- Academic Editor
†These authors contributed equally.
Background: Early identification of sepsis improves the survival rate; however, it is one of the most challenging tasks for physicians, especially since symptoms are easily confused with those of systemic inflammatory response syndrome (SIRS). Our aim was to explore biomarkers for early identification of sepsis that would aid in its differential diagnosis. Methods: Eight patients with SIRS, eight with sepsis, and eight healthy controls were included in this study. Metabolites were screened using gas chromatography-mass spectrometry (GC-MS). Metabolism profiles were analyzed using the untargeted database of GC-MS from Lumingbio (LUG) database, and metabolic pathways were enriched based on the Kyoto Encyclopedia of Genes and Genomes (KEGG) database. The S-plot was used to screen the potential biomarkers distinguishing between patients with SIRS, sepsis, and healthy controls. Receiver operating characteristic (ROC) curve analysis was used to evaluate potential biomarkers between SIRS and sepsis patients. Correlation analysis was used to measure the degree of correlation between differential metabolites. Correlation analysis between 2-deoxy-d-erythro-pentofuranose-5-phosphate and clinical indicators was performed. Results: There were 51 metabolites that were distributed in the SIRS group, and they were enriched with 18 metabolic pathways compared with healthy controls. Moreover, 63 metabolites in the sepsis group were significantly distinguishable compared to the healthy controls, and were associated with 21 metabolic pathways. Methyl 3-o-acetyl-d-galactopyranoside and N-acetylputrescine were found to be candidate biomarkers for distinguishing between SIRS, sepsis, and healthy controls using the S-plot model. Only four differential metabolites, including 2-deoxy-d-erythro-pentofuranose-5-phosphate, terbutaline, allantoic acid, and homovanillic acid (HVA), were enriched in the dopaminergic synapse and tyrosine metabolism pathways when sepsis patients were compared with SIRS patients. The Area Under Curve (AUC) of 2-deoxy-d-erythro-pentofuranose-5-phosphate was 0.9297, indicating a strong diagnostic ability for sepsis. A significant negative correlation was identified between 2-deoxy-d-erythro-pentofuranose-5-phosphate and lactate (r = –0.8756, p = 0.0044). Conclusions: Methyl 3-o-acetyl-d-galactopyranoside and N-acetylputrescine may be used as candidate biomarkers to distinguish SIRS and sepsis patients from healthy controls using GC-MS. 2-deoxy-d-erythro-pentofuranose-5-phosphate may be the candidate biomarker to distinguish sepsis from SIRS. Our study explored candidate biomarkers for the early identification of sepsis, which is vital for improving its prognosis.
Sepsis is a life-threatening condition that affects host tissues and organs and is caused by an imbalance in the host immune response to infection. Although the current clinical guidelines are constantly updated, the mortality rate of sepsis remains high. Sepsis progresses rapidly; therefore, early detection is critical for improving the prognosis. However, owing to its complex etiology and pathogenesis, the identification of sepsis is often difficult, and rapid methods are lacking [1, 2]. Another difficult task in the differential diagnosis of patients with sepsis is distinguishing between infection and non-infectious systemic inflammatory response syndrome (SIRS) [3, 4]. This differential diagnosis is vital for determining the appropriate treatment. Therefore, novel specific biomarkers for sepsis are urgently required.
Many laboratory tests are used for sepsis diagnosis, such as complete blood
count, platelet count, and C-reactive protein levels. However, there is not yet a
specific test for sepsis [5]. Recent studies have shown that procalcitonin is a
useful marker for identifying sepsis in critically ill patients. The accuracy and
clinical value of using procalcitonin as a marker for sepsis have already been
validated. However, when procalcitonin levels are high, sepsis is already severe
[6]. Therefore, this indicator cannot be used as an early predictor of sepsis.
Although the levels of the pro-inflammatory cytokines like tumor necrosis
factor-
Recent studies have reported that metabolic profiling is valuable for identifying sepsis because of its ability to detect differential metabolites. It is well recognized amongst the metabolomics community that gas chromatography-mass spectrometry (GC-MS) is one of the most efficient, reproducible and well used analytical platforms for metabolomics research. GC-MS is a method that combines the characteristics of gas chromatography and mass spectrometry to identify different substances in samples. It is a robust and reproducible technique with high selectivity complemented by a large number of metabolite databases to support the identification of potential biomarkers [9, 10]. Metabolites are the final products of interactions among physiological, genetic, and environmental factors, and metabolic profiling could represent the functional status of individuals [11]. In this study, we aimed to explore the metabolic features and candidate biomarkers for the early identification of sepsis using GC-MS.
Patients with SIRS and sepsis were recruited from Department of Emergency and
Critical Care at the Shanghai General Hospital. The inclusion criteria for
patients with SIRS were the meeting of at least two of the four following
standards: (1) body temperature
Clinical blood samples were collected into heparin-coated tubes and centrifuged at 2200 rpm for 10 min at room temperature. The supernatant was collected, then immediately stored in separate tubes at –80 °C until GC-MS analysis. Next, 20 µL of 2-chloro-l-phenylalanine (catalog number C105993, Aladdin, Shanghai, China) was dissolved in methanol as an internal standard, and 80 µL of serum sample was added. Next, 240 µL of methanol (catalog number 1.06035, Supelco, Bellefonte, PA, USA): acetonitrile (catalog number 1.00029, Supelco, Bellefonte, PA, USA) (2:1 v:v) was added to each sample. The mixtures were extracted by ultrasonication for 8 min in an ice-water bath. Next, the samples were centrifuged at 12,000 rpm for 10 min at 4 °C. An aliquot of the supernatant (150 µL) was transferred to vacuum at 24 °C. Then, 80 µL of methoxylamine (catalog number 89803, Supelco, Bellefonte, PA, USA) was added, followed by vortexing and incubation for 90 min at 37 °C. After that, 50 µL of BSTFA (with 1% TMCS) (catalog number B-023, Supelco, Bellefonte, PA, USA) and 20 µL n-hexane (catalog number 1.00795, Supelco, Bellefonte, PA, USA) were added and mixed, then vortexed and derivatized for 60 min at 70 °C. The samples were placed at 24 °C for 30 min prior to GC-MS analysis.
The samples were acted on by an Agilent 7890B-5977B system (Agilent, Palo Alto,
CA, USA). A 30 m
The parametric Student’s t-test or chi-square test were used to compare
the clinical data between the two groups. Statistical significance of the
metabolites was determined using one-way Analysis of Variance (ANOVA). Receiver
operating characteristic (ROC) curve analysis was used to evaluate the diagnostic
values of the significant differential metabolites. A two-tailed Student’s
t-test was used to verify whether the metabolite differences between
groups were significant. Differential metabolites were selected with variable
importance of projection (VIP) values
Eight patients with SIRS and eight with sepsis were included in this study. Clinical symptoms and Computed Tomography (CT) scan showed that two of SIRS patients have pneumonia, three of them have abdominal infection, other three patients have urethra infection. Two of sepsis patients have pulmonary infection, two patients have abdominal infection, four patients have urethra infection.
Eight healthy individuals were also included as controls. The general
characteristics and laboratory test results of patients with sepsis are shown in
Table 1. There were no significant differences in general characteristics between
patients with SIRS and the healthy controls, nor between patients with sepsis and
the healthy controls. There were significant differences in c-reactive protein
(CRP), interleukin (IL-6), IL-1
Variables | SIRS | SEPSIS | HEALTH |
(n = 8) | (n = 8) | (n = 8) | |
Age (years) | 65 |
66 |
62 |
Males/females | 4/4 | 5/3 | 4/4 |
Pulmonary infection | 2 | 2 | — |
Abdominal infection | 3 | 2 | — |
Urethra infection | 3 | 4 | — |
Temp. sampling time (°C) | 38.1 |
38.1 |
— |
Heart rate (bpm) | 107 |
108 |
— |
Neutrophil (%) | 83.8 |
89.0 |
— |
Lymphocyte (%) | 10.0 |
6.1 |
— |
Monocyte (%) | 4.9 |
3.6 |
— |
Lymphocyte (10 |
1.19 |
1.19 |
— |
Monocyte (10 |
0.40 |
1.06 |
— |
Hemoglobin (g/L) | 141 |
129 |
— |
CRP (mg/L) * | 153.5 |
167.4 |
— |
IL-6 (pg/mL) * | 70.42 |
199.34 |
— |
IL-1 |
276.86 |
469.68 |
— |
TNF- |
133.80 |
133.79 |
— |
Quantitative data are presented as mean
To determine the metabonomic changes, the peripheral blood of patients was collected, with eight samples in each group and eight healthy individuals as controls. Serum was extracted and analyzed by GC-MS. The PLS-DA method is a supervised discriminant statistical method using partial least squares regression for predictive and descriptive modeling, as well as for discriminative variable selection, to explain and predict the differences between the two groups. In our PLS-DA model, owing to the different metabolites, patients with SIRS were significantly distinguished from healthy controls, and patients with sepsis were significantly separated from healthy controls (Fig. 1A). To further improve the analytical ability and effectiveness of the model and maximize the differences between different groups in the model, we modified the PLS-DA to filter out noise irrelevant to the classification information. As a result, OPLS-DA showed that SIRS split with healthy controls. Similarly, there was a split between sepsis and healthy controls between SIRS and sepsis (Fig. 1B).
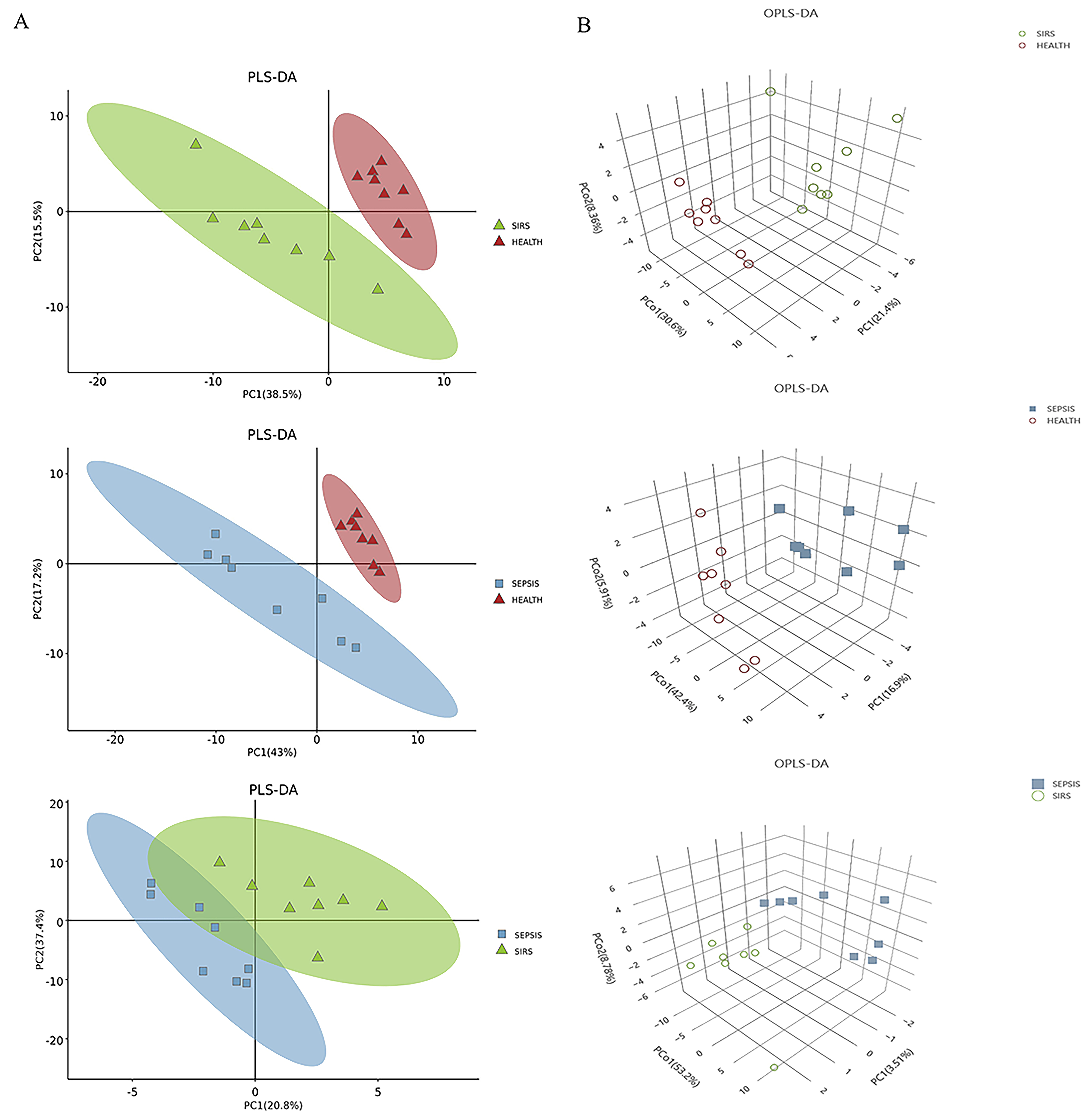
The PLS-DA and OPLS-DA models distinguished patients between groups. (A) The green triangles represent total patients with SIRS, the blue squares represent patients with sepsis, and the red triangles represent heathy controls in the PLS-DA score plots. (B) The green circles represent total patients with SIRS, the blue squares represent patients with sepsis, and the red circles represent heathy controls in the OPLS-DA score plots (PLS-DA, partial least squares discriminant analysis; OPLS-DA, orthogonal projection latent to structure discriminant analysis).
In the OPLS-DA model, the VIP value can be used to evaluate the effects of the
influence intensity and interpretation ability of the expression pattern of each
metabolite on the classification and discrimination of each group, mine the
differential metabolites with biological significance, and then use a
t-test to verify whether the differential metabolite is significant
between groups. The screening criteria were that the VIP value of the first
principal component of the OPLS-DA model was
Metabolite name | SIRS vs. HEALTH | Sepsis vs. HEALTH | SIRS vs. SEPSIS | ||||||
V | VIP | FC | V | VIP | FC | V | VIP | FC | |
Homovanillic acid | — | — | — | — | — | — | ↓ | 2.3804013 | 0.3874618 |
2-deoxy-d-erythro-pentofuranose-5-phosphate | — | — | — | — | — | — | ↓ | 2.0782491 | 0.7222923 |
Terbutaline | — | — | — | — | — | — | ↓ | 1.9669834 | 0.7262929 |
Allantoic acid | — | — | — | — | — | — | ↓ | 1.0306248 | 0.8907599 |
Methyl 3-o-acetyl-d-galactopyranoside | ↑ | 2.9897861 | 71.480053 | ↑ | 3.273992 | 31.431137 | — | — | — |
N-acetylputrescine | ↓ | 2.8419666 | 0.0432872 | ↓ | 2.7994075 | 0.1679551 | — | — | — |
Cadaverine | ↑ | 2.7730684 | 38.21312 | ↑ | 2.9331994 | 17.795369 | — | — | — |
Valyl-leucine | ↑ | 2.7635738 | 21.405291 | ↑ | 2.0790452 | 7.4992831 | — | — | — |
Xanthurenic acid | ↑ | 2.6908135 | 29.936944 | ↑ | 2.7930245 | 12.593242 | — | — | — |
Mannitol | ↓ | 2.5416888 | 0.1587076 | — | — | — | — | — | — |
Spermine | ↑ | 2.5143646 | 14.977715 | ↑ | 2.8699784 | 10.014478 | — | — | — |
Dl-dopa | ↑ | 2.3731151 | 12.102607 | ↑ | 2.2252405 | 8.3510021 | — | — | — |
Lignoceric acid | ↑ | 2.3668183 | 41.598201 | ↑ | 2.6249828 | 13.368459 | — | — | — |
Glycyl tyrosine | ↑ | 2.2843552 | 11.68853 | ↑ | 1.9836009 | 6.8880069 | — | — | — |
Beta-gentiobiose | ↑ | 2.1586369 | 11.045923 | — | — | — | — | — | — |
Norleucine | ↑ | 2.0930844 | 6.9298737 | ↑ | 2.1067457 | 5.2408221 | — | — | — |
Lanosterol | ↑ | 2.0652335 | 10.322189 | ↑ | 2.1999335 | 5.5100827 | — | — | — |
Methyl-ornithine | ↑ | 2.0494509 | 6.3426184 | ↑ | 1.8548467 | 4.4991726 | — | — | — |
L-phenylalanine | ↑ | 2.0352152 | 9.4226303 | ↑ | 1.7352391 | 7.85938 | — | — | — |
N-acetyl-l-tyrosine | ↑ | 1.9737449 | 7.6650888 | ↑ | 1.7700233 | 7.0508449 | — | — | — |
L-isoleucine | ↑ | 1.9504201 | 6.0346911 | ↑ | 1.818343 | 5.8249886 | — | — | — |
Arabinofuranose | ↑ | 1.9150272 | 4.8253548 | ↑ | 1.4816488 | 3.3283155 | — | — | — |
L-methionine | ↑ | 1.8605088 | 10.773329 | ↑ | 1.2911021 | 9.8609908 | — | — | — |
Formononetin | ↑ | 1.8053158 | 9.7609673 | ↑ | 1.7388467 | 6.1410451 | — | — | — |
Maleimide | ↑ | 1.7602332 | 3.9912852 | ↑ | 1.8323139 | 4.2607905 | — | — | — |
Ricinoleic acid | ↑ | 1.7259031 | 6.8452612 | — | — | — | — | — | — |
D-fructose-6-phosphate | ↑ | 1.7180743 | 8.5642974 | ↑ | 1.5012907 | 19.588702 | — | — | — |
L-tryptophan | ↑ | 1.6863417 | 4.2435397 | ↑ | 1.8853432 | 3.6489463 | — | — | — |
2-hydroxyundecanoic acid | ↑ | 1.6844963 | 3.9116267 | ↑ | 1.4612388 | 4.1748894 | — | — | — |
Sinapinic acid | ↑ | 1.656589 | 6.9473043 | — | — | — | — | — | — |
Palmitelaidic acid | ↑ | 1.5698803 | 3.110861 | ↑ | 1.8738145 | 5.9860247 | — | — | — |
2,5-dihydroxy-pyrazine | ↑ | 1.5403106 | 4.2222789 | ↑ | 1.3516741 | 3.3262985 | — | — | — |
Serine | ↑ | 1.519169 | 4.7459609 | ↑ | 1.3870187 | 5.5135532 | — | — | — |
L-tyrosine | ↑ | 1.4727852 | 4.5086267 | — | — | — | — | — | — |
Glutaric acid | ↑ | 1.4364389 | 2.9498953 | ↑ | 1.4287323 | 0.0002747 | — | — | — |
Harmol | ↑ | 1.4304145 | 3.5141869 | ↑ | 1.5864991 | 3.8633207 | — | — | — |
1-monoolein | ↑ | 1.4216273 | 3.310974 | ↑ | 1.8819259 | 3.885722 | — | — | — |
5,6-dihydrouracil | ↑ | 1.4196253 | 2.8252254 | ↑ | 1.4071193 | 2.8933881 | — | — | — |
Cellobiose | ↑ | 1.3719033 | 2.8404857 | ↑ | 1.7359587 | 3.159995 | — | — | — |
L-alanine | ↑ | 1.3621227 | 3.1181925 | — | — | — | — | — | — |
L-threonine | ↑ | 1.3514955 | 3.2680077 | — | — | — | — | — | — |
Isochlorogenic acid | ↑ | 1.350742 | 3.1388726 | ↑ | 1.8510563 | 4.2665425 | — | — | — |
Octanol | ↑ | 1.3007048 | 3.7913169 | — | — | — | — | — | — |
Kynurenic acid | ↑ | 1.2605511 | 2.2365276 | ↑ | 1.2769744 | 1.9100572 | — | — | — |
Guanosine | ↑ | 1.2306354 | 6.05041 | — | — | — | — | — | — |
Coniferin | ↑ | 1.2235195 | 1.8189517 | ↑ | 1.1784971 | 1.7412512 | — | — | — |
3-ureidopropionate | ↑ | 1.1998851 | 2.244907 | ↑ | 1.1167604 | 2.4315167 | — | — | — |
N-acetyl-d-glucosamine | ↑ | 1.1783531 | 0.4978428 | ↓ | 1.2675313 | 0.5062986 | — | — | — |
3-methoxybenzenepropanoic acid | ↓ | 1.1679431 | 0.5008457 | ↓ | 1.2335976 | 0.5174785 | — | — | — |
Tert-butylketene acetal | ↑ | 1.1619286 | 2.4896159 | ↑ | 1.1348412 | 2.0879014 | — | — | — |
2,5-di-tert-butyl-4-(hydroxy)phenol | ↑ | 1.1436208 | 1.9179271 | — | — | — | — | — | — |
Citrulline | ↑ | 1.1062984 | 2.9526565 | — | — | — | — | — | — |
4-fluoroaniline | ↓ | 1.0991659 | 0.6259472 | ↓ | 1.2653923 | 0.622478 | — | — | — |
Desyrel | ↑ | 1.0767652 | 2.5467642 | — | — | — | — | — | — |
N-acetylgalactosamine | ↑ | 1.0033183 | 2.1562394 | — | — | — | — | — | — |
Allose | — | — | — | ↑ | 2.2462404 | 87.409938 | — | — | — |
Sedoheptulose | — | — | — | ↑ | 2.2008564 | 75.499701 | — | — | — |
Linoleic acid | — | — | — | ↑ | 1.807242 | 4.4486483 | — | — | — |
Erucic acid | — | — | — | ↑ | 1.7351023 | 4.2904694 | — | — | — |
Oleic acid | — | — | — | ↑ | 1.7323362 | 4.2786805 | — | — | — |
L-asparagine | — | — | — | ↑ | 1.6858296 | 7.709766 | — | — | — |
2,3-dihydrobutyraldehyde 1-phosphate | — | — | — | ↑ | 1.4891256 | 11.227292 | — | — | — |
Pinitol | — | — | — | ↓ | 1.4705164 | 0.4544384 | — | — | — |
Glucose | — | — | — | ↑ | 1.3543269 | 8.1765831 | — | — | — |
Beta-alanine | — | — | — | ↓ | 1.3519891 | 0.6505915 | — | — | — |
3,6-dimethyl-2,5-dihydroxy-pyrazine | — | — | — | ↑ | 1.4071193 | 2.8933881 | — | — | — |
Phosphoenolpyruvate | — | — | — | ↑ | 1.3016116 | 4.7976509 | — | — | — |
Uridine | — | — | — | ↑ | 1.2924314 | 3.1511016 | — | — | — |
D-myo-inositol 4-phosphate | — | — | — | ↑ | 1.2578405 | 2.7408571 | — | — | — |
3,17,20-trihydroxy-pregn-5-en-11-one | — | — | — | ↓ | 1.2392217 | 0.5580432 | — | — | — |
L-cysteine | — | — | — | ↑ | 1.195239 | 6.0274871 | — | — | — |
1-kestose | — | — | — | ↑ | 1.1745532 | 4.5597282 | — | — | — |
Myristyl myristate | — | — | — | ↓ | 1.172666 | 0.5895407 | — | — | — |
D-ribose | — | — | — | ↑ | 1.1436302 | 2.449142 | — | — | — |
Ribitol | — | — | — | ↑ | 1.1409947 | 1.908032 | — | — | — |
Hypoxanthine | — | — | — | ↓ | 1.1263163 | 0.5952783 | — | — | — |
5-methyluridine | — | — | — | ↑ | 1.0666299 | 3.0846661 | — | — | — |
Dehydroabietic acid | — | — | — | ↑ | 1.014755 | 1.6333058 | — | — | — |
1,5-anhydroglucitol | — | — | — | ↑ | 1.0075411 | 1.5409589 | — | — | — |
Abbreviations:
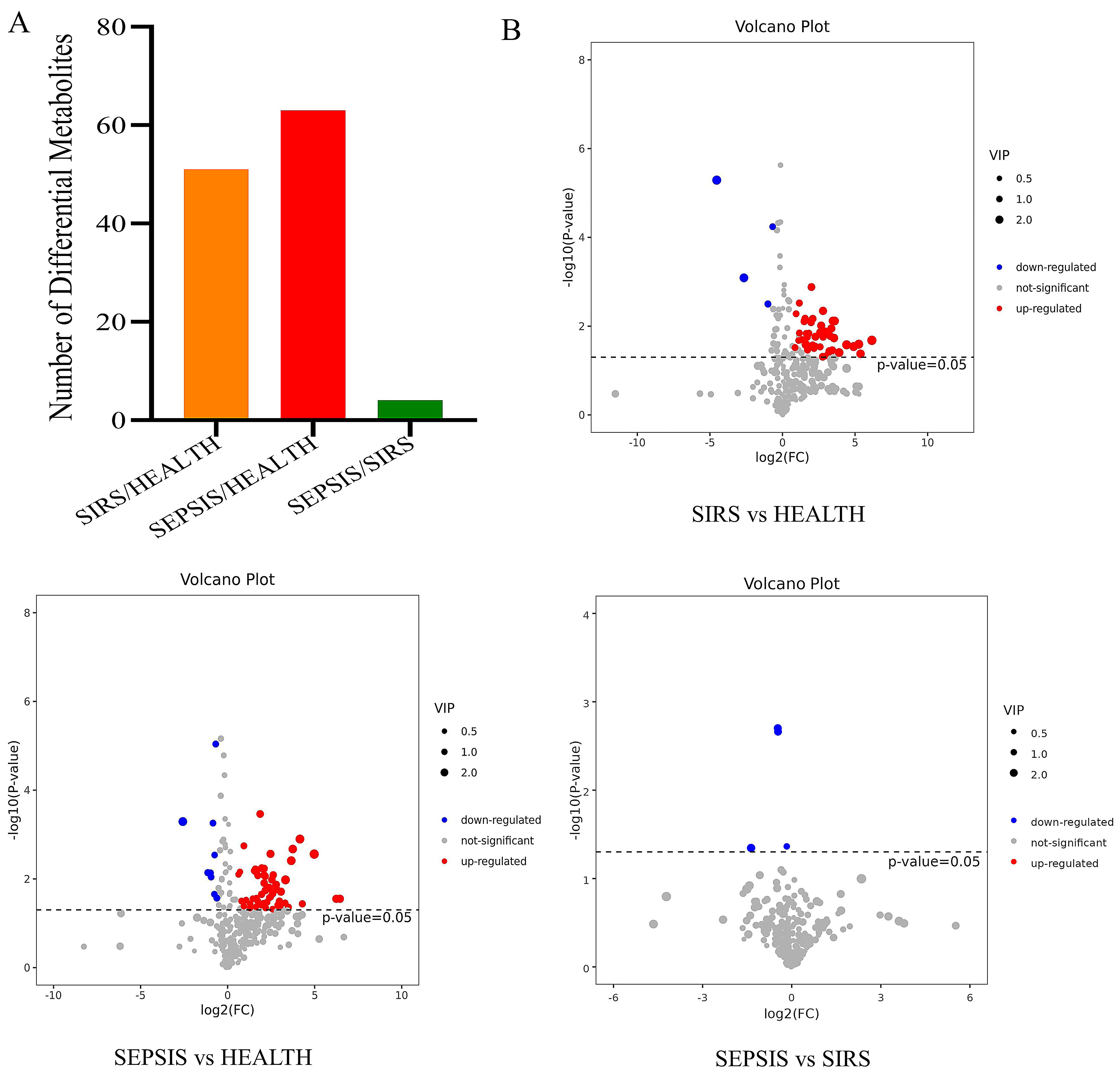
Differential metabolites were detected by GC-MS. (A) The histogram shows the quantity of differential metabolites. (B) The volcanic map visualizes changes in differential metabolites.
The load map can be used to identify the strength of influence of the metabolites in the comparison group. The abscissa of the S-plot is the characteristic value of the effect of the metabolite on the comparison group, and the ordinate is the correlation between the sample score and the metabolite. As the eigenvalues and correlations are both positive and negative, all points in the visualized graph are distributed in the first and third quadrants (similar to the “s” shape) and are called S-plots. The closer the metabolite is to the upper-right corner and lower-left corner, the more significant the difference. In the S-plot of the diagnostic model, we identified methyl 3-o-acetyl-d-galactopyranoside and N-acetylputrescine as candidate biomarkers for distinguishing between SIRS and healthy controls, as well as between sepsis and healthy controls (Fig. 3A). Correlation analysis using the Pearson correlation coefficient was used to evaluate the correlation between the different metabolites. The results showed a significant positive correlation between methyl 3-o-acetyl-d-galactopyranoside and N-acetylputrescine levels (Fig. 3B).
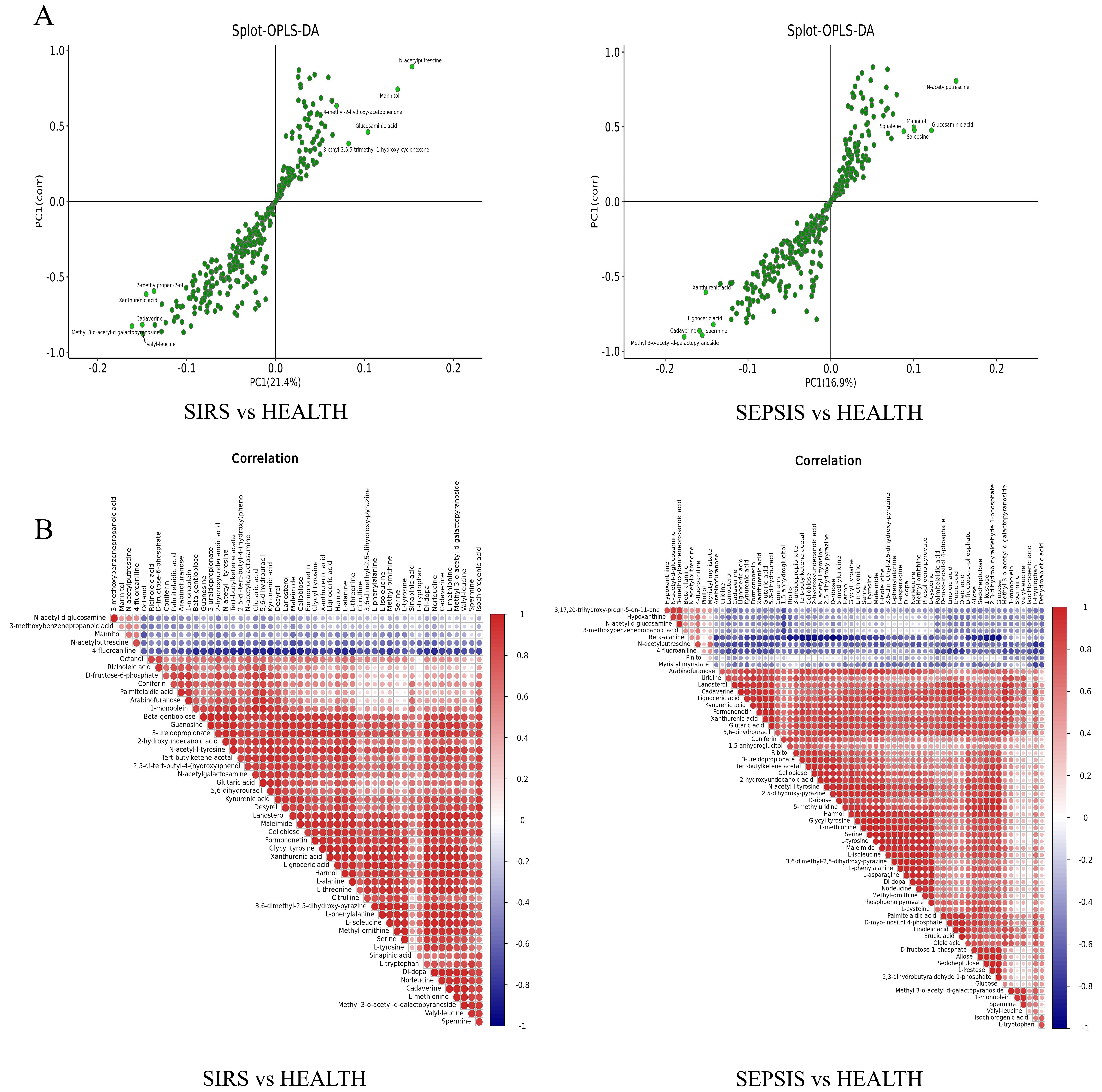
The S-plot was used to screen the potential biomarkers distinguishing between SIRS, sepsis, and healthy controls and the correlation between differential metabolites. (A) The S-plot was used to select the potential biomarkers distinguishing between SIRS, sepsis, and healthy controls. (B) Correlation analysis was used to measure the degree of correlation between differential metabolites. The red dot indicates positive correlation, and the blue dot indicates negative correlation.
To determine a simplified diagnostic biomarker to use in differentiating between SIRS and sepsis, ROC analysis was carried out, and the area under the curve (AUC) of each candidate metabolite was calculated. 2-deoxy-d-erythro-pentofuranose-5-phosphate was the best predictor of SIRS and sepsis (AUC = 0.9297, 95% confidence interval: 0.8069–1.0000), indicating its strong diagnostic performance. The AUC of terbutaline was 0.9063, and that of allantoic acid was 0.7969, indicating that terbutaline and allantoic acid were good discriminators between SIRS and sepsis patients (Fig. 4A). Correlation analysis showed a significant positive correlation between 2-deoxy-d-erythro-pentofuranose-5-phosphate and terbutaline (r = 0.66108) and between 2-deoxy-d-erythro-pentofuranose-5-phosphate and allantoic acid (r = 0.55947) (Fig. 4B).
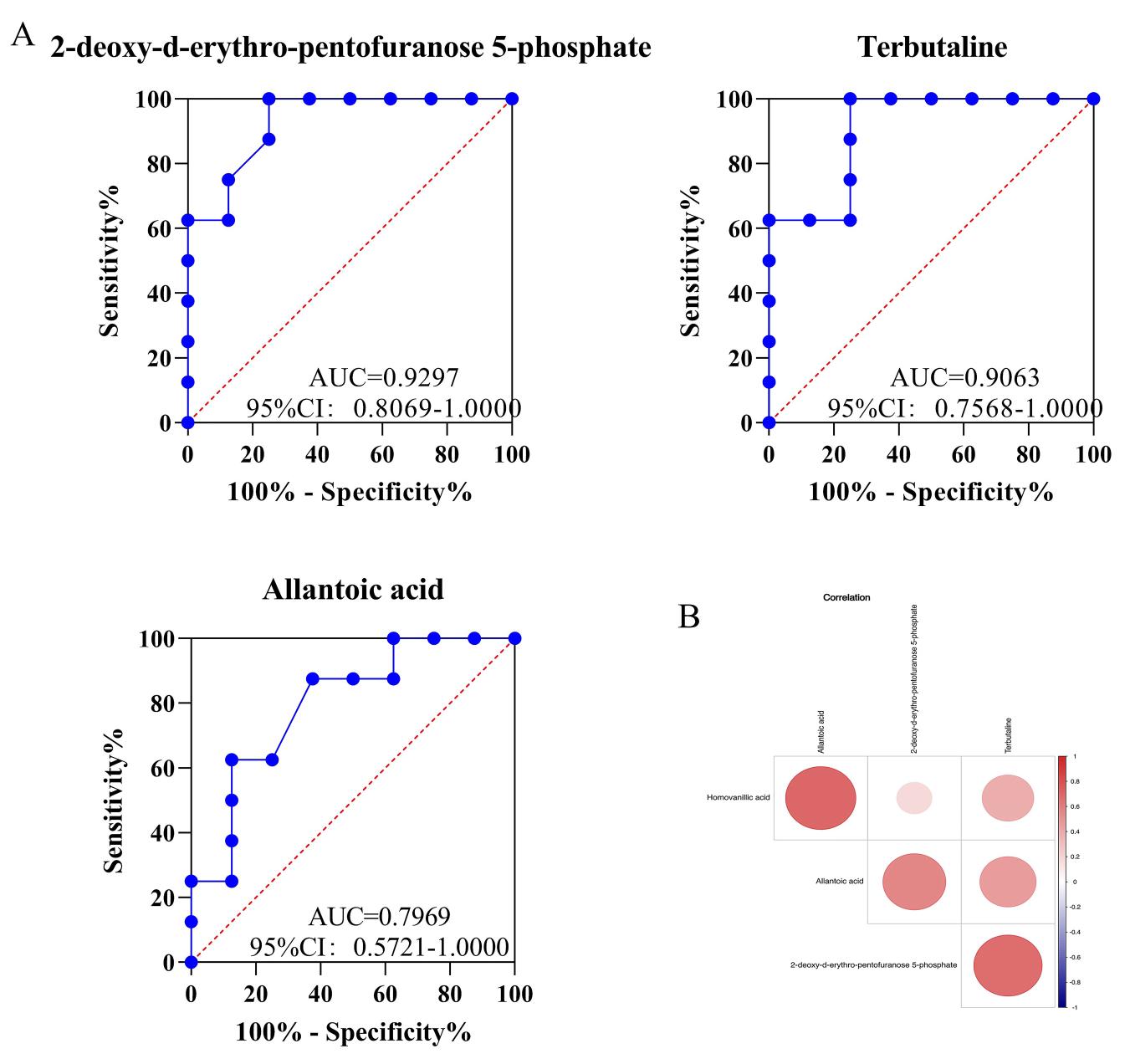
The ROC was used to analyze the potential biomarkers between SIRS and sepsis patients and the correlation between differential metabolites. (A) The ROC was used to analyze the potential biomarkers for differentiating patients with SIRS and sepsis (ROC, receiver operating characteristic). (B) Correlation analysis was used to measure the degree of correlation between differential metabolites.
To determine the metabolic pathways enriched in SIRS and sepsis, differential metabolites were subjected to pathway analysis using the Kyoto Encyclopedia of Genes and Genomes database (KEGG). These results are shown in Fig. 5. Eighteen pathways were enriched in SIRS patients compared to healthy controls. Nine metabolic pathways were related to amino acid biosynthesis and metabolism, including protein digestion and absorption; aminoacyl-tRNA biosynthesis; beta-alanine metabolism; phenylalanine, tyrosine, and tryptophan biosynthesis; glycine, serine, and threonine metabolism; cysteine and methionine metabolism; valine, leucine, and isoleucine biosynthesis; tryptophan metabolism; and glutathione metabolism. There were seven atypical pathways which are related to the use of glucocorticoids and catecholamines, including cocaine addiction; amphetamine addiction; alcoholism; prolactin signaling pathway; dopaminergic synapses; Parkinson’s disease and melanogenesis. The other two important pathways were the central carbon metabolism in cancer and the biosynthesis of pantothenate and coenzyme A (CoA).
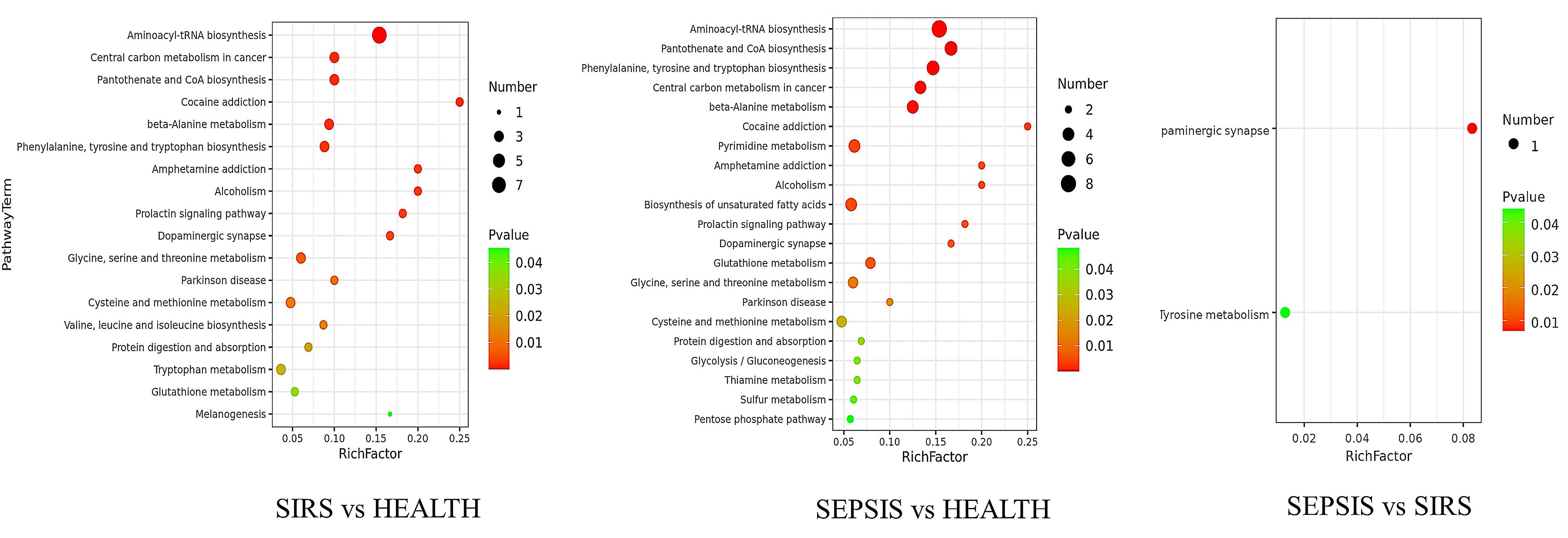
The bubble diagram was analyzed using KEGG metabolism pathway enrichment. The p value is the significance of enrichment of this metabolic pathway. The ordinate is the name of the metabolic pathway; the abscissa is the rich factor (rich factor = the number of significantly different metabolites/the total number of metabolites in this pathway). The larger the rich factor, the more metabolites enriched in the pathway. The color from green to red indicates that p-value decreases in turn.
There were 21 enriched pathways in sepsis patients compared with healthy controls. Eight metabolic pathways were related to amino acid biosynthesis and metabolism, including protein digestion and absorption; aminoacyl-tRNA biosynthesis; beta-alanine metabolism; phenylalanine, tyrosine, and tryptophan biosynthesis; glycine, serine, and threonine metabolism; cysteine and methionine metabolism; glutathione metabolism; and thiamine metabolism. Two pathways related to glucose metabolism (glycolysis/gluconeogenesis and the pentose phosphate pathway), one pathway related to pyrimidine metabolism, and one was related to the biosynthesis of unsaturated fatty acids. Three other important pathways were identified: central carbon metabolism in cancer; pantothenate and CoA biosynthesis, and sulfur metabolism. There were six atypical pathways, including cocaine addiction; amphetamine addiction; alcoholism; prolactin signaling pathway; dopaminergic synapses; and Parkinson’s disease.
Only two pathways were enriched in sepsis compared to SIRS. One was the dopaminergic synapse, and the other was tyrosine metabolism.
In order to further verify the clinical value of
2-deoxy-d-erythro-pentofuranose-5-phosphate, we analyzed the correlation between
2-deoxy-d-erythro-pentofuranose-5-phosphate and some clinical indicators.
Analysis showed no significant correlation between
2-deoxy-d-erythro-pentofuranose-5-phosphate and clinical indicators, such as
IL-6, IL-1
Correlation | 2-deoxy-d-erythro-pentofuranose-5-phosphate | |
IL-6 (pg/mL) | r = –0.2383 | p = 0.5698 |
IL-1 |
r = –0.3532 | p = 0.1247 |
TNF- |
r = –0.4734 | p = 0.2241 |
CRP (mg/L) | r = –0.4419 | p = 0.2730 |
Platelet (× 10 |
r = –0.2685 | p = 0.5202 |
Ca |
r = –0.3535 | p = 0.1249 |
Lactate (mmol/L) * | r = –0.8756 | p = 0.0044 |
Monocyte (× 10 |
r = –0.2208 | p = 0.5995 |
Lymphocyte (× 10 |
r = –0.0445 | p = 0.9166 |
Abbreviations: IL-6, interleukin-6; IL-1
Sepsis is one of the most common diseases and is associated with high mortality. Early identification of sepsis from SIRS is beneficial for early intervention, which is key to achieving improved outcomes [12]. Although many biomarkers related to sepsis have been reported in the past, their predictive accuracy remains unclear. Application of available biomarkers is urgently needed for early identification of patients at the highest risk of having poor outcome. To our knowledge, our work can contribute to the discovery of new biomarkers with significant efficacy and predictive value in the diagnosis of sepsis using a GC-MS-based metabolomic method.
Our results showed that 51 metabolites were clearly distributed in the SIRS group and were associated with 18 metabolic pathways compared with healthy controls. Furthermore, 63 metabolites in the sepsis group were significantly distinguished from those in the healthy controls and were associated with 21 metabolic pathways. Among these differential metabolites and pathways, there were 14 metabolic pathways containing metabolites that were enriched in both the SIRS and sepsis groups. Nine metabolic pathways in the SIRS patients and eight in the sepsis patients were related to amino acid biosynthesis and metabolism. This indicates that when SIRS and sepsis occur, both the biosynthesis and metabolism of amino acids are activated. Compared with SIRS, the biosynthesis of unsaturated fatty acids and glycolysis/gluconeogenesis pathways were enriched in sepsis. The reason for which the glycolysis/gluconeogenesis pathway enriched in sepsis is that the body needs more energy to defeat inflammation. This is referred to as the Warburg effect which is an essential component of metabolic reprogramming during sepsis. Aerobic glycolysis, which generates energy through glycolysis rather than through oxidative phosphorylation, is a hallmark of sepsis [13, 14].
Our results showed strong differentiation between SIRS, sepsis, and healthy controls using the PLS-DA and OPLS-DA models. Based on the S-plot results, methyl 3-o-acetyl-d-galactopyranoside and N-acetylputrescine in the lower-left corner and upper-right corner are differentiators between SIRS, sepsis, and healthy controls. Methyl 3-o-acetyl-d-galactopyranoside is a monosaccharide, and galactose is usually present in the body in the form of d-galactopyranoside. Galactose interacts with ATP to form UDP glucose and 1-phosphate glucose, participate in glucose metabolism, and provide cellular energy [15]. N-acetylputrescin is a polyamine that is metabolized by amino acids such as lysine, L-arginine, and L-ornithine; plasma n-acetylputrescine has been shown to be a potential biomarker of lung cancer and can be used to evaluate the efficacy of antitumor drugs [16]. A previous study demonstrated that plasma polyamines, including n-acetylputrescine, may provide means to identify patients with multiple endocrine neoplasia type 1 who are harboring or developing an aggressive form of the disease [17]. According to our results, methyl 3-o-acetyl-d-galactopyranoside and N-acetylputrescine can be used as diagnostic biomarkers for SIRS or sepsis. Detection of methyl 3-o-acetyl-d-galactopyranoside and N-acetylputrescine indicated the patient was either in the SIRS period or quickly progressing to sepsis. Six atypical pathways were detected in SIRS and sepsis, including cocaine addiction, amphetamine addiction, alcoholism, prolactin signaling pathway, dopaminergic synapse, and Parkinson’s disease, which may be related to use of glucocorticoids and catecholamines.
However, the distinction between SIRS and sepsis is a complicated task. To identify the differential capacity of metabolites in separating sepsis from SIRS, PLS-DA and OPLS-DA models were conducted for patients with SIRS and sepsis. Four differential metabolites, including 2-deoxy-d-erythro-pentofuranose-5-phosphate, terbutaline, allantoic acid, and homovanillic acid (HVA), were enriched in the dopaminergic synapse and tyrosine metabolism pathways. 2-deoxy-d-erythro-pentofuranose-5-phosphate is an intermediate product of glycol metabolism. Allantoic acid is an important part of purine degradation, terbutaline is a beta-2-adrenoreceptor agonist, and HVA is a metabolite produced by dopamine and tyrosine metabolism. The reason for dopaminergic synapse enrichment may be that patients with septic shock need to stimulate dopamine secretion to increase their blood pressure. The other is tyrosine metabolism, which is related to many diseases such as allergic airway inflammation, glioma, and pulmonary tuberculosis [18, 19, 20]. Furthermore, in order to determine a simplified diagnostic biomarker in differentiating between SIRS and sepsis, ROC curves were analyzed. The results showed that the AUC of 2-deoxy-d-erythro-pentofuranose-5-phosphate was 0.9297, that of terbutaline was 0.9063, and that of allantoic acid was 0.7969, indicating their good predictive capacity for sepsis. To further verify the clinical value of 2-deoxy-d-erythro-pentofuranose-5-phosphate, we analyzed the correlation between 2-deoxy-d-erythro-pentofuranose-5-phosphate and clinical indicators. A significant negative correlation between 2-deoxy-d-erythro-pentofuranose-5-phosphate and lactate was identified (r = –0.8756, p = 0.0044). This means that as more 2-deoxy-d-erythro-pentofuranose-5-phosphate is consumed, more lactic acid will be produced. This may be related to metabolic pathway shifts to glycolysis which generated lactate. 2-deoxy-d-erythro-pentofuranose-5-phosphate is substrate produced from pentose phosphate pathway. 2-deoxy-d-erythro-pentofuranose-5-phosphate decreased in septic shock indicated that pentose phosphate pathway is active because of 2-deoxy-d-erythro-pentofuranose-5-phosphate is continuously consumed. Pentose phosphate pathway, which can produce a large amount of NADPH, and the intermediates as raw materials for glycolysis, is an important pathway for glucose metabolism [14]. It is the characteristic of the Warburg effect of sepsis. Our results demonstrated that 2-deoxy-d-erythro-pentofuranose-5-phosphate may be the candidate biomarker to distinguish sepsis from SIRS which may related with metabolic pathway change in sepsis.
Our study suggested that 2-deoxy-d-erythro-pentofuranose-5-phosphate could be used as a candidate biomarker to distinguish sepsis from SIRS and provided a potential method for the early identification of sepsis using GC-MS. Our study yielded useful findings but had some limitations. First, our sample size was small and for further verification, we would need a larger number of samples. Second, in order to validate the clinical utility and specificity of metabolites as biomarkers for sepsis also need to be verified. Moreover, for clarify the specific function of this metabolite, some molecular biology experiments need to be improved in the future.
The dataset that used, analyzed, or generated in this research is available from the corresponding author upon reasonable request.
CC and RW designed the research study. CC and XM performed the research. YZ collected the clinical data. JZ analyzed the data. All authors contributed to editorial changes in the manuscript. All authors read and approved the final manuscript.
The ethics committee of Shanghai General Hospital reviewed and approved the study protocol and procedures. All participants signed an informed consent form before the procedure. All procedures were performed in accordance with the Declaration of Helsinki (ethics approval number: 2022SQ146).
Thanks to Professor Zhengfeng Yang (Clinical Translational Research Institute of Shanghai General Hospital) for their guidance and help during the experiment.
This research was supported by the Construction and application of integrated management model for emergency and critical care (Grant No. SHDC 22021211), Construction of Shanghai infectious disease clinical diagnosis and treatment center and network system (Grant No. 04.05.01.21.03), Medical engineering cross research fund of “Jiaotong University star” program of Shanghai Jiaotong University (Grant No. YG2021QN84).
The authors declare no conflict of interest.
Publisher’s Note: IMR Press stays neutral with regard to jurisdictional claims in published maps and institutional affiliations.