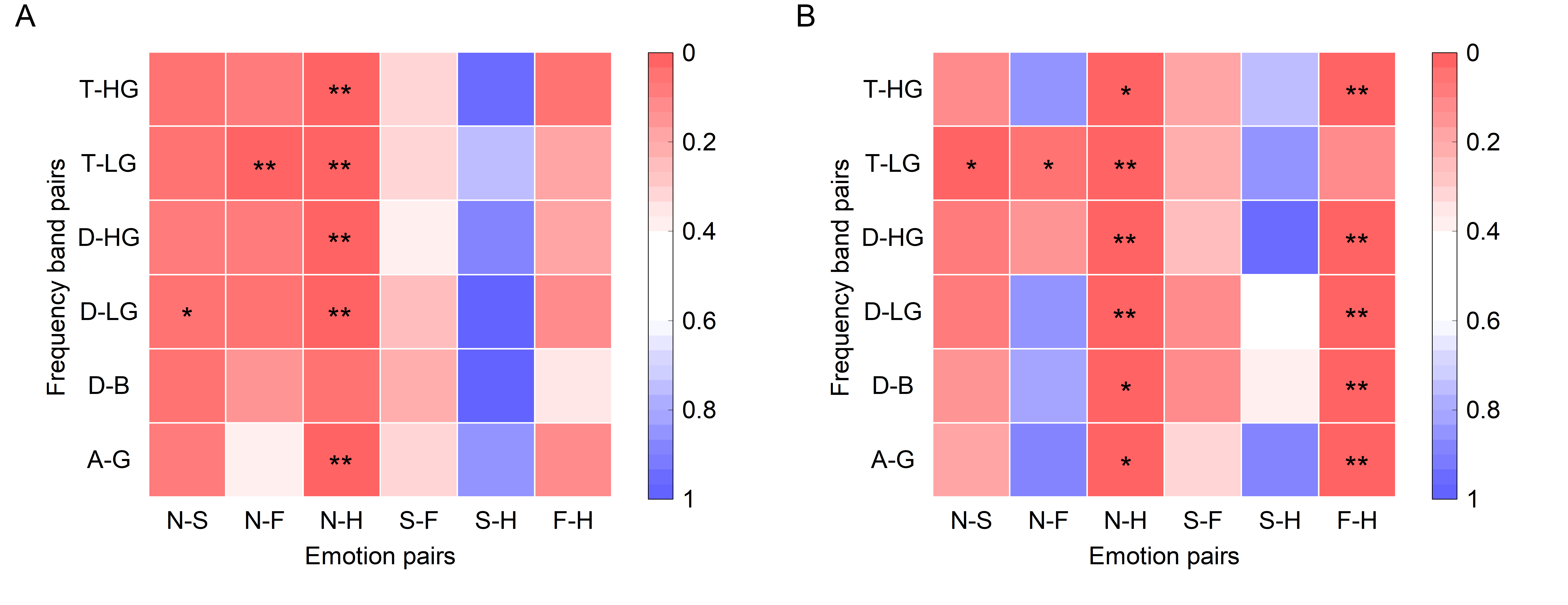
Statistical differences among emotions in the frontal lobe
(N = 15 subjects, **p
Results showed that removing PCB enlarged the difference in coupling strength between fear and happy emotions significantly, but seemingly reduced differences in coupling strength between neutral and fear emotions. Notably, a statistically significant difference existed in EEG phase-amplitude coupling in the frontal lobe between neutral and happy emotions, while the p-values are almost equal whether or not PCB was removed. The six p-values in the third column of PAC and DPAC were compared by the Wilcoxon signed rank test and the difference was not significant. This implies that the difference in EEG phase-amplitude coupling in the frontal lobe between neutral and happy emotions was not influenced by PCB (Z = 0.105, p = 0.916). After removing PCB, DPAC between fear and happy emotions (Z = 2.439, p = 0.015), as well as neutral and happy emotions, in the D-B frequency band pair showed significant differences (Z = 2.296, p = 0.022). Similar results were also obtained in Section 3.2.
The emotion recognition outcomes depicted in Fig. 6 present the average classification accuracy of 15 subjects as a function of feature numbers on the horizontal axis. The results indicated that with or without removal of the phase clustering bias, each frequency band pair exhibited a pattern of increasing initially and then decreasing classification accuracy as the feature numbers increased, which could be attributed to classifier overfitting due to the high number of feature dimensions.
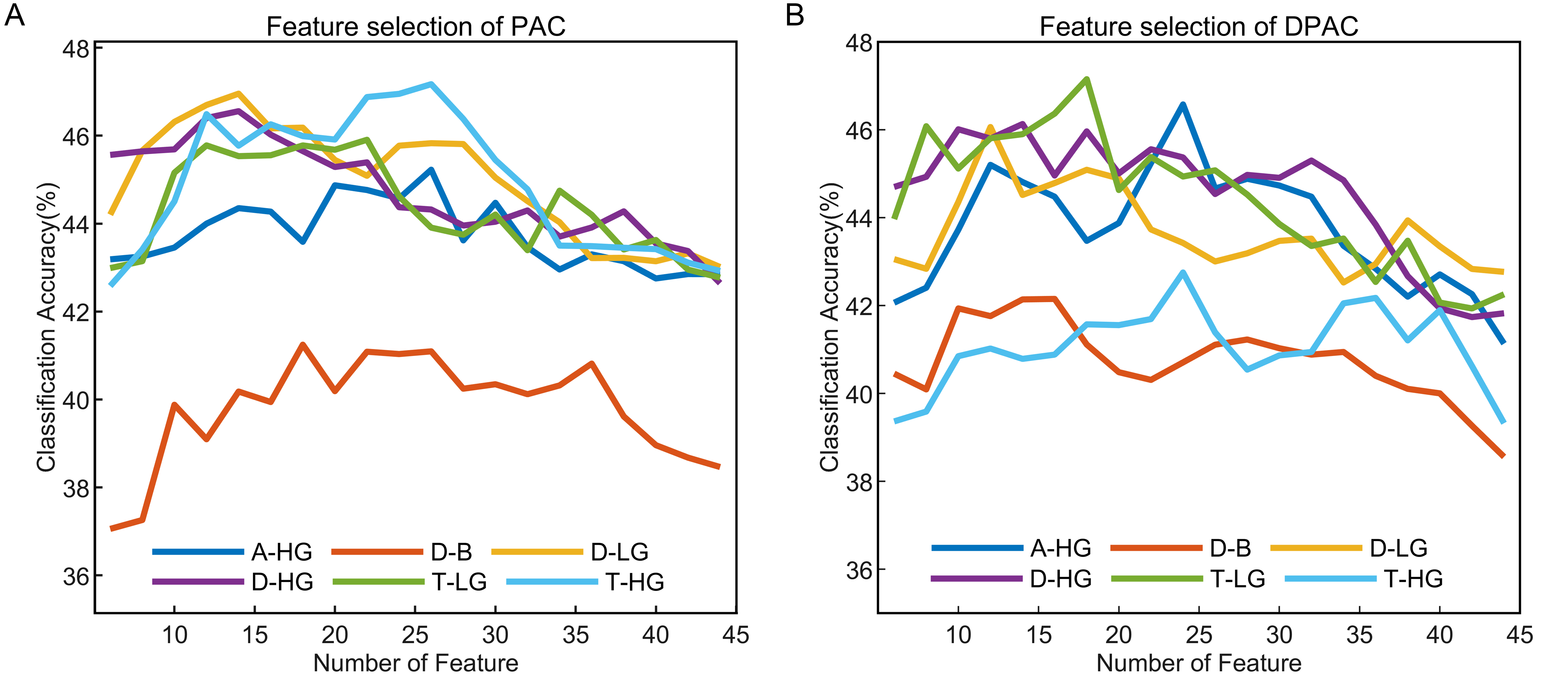
Emotion recognition performance of PAC and DPAC with different feature numbers. (A) PAC. (B) DPAC. The horizontal axis denotes the number of features, the vertical axis represents the average classification accuracy of 15 subjects.
The optimal number of features with the best classification accuracy of PAC vs. DPAC were 26 vs. 24 (A-HG), 18 vs. 16 (D-B), 14 vs. 12 (D-LG), 14 vs. 14 (D-HG), 22 vs. 18 (T-LG), and 26 vs. 24 (T-HG). Their corresponding accuracy is shown in Table 1. The performance in the four-class emotion recognition task is above the chance level (25%) for each subject. The Wilcoxon signed rank test revealed that the number of features with the best classification accuracy of PAC and DPAC were significantly different (Z = 2.121, p = 0.034), while the difference between their best classification accuracy was not statistically significant (Z = 0.210, p = 0.833). Furthermore, the top-ranked features for both PAC and DPAC were mainly from the prefrontal and lateral temporal lobes, especially FP2 and T7 channels, a phenomenon irrelevant to the subject. Table 1 also showed that D-LG and T-HG achieved higher classification accuracy among the six band pairs of PAC. A-HG and T-LG achieved higher classification accuracy among the six frequency band pairs of DPAC.
Feature | A-HG | D-B | D-LG | D-HG | T-LG | T-HG |
PAC | 45.23 |
41.25 |
46.96 |
46.56 |
45.91 |
47.17 |
DPAC | 46.57 |
42.15 |
46.06 |
46.13 |
47.15 |
42.75 |
PAC, phase-amplitude coupling using the regular MVL method; MVL, mean vector length; DPAC, debiased PAC.
Data are the mean
Phase-amplitude coupling is a promising tool for studying multiscale interactions in complex systems [45]. Although numerous studies have confirmed the correlation between emotions and EEG, few studies have focused on phase-amplitude coupling between different frequencies within single-channel emotional EEG as well as PCB caused by non-sinusoidal characteristics of EEG. This study explored the effect of PCB on phase-amplitude coupling for emotional EEG. Consistent with the hypothesis, removing PCB might produce a relatively positive effect.
The non-uniform distribution of phase angles may lead to either overestimation or underestimation of genuine phase-amplitude coupling, depending on the strength of the bias and the coupling angle [31]. Strong PCB that is in-phase with phase-amplitude coupling can result in overestimations of coupling strength, whereas anti-phase clustering-coupling angles may cause underestimations, as revealed by simulation results from [31]. The experimental results presented in Figs. 1,2 show a reduction of phase-amplitude coupling values was observed after removing PCB. In other words, emotional EEG exhibits a considerable PCB with an in-phase clustering-coupling angle.
Phase-amplitude coupling is a mechanism for neuronal communication, and the strength of phase-amplitude coupling between high-frequency activity and low-frequency activity varies across brain areas based on tasks [46]. The topographic maps depicted in Figs. 3,4 show that PAC and DPAC are strong in both the prefrontal and temporal lobes, and exist in the occipital lobe. These brain regions may be associated with the processing of emotions and exist cross-frequency information communication. Our findings reveal the differences of phase-amplitude coupling pertaining to emotional states in the frontal regions. A possible explanation is that the dorsolateral prefrontal and orbitofrontal areas are key locations responsible for emotions [47]. The amygdala, an almond-shaped structure in the medial temporal lobe, assumes a significant function in emotional functions [48]. The phase-amplitude coupling in the temporal regions may indicate the activity of the amygdala. Additionally, the auditory cortex, situated in the temporal lobe, is responsible for processing auditory information [49]. It is plausible that the temporal regions generate phase-amplitude coupling responses in reaction to auditory stimulus. The occipital regions, housing the visual cortices, are known for their role in visual information processing [50]. The processing of emotional visual stimulation necessitates the handling of visual perception information [51]. Seymour et al. [52] and Voytek et al. [53] demonstrated that the existence of phase-amplitude coupling in visual cortex of occipital regions during visual tasks. The presence of phase-amplitude coupling in the occipital regions likely indicates visual-induced emotional information processing. Evoked emotions are the brain’s response to audio-visual stimuli, thereby involving the sensory modalities integration of auditory and visual. Phase-amplitude coupling has been implicated in multisensory integration [54].
According to Russell’s Valence-Arousal emotion model [55], fear and happy emotions have opposite valence but similar arousal levels. After the removal of PCB, significant differences between neutral and happy, as well as fear and happy in the frontal lobe suggest that DPAC has the potential to differentiate emotional valence. This finding supports the study by Clerico et al. [56]. Happy emotion tends to motivate us to approach stimuli, while fear emotion motivates withdrawal. Davidson et al. [57] found that frontal EEG asymmetry is associated with approach and withdrawal emotions, with left frontal activity linked to approach tendencies and right frontal activity related to withdrawal tendencies. These findings suggest that accurate estimation of phase-amplitude coupling may amplify the difference in fear and happy emotion for these physiological characteristics. Frontal D-B phase-amplitude coupling has been found sensitive to mild state anxiety [58]. Topographic maps of DPAC in the D-B frequency band pair show more obvious asymmetry patterns of the left and right hemispheres than PAC, especially for sad and fear emotions. Since mild state anxiety also motivates withdrawal from stimuli, it is speculated here that spatial asymmetry distributions exist in the D-B frequency band pair and become more obvious due to PCB removal. From the results in Section 3.4, PAC showed no difference between any two emotions. However, DPAC has the potential to differentiate emotional valence in the D-B frequency band pair, indicating that emotional information in the D-B frequency band pair is most disturbed by PCB.
DPAC requires fewer features than PAC but achieves comparable emotion recognition performance, implying that the top-ranked DPAC features contain more emotional information. In other words, by using DPAC features, the input dimension of emotion recognition model is effectively reduced, leading to reduced computational complexity and improved real-time processing capabilities. Additionally, the linear subtraction of phase clustering bias from each phase angle produces little negative effect on emotional EEG phase-amplitude coupling, indicates that phase clustering bias may contain fairly limited emotional information. Therefore, we consider the application potential of EEG-based DPAC for differentiating human emotions.
Gamma oscillations are often considered integrating distributed neural processes into higher-order cognitive functions, such as emotion processing [59]. In terms of frequency band pairs, it was found that D-LG and T-HG achieved higher classification accuracy among the six band pairs of PAC. Similarly, A-HG and T-LG achieved higher classification accuracies among the six frequency band pairs of DPAC. These findings highlight the importance of Gamma band in emotion recognition task. Previous studies by Zheng et al. [60] using differential entropy and Yang et al. [61] based on brain functional networks have also shown that EEG signals in the Gamma band are highly sensitive to human emotions.
Despite the fact that the physiological mechanisms underlying emotional EEG phase-amplitude coupling remain unclear, a reasonable attempt has been made to comprehend the multi-frequency interaction of emotional EEG. To our knowledge, this may be the first study to show the effect of PCB on emotional EEG phase-amplitude coupling. These findings may be advantageous for researchers to take the next step in analyzing emotional EEG phase-amplitude coupling.
One limitation of this study is that emotional EEG data were collected in a laboratory environment, which may not represent natural conditions. Additionally, this study did not apply a mathematical model to assess the effect. Future research should include more participants in real-life scenarios and mathematical modeling should be utilized to quantify this effect. Examining the phase-amplitude coupling across different channels will enable us to gain a more comprehensive understanding of information transmission between different brain regions. We will explore the effect of PCB on inter-channel phase-amplitude coupling for emotional EEG in future work.
In this study, statistical analysis and classification were conducted to explore the effect of phase clustering bias on emotional EEG phase-amplitude coupling.
The results of this study show: (i) Emotional EEG phase-amplitude coupling values are overestimated due to PCB. (ii) Removing PCB enhances the difference in coupling strength between fear and happy emotions in the frontal lobe. (iii) Comparable emotion recognition performance was achieved with fewer features after removing PCB.
These findings suggest that removing PCB may be advantageous for analyzing emotional EEG phase-amplitude coupling and recognizing human emotions. This work provides a reference for studying the multi-frequency interaction of emotional EEG to reveal the physiological mechanisms underlying human emotion, and also illustrates the application potential of EEG-based DPAC for differentiating human emotions.
PAC, phase-amplitude coupling; DPAC, debiased phase-amplitude coupling; PCB, phase clustering bias; MVL, mean vector length; EEG, electroencephalogram; REST, reference electrode standardization technique; ICA, independent component analysis; EMG, electromyography; FDR, false discovery rate.
The EEG data analyzed during the current study are from a public dataset SEED-IV (https://bcmi.sjtu.edu.cn/~seed/seed-iv.html). The EEGLAB toolbox used for EEG preprocessing is freely available online (https://github.com/sccn/eeglab). Other code will be made available from the corresponding author on reasonable request.
TS and QF designed the research. TS performed the research. QF analyzed the data. ZL interpret the results. ZL and SZ revised the paper. SZ conceptualized the method. MX administrated the project and made some contributions to the figures. DM, YY, and EY investigated the dataset and supported funding acquisition. DM provided analysis tools. YY and EY provided computing resource and supervised the research. TS and QF wrote the manuscript. All authors contributed to editorial changes in the manuscript. All authors read and approved the final manuscript. All authors have participated sufficiently in the work and agreed to be accountable for all aspects of the work.
Not applicable.
We thank Prof. Baoliang Lu in Shanghai Jiao Tong University for providing the emotional EEG dataset. Also, we wish to thank the two anonymous reviewers for their valuable suggestions.
This research was funded by the National Natural Science Foundation of China, grant number 61703407 and 62332019.
The authors declare no conflict of interest.
Publisher’s Note: IMR Press stays neutral with regard to jurisdictional claims in published maps and institutional affiliations.