Academic Editor: Jerome L. Fleg
Background: Heart failure remains a considerable burden to healthcare in Asia. Early intervention, mainly using echocardiography, to assess cardiac function is crucial. However, due to limited resources and time, the procedure has become more challenging during the COVID-19 pandemic. On the other hand, studies have shown that artificial intelligence (AI) is highly potential in complementing the work of clinicians to diagnose heart failure accurately and rapidly. Methods: We systematically searched Europe PMC, ProQuest, Science Direct, PubMed, and IEEE following the Preferred Reporting Items for Systematic Reviews and Meta-Analyses (PRISMA) guidelines and our inclusion and exclusion criteria. The 14 selected works of literature were then assessed for their quality and risk of bias using the QUADAS-2 (Quality Assessment of Diagnostic Accuracy Studies). Results: A total of 2105 studies were retrieved, and 14 were included in the analysis. Five studies posed risks of bias. Nearly all studies included datasets in the form of 3D (three dimensional) or 2D (two dimensional) images, along with apical four-chamber (A4C) and apical two-chamber (A2C) being the most common echocardiography views used. The machine learning algorithm for each study differs, with the convolutional neural network as the most common method used. The accuracy varies from 57% to 99.3%. Conclusions: To conclude, current evidence suggests that the application of AI leads to a better and faster diagnosis of left heart failure through echocardiography. However, the presence of clinicians is still irreplaceable during diagnostic processes and overall clinical care; thus, AI only serves as complementary assistance for clinicians.
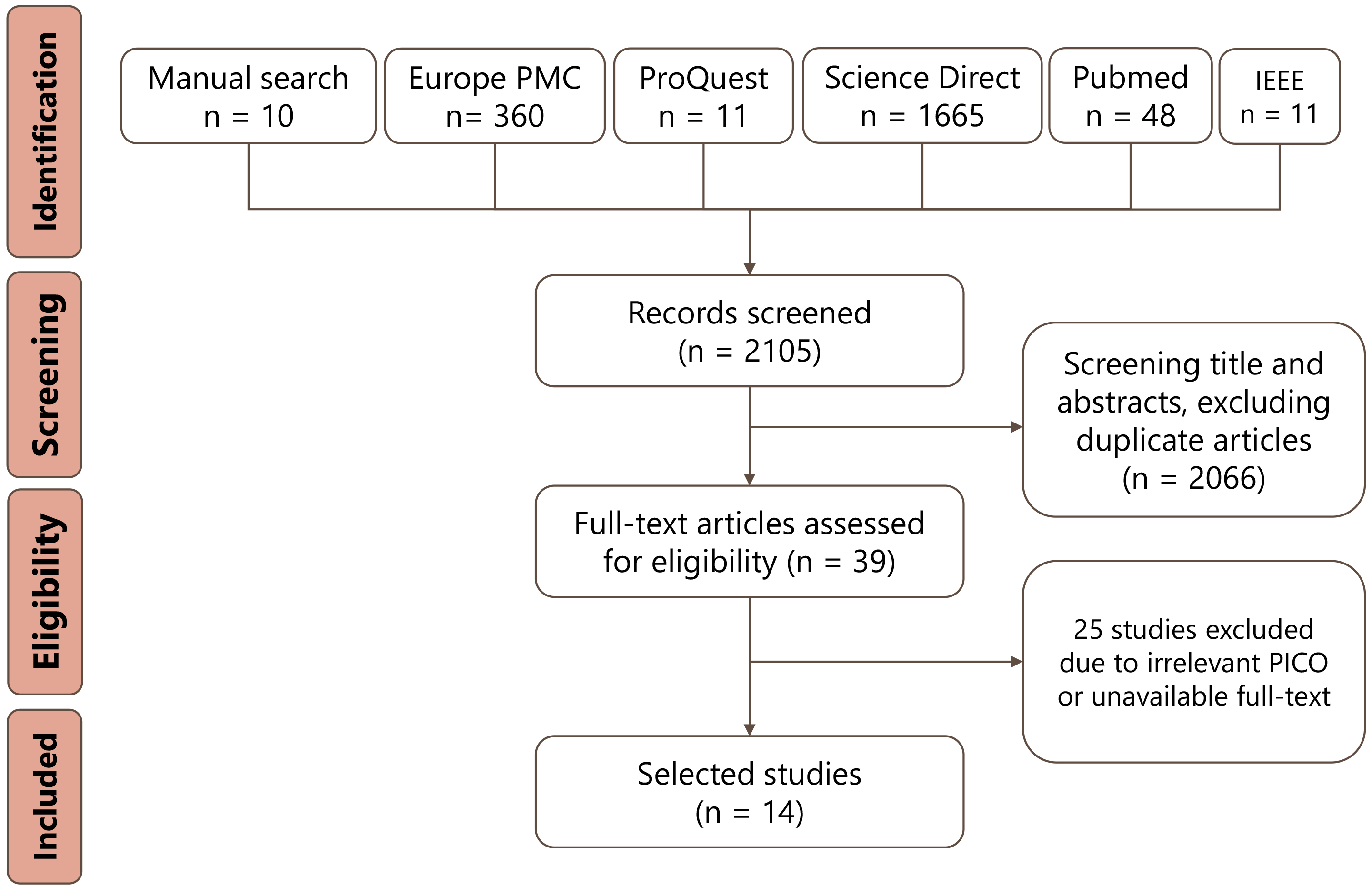