Academic Editor: Elisa Belluzzi
Objective: The aim of this study was to identify potentially important
Rheumatoid arthritis (RA) targets related to immune cells based on bioinformatics
analysis, and to identify small molecules of traditional Chinese medicine (TCM)
associated with these targets that have potential therapeutic effects on RA.
Methods: Gene expression profile data related to RA were downloaded from
the Gene Expression Omnibus (GSE55235, GSE55457, and GSE77298), and datasets were
merged by the batch effect removal method. The RA key gene set was identified by
protein-protein interaction network analysis and machine learning-based feature
extraction. Furthermore, immune cell infiltration analysis was carried out on all
DEGs to obtain key RA markers related to immune cells. Batch molecular docking of
key RA markers was performed on our previously compiled dataset of small
molecules in TCM using AutoDock Vina. Moreover, in vitro experiments
were performed to examine the inhibitory effect of screened compounds on the
synovial cells of an RA rat model. Results: The PPI network and feature
extraction with machine learning classifiers identified eight common key RA
genes: MYH11, CFP, LY96, IGJ,
LPL, CD48, RAC2, and CSK. RAC2 was
significantly correlated with the infiltration and expression of five immune
cells, with significant differences in these immune cells in the normal and RA
samples. Molecular docking and in vitro experiments also showed that
sanguinarine, sesamin, and honokiol could effectively inhibit the proliferation
of RA rat synovial cells, also could all effectively inhibit the secretion of
TNF-
Rheumatoid arthritis (RA) is a chronic, systemic, inflammatory progressive autoimmune disease occurring in the synovial joints and other organ systems. Although the underlying pathogenesis remains unclear, RA is generally considered to be caused by infections and inflammatory mediators [1, 2]. The incidence of RA increases with age, which most commonly manifests in adults aged 35–50 years. The global prevalence rate is approximately 0.5%–1.0% and the annual average incidence rate is 0.02%–0.05% [3], which is approximately 3 times greater in women than that in men [4]. At present, western medicines are the main treatment for RA in clinical practice; however, these drugs are associated with high toxicity and side effects, as well as high treatment costs [5, 6]. Traditional Chinese medicine (TCM) has a long history of treating RA, and rich experience has accumulated in clinical application [7]. In recent years, studies have shown that anti-RA traditional Chinese medicinal materials and compound prescriptions have anti-inflammatory, analgesic, immunomodulatory, as well as multi-level and multi-link therapeutic effects, with additional advantages such as high safety, less adverse reactions, and low price [8, 9].
With recent developments of molecular biology, immunology, and genetics, the pathogenesis of RA has been shown to be related to the changes and influences of genetic, bacterial, and viral factors, as well as associated immune cells (e.g., T lymphocytes, B lymphocytes) and cytokines. Indeed, immune cell infiltration plays an important role in the development and progression of RA. For example, CD4+ T cells account for the main inflammatory cell type invading the synovial tissue, which participate in the pathological process of RA [10]. Therefore, from the viewpoint of the immune system, it is of great value to clarify the molecular mechanism of RA and identify new therapeutic targets by evaluating the infiltration degree of immune cells and determining the differences in components among infiltrating immune cells.
Moreover, given the above-mentioned advantages of many TCMs and their components, it is of great practical significance to identify potential effective anti-RA components from a large number of TCMs reported to have pharmacological effects, and directly or indirectly correlate these components with RA immune cell infiltration therapy. In other words, the core problem to be solved at present is to identify new key therapeutic targets related to immune infiltration in RA, and to screen out the effective components of TCMs that act on these targets with potential therapeutic effects.
Toward this end, in this study, we analyzed public gene expression profile data from the Gene Expression Omnibus (GEO), and screened out a differentially expressed gene (DEG) set for RA. We further applied bioinformatics approaches, including protein–protein interaction (PPI) network analysis, machine learning, and immune cell infiltration calculation, to identify new potential targets of RA. Based on our previously compiled active ingredient set of TCMs, associated small molecules in TCM were screened out according to molecular fingerprint similarity and molecular docking technology. Finally, these targets were verified and analyzed by in vitro cell experiments using synovial cells of an RA rat model. The flow chart of the study design is provided in Fig. 1.
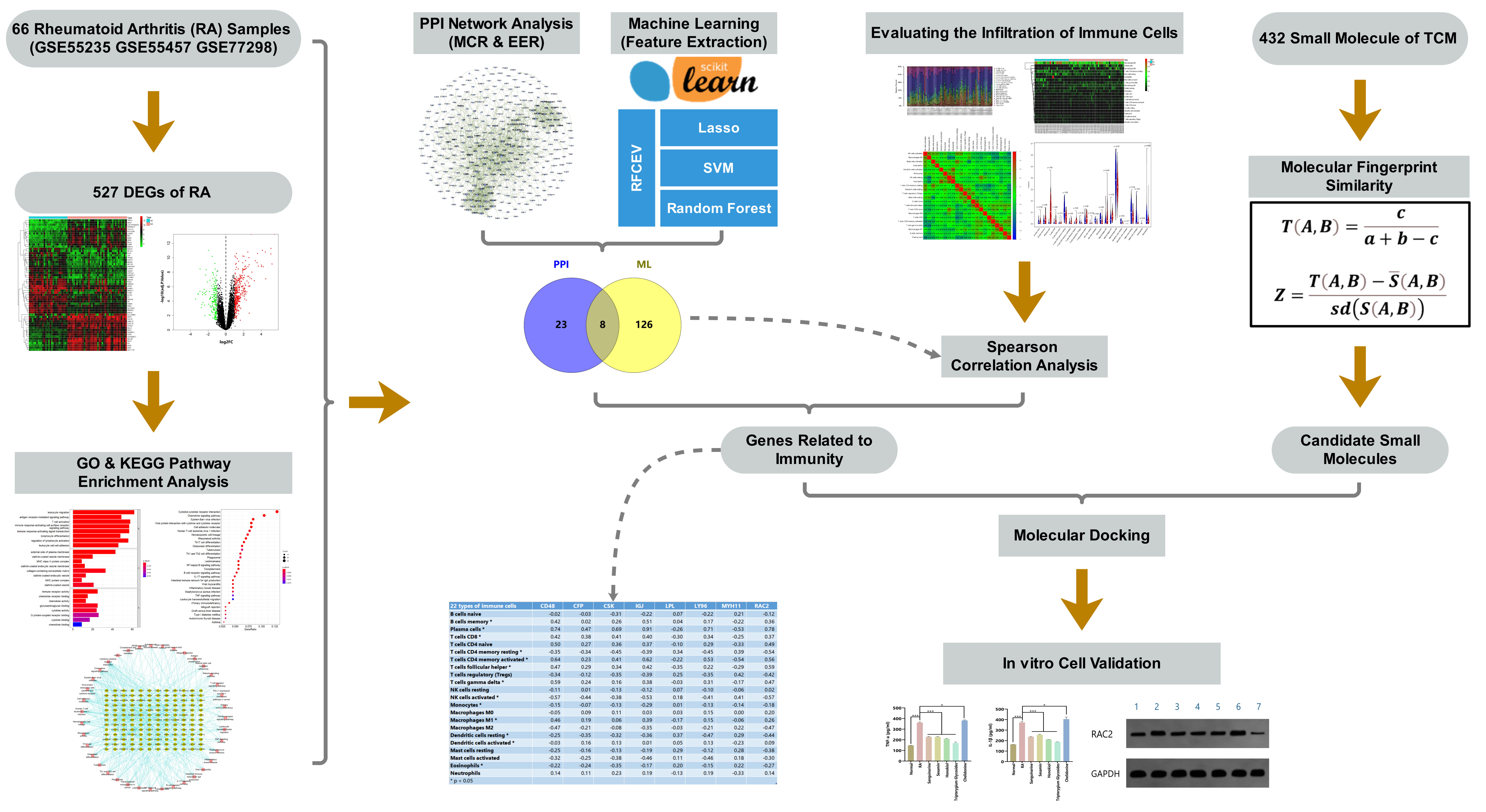
Flow chart of the study design.
The search term “Rheumatoid Arthritis” was queried in the GEO to obtain expression profile data of genes related to RA from three datasets GSE55235, GSE55457, and GSE77298 based on 10 normal samples and 10 RA samples, 10 normal and 13 RA samples, and 7 normal and 16 RA samples, respectively.
The raw gene expression profile data of all three RA GEO datasets were
downloaded and subjected to pre-processing, such as standardization, correction,
and gene name annotation using the limma package (version 3.42.2). The
data were combined, and the sva package (version 3.36.0) was used to
perform batch-removal correction on all chip data. DEGs in RA were screened
according to
The clusterProfiler package (version 3.14.3) was used for Gene
Ontology (GO) functional annotation and Kyoto Encyclopedia of Genes and Genomes
(KEGG) pathway enrichment analysis of all DEGs according to p
All DEGs identified in RA were imported into the STRING (version 11.0, https://string-db.org/) database to construct a PPI network of RA targets, with the parameters set as the following: organism, Homo sapiens; combined score threshold, 0.7. The PPI network for RA was plotted using Gephi (version 0.9.2, https://gephi.org/).
To identify key RA genes in the PPI network, we used two methods for considering the network node degree, which differ from previous methods in that they focus on examining the efficiency of nodes in the disease network.
The maximum connected reduction (MCR
where N represents the total number of nodes connected to the network
before node deletion, and N
Similarly, the efficiency relative reduction (ERR
where E is the average value of the reciprocal of the shortest distance
between network nodes, d
We included the top genes in terms of the above two indicators into the RA key gene set based on PPI network efficiency analysis.
According to the sample classification of the normal group and RA group, we used
the RFECV function in the sklearn
The key RA gene sets based on PPI network analysis and feature extraction were intersected again to obtain the core gene set of RA. To verify the disease classification effect of these core genes, the three different machine learning classifiers mentioned above were used to predict RA classification, which was evaluated by the area under the receiver operating characteristic (ROC) curve (AUC) value. All classification prediction analyses were performed on the Anaconda3 (https://www.anaconda.com/).
Based on GEO gene expression profile data, CIBERSORT Toolkit (version 1.03) was used to analyze immune cell infiltration in RA, and the abundances of 22 immune cell types in RA were calculated. The abundance difference heat map, correlation heat map, and violin plot of immune cells in each sample were plotted using the pheatmap (version 1.0.12), corrplot (version 0.84), and vioplot (version 0.3.5) packages, respectively. Spearman correlation analysis was used to analyze the correlations between 22 types of infiltrating immune cells and key genes to identify genes closely correlated with immune cell infiltration.
The chemical information and data of each component in our previously compiled active ingredient set of natural traditional Chinese medicines proven to have clear pharmacological effects, including 432 small molecules [11], were obtained through the PubChem database (https://pubchem.ncbi.nlm.nih.gov/), including the English name, CAS, PubChem CID, molecular formula, canonical Simplified Molecular-Input Line-Entry System (SMILES), and SDF file.
Before molecular docking of TCM small molecules according to known target receptors, the receptor file containing the original ligands was downloaded from the PDB database (https://www.rcsb.org/); molecules with a similar structure to the original ligand were considered as the potential and optimal candidates for further screening using molecular fingerprint similarity.
According to canonical SMILES, the molecular fingerprint of each compound was calculated using DRAGON (version 7.0, Kode, Pisa, Italy) software, and the similarity between the original ligands and TCM small molecules was calculated by the Tanimoto coefficient:
Among them, a is the number of descriptors of the basic fragment in compound A, b is the number of descriptors of the basic fragment in compound B, and c is the number of descriptors of the basic fragment shared by compounds A and B.
Further, the molecular fingerprint similarity coefficient
where
The ligand SDF file of all collected TCM small molecules was downloaded from the PubChem, wherein the three-dimensional (3D) structure file was directly converted into a PDB file using PyMOL (version 1.6, https://pymol.org/2/) and the two-dimensional file was converted into a PDB file in 3D format using Chem3D (version 19.0, PerkinElmer, Waltham, Massachusetts, USA). The PDB file of target receptors was downloaded from the PDB database, and key target proteins were pre-processed using PyMOL to remove impurities such as water molecules. The PDB file of receptors and ligands was then converted to a PDBQT file using AutoDockTools (version 1.5.6, https://autodock.scripps.edu/).
The coordinates of the central position for molecular docking were determined by
referring to the binding site of the protein receptor and the original ligand,
and the docking center parameter was defined as a box size of 30
Eight female clean-grade Wistar rats (each weigh = 120
3 mg/mL of bovine CII in 0.1 M acetic acid was emulsified with an equal volume of complete Freund’s adjuvant (CFA) to create a stable CII/CFA emulsion (10 mg/mL). Four rats were injected (i.d.) with the CII/CFA emulsion in the back (0.1 mL) and tail (0.1 mL); the day of administration was referred to as “day-0”. On day-7, all rats received 0.1 mL of a CII/CFA booster via tail injection (i.d.).
After 14 days of modeling, the degree of posterior plantar redness and swelling
of CIA rats was significantly different from that of the normal group according
to measurement of the plantar volume and Arthritis Score (p
All rats were killed, and soaked by 75% alcohol for 2 minutes. Fur was removed
from the rats knee and muscle was separated. The synovial layer tissue was
removed and was placed in physiological saline with Penicillin-Streptomycin for 5
minutes. The synovial layer tissue from different group was rinsed by PBS, and
then cut into pieces on petri dish. We used collagenase II mixed with 10% FCS to
digest the pieces of synovial layer, and then added DMEM to mix all evenly. After
the synovial tissue passing through 200-mesh sieve, we added 4 mL DMEM to mix
these synovial cells well. After Synovial cells were cultured in the incubator,
cells were harvested and adjusted to a concentration of 1
Rat RA synovial cells were cultured in RPMI-1640 medium containing 10% fetal
bovine serum (Bio IND, Kibbutz Beit Haemek, Israel), inoculated in 96-well
culture plates at 2000 cells/well, and then various compounds containing
sanguinarine, chelidonine, sesamin, honokiol, and tripterygium glycoside
(National Institutes for Food and Drug Control, Beijing, China) were added at
different concentrations (1000, 500, 250, 125, 62.5, 31.25, 0
The cells of CIA rats were subjected to maintenance culture in RPMI-1640 medium
containing 10% FBS on 6-well plates at 4
The cell samples treated with the various compounds as described above were collected, subjected to protein determination by the Bradford method, and boiled at 100 °C for 5 min in loading buffer. The samples were then loaded onto a 10% sodium dodecyl sulfate-polyacrylamide gel electrophoresis gel at equivalent volumes for electrophoresis, and the proteins on the gel were transferred onto cellulose acetate membranes using transfer buffer solution. The samples were blocked with 5% skimmed milk powder for 1 h, and rabbit derived anti rat RAC2 antibody (Abcam, Cambridge, England) was added at 1:1000. The samples were placed in a refrigerator at 4 °C overnight, and the goat anti rabbit IgG (H+L) (Beyotime, ShangHai, China) labeled with horseradish peroxidase was added at 1:1000. After washing in Tris-buffered saline with Tween, the samples were subjected to color development by enhanced chemiluminescence and images were captured and analysis using Fluorescence imaging system (CLiNX, Shanghai, China). In addition, Image J (version 1.80, https://imagej.nih.gov/ij/) was used to measure and analyze the gray level of WB expression.
One-way ANOVA for multiple comparisons, and Student’s t test for two
groups comparisons were utilized to analyze the significance of differences. Data
were analyzed by SPSS software (version 18.0, IBM Corp., Chicago, IL, USA),
results were considered as statistically significant if the p-value was
After the combination and batch removal correction of the GSE55235, GSE55457, and GSE77298 datasets, a total of 527 DEGs in RA were screened out, including 348 up-regulated genes and 179 down-regulated genes. The heat map and volcano plot for the expression levels of the DEGs are shown in Fig. 2.
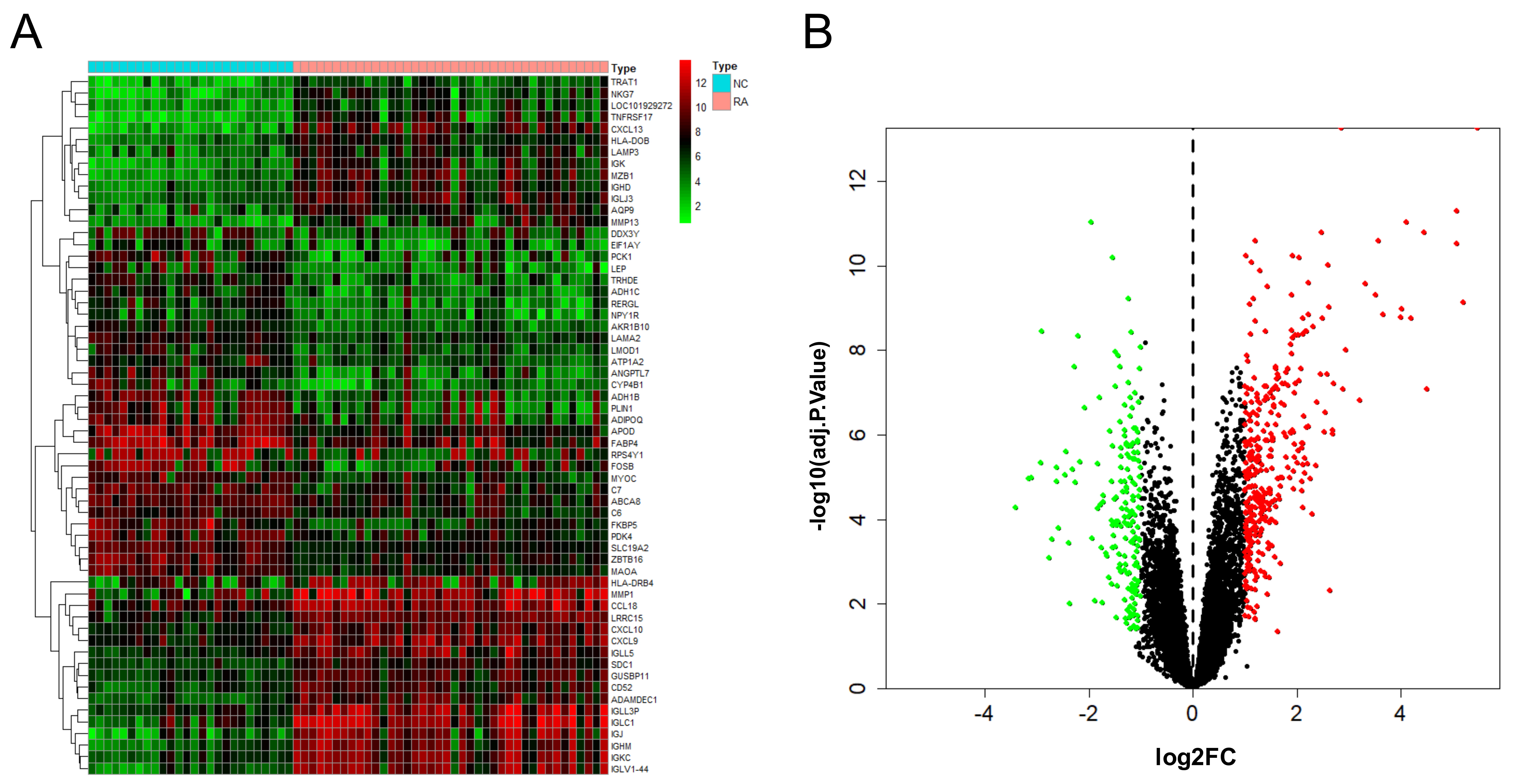
DEGs analysis results of RA. Heat map (A) and volcano plot (B) of expression analysis of 527 differentially expressed genes in rheumatoid arthritis (RA) compared with normal control (NC) samples after combination and batch removal of three groups of GEO chip datasets (GSE55235, GSE55457, and GSE77298).
Functional annotation and enrichment analysis suggested that the DEGs in RA are mainly involved in various immune-related functions and pathways. Among the 1233 associated GO biological functions, the DEGs were mainly concentrated in 24 GO functions, as shown in Fig. 3A., namely leukocyte migration (GO:0050900), T cell activation (GO:0042110), immune response-activating cell surface receptor signaling pathway (GO:0002429), immune response-activating signal transduction (GO:0002757), etc.
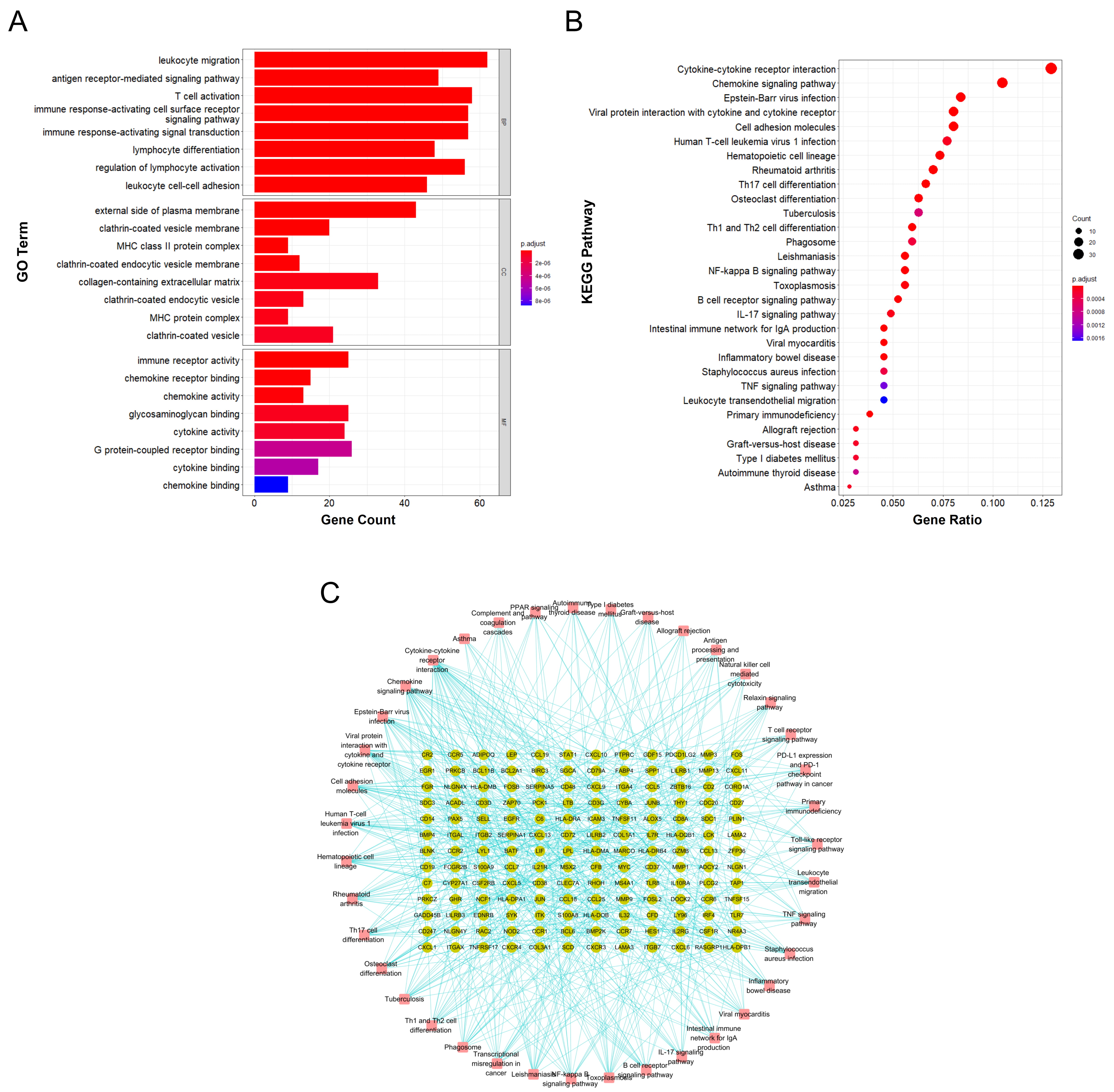
Functional annotation and enrichment analysis results of DEGs in RA. (A) GO functional enrichment analysis of differentially expressed genes in RA. (B) KEGG pathway enrichment analysis of differentially expressed genes in RA. (C) Gene-pathway enrichment network; the dots represent genes and the squares represent pathways.
KEGG pathway enrichment analysis suggested that the DEGs are mainly involved in 39 signaling pathways, including cytokine-cytokine receptor interaction (hsa04060), chemokine signaling pathway (hsa04062), Epstein-Barr virus infection (hsa05169), viral protein interaction with cytokine and cytokine receptor (hsa04061), and cell adhesion molecules (hsa04514); the top 30 main signaling pathways with enrichment of the highest number of genes are shown in Fig. 3B and the gene-enrichment pathway network is shown in Fig. 3C.
We imported the 527 DEGs into the STRING to obtain the PPI network of RA, as shown in Fig. 4A. Two types of importance degree values of all genes in the PPI network were obtained using the MCR and ERR algorithms, respectively, as shown in Fig. 4B.
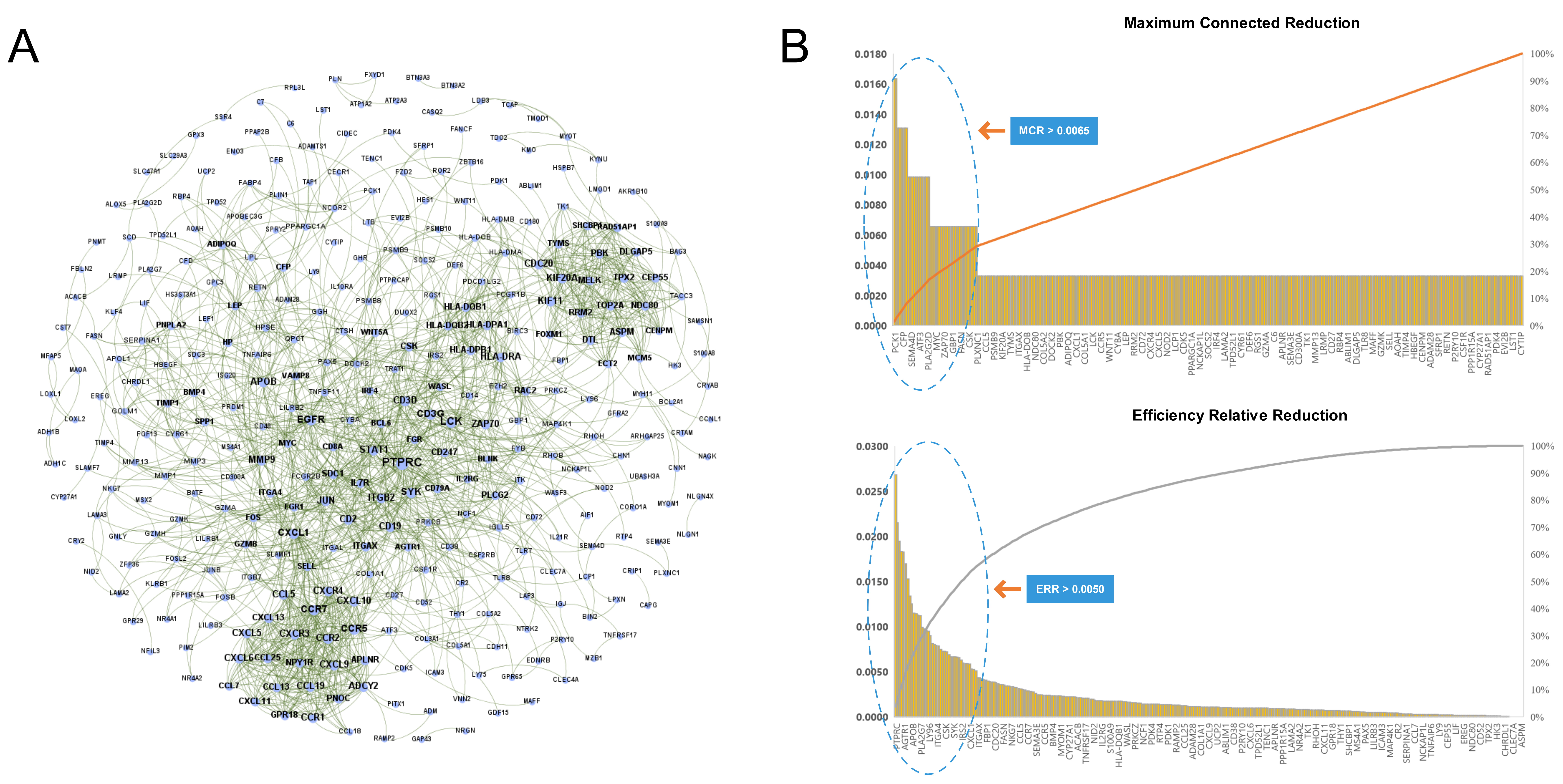
Identification key RA genes by PPI network. (A) Protein-protein interaction network of RA. (B) Screening of key RA genes based on the MCR (top) and ERR (bottom) algorithms.
According to the calculation results, genes that met the threshold conditions
(MCR
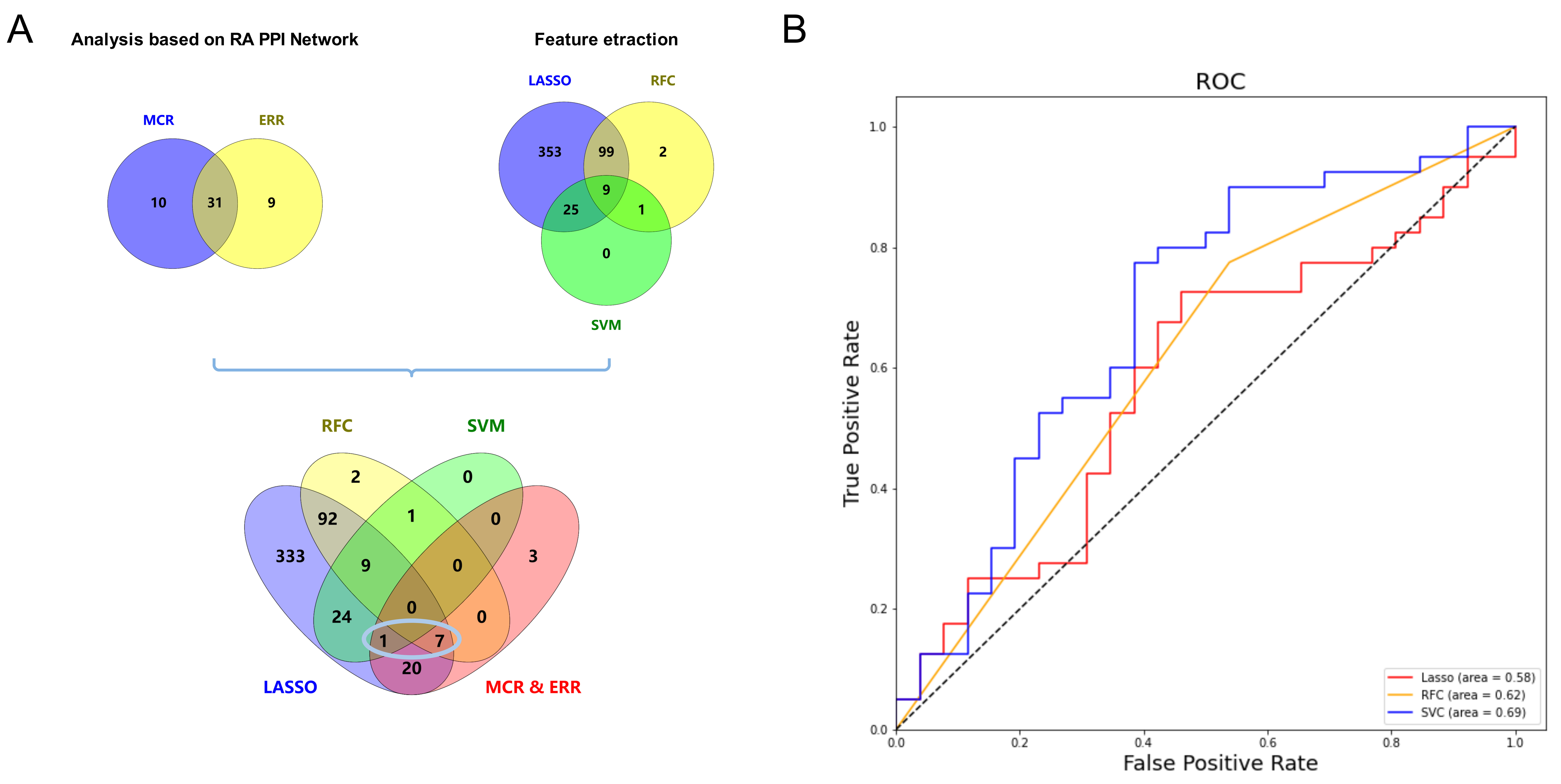
Identification key RA genes by machine learning. (A) Results of key RA gene screening based on the lasso regression model, random forest classifier (RFC), and support vector classifier (SVC) feature extraction, and RA core gene discovery based on PPI network analysis and machine learning feature extraction. (B) Receiver operating characteristic (ROC) curves of disease prediction classification of the eight core genes.
Using the RFECV tool to extract features and combining with each of the three machine learning algorithms, 486 genes related to classification were screened out by the lasso regression model, 111 were obtained by the RF classification model, and 35 were extracted by the linear SVM model. After taking the intersection of the genes extracted by the three classification models, a total of 134 genes with classification characteristics were obtained (Fig. 5A). After further intersecting with the core gene set obtained by PPI network analysis, eight common genes were found: MYH11, CFP, LY96, IGJ, LPL, CD48, RAC2, and CSK (Table 1).
Gene names | Protein names | Uniprot ID |
CD48 | CD48 antigen | P09326 |
CFP | Properdin | P27918 |
CSK | Tyrosine-protein kinase CSK | P41240 |
IGJ | Immunoglobulin J chain | P01591 |
LPL | Lipoprotein lipase | P06858 |
LY96 | Lymphocyte antigen 96 | Q9Y6Y9 |
MYH11 | Myosin-11 | P35749 |
RAC2 | Ras-related C3 botulinum toxin substrate 2 | P15153 |
Further, we took these eight genes as the classification feature indicators of data samples, used the three machine learning classification models (Lasso, RF, and SVM) in the sklearn package again for 50% cross-validation, and calculated and plotted AUC values and ROC curves of the three classifier models, as shown in Fig. 5B. The average classification prediction AUC value of these eight genes in the three classifiers was 0.63, and the highest value was 0.69. Even though the sample size of RA data included in this analysis was relatively small, the AUC values of the three classification models were all greater than 0.6, indicating that these eight core genes do have certain classification potential for the existing RA samples, suggesting that these genes have a certain correlation with RA.
The results of immune cell infiltration analysis are shown in Fig. 6. Among the
22 types of immune cells in RA, correlations were found between neutrophils and
activated dendritic cells (
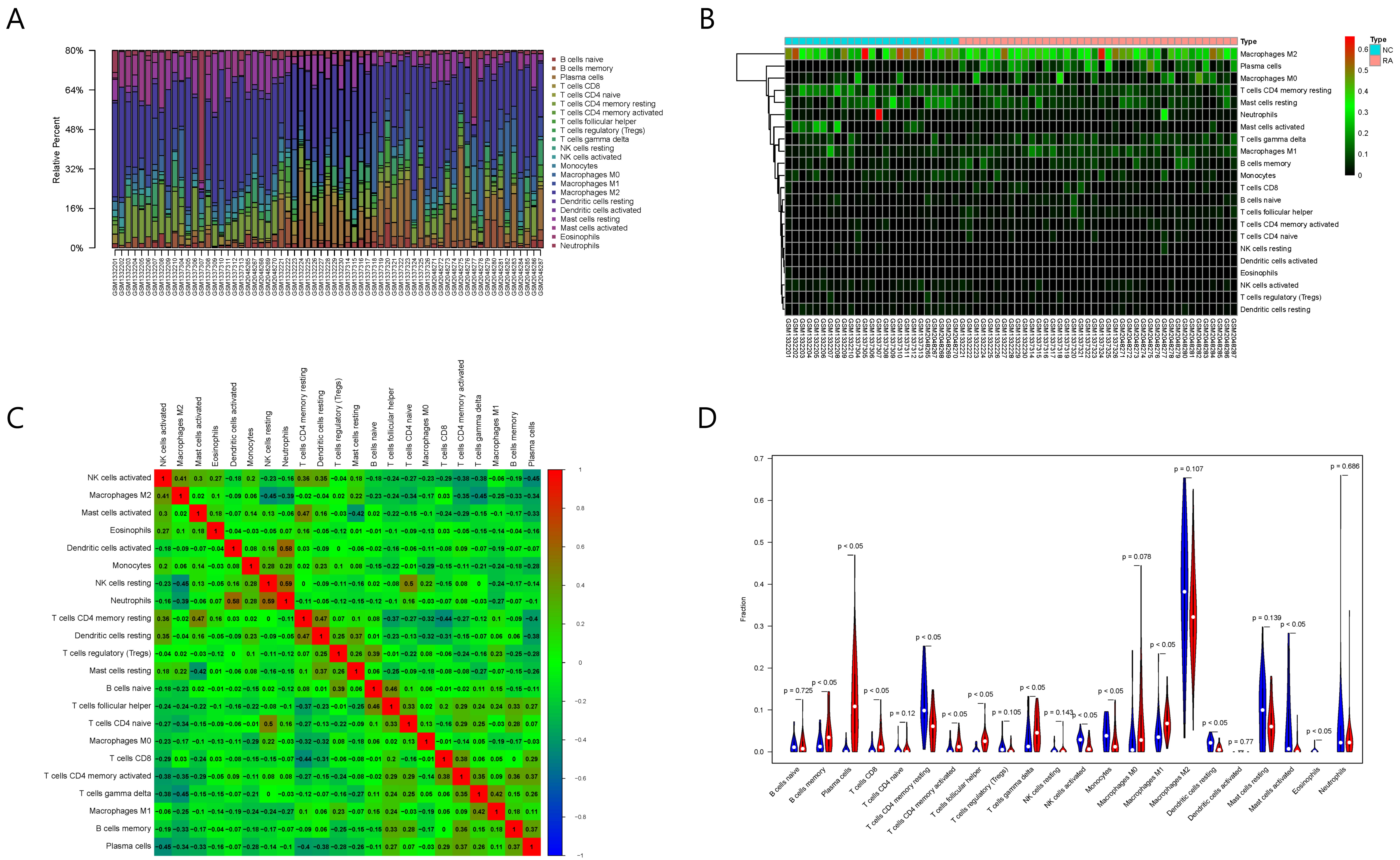
Results of immune cell infiltration evaluation and gene correlation analysis. (A) Infiltration and abundance of 22 types of immune cells in all RA samples. (B) Heat map of the infiltration and abundance of 22 types of immune cells in RA. (C) Correlation heat map of 22 types of immune cells in RA. (D) Differences in the infiltration of 22 types of immune cells between RA and normal samples.
Among the 22 types of immune cells, the infiltration of memory B cells, plasma
cells, CD8+ T cells, CD4+ resting memory T cells, CD4+ activated memory T cells,
follicular helper T cells, gamma delta T cells, activated NK cells, M1
macrophages, resting dendritic cells, activated mast cells, and eosinophils was
significantly different between the normal group and RA group (p
Spearman correlation analysis showed that six out of the eight key RA genes were
significantly correlated with the infiltration and abundance of immune cells
(p
22 types of immune cells | CD48 | CFP | CSK | IGJ | LPL | LY96 | MYH11 | RAC2 |
B cells naive | –0.02 | –0.03 | –0.31 | –0.22 | 0.07 | –0.22 | 0.21 | –0.12 |
B cells memory * | 0.42 | 0.02 | 0.26 | 0.51 | 0.04 | 0.17 | –0.22 | 0.36 |
Plasma cells * | 0.74 | 0.47 | 0.69 | 0.91 | –0.26 | 0.71 | –0.53 | 0.78 |
T cells CD8 * | 0.42 | 0.38 | 0.41 | 0.40 | –0.30 | 0.34 | –0.25 | 0.37 |
T cells CD4 naive | 0.50 | 0.27 | 0.36 | 0.37 | –0.10 | 0.29 | –0.33 | 0.49 |
T cells CD4 memory resting * | –0.35 | –0.34 | –0.45 | –0.39 | 0.34 | –0.45 | 0.39 | –0.54 |
T cells CD4 memory activated * | 0.64 | 0.23 | 0.41 | 0.62 | –0.22 | 0.53 | –0.54 | 0.56 |
T cells follicular helper * | 0.47 | 0.29 | 0.34 | 0.42 | –0.35 | 0.22 | –0.29 | 0.59 |
T cells regulatory (Tregs) | –0.34 | –0.12 | –0.35 | –0.39 | 0.25 | –0.35 | 0.42 | –0.42 |
T cells gamma delta * | 0.59 | 0.24 | 0.16 | 0.38 | –0.03 | 0.31 | –0.17 | 0.47 |
NK cells resting | –0.11 | 0.01 | –0.13 | –0.12 | 0.07 | –0.10 | –0.06 | 0.02 |
NK cells activated * | –0.57 | –0.44 | –0.38 | –0.53 | 0.18 | –0.41 | 0.41 | –0.57 |
Monocytes * | –0.15 | –0.07 | –0.13 | –0.29 | 0.01 | –0.13 | –0.14 | –0.18 |
Macrophages M0 | –0.05 | 0.09 | 0.11 | 0.03 | 0.03 | 0.15 | 0.00 | 0.20 |
Macrophages M1 * | 0.46 | 0.19 | 0.06 | 0.39 | –0.17 | 0.15 | –0.06 | 0.26 |
Macrophages M2 | –0.47 | –0.21 | –0.08 | –0.35 | –0.03 | –0.21 | 0.22 | –0.47 |
Dendritic cells resting * | –0.25 | –0.35 | –0.32 | –0.36 | 0.37 | –0.47 | 0.29 | –0.44 |
Dendritic cells activated * | –0.03 | 0.16 | 0.13 | 0.01 | 0.05 | 0.13 | –0.23 | 0.09 |
Mast cells resting | –0.25 | –0.16 | –0.13 | –0.19 | 0.29 | –0.12 | 0.28 | –0.38 |
Mast cells activated | –0.32 | –0.25 | –0.38 | –0.46 | 0.11 | –0.46 | 0.18 | –0.30 |
Eosinophils * | –0.22 | –0.24 | –0.35 | –0.17 | 0.20 | –0.15 | 0.22 | –0.27 |
Neutrophils | 0.14 | 0.11 | 0.23 | 0.19 | –0.13 | 0.19 | –0.33 | 0.14 |
With * indicating the significant differences (p |
Moreover, the five types of immune cells correlated with RAC2 showed significant differences between the normal and RA samples. Therefore, we selected RAC2 protein for the subsequent screening and verification of TCM small molecules.
A PDB file (PDB ID: 2W2V) [12] of RAC2 protein was downloaded from the PDB database. All four chains of RAC2 protein had the known ligand guanosine-5’-triphosphate (GTP). Therefore, we screened for TCM small molecules with high similarity to GTP in terms of the chemical structure.
We collected one SMILES string of GTP (PubChem CID: 135398633) from the PubChem. The TCM small molecules and the SMILES strings of GTP were imported into DRAGON software to calculate the 1024-dimensional extended-connectivity molecular fingerprints of all small molecules.
A total of 31 TCM small molecules with similar structures to GTP were screened
out according to a threshold of Z
Since RAC2 protein has four chains (A, B, C, and D) and each chain has a corresponding ligand-binding site, we performed batch molecular docking on four sites in the four chains of RAC2. Among them, the A-chain binding site coordinate is (5.711, –4.855, 41.735), the B-chain binding site coordinate is (–10.673, –5.506, 23.999), and the C-chain binding site coordinate is (10.654, 9.806, –11.671), the D-chain binding site coordinate is (14.897, 58.007, 29.835).
According to the scores of docking the 31 small molecules with the molecules on the different chains of RAC2, the top 14 TCM small molecules with average affinity values less than –7.0 kcal/mol and with relatively good scores were as follows: jatrophon, sanguinarine, miroestrol, withaferin A, pristimerin, chelidonine, picroside I, sesamin, gnidimacrin, cephalotaxine, honokiol, oleuropein, atractylenolide I, and dracorhodin.
Finally, based on the molecular docking results and the reported literature, we selected four TCM small molecular compounds for subsequent experimental verification: sanguinarine, chelidonine, sesamin, and honokiol. It should be noted that since Jatrophon was out of stock, we chosen sanguinarine with the second-ranked molecular docking score. The other three components were all reported small molecules of TCM with potential to treat RA (Table 3, Ref. [13, 14, 15, 16, 17, 18, 19, 20, 21, 22, 23, 24, 25, 26, 27, 28, 29, 30, 31, 32, 33, 34]).
Name | Formula | PubChem CID | Mean affinity (kcal/mol) | Bioactivity |
Jatrophon | C |
5281373 | –8.4 | Triple-negative breast cancer [13] |
Sanguinarine | C |
5154 | –8.1 | Epithelial ovarian cancer [14] |
Miroestrol | C |
165001 | –7.7 | Improving the antioxidation state in |
Withaferin A | C |
265237 | –7.7 | Antidiabetic properties [16]; anti-cancer properties [17] |
Pristimerin | C |
159516 | –7.7 | Breast cancer [18]; colon cancer [19] |
Chelidonine | C |
197810 | –7.6 | Lipopolysaccharide-induced production of inflammatory mediators [20]; non-small cell lung cancer [21] |
Picroside I | C |
6440892 | –7.6 | Hepatic fibrosis [22] |
Sesamin | C |
72307 | –7.4 | Rheumatoid arthritis [23, 24] |
Gnidimacrin | C |
3085204 | –7.3 | HIV [25] |
Cephalotaxine | C |
65305 | –7.2 | Anti-varicella-zoster virus [26]; inhibiting Zika infection [27] |
Honokiol | C |
72303 | –7.2 | Rheumatoid arthritis [28] |
Oleuropein | C |
5281544 | –7.1 | Metabolic syndrome [29]; antitumor activity [30] |
Atractylenolide I | C |
5321018 | –7.1 | Colon adenocarcinoma [31]; ovarian cancer [32]; anti-melanoma [33] |
Dracorhodin | C |
69509 | –7.1 | Esophageal squamous cell carcinoma [34] |
The 2D binding conformations of these 4 small molecules and RAC2 were displayed using LigPlot (version 2.2, https://www.ebi.ac.uk/thornton-srv/software/LIGPLOT/), as shown in Fig. 7.
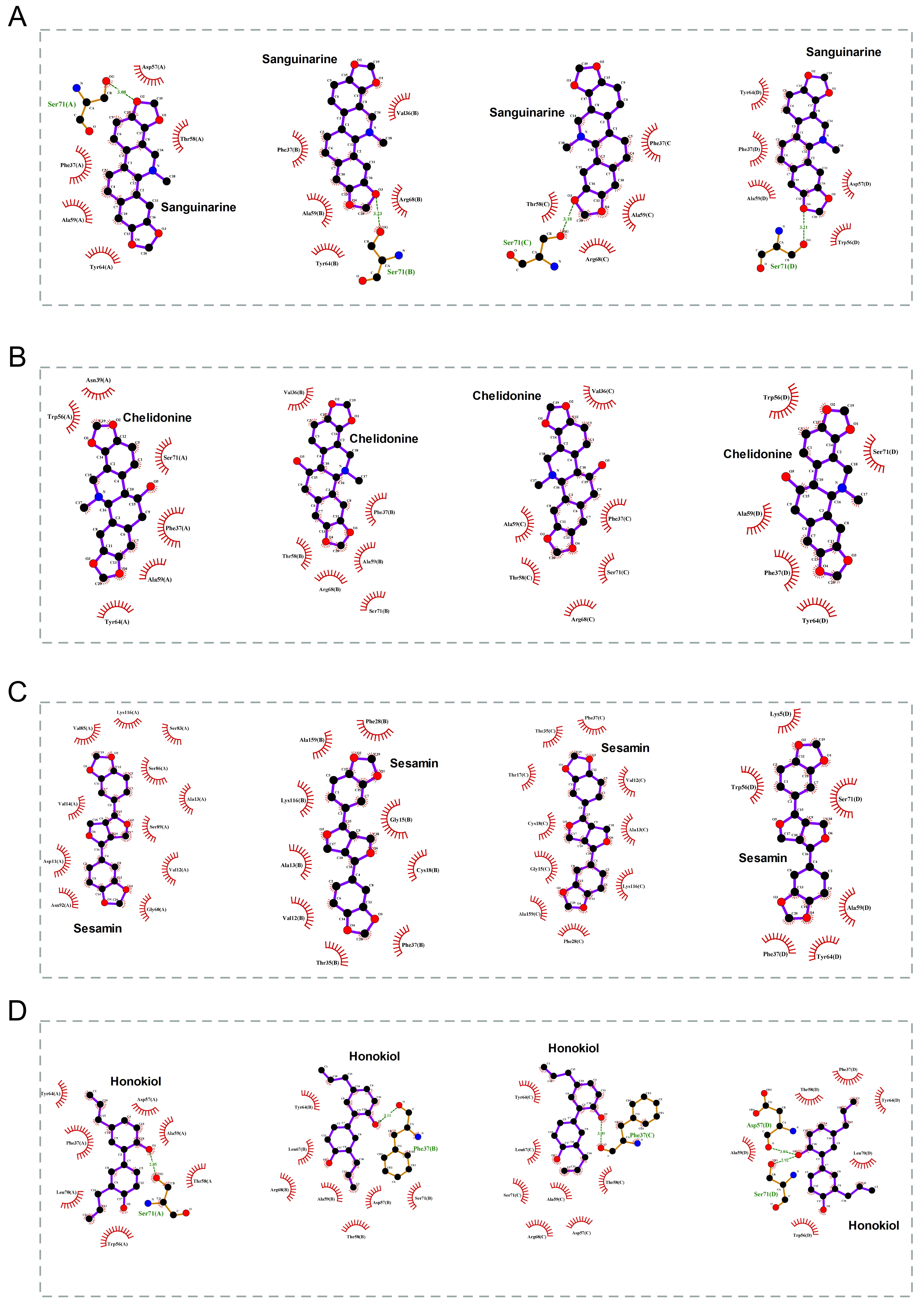
The molecular docking results of these 4 small molecules and RAC2. The 2D conformation diagram of (A) Sanguinarine, (B) Chelidonine, (C) Sesamin, and (D) Honokiol in the 4 chains of RAC2, from left to right shown the ligand binding poses in chain A, chain B, chain C, and chain D.
The in vitro CCK-8 assays showed that none of the screened out TCM
compounds had a significant effect on the proliferation of normal rat synovial
cells; however, the proliferation of RA rat synovial cells to sanguinarine,
chelidonine, sesamin, and honokiol was significantly decreased, with IC
Compared with the normal control group, the levels of TNF-
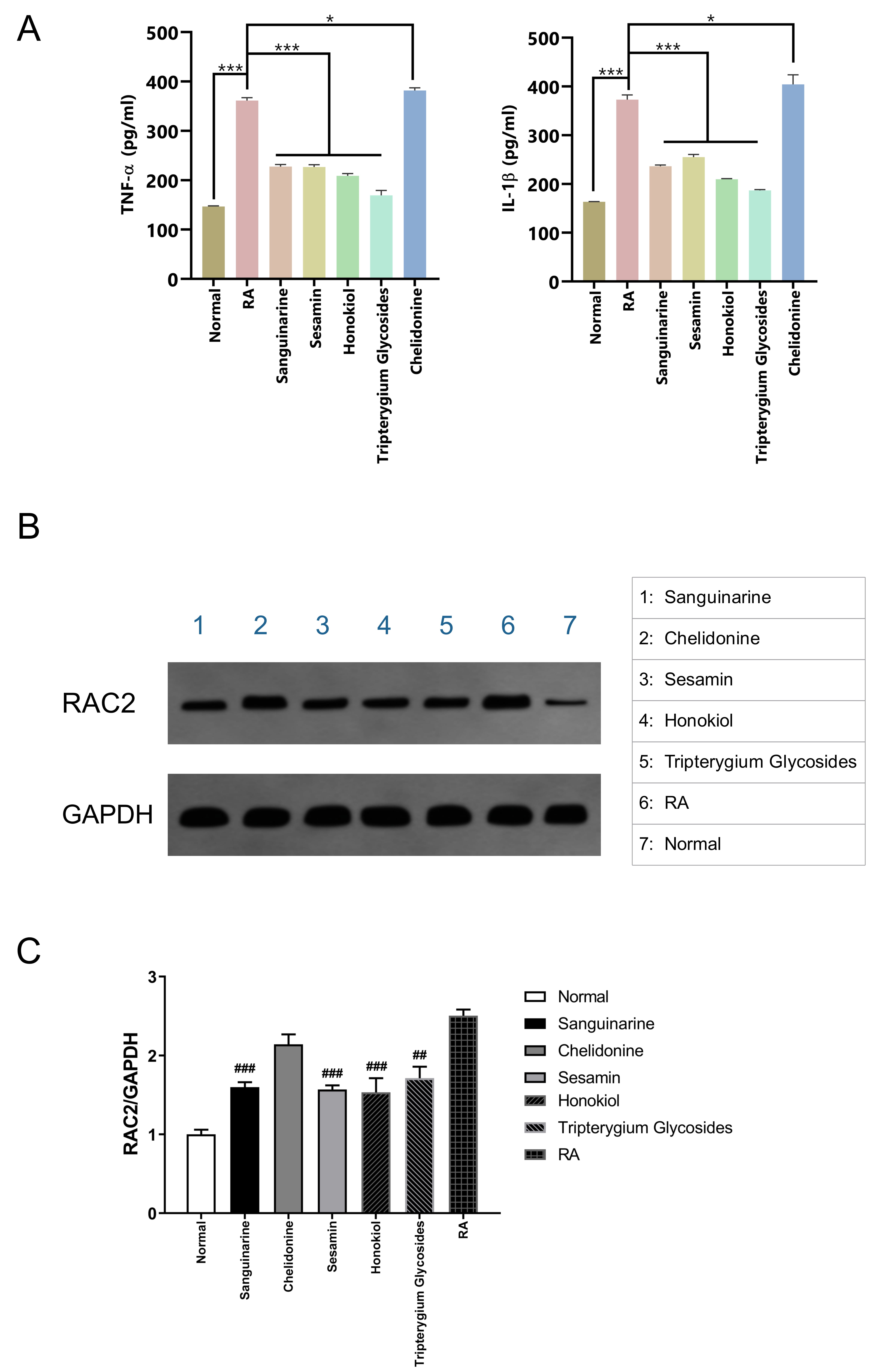
Verification with RA rat model synovial cells. (A) Effects of
different small-molecule compounds of TCM on the secretion levels of
TNF-
Western blotting confirmed that the expression of RAC2 protein in RA rat synovial cells was significantly higher than that in normal synovial cells; however, treatment with sanguinarine, sesamin, and honokiol could significantly suppress the expression of RAC2 protein (Fig. 8B), and the significant differences in the WB gray values of the RA group and the three small molecules were shown in Fig. 8C.
RA is a chronic inflammatory autoimmune disease characterized by infiltration of immune cells into the affected synovium, release of inflammatory cytokines and degradation of mediators, and subsequent joint damage [35, 36]. With the rapid development of molecular biology and analysis tools, bioinformatics has provided powerful strategic markers for the screening of molecules [37, 38], and the CIBERSORT tool has promoted disease analysis with patterns of immune cell infiltration [39].
Given the limited sequencing data available on RA patients in existing public
databases, we performed DEG analysis by combining RA chip data from three
datasets. Further, to ensure the reliability of the results, batch correction was
first performed. Nearly 66% of the DEGs in RA were up-regulated and 34% were
down-regulated. Among the key genes correlated with immune cell infiltration,
CD48 (log
Many reports have shown that nodes with relatively large degree values in some networks often do not truly reflect their actual roles in PPI networks. Moreover, if the efficiency of the entire disease network will be substantially reduced after a node is removed from the network, this can indicate that the node plays a more significant role in the network. If drugs can interfere with these targets, the protein regulation relationship within the disease would be destroyed, offering an effective treatment target. Therefore, in this study, we used two methods for analysis of network node degree values to identify important nodes in the PPI networks, which differ from the methods conventionally used for such analyses. The MCR and ERR algorithms adopted in this study were used to measure the actual efficiency of nodes in the networks to evaluate their importance.
Based on the combined data of the RA gene expression profiles from 66 samples, we used feature selection and adopted three machine-learning classification models (Lasso, RF, and SVM) to identify the gene set with a significant classification effect on RA samples. Finally, through these two analysis methods, we could ensure that the key genes obtained are not only the important nodes in the disease protein regulatory network but also characteristic genes in RA sample classification.
According to the six key genes we finally obtained with this approach, CD48 is a member of the signaling lymphocyte activation molecule family, with a primary role in the adhesion and activation of immune cells [40]. Other studies revealed that CD48 is a key immunomodulatory molecule that affects the prognosis of glioma [41], and it was also suggested as a potential new target for asthma treatment [42]. Clinical research has shown that another one of our key genes in RA, IGJ, is a marker of antibody-secreting plasma blasts, and 25% of the treated subjects had an increased mRNA expression level of IGJ at baseline [43]. Polymorphism of LY96 was correlated with susceptibility to inflammatory bowel disease in the Danish population [44], and also emerged as a significant predictor of a poorly induced treatment response and the worst survival rate. A single nucleotide repeat (C8) in the coding sequence of MYH11 was proposed as a mutation target of cancer showing microsatellite instability [45]. CSK plays an important role in regulating intestinal epithelial barrier function and preventing colitis [46], and can also enhance the innate immune response to DNA viruses by phosphorylating MITA [47].
At present, there have been some reports on the identification of potential targets of RA based on bioinformatics methods [48, 49]. In the work of [41], it was identified STAT1, RAC2 and KYNU as hub proteins. Consistent with our findings, RAC2 was also found to be a potential therapeutic target in RA.
RAC2 activation is necessary for macrophages to polarize to the
fibrosis phenotype and is associated with the progression of pulmonary fibrosis
[50]. RAC2 can regulate the calcification in atherosclerosis by
regulating the production of IL-1
In the present study, the enrichment analysis showed that the GO functions of RAC2 mainly involved processes related to T cell function, such as T cell activation, regulation of immune effector process, regulation of T cell activation, and T cell proliferation. In line with this finding, previous studies showed that RAC2 plays a unique role in the production of common lymphoid progenitors, plays diverse and indispensable roles in the later stage of T cell development by regulating survival and proliferation signals, and plays a very important role in mediating transcription and cytoskeleton changes during T cell activation [57].
In addition, our KEGG pathway enrichment analysis showed that RAC2 mainly participates in regulation of the chemokine signaling pathway, B cell receptor signaling pathway, viral myocarditis, leukocyte transendothelial migration, NK cell-mediated cytotoxicity, and other signaling pathways. Among these pathways, previous studies have confirmed that the chemokine signaling pathway is involved in the expression of CCL2 in patients with RA [58], and that the B cells and the B cell signaling pathway are associated with the pathogenesis and therapeutic targets of RA [59, 60]. Collectively, these findings indicate that RAC2 may be a potential new therapeutic target for RA.
We therefore screened for TCM small molecules as RAC2 targets focusing on GTP small molecules in the original ligands based on molecular fingerprint similarity, followed by molecular docking based on the reference positions on the four different chains of the original ligands to screen candidates with high treatment potential. Based on this analysis, four small molecules were screened out with high affinity: sanguinarine, sesamin, and honokiol, which were verified to have anti-inflammatory effects using in vitro assays with synovial cells of a collagen-induced RA rat model.
To our knowledge, this is the first study that a multidisciplinary approach has been integrated to discover potential targets of RA and screen small molecules of TCM for their treatment, and we have established a feasible anti-RA drug discovery process. However, due to the complexity of RA and the limitations of experimental conditions, we did not conduct a comprehensive verification of the molecular mechanisms and functions of all eight potential RA targets, but only selected RAC2 for in vitro experimental verification. Therefore, the precise functions of targets should be further elucidated in the future. Although this study found four small molecules of TCM targeting RAC2 through in vitro experiments, we did not perform the corresponding in vivo verification. Further studies are warranted to the actual therapeutic effect of these small molecules in RA by in vivo experiments.
In summary, we attempted to identify new potential diagnostic markers of RA with the combination of PPI network analysis and machine learning, and further used computer-aided drug design methods such as chemo-informatics and molecular docking to screen out therapeutic TCM small molecules with therapeutic potential for RA. The results of this study can provide strong support for further appropriate development of TCMs in the treatment of RA.
The data used to support the findings of this study are included within the article and Support material.
All authors read and approved the final manuscript. JS conducted the analyses and wrote the manuscript. BL carried out experimental validation. YY contributed to revising and proof-reading the manuscript. LZ and JW provided the concept and designed the study. JS and BL equally contributed to this work and should be considered co-first authors. LZ and JW contributed equally to this work and should be considered co-corresponding authors.
The animal study was reviewed and approved by Shanghai Model Organisms Center (IACUC No. 2021-0034).
Not applicable.
This work was supported by the Three-year action plan for Shanghai (ZY (2021-2023)-0211); the Shanghai Collaborative Innovation Center for Chronic Disease Prevention and Health Services (2021 Science and Technology 02-37); the General Project of Chinese Medicine Scientific Research Project Plan of Shanghai Municipal Health Commission (2020JP002); the Shanghai Natural Science Fund (19ZR1452000).
The authors declare no conflict of interest.
Publisher’s Note: IMR Press stays neutral with regard to jurisdictional claims in published maps and institutional affiliations.