†These authors contributed equally.
Academic Editor: Zhonghua Sun
Background: Recent studies have shown that epicardial adipose tissue
(EAT) is an independent atrial fibrillation (AF) prognostic marker and has
influence on the myocardial function. In computed tomography (CT), EAT volume
(EATv) and density (EATd) are parameters that are often used to quantify EAT.
While increased EATv has been found to correlate with the prevalence and the
recurrence of AF after ablation therapy, higher EATd correlates with inflammation
due to arrest of lipid maturation and with high risk of plaque presence and
plaque progression. Automation of the quantification task diminishes the
variability in readings introduced by different observers in manual
quantification and results in high reproducibility of studies and less
time-consuming analysis. Our objective is to develop a fully automated
quantification of EATv and EATd using a deep learning (DL) framework.
Methods: We proposed a framework that consists of image classification
and segmentation DL models and performs the task of selecting images with EAT
from all the CT images acquired for a patient, and the task of segmenting the EAT
from the output images of the preceding task. EATv and EATd are estimated using
the segmentation masks to define the region of interest. For our experiments, a
300-patient dataset was divided into two subsets, each consisting of 150
patients: Dataset 1 (41,979 CT slices) for training the DL models, and Dataset 2
(36,428 CT slices) for evaluating the quantification of EATv and EATd.
Results: The classification model achieved accuracies of 98% for
precision, recall and F
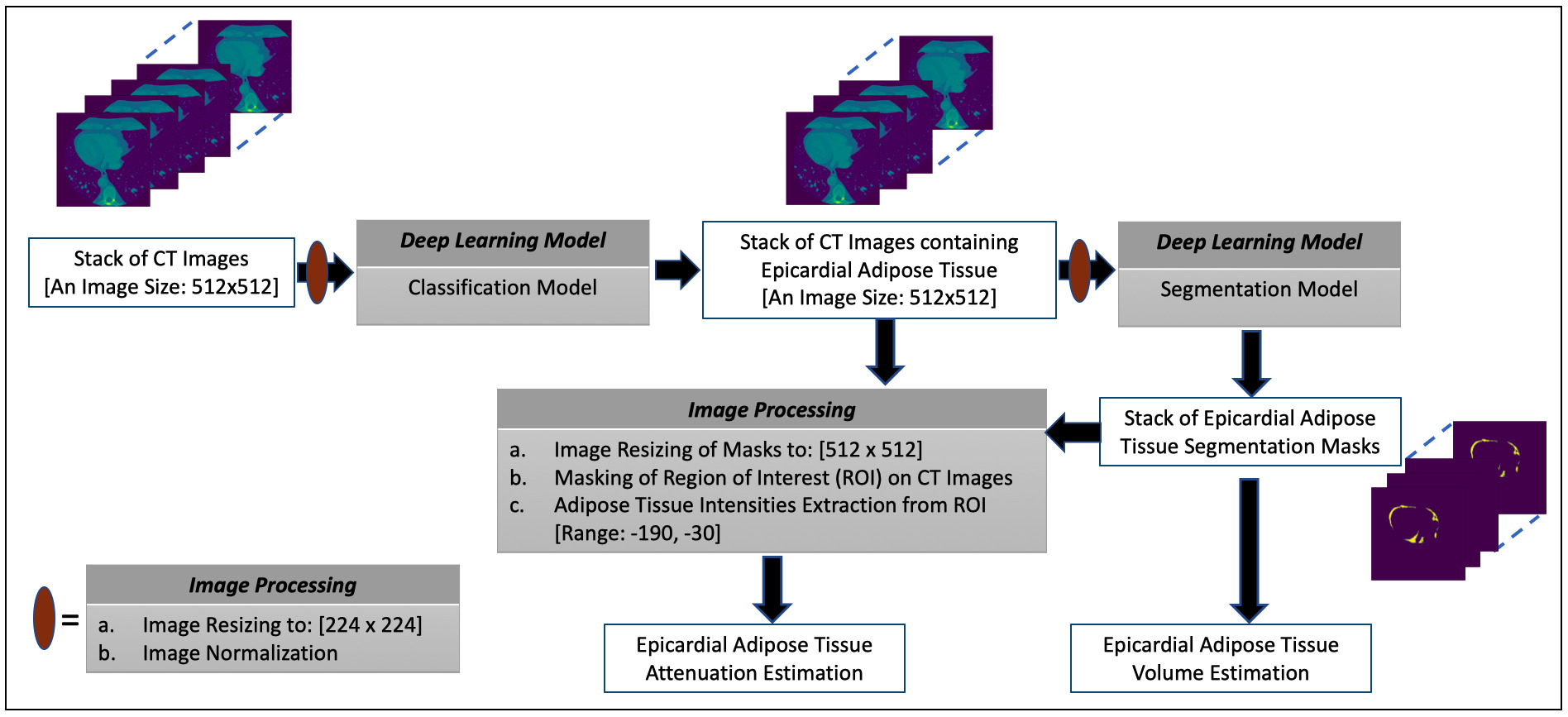