- Academic Editor
Background: Cancer is the biggest cause of mortality globally, with approximately 10 million
fatalities expected by 2020, or about one in every six deaths. Breast, lung,
colon, rectum, and prostate cancers are the most prevalent types of cancer.
Methods: In this work, fractional modeling is presented which describes the dynamics of
cancer treatment with mixed therapies (immunotherapy and chemotherapy).
Mathematical models of cancer treatment are important to understand the dynamical
behavior of the disease. Fractional models are studied considering immunotherapy
and chemotherapy to control cancer growth at the level of cell populations. The
models consist of the system of fractional differential equations (FDEs).
Fractional term is defined by Caputo fractional derivative. The models are solved
numerically by using Adams-Bashforth-Moulton method.
Results: For all fractional models the reasonable range of fractional order is between
Hippocrates, the Greek physician famed as the “Father of Medicine”, is credited with introducing the term “cancer”. Sarcomas, lymphomas, carcinomas, leukemias, and brain tumors were the five main cancer groups identified by Hippocrates [1]. Cancer is one of the deadliest diseases in the history of medicine. It is defined as an abnormal development of defective cells within the body [2]. Several causes of cancer have been identified, including chemical exposure, alcohol consumption, smoking, excessive sun exposure, and genetic variations [3]. Cancer is a life-threatening and dreaded disease that causes people significant suffering. A cancer diagnosis causes more distress than non-neoplastic disorders with an inferior prognosis [4]. Anxiety, sadness, or both may develop because of prolonged emotional suffering in cancer patients. Cancer patients are estimated to be three times more likely than the general population to suffer from depression [5]. Immunotherapy, chemotherapy, surgery, and radiotherapy are the four basic types of cancer treatment procedures. Radiation therapy is a treatment that is used to kill cancerous cells. Radiation therapy is a vital and effective tool for halting tumor growth [6]. Treatment aims to kill or remove the cancerous cells (basic treatment), destroy the remaining cancer cells (helper treatment), or treat the side effects caused by the disease (supportive treatment).
The objective of immunotherapy is to reinforce the body’s inherent capacity to battle against disease by upgrading the viability of the insusceptible framework [7]. Chemotherapy is a type of cancer treatment that includes drugs that inhibit tumor cells’ ability to grow and causes them to die in the circulation. Chemotherapy’s impact on both healthy tissue and malignant cells remains a major concern [8].
Mathematical modeling and computation are important tools that can help control epidemics and human diseases [9, 10, 11, 12]. Different models of cancer treatment were suggested by several authors; for example, D’Onofrio et al. [13] investigated the role of mathematical modeling in combination therapy for tumors; Kermack and McKendrick’s [14] work demonstrated the importance of mathematical modeling of biological events in dealing with epidemics. The readers are referred to the research on epidemics [14, 15, 16, 17, 18, 19, 20, 21, 22].
Recently, various mathematical models that concentrate on the radiation used to treat cancer have been presented and studied. Liu et al. [23] concentrated on the dynamical behaviors of healthy cells that were influenced by periodic radiation and established certain requirements for the survival and extinction of healthy and radioactive cells. In addition, they discovered conditions for the system’s positive periodic solutions and their existence and global asymptotic stability [23, 24].
Dynamical modeling and bifurcations theory is important to study real world phenomena [25, 26, 27, 28]. Fractional calculus is applied in different fields of physics, mathematical biology, flow mechanics, electrochemistry, signal processing, viscoelasticity, finance, etc. In the field of fractional calculus, fractional derivatives and fractional integrals are essential aspects [29].
In this work, fractional modeling is used to study cancer treatment. Several aspects of cancer treatment are considered, such as, cancer treatment with immunotherapy, and chemotherapy with the inclusion of depression effects. Furthermore, positivity and boundedness of the solutions are proved. Afterwards, Lyapunov stability for fractional models is studied. Adams-Bashforth Moulton (ABM) method is applied to find the solution of fractional differential equations (FDEs) numerically. Test problems are formulated to validate the fractional models. The parametric study is presented, and several elements of the illness are emphasized. Furthermore, the procedure of the curve fitting technique is explained and implemented on the proposed models. This study is an effort to provide more profound insights into various aspects of mixed therapies and cab contribute for the cancer treatment. Fractional modeling of cancer treatment gives us more accurate and realistic results than integer order. Cancer treatment takes longer time period which is clearly depicted by using fractional modeling. Finally, using the curve fitting procedure, a function is derived, which may be helpful for medical practitioners to treat the cancer patients which enhances the importance of this study. The work is novel as different models are studied and their analysis are presented in detail.
The layout of the paper is as follows: The fractional-order cancer therapeutic models are described in Section 2. Lyapunov stability, positivity and bounded solutions are carried out in Section 3. In Section 4, the results of the considered models using ABM technique are presented. The parametric study of the model is also presented. Furthermore, the procedure of the curve fitting technique is explained and implemented. Section 5 is related to the conclusion and future recommendations of the research work.
This section comprises fractional modeling for the treatment of cancer. In fractional calculus, we study the derivatives (rate of change) between 0 and 1. Three different classes are introduced, i.e.,
The terms of developed models are further defined as below:
Effector (E): Effector cells that have the ability to destroy cancer cells and virus-infected cells.
Tumor (T): Tumor cells are lumps of tissue. Every cancer must be a tumor. Tumors can be cancerous or not cancerous.
Concentration (C): Concentration of chemotherapy in the blood which influences the number of treatment doses set during each cycle.
Model 1:
Consider FDEs with immunotherapy is as follows:
and
In the absence of therapy, the tumor develops logistically. The term
Model 2:
Consider the FDEs with imunotherapy and chemotherapy as follows:
Here,
Model 3:
Consider FDEs with inclusion of depression effects as follows:
where
The terms
Model 4:
Consider FDEs with inclusion of depression effects at at secondary stage as follows:
In Eqn. 4 model, the secondary stage of cancer is presented where the depression effects are considered 0.08 in the patient. Depression causes an increase in cancerous cells in patients, and high dose of chemotherapy is required. Tumor cells decrease due to mixed therapy, but immune cells also decrease because of chemo-concentration.
Here, the initial conditions for Eqn. 3 and Eqn. 4 are as follows:
For many Years, fractional operators have been defined in different ways [30]. The most common definition of the fractional derivative are the Riemann-Liouville (RL) derivative and the Caputo derivative [31]. RL derivative is defined as follows:
where,
The Caputo derivative is defined as follows [32]:
with
Models will be useful if the system’s solution is non-negative and the starting condition remains positive for all
For all
Proof:
Consider the first equation of Model 1 given in Eqn. 1:
Now, we let
Hence,
Thus the solution of the above equation is
Similarly, it can be shown that the quantities
The solution
This section comprises the analysis of the proposed models. The stability analysis is done by using lyapunov stability and the positivity of the solutions is proved. Furthermore, ABM method is presented and applied to solve proposed models numerically.
Equilibrium points of a dynamical system of equations are the points in which the variables remain constant over time. This means that the system is in a steady state and the derivatives of the equations are all zero. There may be multiple equilibrium points for a given system of equations, and each one represents a different steady state of the system.
To evaluate the equilibrium points
Using MATLAB system of model 1 (c.f Eqn. 1) has two equilibrium points
Model 2 (c.f Eqn. 2) has two equilibrium points
Here,
Model 3 (c.f. Eqn. 3) has one equilibrium point i.e
The stability analysis of the steady state solution is also obtained using all considered models.
The parameters values are given in Table 1 and taken from literature [6, 18, 22].
Name of parameters | Notation | Values |
Immune cells flow into the tumor site at a regular rate | 1.3 | |
Maximal rate of immune cell recruitment | 1.245 | |
Chemotherapy kills fractional immune cells | 3.4 | |
Chemotherapy kills fractional tumor cells | 0.9 | |
Death rate of immune cells due to malignant cells attachment | 3.42 | |
Immune cells kills fractional tumor cells | 1.1 | |
Death rate of immune cells | 4.120 | |
Chemotherapy degradation rate | 4.466 | |
Immune cell recruitment steepness coefficient | 2.020 |
Let
A Lyapunov function is a scalar function defined on a dynamical system that is useful in stability analysis. It is named after Russian mathematician Aleksandr Lyapunov. Lyapunov function is used to measure the amount of potential energy of a system, and is useful in determining the stability of a system. In fractional dynamical systems, this function is a generalization of the classic Lyapunov function used in standard dynamical systems.
Let
(1) If
(2) If
(3) If
Here,
By using MAPLE we generated a Jacobian matrix of Model 1 (c.f. Eqn. 1, denoted by
Jacobian matrix of Model 2 (c.f. Eqn. 2), denoted by
Jacobian matrix of Model 3 (c.f. Eqn. 3), denoted by
Jacobian matrix of Model 4 (c.f. Eqn. 4), denoted by
We use the Lyapunov function method to study the stability of the unique positive equilibrium points. We first define the following Lyapunov function of Model 1 (c.f. Eqn. 1).
Let
where
After simplifications, we obtain
we get
Lyapunov function for the Model 2 (c.f. Eqn. 2) is
where
we obtain
Similarly, Using Lyapunov function for the Model 3 (c.f. Eqn. 3) and Eqn. 25, we get
where
where
we obtain
Similarly, Using Lyapunov function for the Model 4 (c.f. Eqn. 4), we have
where,
where
we obtain
The exact solution is not always possible, therefore, one can use different numerical techniques to solve the problems. In this work, ABM technique is applied to solve the model equations and explained subsequently.
The system of fractional model has the following form:
We can transform the differential equation into the equivalent equation by using a fractional integral operator and including the initial conditions.
which is also a volterra equation of the second kind.
Here,
where
An explicit calculation yields that we can write the integral on the right-hand side of Eqn. 37 as
where
which is our corrector formula, i.e., the fractional variant of the one step ABM technique, which is
The excess problem is the determination of the predictor formula that we need to compute the value
where,
The predictor
The complete description of our fundamental calculation, which is the fractional version of the one step ABM.
First, we need to compute the predictor according to Eqn. 43 then we estimate
In this section, the numerical solutions of the models are presented. Following that, a parametric study of cancer treatment for depression is presented.
Model 1:
In Test Problem 1, the primary stage of cancer is considered with immunotherapy. Simulation results are obtained using ABM technique.
Fig. 1 depicts the proliferation of cancer cells at different fractional orders of
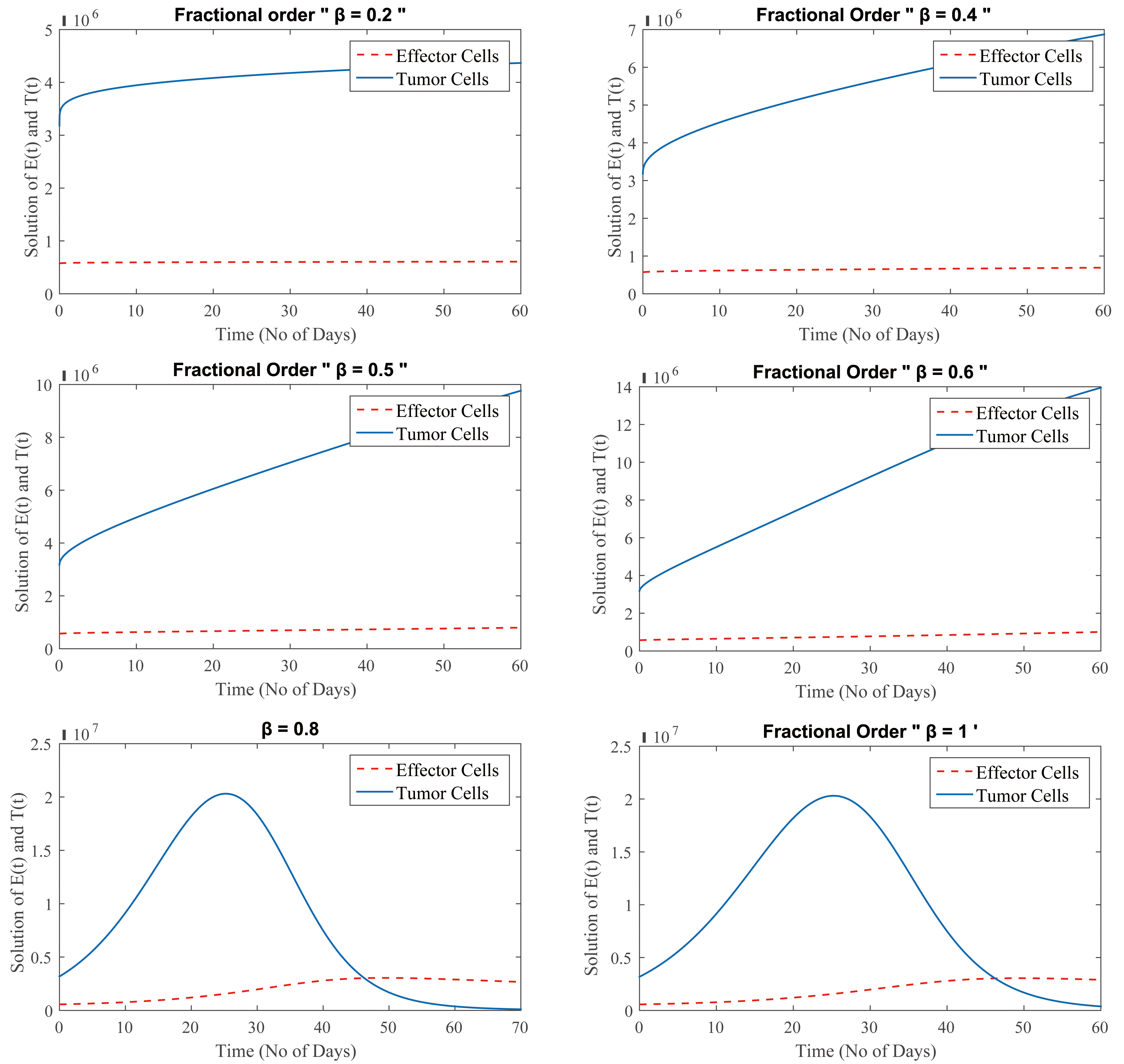
(Model 1) Level of effector and tumor cells at
Moreover, Fig. 1 shows that at
Generally, the cancer treatment takes longer time when effector cells increase and tumor cells reduce which is depicted in Fig. 1 for
Model 2:
In this Test Problem 2, FDEs of cancer treatment with immunotherapy and chemotherapy are considered while taking low-dose chemotherapy and immunotherapy into account. Tumor cells first shrink and then multiply, but as effector cells proliferate, tumor cell development slows again. This process has been shown at different fractional orders.
Fig. 2 shows the graphical representation of Eqn. 2, using immunotherapy and chemotherapy (low concentration) in combination. Fig. 2 depicts that the patient receives combined therapy, the outcome is also favourable, but the immune system is weakened slightly since the chemotherapy kills some effector cells along with the cancer cells. It is also a treatment for early-stage cancer; however, chemotherapy is administered in extremely low dosages. In summary, immunotherapy is used to treat patients with lesser dosages of chemotherapy, resulting in a better prognosis.
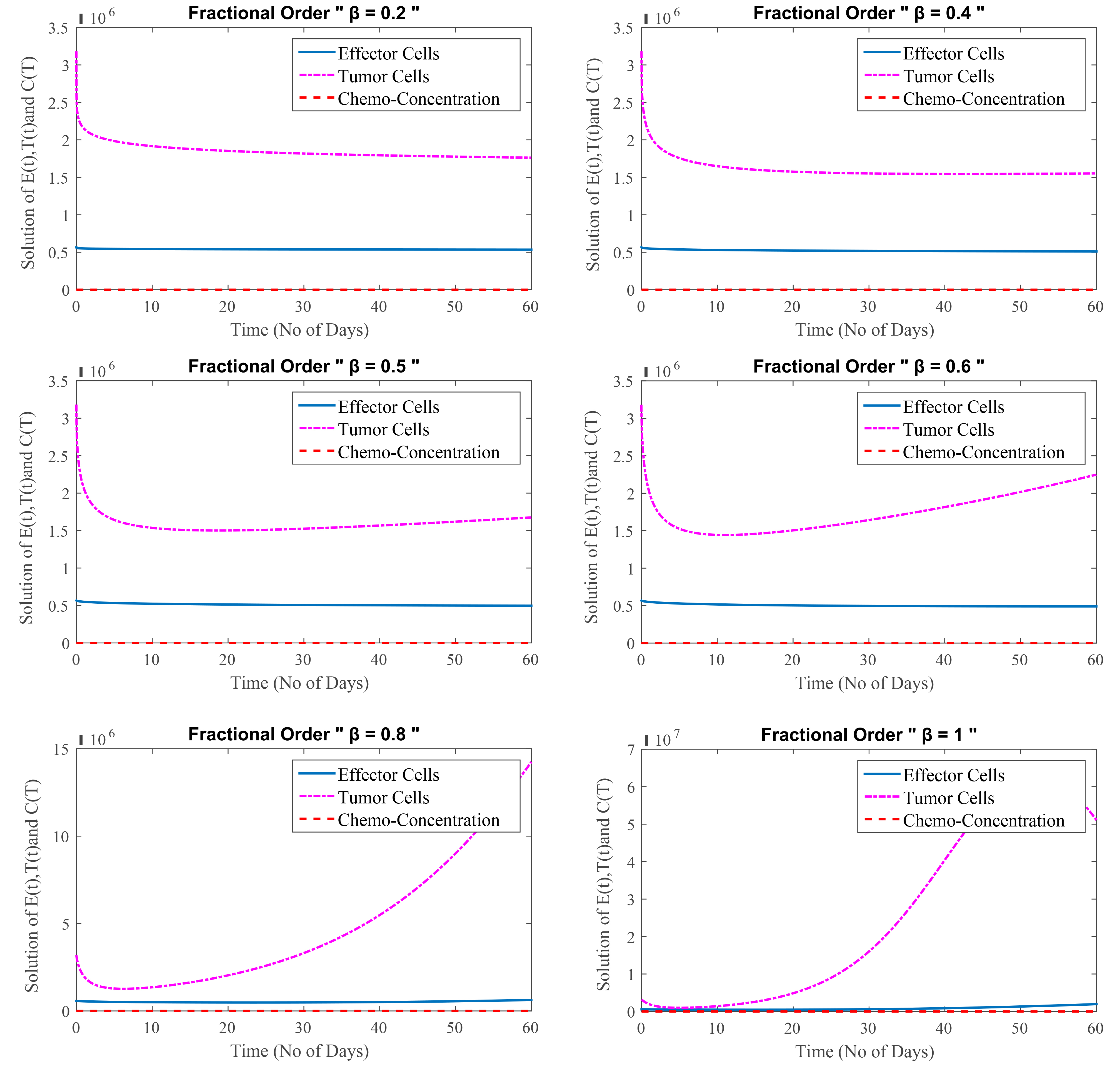
(Model 2) Level of effector cells, tumor cells and concentration of chemotherapy at
Fig. 2 shows that at
Model 3:
In this test problem, FDEs with inclusion of depression effects are studied. Cancer is being treated intensively because cancer cells have grown extremely powerful. High doses of chemotherapy are combined with immunotherapy, resulting in an increase in effector cells from the immunotherapy and a decrease in tumor cells from the chemotherapy. Chemotherapy, as the treatment progresses, affects the effector cells as well as the tumor cells.
Fig. 3 depicts the relation among effector cells, tumor cells and chemo-concentration for different values of
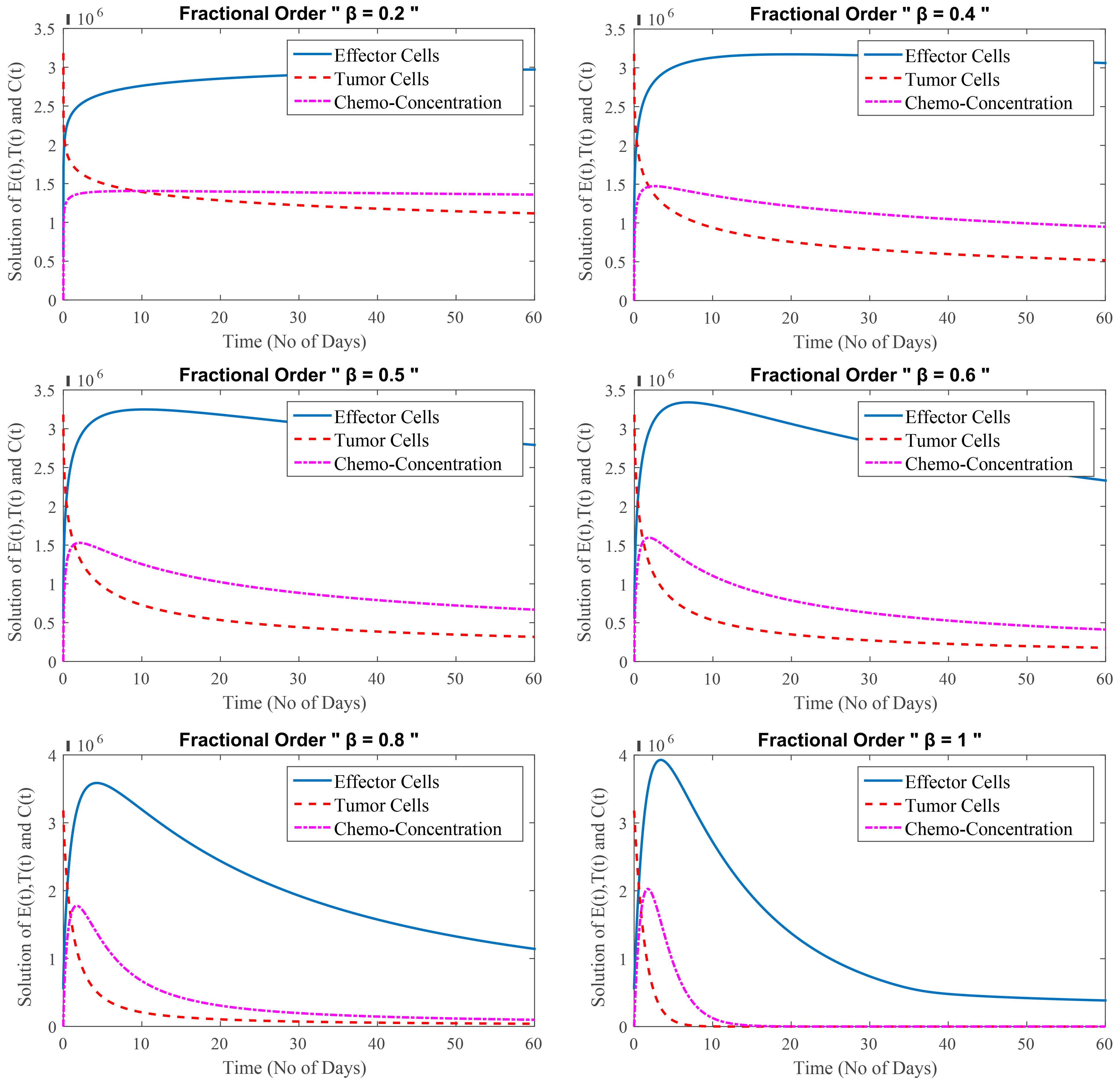
(Model 3) Level of effector cells, tumor cells and concentration of chemotherapy at
Fig. 3 shows that at
Model 4:
In this test Problem, FDEs of cancer treatment at secondary stage are taken into account. This stage of cancer is known as the secondary stage, or metastasis, and it is extremely dangerous. The effector cells are reduced from the start at this stage, while the tumor cells are suppressed owing to chemotherapy. It is detrimental to patients and can potentially kill them over time because of the weak immune system. Chemotherapy and depression both reduce the number of effector cells. The severe impact of depression with chemotherapy has a negative influence on the immune system, effector cells do not rise with immunotherapy. As a result, the patient is on the verge of death.
Fig. 4 shows that even after a combination of therapies, sadness plays an important role in malignant cells growth. The number of cancerous cells in the first stage is lower than in the later stage. Depression has a greater impact during the secondary stage and has not significant impact during the primary stage. The primary stage of cancer is when the disease first appears. A secondary cancer, on the other hand, can be classified as either a new primary cancer in a different part of the body or a metastasis of the original primary cancer to another part of the body.
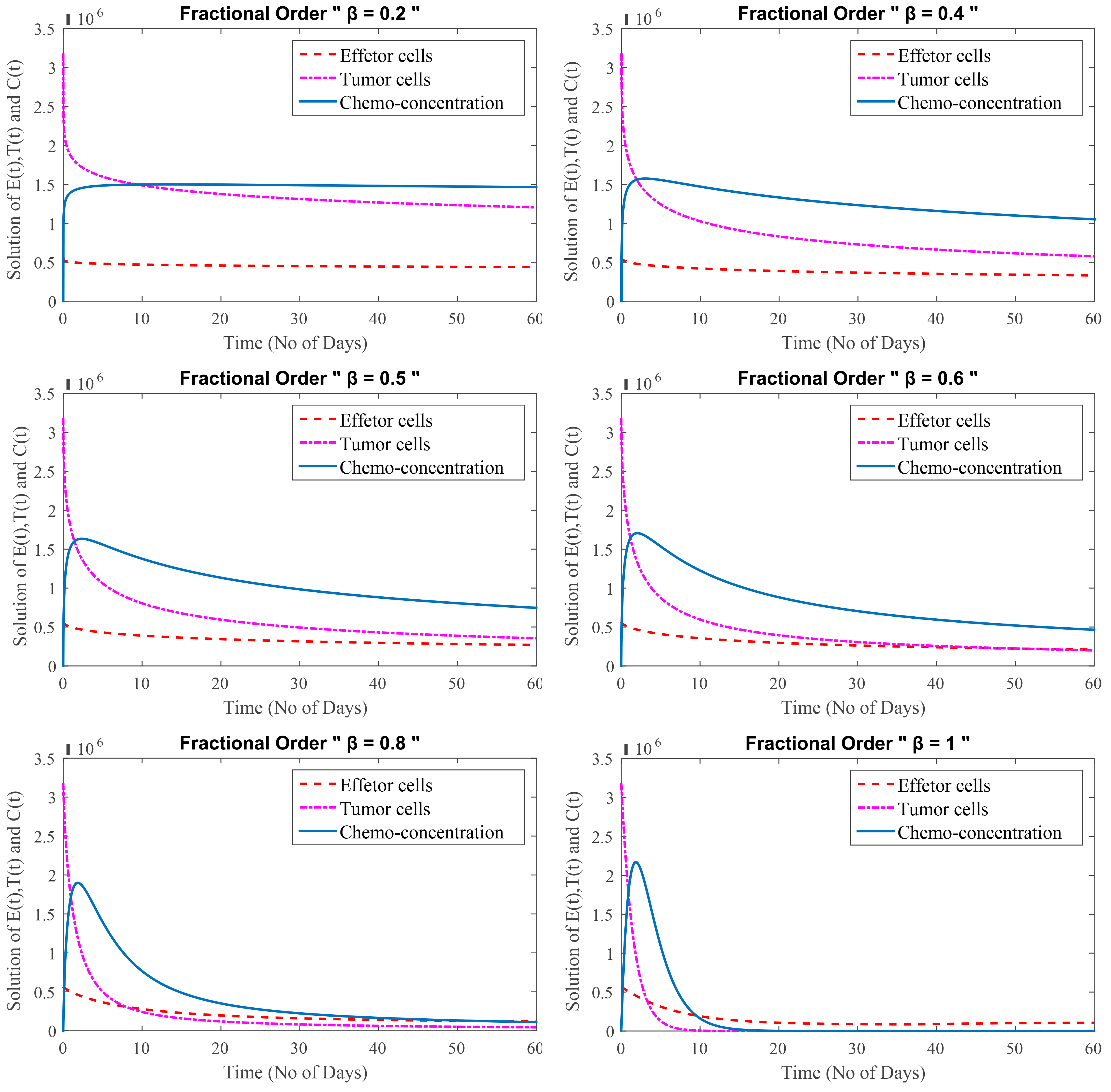
(Model 4) Level of effector cells, tumor cells and concentration of chemotherapy with minimum depression effect at
Fig. 4 shows that at
Cancer survival rates, or survival statistics, indicate the percentage of people who survive a specific type of cancer over a given time period. Statistics on cancer frequently use a five-year overall survival rate. The cancer survival rate is affected by a variety of factors, including the type of cancer, the time since diagnosis, and the patient’s age. For example, typically, survival rates are expressed in percentages. For instance, 77 percent of bladder cancer patients survive for at least five years overall. In other words, 77 out of every 100 bladder cancer patients are still alive five years after their diagnosis. In contrast, 23 out of every 100 people who are diagnosed with bladder cancer pass away within five years.
On the basis of these results, we conclude that the fractional models gives a better approximation for
Here, we are discussing the secondary stage, during which the patient is unable to handle such strong medication for an extended period of time since his immune system has already been weakened significantly as a result of cancer cells and depression. This estimate states that the patient has an average remaining life expectancy of five years, or 1825 days, at this point. Here,
One of the most significant concern among cancer patients in the secondary stage is depression. Fig. 5 displays the depression effects on patient’s health considering
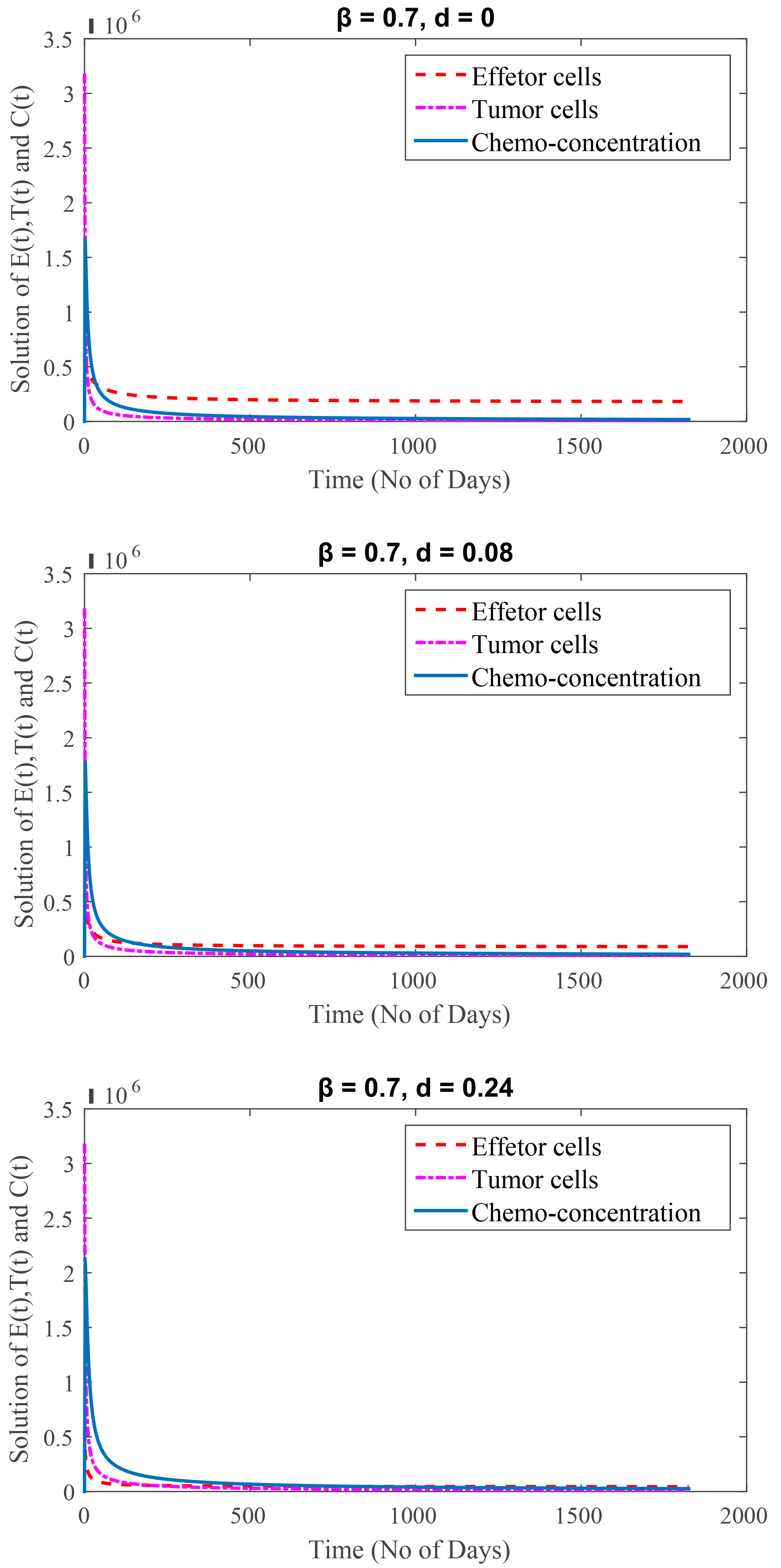
(Model 4) Time period of 5 years.
The graphical representation clearly shows how high depression affects immune cells, causing them to weaken. It means cancer patients not only need medical treatment but also your care and support to fight against cancer.
This section focuses on the effects of several important parameters. In the simulations, we change one parameter while fixing the values of the other parameters. Here,
In Fig. 6, the value of
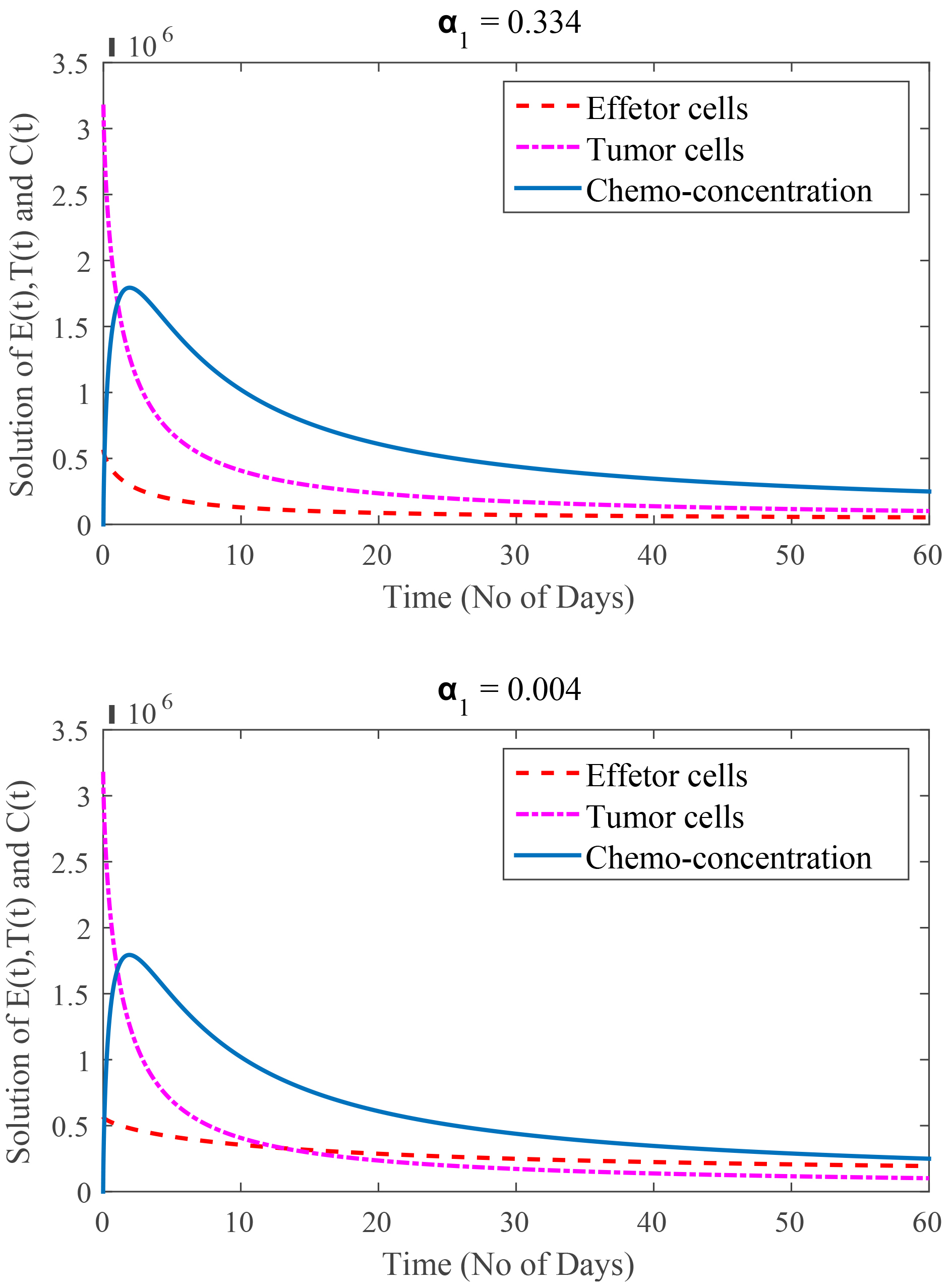
(Model 4) Rate of immune cells kill by chemotherapy are
In Fig. 7, the value of
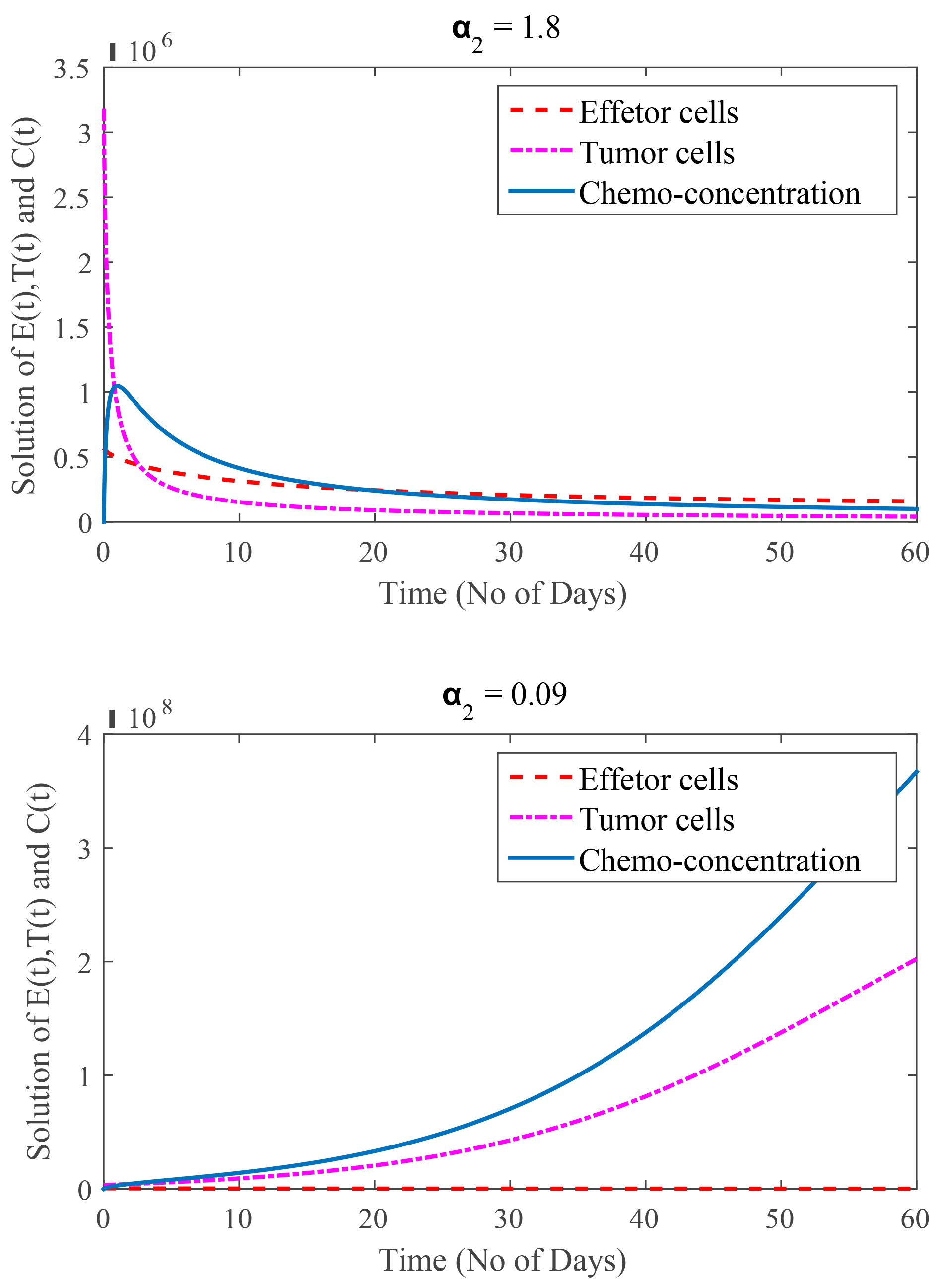
(Model 4) Rate of tumor cells kill by chemotherapy are
In Fig. 8, the value of
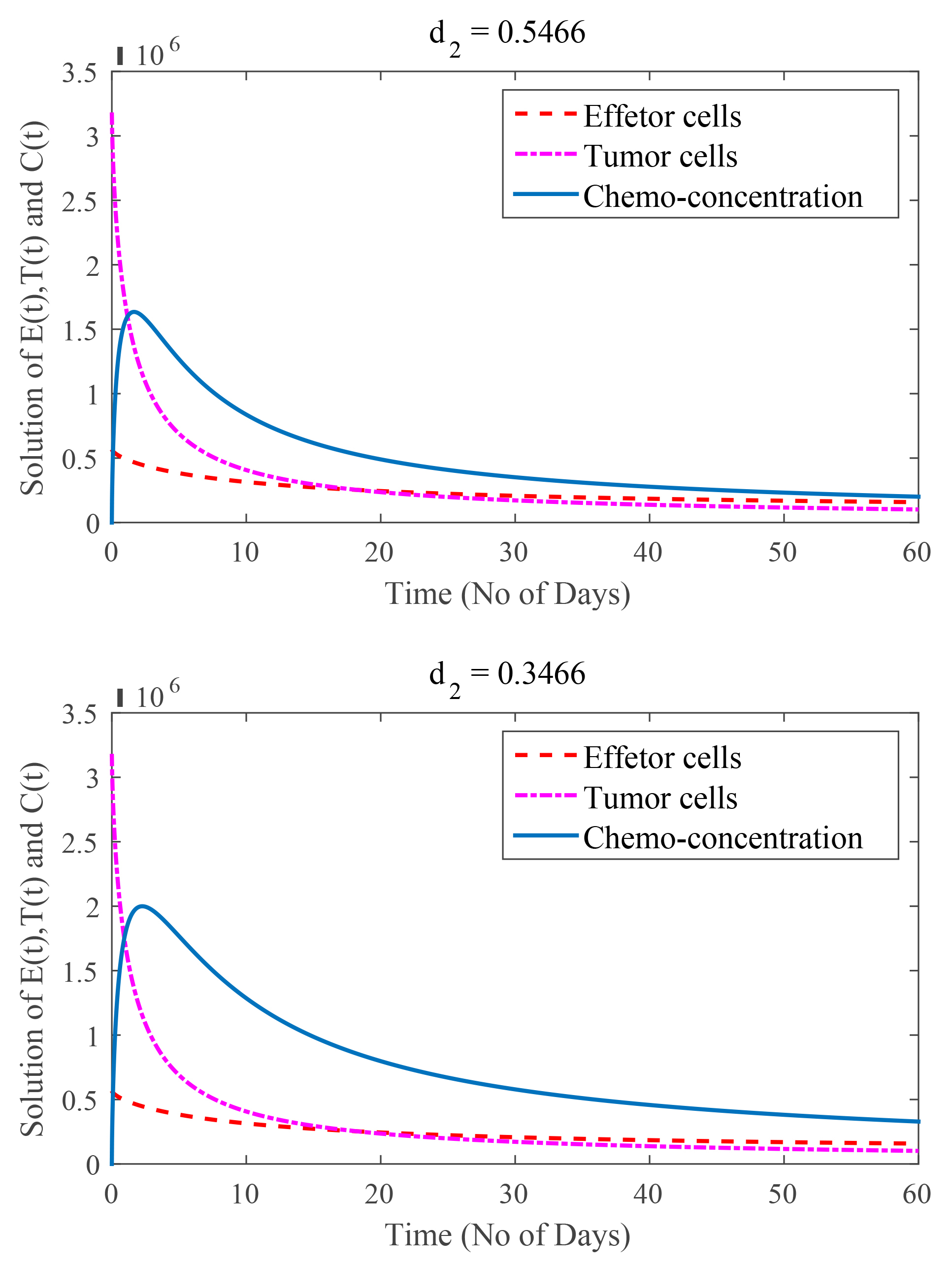
(Model 4) Rate of chemotherapy drug decay are
The act of creating the mathematical function or curve that best fits a set of data points, perhaps subject to limitations, is known as “curve fitting”. A “smooth” function that roughly matches the data is created during curve fitting, as opposed to interpolation, which requires a perfect fit to the data. Discrete data points are connected using interpolation in order to provide realistic estimates of the data points between the given ones. Finding the curve that would best represent the trend of a given collection of data is known as “curve fitting”.
The curve fitting is applied for Model 4. The procedure is repeated for tumor cells, chemo-concentration (chemotherapy), and effector Cells, graphically presented in Fig. 9 and Fig. 10 Tables 2,3 display the information. It has been proposed to have a bi-exponential model as presented in Eqn. 44 by observing the nature of the data.
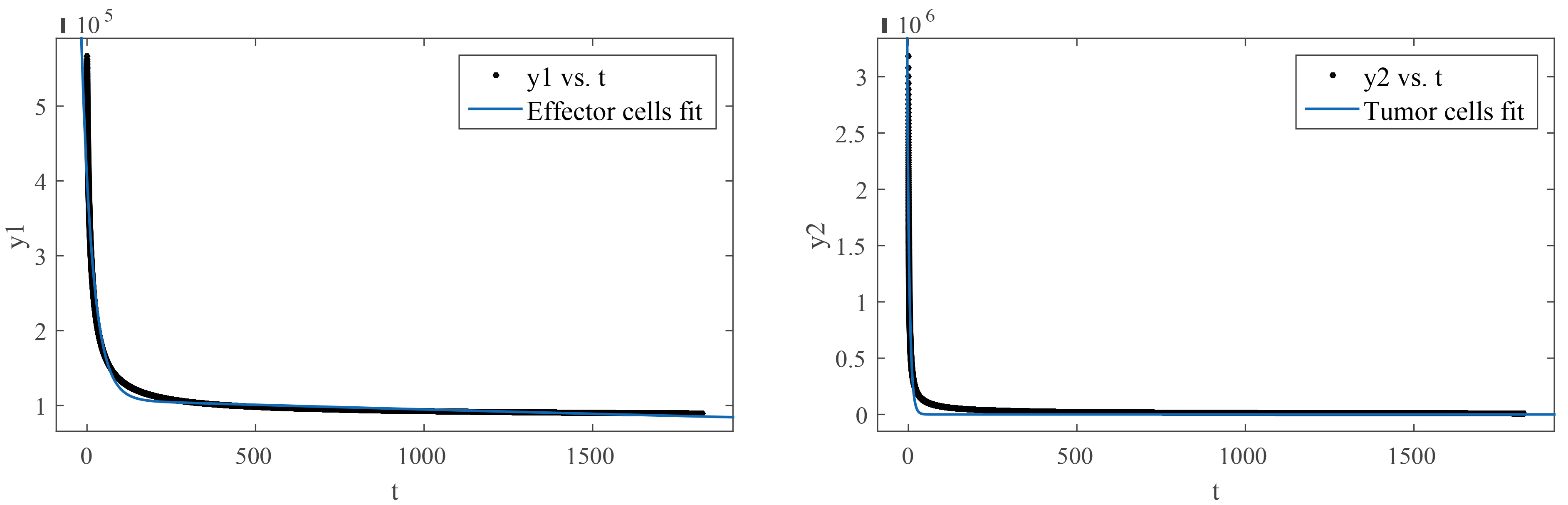
(Model 4) Curve fitting on effector cells and tumor cells.
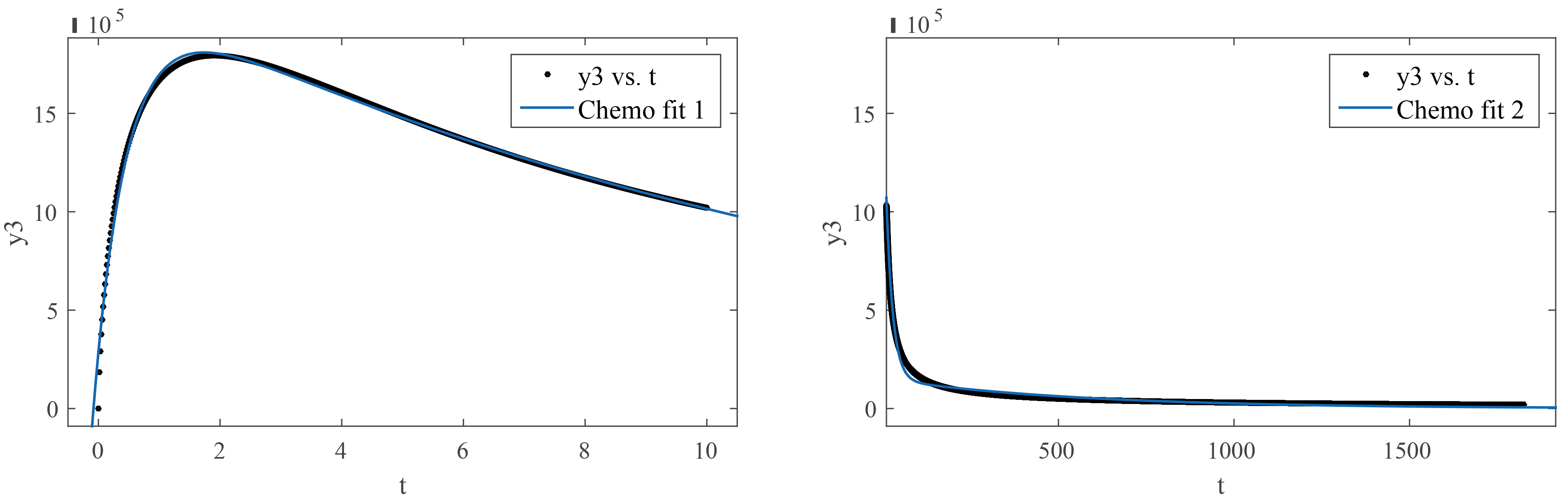
(Model 4) Curve fitting of chemo-concentration at time 0 to 10 and 10 to 1825 days.
Name of variables | a |
b |
c |
d |
Effector cells | ||||
Tumor cells | ||||
Chemo-concentration (0–10) | ||||
Chemo-concentration (10–1825) |
Name of variables | SSE | R-square | Adjusted R-square | RMSE |
Effector cells | 0.9645 | 0.9645 | 5917 | |
Tumor cells | 0.9544 | 0.9544 | ||
Chemo-concentration (0–10) | 0.995 | 0.9949 | ||
Chemo-concentration (10–1825) | 0.9685 | 0.9685 |
Abbreviations: SSE, Sum of Squares; RMSE, Root mean square error.
where
Exponentials are often used when the rate of change of a quantity is proportional to the initial amount of the quantity. If the coefficient associated with
The careful selection of a mathematical function able to accurately represent the data was based on various parameters of “goodness of fit” like SSE, R-squared values, adjusted R-squared values, and root mean square error (RMSE). Other functions are likely to present poor values for goodness of fit. We have bi exponential model of Eqn. 44 for representation of all experiments. It can be observed that “
Eqn. 44 is very useful for medical practitioners to treat the cancer patients. In Eqn. 44,
The purpose of this work was to study a fractional models for cancer treatment with mixed therapies. In this work, different fractional models was theoretically studied. The model contains system of FDEs which incorporate the effector cells, tumor cells and rate of chemotherapy. Stability analysis was done using Lyapunov function. Moreover, positivity and boundedness of the solution was proved. Furthermore parametric study and curve fitting procedure was also discussed. The results are presented for four different models of primary and secondary stage for cancer treatment.
The results indicate that, before treating a cancer patient, it is important to know the stage of the disease. We were able to control tumor cells during the primary stage, but it was more difficult to control cancer cells during the secondary stage due to a reduction in immune cells caused by chemotherapy and depression. We found that the first stage of cancer or tumor growth is slower than the secondary stage. Effect of some positive parameters are weakens because effect of depression is higher than secondary stage.
The loss of immune cells is a key factor in tumor progression. In this paper, we provided the findings of a mathematical model that shows sadness decreases immune cell counts while increases tumor cell counts. As the patient is unaware that he has cancer in its early stages, depression has little impact on either immune cells or tumor cells. As soon as he learns, the patient is affected by depression. As the effects of depression worsen, the patient’s immunity declines, which increases the number of tumor cells. The impact of chemotherapy is very low, nearly inert, and the patient might die from depression as the effect of depression develops and the tumor cells grow significantly. The fractional model of the secondary stage gave a better approximation for
Future researches may explore parameter estimation, the inclusion of different doses in chemotherapy which may not weak the immune system. This study is an effort to provide more profound insights into various aspects of cancer disease and its treatment.
Datasets used and/or analyzed for this study are available from the corresponding author upon appropriate request.
SJ: Conceptualization, investigation, methodology, analysis, and writing–original draft, review, supervision and project administration. ZA: Investigation, analysis, validation and writing–original draft and review. DB: Validation, formal analysis, supervision, and project administration. All authors have contributed to the manuscript and approved the submitted version. All authors have read and agreed to the published version of the manuscript. All authors have participated sufficiently in the work and agreed to be accountable for all aspects of the work.
Not applicable.
Not applicable.
This research received no external funding.
The authors declare no conflict of interest. DB is serving as one of the Guest editors of this journal. We declare that DB had no involvement in the peer review of this article and has no access to information regarding its peer review. Full responsibility for the editorial process for this article was delegated to SL.
Publisher’s Note: IMR Press stays neutral with regard to jurisdictional claims in published maps and institutional affiliations.