- Academic Editor
†These authors contributed equally.
Background: Cerebral stroke is a leading cause of death and disability worldwide. Ligusticum Chuanxiong Hort. (LCH), a well-known Chinese herb, is widely used for the treatment of cerebral stroke. This study aimed to investigate the underlying mechanisms of LCH in cerebral stroke and develop a diagnostic model. Methods: We employed network pharmacology analyses to identify the active compounds, targets, and underlying mechanisms of LCH for treating cerebral stroke. Molecular docking was performed to visualize the binding site between the core active compounds and hub targets. Furthermore, a diagnostic model for cerebral stroke was constructed based on transcriptomic analysis. Results: Our findings revealed that LCH contains multiple active ingredients, including oleic acid and caffeic acid. Protein-protein interaction network analysis identified IL1B, CCL2, MAPK3, PTGS2, JUN, MMP9, TLR4, HIF1A, PPARA, FOS, PTEN, NFE2L2, TLR2, TIMP1, and SOD2 as the top 15 hub genes. Kyoto Encyclopedia of Genes and Genomes pathway analysis highlighted the enrichment of TNF and IL-17 signaling pathways. Molecular docking analysis demonstrated binding sites between oleic acid, caffeic acid, and MMP9, PPARP, PTEN, and TIMP1. The diagnostic model indicated that FOS, MMP9, PPARA, PTEN, TIMP1, and TLR2 serve as blood biomarkers for cerebral stroke. Conclusions: This study demonstrates that LCH alleviates the symptoms following cerebral stroke through interactions with the TNF and IL-17 signaling pathways. The findings contribute to a better understanding of the therapeutic mechanisms of LCH and offer insights into the development of a diagnostic model for cerebral stroke.
Cerebral stroke, also known as a stroke or cerebrovascular accident, is a leading cause of long-term disability and mortality in adults worldwide [1]. It occurs when the blood supply to a specific region of the brain is disrupted, manifesting as ischemic or hemorrhagic stroke [2]. Cerebral ischemic stroke, accounting for 75% of cases, has been demonstrated to be a manifestation of a hematological disorder [3]. It arises from the obstruction of cerebral blood vessels by plaque formation or an embolus formed elsewhere in the body. Hemorrhagic stroke accounts for 25% of cases and is caused by the rupture of a cerebral blood vessel [4]. The majority of stroke patients are over 40 years old, with a higher incidence in males than females [5]. Cerebral stroke leads to over 5–6 million deaths worldwide every year [6]. Therefore, it is important to further explore preventive and therapeutic approaches for cerebral stroke.
Traditional Chinese medicine (TCM) has gained recognition as an effective approach for stroke prevention and treatment after years of clinical application. Ligusticum Chuanxiong Hort. (LCH) is one TCM commonly used to treat headaches, post-traumatic swelling pain, rheumatic arthralgia, etc. [7]. It is known to promote blood circulation and alleviate the symptoms of stroke caused by blood stasis [8]. Previous studies have reported the therapeutic effects of LCH on cerebral ischemia and hemorrhage [9, 10]. However, the precise mechanisms through which LCH alleviates the symptoms of cerebral stroke require further investigation.
Network pharmacology can systematically evaluate the interactions between diseases and drugs. It unravels the specific mechanisms by which drugs act on their target genes [11]. Transcriptomics, on the other hand, enables a comprehensive analysis of the entire genome by shedding light on the biological processes involved in stroke pathogenesis and medical intervention [12]. These strategies provide novel insights and facilitate the accurate application of TCM. In this study, we aimed to explore the potential diagnostic targets and underlying mechanisms through which LCH can be used to treat cerebral stroke. These findings could provide new possibilities and directions for the treatment of cerebral stroke.
Cerebral stroke-related genes were retrieved from the GeneCards (https://www.genecards.org), DisGeNET (https://www.disgenet.org/), and DigSee (http://210.107.182.61/geneSearch/) databases. Duplicate entries were removed. Active compounds of LCH were obtained from the HIT (http://hit2.badd-cao.net), TCMSP (http://tcmspw.com/tcmsp.php), and TCMID (https://bidd.group/TCMID/) databases. Repetitive compounds and those lacking Pubchem IDs were excluded. According to Pubchem ID, targets of the active compounds in LCH were searched in the HIT database.
Cerebral stroke blood transcriptome datasets GSE16561 and GSE22255 for cerebral
stroke were retrieved from the Gene Expression Omnibus (GEO) database (https://www.ncbi.nlm.nih.gov/geo).
Batch effects between datasets obtained from different platforms were removed
using the surrogate variable analysis (SVA) algorithm. Screen for Differentially
Expressed Genes (DEGs) between the disease and control groups were identified
using the limma program package (https://bioconductor.org/packages/release/bioc/html/limma.html) [13] with p
The cerebral stroke-related genes obtained, the active compound-targeted genes of LCH, and the DEGs in cerebral stroke were intersected using a Venn diagram (version 1.7.3) in R 4.0 software (https://www.r-project.org/).
Interactions between the 55 intersecting genes and their reciprocal proteins
were predicted using the STRING database
(https://string-db.org/, version 11.0). The
screening conditions included a score cutoff
Gene Ontology (GO) and Kyoto Encyclopedia of Genes and Genomes (KEGG) enrichment
analyses of genes in the PPI network were performed using clusterProfiler
(http://www.bioconductor.org/packages/release/bioc/html/clusterProfiler.html,
version 4.4.2), where p
A diagnostic marker model for cerebral stroke was constructed using the machine
learning algorithm lasso regression applied to the screened hub genes. To avoid
overfitting the model and evaluate the stability of the model prediction, 60% of
the samples were randomly assigned to the training set and the remaining 40%
constituted the test set. Considering sex and age as relevant risk factors for
cerebral stroke, the predictive performance of the model was further assessed in
the training and test sets for sex and age groups. The difference in the mean
values of the basic blood test items were compared and items exhibiting
statistically significant differences were included in the lasso regression
analysis. The regularization parameter
Molecular docking was conducted to examine the interactions between marker genes in the model and the active ingredients of LCH. Proteins structures were downloaded from the RCSB protein database (http://www.rcsb.org), while structure files of the active ingredients in sdf format were obtained from PubChem. They were then converted to PDBQT format using Open Babel GUI (http://openbabel.org/docs/2.3.1/GUI/GUI.html). The protein was optimized by removing original ligands, dehydration, adding hydrogen bond and amino acids, and calculating charges using Pymol (https://pymol.org/2/) and Autodock 1.5.6 (http://mgltools.scripps.edu/). The molecular docking results were visualized using Autodock 1.5.6 and Pymol.
SH-SY5Y cells were purchased from Procell Life
Science&Technology Co., Ltd. (#CL0208; Wuhan, Hubei, China) and cultured in DMEM/F12
(Thermo Fisher Scientific, Waltham, MA, USA) with 10% FBS and
100 U/mL penicillium-streptomycin. The cells were authenticated at Procell Life
Science&Technology Co., Ltd. by short tandem repeat profiling, and no
contamination of mycoplasma had been identified. The cells were maintained in an
incubator at 37 °C with 5% CO
The SH-SY5Y cell suspension (100 µL) was seeded into 96-well plates at a concentration of 2000 cells/well. After treatment, the cells were incubated with 10 µL Cell Counting Kit-8 (CCK-8) solution (Beyotime, Shanghai, China) for 2 h. The absorbance at 450 nm was determined using a microplate reader (Wuxi Hiwell Diatek, Wuxi, Jiangsu, China).
A lactate dehydrogenase (LDH) assay kit (Nanjing Jiancheng Science and Technology Ltd., Nanjing, Jiangsu, China) was utilized to examine the production of LDH response to pesticide exposures using the manufacturer protocol. The test well was supplemented with 20 µL sample, 5 µL coenzyme I, and 25 µL substrate buffer. After a 15 min incubation at 37 °C, 25 µL of 2,4-Dinitrophenylhydrazine was added to each well and incubated for another 15 min at 37 °C. A 250 µL of NaOH solution (0.4 M) was then added to each well and incubated at room temperature for 5 min. Absorbance at 450 nm was measured using a microplate reader (Wuxi Hiwell Diatek, Wuxi, Jiangsu, China).
Total protein was extracted from cell lysates using radio-immunoprecipitation assay (RIPA) lysis buffer (Beyotime, Shanghai, China). A bicinchoninic acid (BCA) assay kit (Beyotime, Shanghai, China) was employed to measure protein concentration. Following protein quantification, 25 µg proteins were separated using a 10% separation sodium dodecyl sulfate polyacrylamide gel electrophoresis (SDS-PAGE) gel. Proteins were transferred to a polyvinylidene difluoride (PVDF) membrane and blocked with 5% milk dissolved in TBST for 1 h at room temperature. The membranes were probed overnight at 4 °C with the primary antibodies: anti-MMP9 (1:1000; #ab76003, Abcam, Cambridge, UK), anti-PPARA (1:1000; #ab3484, Abcam), anti-PTEN (1:1000; #ab137337, Abcam), anti-TIMP1 (1:1000; #ab61224, Abcam), and anti-GAPDH (1:10,000; #ab245355, Abcam). After incubation with horseradish peroxidase-conjugated secondary antibodies (1:2000; #ab6721, Abcam) for 1 h at room temperature, western blots were visualized with chemiluminescence. The protein levels were quantified through densitometry using ImageJ software (version 1.8.0, U. S. National Institutes of Health, Bethesda, MD, USA).
All data are presented as mean
A total of 2603 DEGs were identified. After removing batch effects in the
combined GSE16561 and GSE22255 datasets, the distribution trend of gene
expression levels was more consistent (Fig. 1A,B). The DEGs were visualized using
a heatmap and a volcano plot (Fig. 1C,D). Red represents upregulated genes, while
blue represents downregulated genes on the heatmap (Fig. 1C). The significance
cut-off criterion for differential expression was set at p
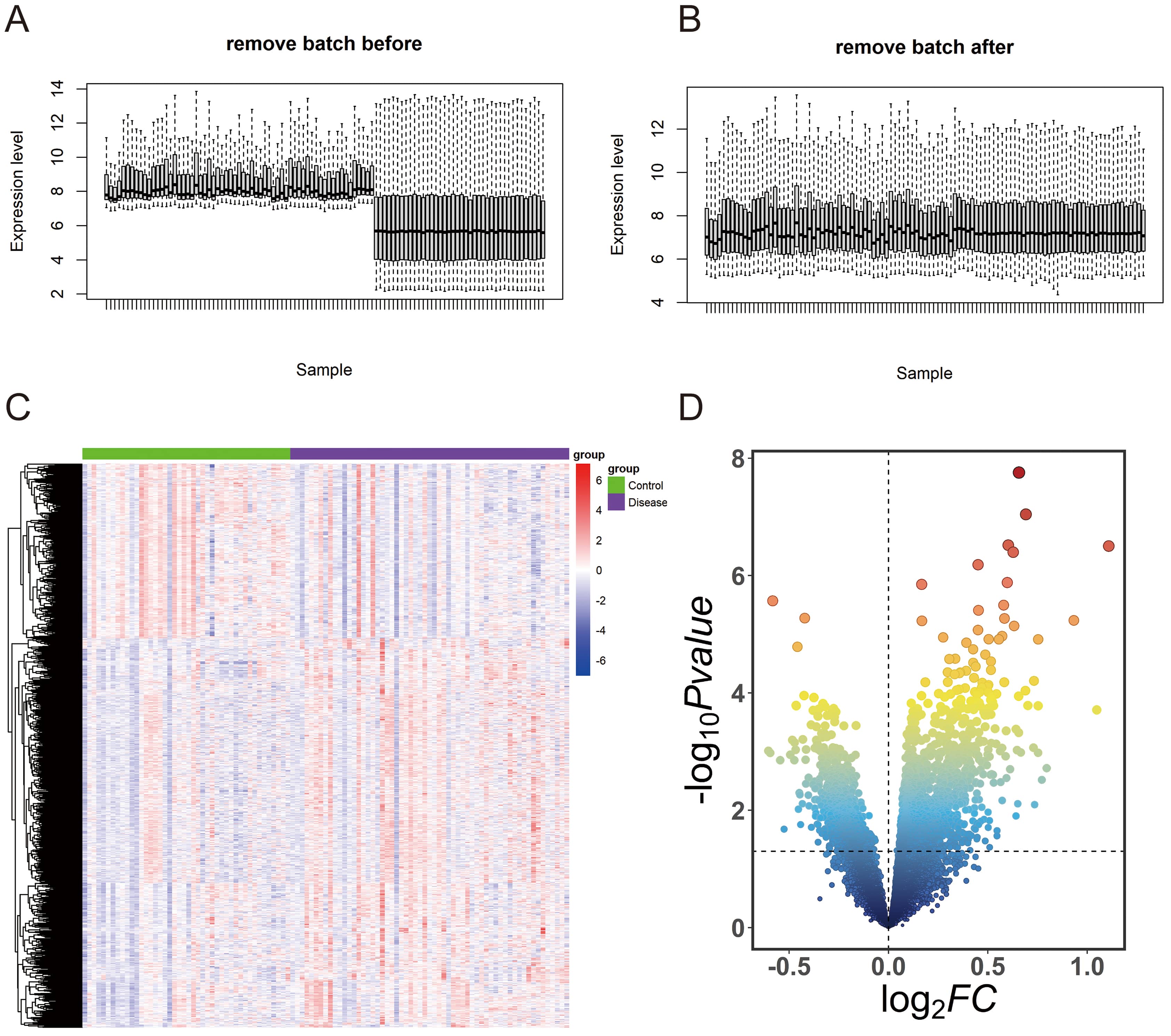
Differentially expressed genes (DEGs) analysis in cerebral stroke. (A) Expression level of sample screened in GEO database before removing batch. (B) Expression level of sample screened in GEO database after removing batch. (C) Heatmap of DEGs. (D) Volcano plot of DEGs. GEO, Gene Expression Omnibus.
We obtained a total of 9217 cerebral stroke-related genes and 191 active compounds of LCH. Among these, 43 active compounds were found to target 195 specific genes. By intersecting the cerebral stroke-related genes, LCH target genes, and DEGs, we identified a set of 55 genes that included 52 upregulated genes and 3 downregulated genes (Fig. 2A and Table 1). The 55 genes and active compounds of LCH were intersected using Cytoscape to construct a compound-target network (Fig. 2B). This network consisted of 85 nodes and 78 relationships. Oleic acid (CID: 445639) exhibited the highest degree of connectivity, interacting with 11 targets. Caffeic acid (CID: 689043) was the second most connected compound, interacting with 10 targets.
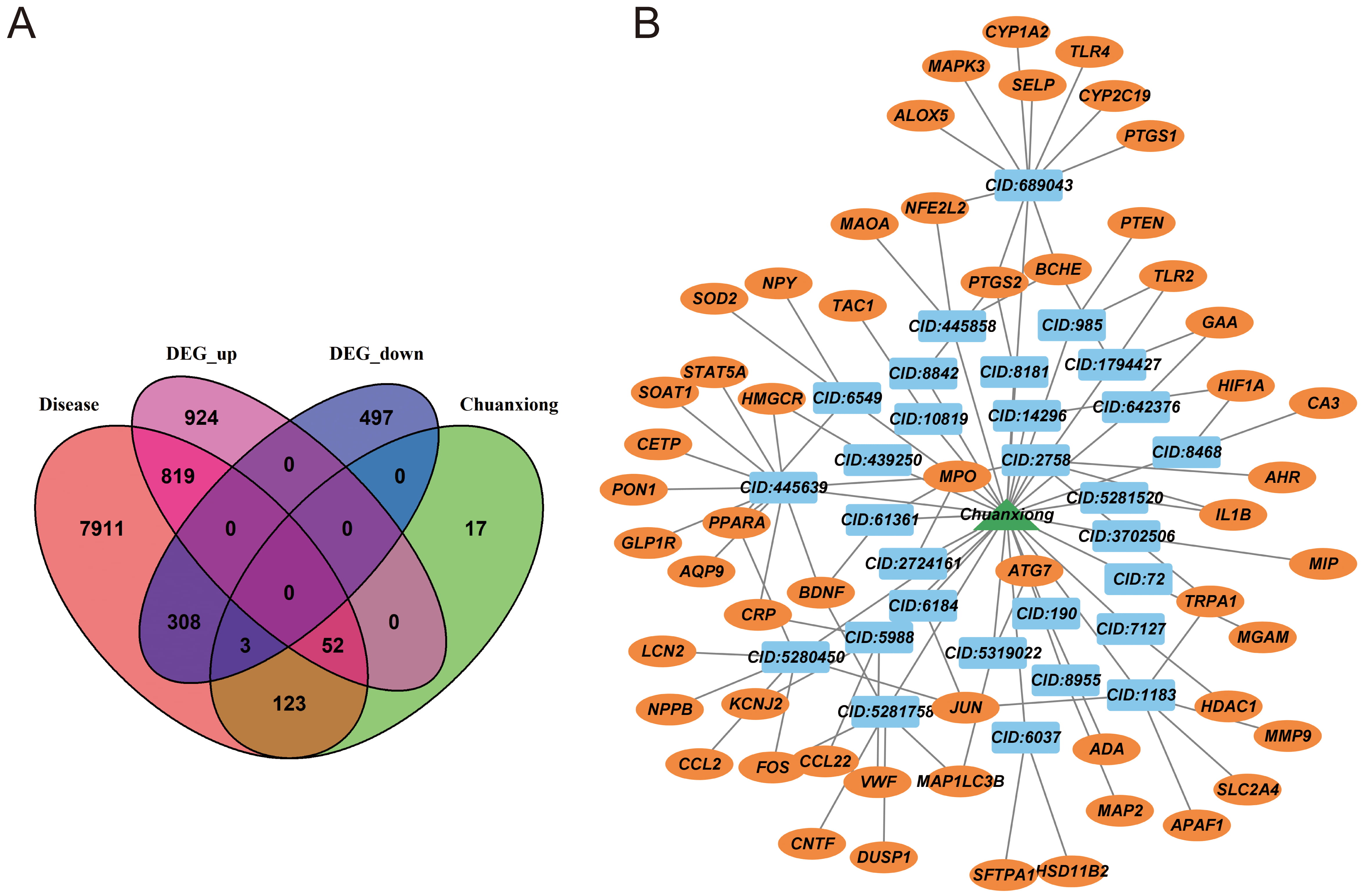
Targets of Ligusticum Chuanxiong Hort. (LCH) against cerebral stroke. (A) Venn diagram of cerebral stroke-related genes, DEGs, and LCH-target genes. (B) Compound-target network.
Gene name | Source | Uniprot | Gene name | Source | Uniprot |
PPARA | DisGeNET | Q07869 | TLR2 | DisGeNET | O60603 |
ADA | GeneCards | P00813 | ATG7 | DisGeNET | O95352 |
HDAC1 | GeneCards | Q13547 | FOS | DisGeNET | P01100 |
CRP | DisGeNET | P02741 | NPPB | DisGeNET | P16860 |
CYP2C19 | DisGeNET | P33261 | SOD2 | DisGeNET | P04179 |
ALOX5 | DisGeNET | P09917 | STAT5A | DisGeNET | P42229 |
IL1B | DisGeNET | P01584 | TAC1 | DisGeNET | P20366 |
MMP9 | DisGeNET | P14780 | GAA | GeneCards | P10253 |
PON1 | DisGeNET | P27169 | HMGCR | GeneCards | P04035 |
PTGS2 | DisGeNET | P35354 | MAP2 | GeneCards | P11137 |
BDNF | DisGeNET | P23560 | KCNJ2 | GeneCards | P63252 |
TLR4 | DisGeNET | O00206 | HSD11B2 | GeneCards | P80365 |
VWF | DisGeNET | P04275 | SLC2A4 | GeneCards | P14672 |
SELP | DisGeNET | P16109 | BCHE | GeneCards | P06276 |
NFE2L2 | DisGeNET | Q16236 | MAOA | GeneCards | P21397 |
HIF1A | DisGeNET | Q16665 | CYP1A2 | GeneCards | P05177 |
CCL2 | DisGeNET | P13500 | SFTPA1 | GeneCards | Q8IWL2 |
CETP | DisGeNET | P11597 | SOAT1 | GeneCards | P35610 |
PTGS1 | DisGeNET | P23219 | CNTF | GeneCards | P26441 |
MAPK3 | DisGeNET | P27361 | MGAM | GeneCards | O43451 |
LCN2 | DisGeNET | P80188 | TRPA1 | GeneCards | O75762 |
MPO | DisGeNET | P05164 | APAF1 | GeneCards | O14727 |
NPY | DisGeNET | P01303 | DUSP1 | GeneCards | P28562 |
GLP1R | DisGeNET | P43220 | MAP1LC3B | GeneCards | Q9GZQ8 |
AQP9 | DisGeNET | O43315 | CCL22 | GeneCards | O00626 |
JUN | DisGeNET | P05412 | AHR | GeneCards | P35869 |
MIP | DisGeNET | P30301 | CA3 | GeneCards | P07451 |
PTEN | DisGeNET | P60484 |
LCH, Ligusticum Chuanxiong Hort.
The reciprocal proteins of the 55 intersecting genes were predicted by the STRING database and a PPI network was constructed in Cytoscape. The PPI network consisted of 62 nodes and 393 edges in the network (Fig. 3A). To identify the core genes within the PPI network, the MCC algorithm in the CytoHubba plugin was applied. The top 15 hub genes, ranked by node degree, were IL1B, CCL2, MAPK3, PTGS2, JUN, MMP9, TLR4, HIF1A, PPARA, FOS, PTEN, NFE2L2, TLR2, IMP1, and SOD2 (Fig. 3B). These genes showed a high level of connectivity within the network, indicating their potential importance in the context of cerebral stroke.
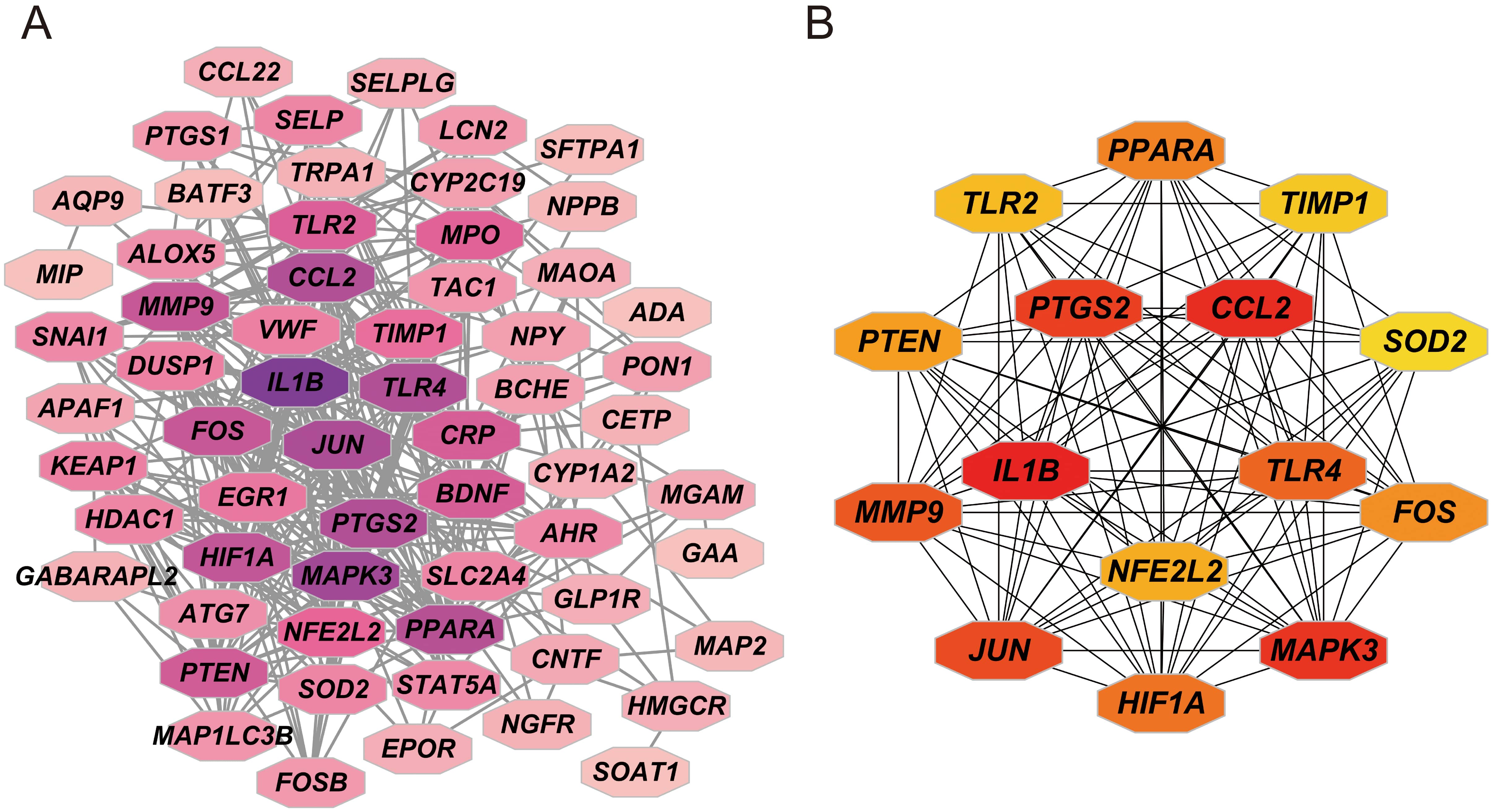
Protein-protein interaction (PPI) network. (A) PPI network of targets in Ligusticum Chuanxiong Hort. (LCH) against cerebral stroke. (B) PPI network of top 15 hub genes.
A total of 743 results were enriched in the GO enrichment analysis. The top 15 results were filtered based on the p-value. In the KEGG pathway enrichment analysis, 93 signaling pathways were enriched and the top 15 results were displayed based on the p-value. The GO enrichment analysis results were primarily related to cellular response to chemical stress, response to oxidative stress, and response to extracellular stimulus, etc. (Fig. 4A). The KEGG pathways include the TNF signaling pathway, Lipid and atherosclerosis, and IL-17 signaling pathway, etc. (Fig. 4B).
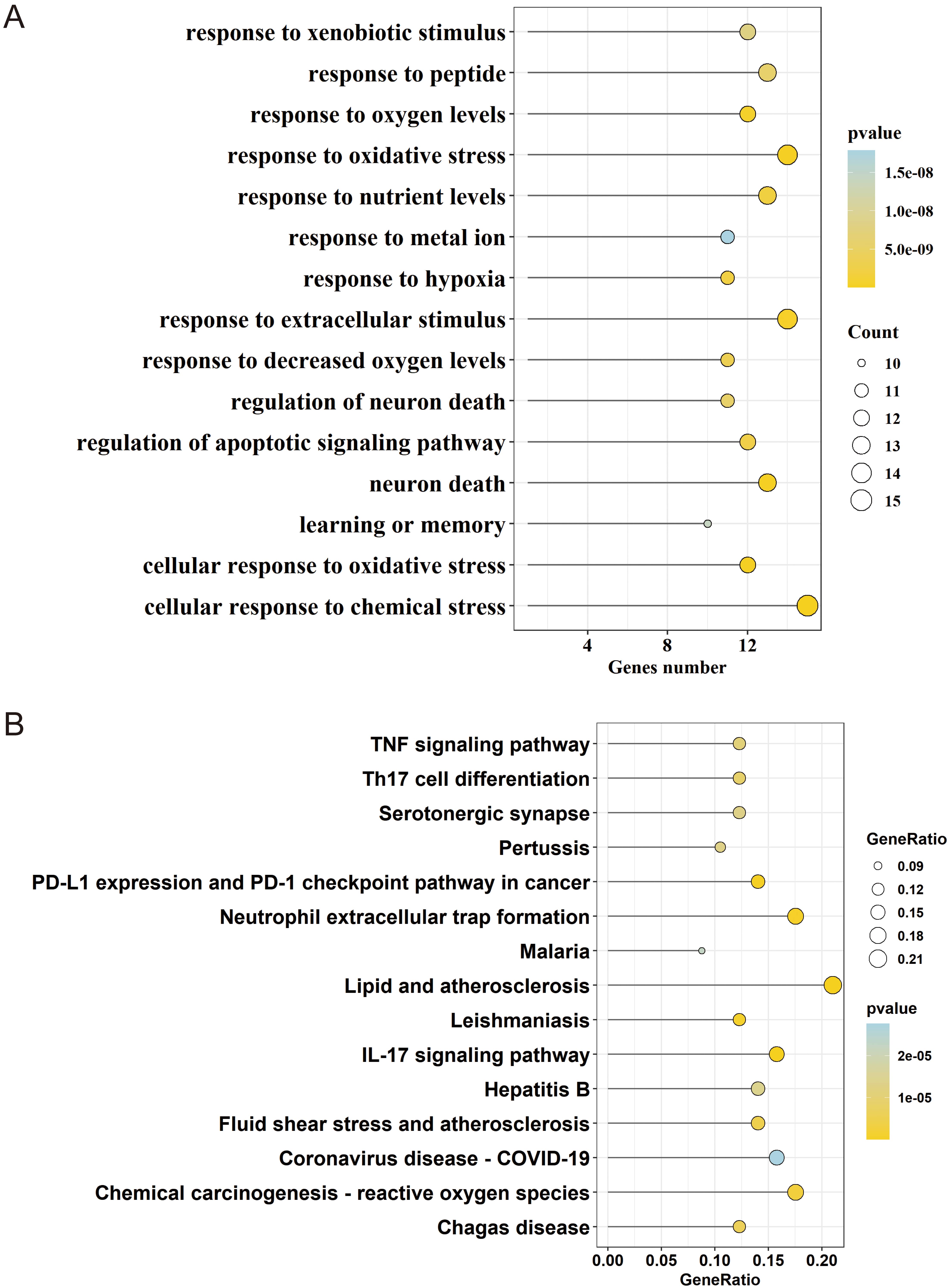
Gene Ontology (GO) biological functional analysis and Kyoto Encyclopedia of Genes and Genomes (KEGG) pathway enrichment analysis. (A) Bubble of GO enrichment analysis. (B) Bubble of KEGG enrichment analysis. The size of the dot indicates the number of genes annotated in the entry and the color indicates the range of the p-value, with lower values represented by darken shades of yellow.
To assess the diagnostic capability of the identified hub genes in cerebral stroke, the lasso logistic regression algorithm was used. The penalty parameter tuning process was performed using 10-fold-cross-validation (Fig. 5A). Based on the analysis, six genes (FOS, MMP9, PPARA, PTEN, TIMP1, and TLR2) were identified as diagnostic markers for cerebral stroke. The model parameters are shown in Table 2. A total of 60% of samples screened from GEO were randomly selected as the training set, and the rest of the samples were set as the test set. The area under curve (AUC) value of the model in the training set was 0.927 and 0.855 in the test set (Fig. 5B). Considering that age and sex are also important risk factors for cerebral stroke, the predictive performance of the model was further examined by sex and age within the training and test sets. The model had AUC values greater than 0.915 in the training set for sex and were greater than 0.86 for age (greater than 60 for the high age group and less than 60 for the low age group) (Fig. 5C,D). The values in the test set were more than 0.8 for sex and more than 0.78 for age (Fig. 5E,F).
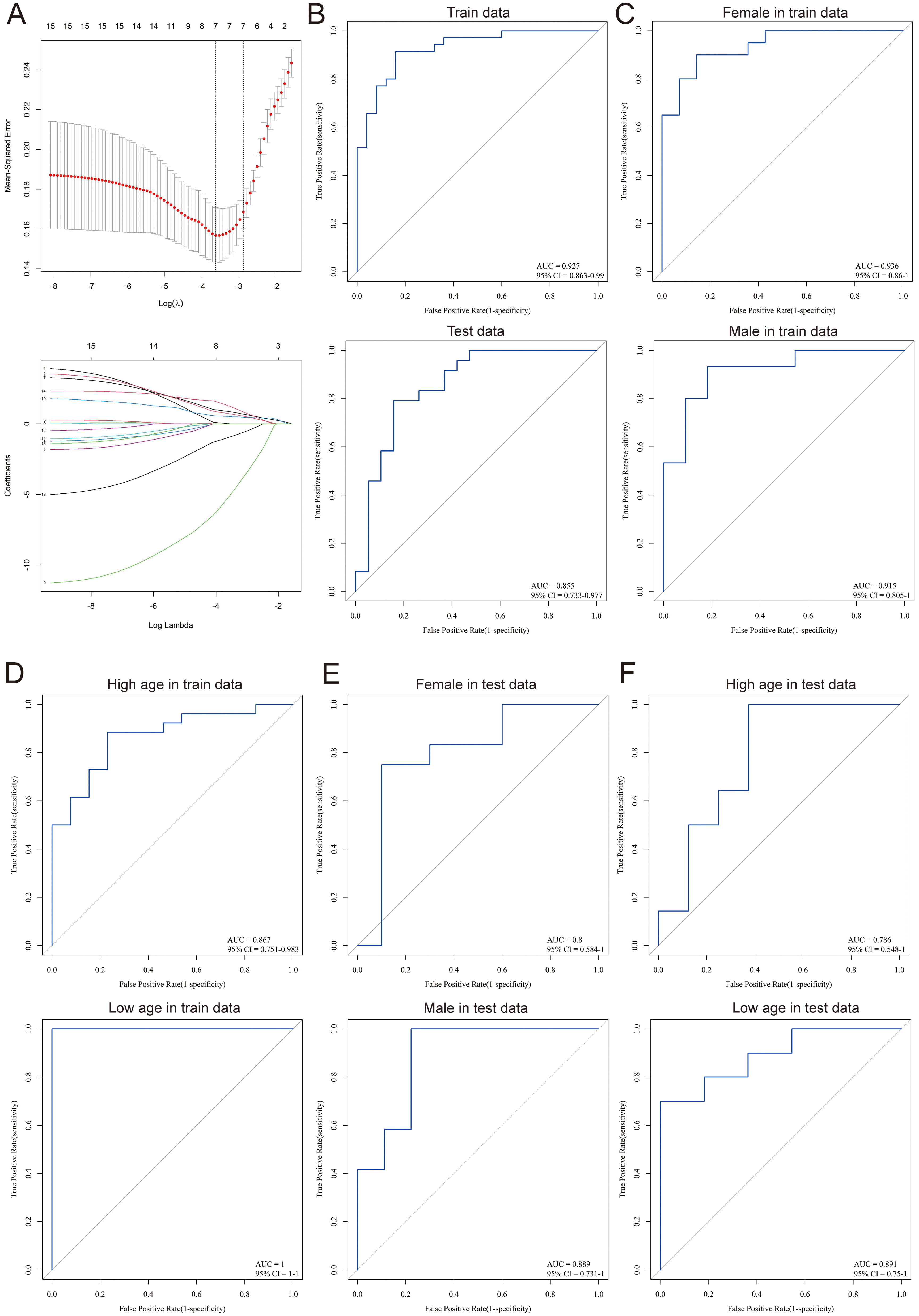
Diagnostic model of cerebral stroke. (A) Screening processes for diagnostic biomarkers for cerebral stroke. (B) Receiver operating characteristic curve (ROC) of the training set and test set. (C) Sex-related ROC in the training set. (D) Age-related ROC in the training set. (E) Sex-related ROC in the test set. (F) Age-related ROC in the test set. CI, confidence interval; AUC, area under curve.
Biomarkers | Coefficient |
(Intercept) | 2.911167399 |
FOS | 0.438506697 |
MMP9 | 0.590230333 |
PPARA | –3.033421637 |
PTEN | 0.454911348 |
TIMP1 | –0.392333131 |
TLR2 | 0.625647023 |
The target genes corresponding to each active compound in LCH were individually intersected with the 55 genes and the results are shown in Table 3. Among them, oleic acid (CID: 445639) and caffeic acid (CID: 689043) emerged as the core active compounds of LCH. The model marker genes FOS, MMP9, PPARA, PTEN, TIMP1, and TLR2 were selected for molecular docking with oleic acid and caffeic acid. The criteria were that the binding energy be less than –5.0 kcal/mol and that hydrogen bonds could be formed between the receptor ligands [14]. The docking results are summarized in Table 4. Caffeic acid was combined with MMP9, PPARP, PTEN, and TIMP1, with binding energies of –7.9, –6.4, –5.9, and –6.9 kcal/mol, respectively (Fig. 6A). Oleic acid bound well with MMP9 (–6.6 kcal/mol), PPARA (–6 kcal/mol), and TIMP1 (–5.5 kcal/mol) (Fig. 6B). Molecular docking simulations indicated a stable point docking structure for the binding of small-molecule ligands and protein receptors (Fig. 6A,B).
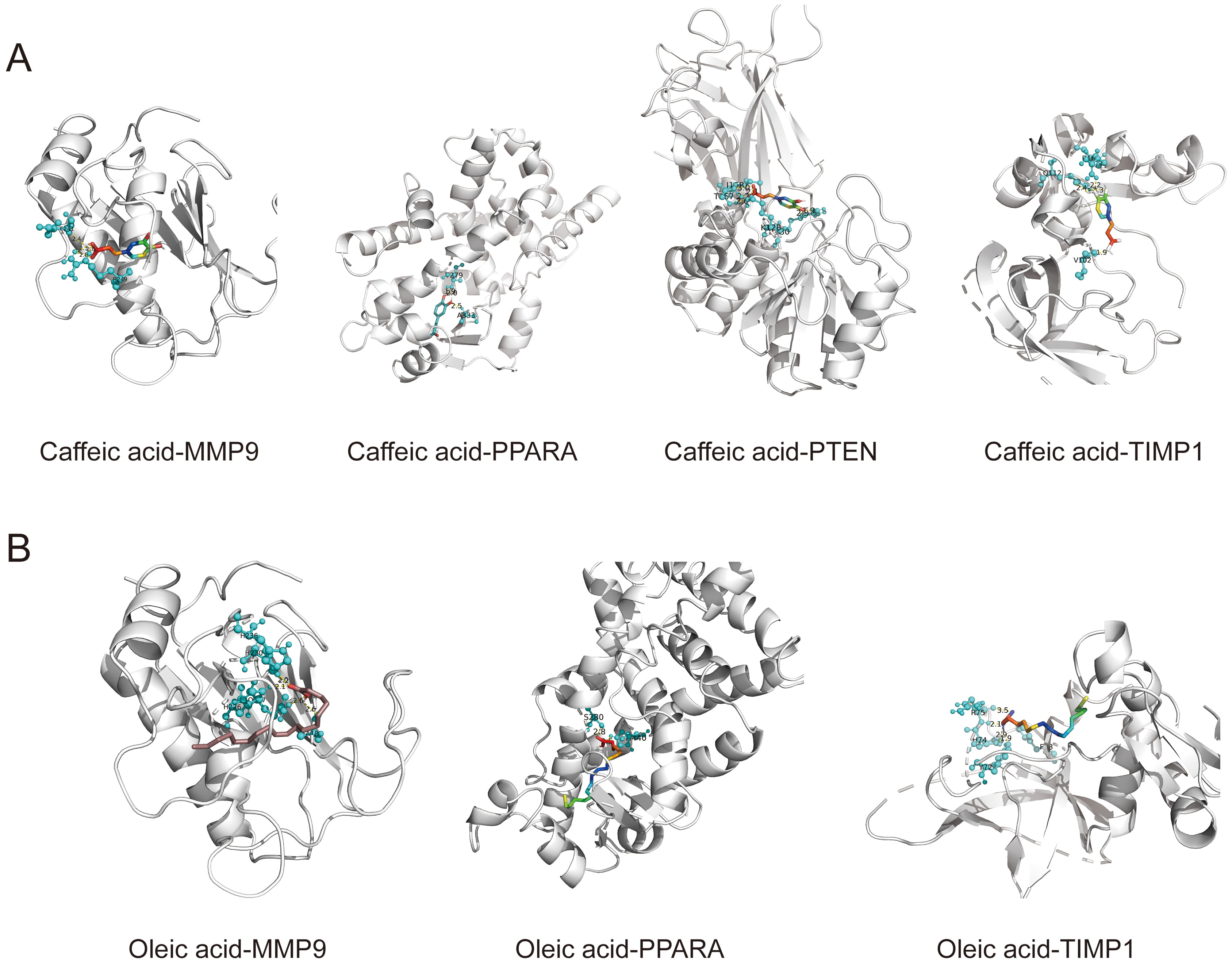
Docking model. (A) Caffeic acid with MMP9, PPARA, PTEN, and TIMP1. (B) Oleic acid with MMP9, PPARA, and TIMP1.
Compound | Count | Gene |
CID: 445639 | 11 | MPO, PPARA, HMGCR, BDNF, CRP, PON1, CETP, SOAT1, AQP9, GLP1R, STAT5A |
CID: 689043 | 10 | ALOX5, CYP2C19, BCHE, CYP1A2, TLR4, PTGS1, PTGS2, MAPK3, SELP, NFE2L2 |
CID: 5280450 | 6 | PPARA, LCN2, CCL2, FOS, NPPB, JUN |
CID: 1183 | 5 | MMP9, JUN, APAF1, TRPA1, SLC2A4 |
CID: 5281758 | 5 | DUSP1, FOS, BDNF, MAP1LC3B, CNTF |
CID: 2758 | 4 | TRPA1, IL1B, AHR, MPO |
CID: 5988 | 4 | CCL22, KCNJ2, CRP, VWF |
CID: 1794427 | 3 | GAA, BCHE, TLR2 |
CID: 445858 | 3 | NFE2L2, BCHE, MAOA |
CID: 6549 | 3 | PPARA, NPY, SOD2 |
CID: 5319022 | 2 | ATG7, MAP1LC3B |
CID: 6037 | 2 | HSD11B2, SFTPA1 |
CID: 61361 | 2 | MPO, BDNF |
CID: 8468 | 2 | HIF1A, CA3 |
CID: 985 | 2 | TLR2, PTEN |
CID: 10819 | 1 | TAC1 |
CID: 14296 | 1 | HIF1A |
CID: 190 | 1 | ADA |
CID: 2724161 | 1 | MPO |
CID: 3702506 | 1 | MIP |
CID: 439250 | 1 | HMGCR |
CID: 5281520 | 1 | IL1B |
CID: 6184 | 1 | JUN |
CID: 642376 | 1 | GAA |
CID: 7127 | 1 | HDAC1 |
CID: 72 | 1 | MGAM |
CID: 8181 | 1 | PTGS2 |
CID: 8842 | 1 | PTGS2 |
CID: 8955 | 1 | MAP2 |
LCH, Ligusticum Chuanxiong Hort.
Target | Pubchem_ID | Compound | Free binding energy (kcal/mol) |
MMP9 | 689043 | Caffeic Acid | –7.9 |
445639 | Oleic Acid | –6.6 | |
PPARA | 689043 | Caffeic Acid | –6.4 |
445639 | Oleic Acid | –6 | |
PTEN | 689043 | Caffeic Acid | –5.9 |
TIMP1 | 689043 | Caffeic Acid | –6.9 |
445639 | Oleic Acid | –5.5 |
Cell validation experiments were performed to validate the results obtained from
molecular docking. An OGD model was induced in SH-SY5Y cells, which were then
treated with LCH extract. After treatment with 0, 12.5, 25, 50, and 100
µg/mL LCH, cell viability showed no significant difference (Fig. 7A), indicating the safety of LCH in SH-SY5Y cells. Subsequently, 25, 50, and 100
µg/mL LCH were chosen for further experiments. In comparison with
control cells, the OGD-induced cells showed lower cell viability and higher LDH
releasing (p
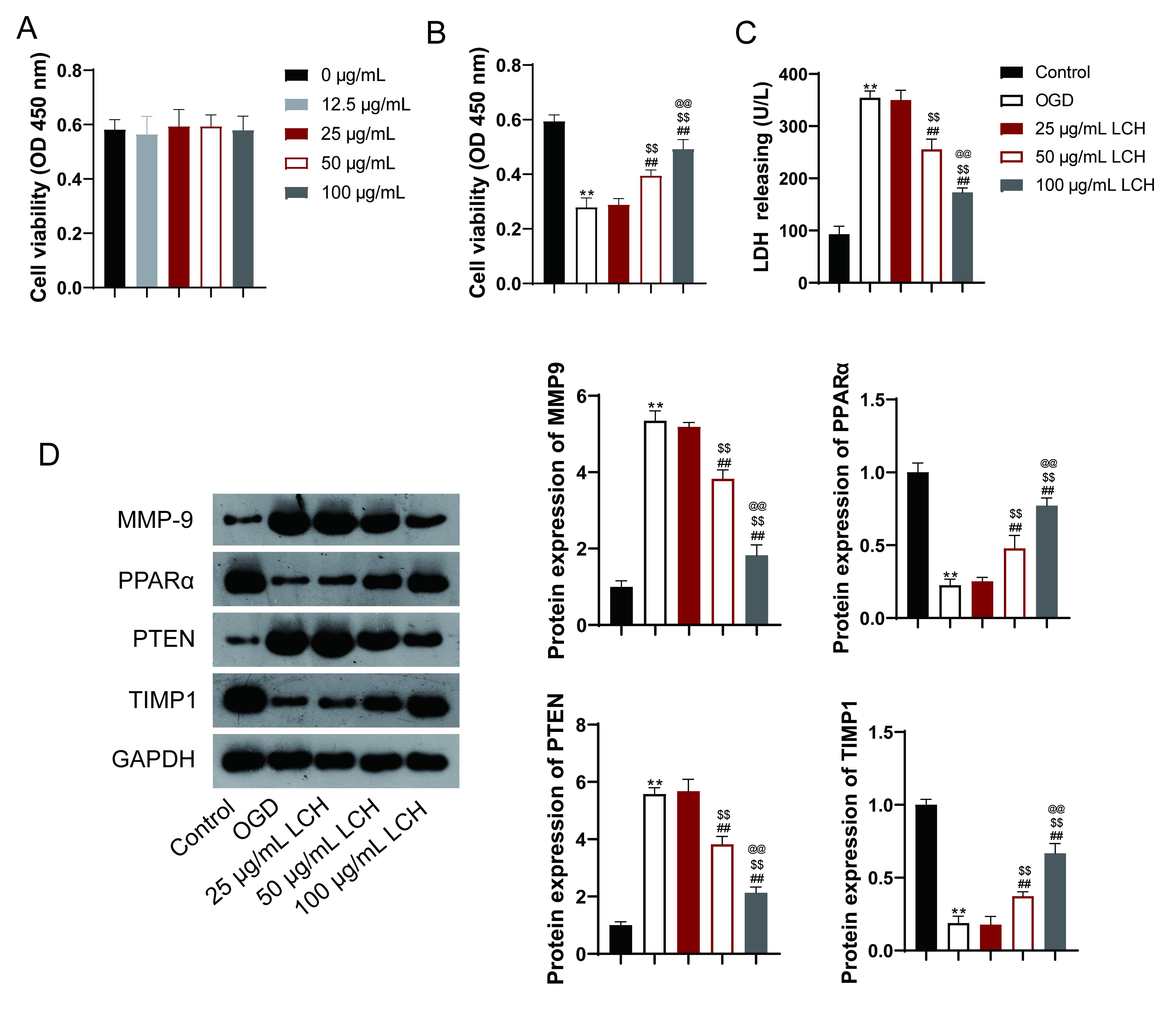
LCH regulates the protein expression of identified targets. (A)
Cell viability was determined by a Cell Counting Kit-8 (CCK-8) assay. SH-SY5Y
cells were treated with different concentrations of LCH extract (0, 12.5, 25, 50,
and 100 µg/mL). (B) Cell viability was determined using a CCK-8 assay. (C)
LDH analysis. (D) Western blotting detected expression of MMP9, PPARA, PTEN, and
TIMP1. Oxygen and glucose deprivation (OGD)-induced SH-SY5Y cells were treated
with different concentrations of LCH extract (25, 50, and 100 µg/mL).
Regarding the identified targets, the protein expression levels of MMP9 and PTEN
were found to be increased, while those of PPARA and TIMP1 were decreased in the
OGD-induced cells compared to control cells (p
In this study, we used a combination of network pharmacology, transcriptomic, and molecular docking analyses to explore the potential mechanisms of LCH in treating cerebral stroke. The analysis of the active compound-target network showed that compounds such as oleic acid and caffeic acid, among others, interact with various targets in the network. This suggests that these compounds may be crucial for the therapeutic effect of LCH in treating cerebral stroke. Oleic acid is an important constituent of membrane phospholipids in the brain and is highly concentrated in myelin [15]. It has been shown to act as a neurotrophic factor and contribute to the synthesis of myelin phospholipids during brain development [16, 17]. Additionally, oleic acid is released from brain phospholipids and exhibits neuroprotective effects after cerebral ischemia [18]. Caffeic acid is a phenolic compound with many pharmacological functions, including antioxidant and anti-inflammatory properties [19]. It has been shown to protect against cerebral ischemia-reperfusion injury and reduce cerebrovascular inflammation in an animal model of stroke [20].
Furthermore, our results revealed that both caffeic acid and oleic acid showed favorable binding energy with proteins such as MMP9, PPARA, PTEN, and TIMP1. These proteins are related to inflammation, oxidative stress, and vascular permeability in brain injury. MMP9 is an important prognostic factor for ischemic stroke [21], and caffeic acid has been reported to inhibit MMP9 expression in various forms of cancer [22]. PPARA regulates autophagy in the nervous system [23]. PTEN participates in the regulation of blood-brain barrier permeability [24]. TIMP1 can preserve the blood-brain barrier [25]. Additionally, our validation experiments revealed that LCH reduced injury of OGD-induced cells and modulated protein expression of MMP9, PPARA, PTEN, and TIMP1. Together these results demonstrate that LCH may exert its therapeutic effects on cerebral stroke by targeting MMP9, PPARA, PTEN, and TIMP1.
To gain further insight into the mechanisms underlying the therapeutic effects
of LCH for cerebral stroke we performed GO and KEGG enrichment analyses. The
results showed that the top 15 BP terms were mainly associated with chemical
stress, extracellular stimulus, oxidative stress, neuron death, and apoptosis.
The pathophysiological mechanisms of stroke are complex. It is well known that
cerebral stroke is related to lifestyle factors (hypertension, obesity, and
smoking), oxidative stress, inflammation, etc. [26, 27]. Ischemic stroke
generally affects a large area of the brain [28]. The KEGG pathway is mainly
enriched in the TNF signaling pathway and IL-17 signaling pathway. Both are
related to inflammation, a hallmark of stroke pathology [29]. TNF inhibitors are
candidate therapies for ischemic stroke. According to a previous study,
TNF-
Additionally, we constructed a diagnostic model for LCH against cerebral stroke. Results revealed that FOS, MMP9, PPARA, PTEN, TIMP1, and TLR2 are blood biomarkers of cerebral stroke. Previous studies have also demonstrated the association between these genes and cerebral stroke. For example, MMP9, TLR2, and TIMP1 are related to proinflammatory pathways following stroke [35, 36, 37] and inducing PTEN degradation can attenuate cerebral stroke [38]. Our data also revealed that age and sex can affect the diagnosis of stroke.
Our study revealed the therapeutic value of LCH for treating cerebral stroke; however, there are some limitations. First, this study lacked the use of in vivo animal models, limiting the translation of our findings. Second, cerebral stroke is a multifactorial condition and further study could focus on the role of LCH in lacunar ischemic strokes, a common type of stroke and the first clinical manifestation of small vessel disease [39].
The present study analyzed the mechanisms underlying the therapeutic effect of LCH in cerebral stroke. Our research revealed that LCH has various active compounds that are likely to underlie its therapeutic effects following a cerebral stroke, including oleic acid and caffeic acid. These active compounds target multiple genes to exert their function via TNF and IL-17 signaling pathways. Molecular docking showed that oleic acid and caffeic acid had good binding sites with hub targets. Additionally, FOS, MMP9, PPARA, PTEN, TIMP1, and TLR2 were identifies as blood biomarkers of cerebral stroke. Our study offers a reference for further exploration of the mechanism of LCH in cerebral stroke.
TCM, traditional Chinese medicine; LCH, Ligusticum Chuanxiong Hort.; DEGs, Differentially Expressed Genes; PPI, Protein-protein Interaction; GO, Gene Ontology; KEGG, Kyoto Encyclopedia of Genes and Genomes.
The datasets used and/or analyzed during the current study are available from the corresponding author on reasonable request.
JZ, CC, and FQ designed the research study. JZ, CC, YL and YW performed the research. FQ provided help and advice on the introduction and discussion writing. JZ, CC, and FQ analyzed the data. All authors contributed to editorial changes in the manuscript. All authors read and approved the final manuscript. All authors have participated sufficiently in the work and agreed to be accountable for all aspects of the work.
Not applicable.
Not applicable.
This research received no external funding.
The authors declare no conflict of interest.
Publisher’s Note: IMR Press stays neutral with regard to jurisdictional claims in published maps and institutional affiliations.