Background: The computer-based simulation of the whole processing route for speech production and speech perception in a neurobiologically inspired way remains a challenge. Only a few neural based models of speech production exist, and these models either concentrate on the cognitive-linguistic component or the lower-level sensorimotor component of speech production and speech perception. Moreover, these existing models are second-generation neural network models using rate-based neuron approaches. The aim of this paper is to describe recent work developing a third-generation spiking-neuron neural network capable of modeling the whole process of speech production, including cognitive and sensorimotor components. Methods: Our neural model of speech production was developed within the Neural Engineering Framework (NEF), incorporating the concept of Semantic Pointer Architecture (SPA), which allows the construction of large-scale neural models of the functioning brain based on only a few essential and neurobiologically well-grounded modeling or construction elements (i.e., single spiking neuron elements, neural connections, neuron ensembles, state buffers, associative memories, modules for binding and unbinding of states, modules for time scale generation (oscillators) and ramp signal generation (integrators), modules for input signal processing, modules for action selection, etc.). Results: We demonstrated that this modeling approach is capable of constructing a fully functional model of speech production based on these modeling elements (i.e., biologically motivated spiking neuron micro-circuits or micro-networks). The model is capable of (i) modeling the whole processing chain of speech production and, in part, for speech perception based on leaky-integrate-and-fire spiking neurons and (ii) simulating (macroscopic) speaking behavior in a realistic way, by using neurobiologically plausible (microscopic) neural construction elements. Conclusions: The model presented here is a promising approach for describing speech processing in a bottom-up manner based on a set of micro-circuit neural network elements for generating a large-scale neural network. In addition, the model conforms to a top-down design, as it is available in a condensed form in box-and-arrow models based on functional imaging and electrophysiological data recruited from speech processing tasks.
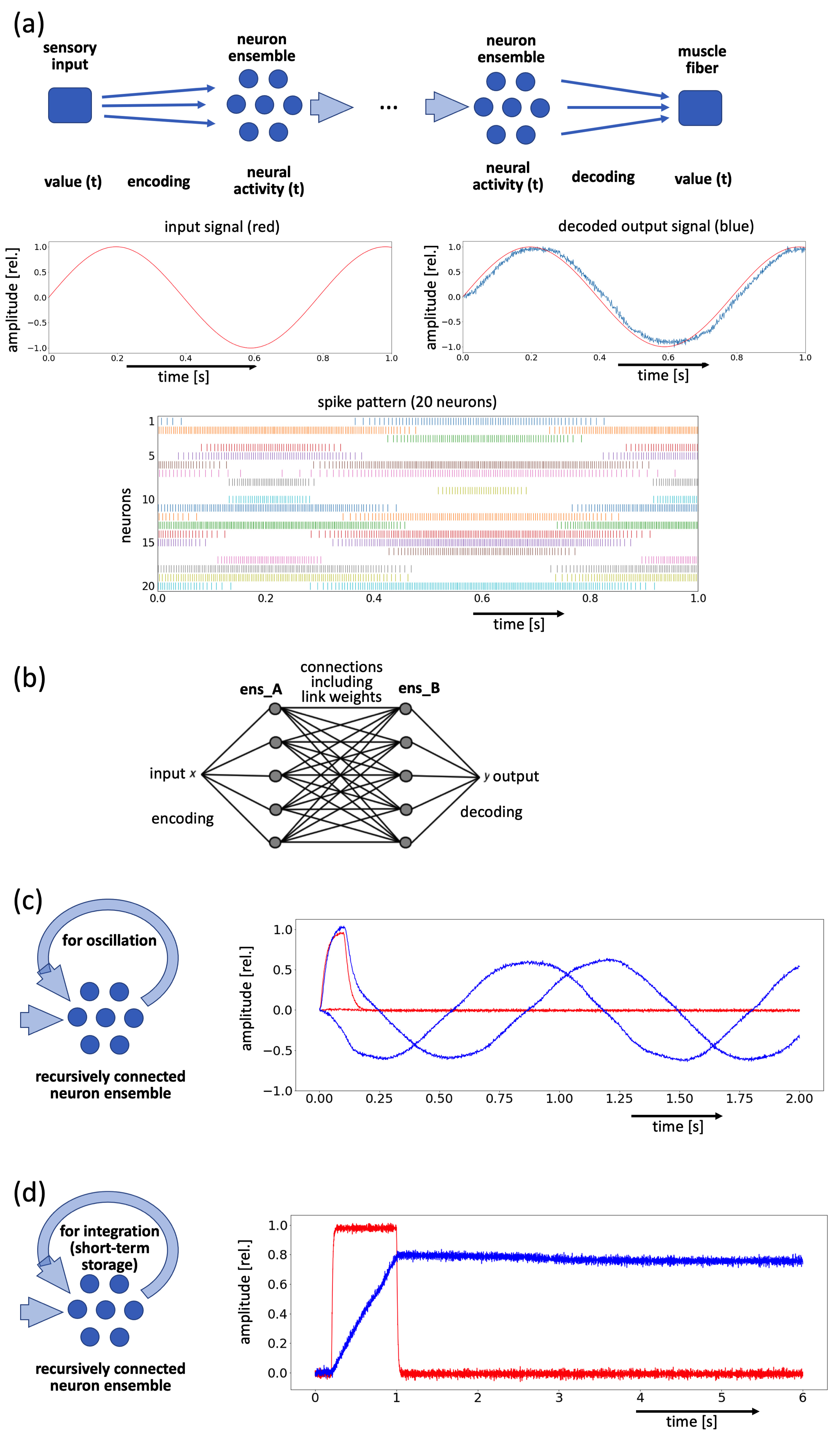