- Academic Editor
-
-
-
†These authors contributed equally.
Background: The Fazekas scale is one of the most commonly used visual
grading systems for white matter hyperintensity (WMH) for brain disorders like
dementia from T2-fluid attenuated inversion recovery magnetic resonance (MR)
images (T2-FLAIRs). However, the visual grading of the Fazekas scale suffers from
low-intra and inter-rater reliability and high labor-intensive work. Therefore,
we developed a fully automated visual grading system using quantifiable
measurements. Methods: Our approach involves four stages: (1) the deep
learning-based segmentation of ventricles and WMH lesions, (2) the categorization
into periventricular white matter hyperintensity (PWMH) and deep white matter
hyperintensity (DWMH), (3) the WMH diameter measurement, and (4) automated
scoring, following the quantifiable method modified for Fazekas grading. We
compared the performances of our method and that of the modified Fazekas scale
graded by three neuroradiologists for 404 subjects with T2-FLAIR utilized from a
clinical site in Korea. Results: The Krippendorff’s alpha across our
method and raters (A) versus those only between the radiologists (R) were
comparable, showing substantial (0.694 vs. 0.732; 0.658 vs. 0.671) and moderate
(0.579 vs. 0.586) level of agreements for the modified Fazekas, the DWMH, and the
PWMH scales, respectively. Also, the average of areas under the receiver
operating characteristic curve between the radiologists (0.80
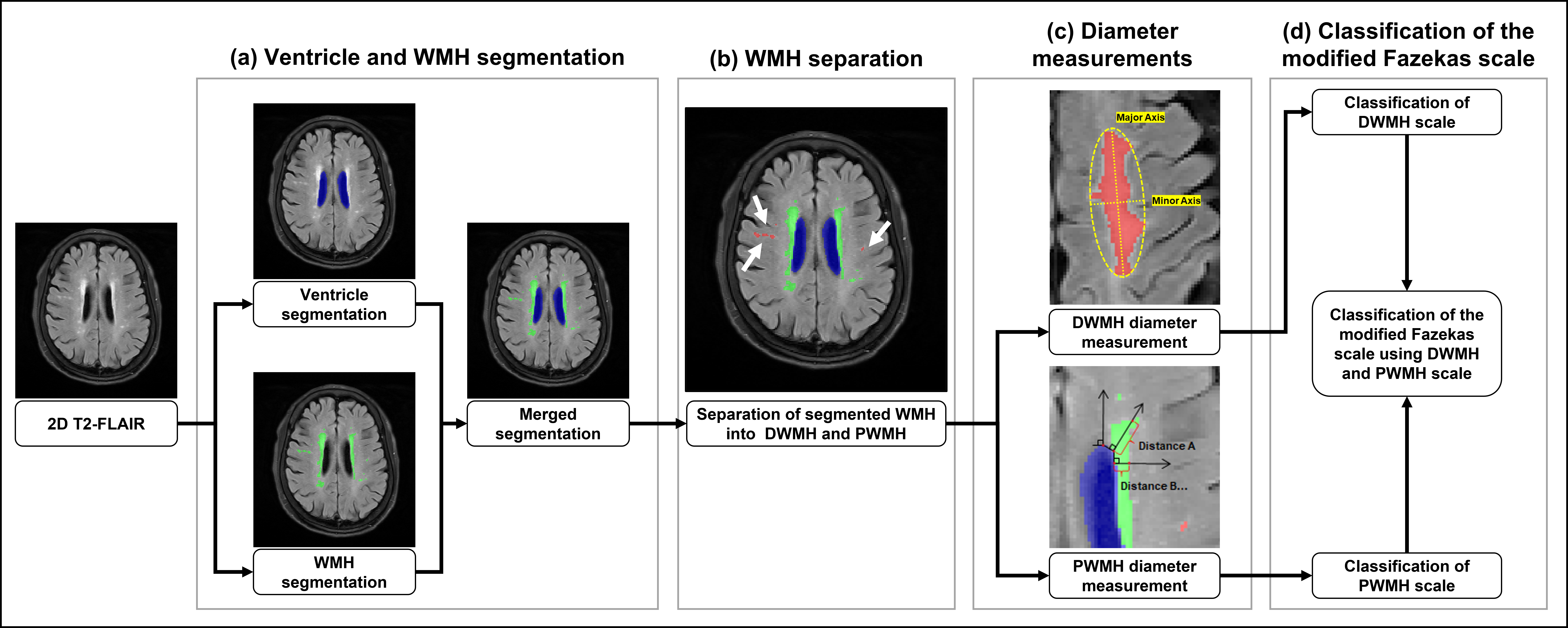