- Academic Editor
Background: With the advent of portable neurophysiological methods, including electroencephalography, progress in studying brain activity during physical tasks has received considerable attention, predominantly in clinical exercise and sports studies. However, the neural signatures of physical tasks in everyday settings were less addressed. Methods: Electroencephalography (EEG) indices are sensitive to fluctuations in the human brain, reflecting spontaneous brain activity with an excellent temporal resolution. Objective: In this regard, this study attempts to systematically review the feasibility of using EEG indices to quantify human performance in various physical activities in both laboratory and real-world applications. A secondary goal was to examine the feasibility of using EEG indices for quantifying human performance during physical activities with mental tasks. The systematic review was conducted based on the updated Preferred Reporting Items for Systematic Reviews and Meta-Analyses guidelines. Results: Out of 81 studies, 64 task studies focused on quantifying human performance concerning physical activity, whereas 17 studies focused on quantifying human performance on physical activities associated with mental tasks. EEG studies have primarily relied on linear methods, including the power spectrum, followed by the amplitude of Event-related potential components, to evaluate human physical performance. The nonlinear methods were relatively less addressed in the literature. Most studies focused on assessing the brain activity associated with muscular fatigue tasks. The upper anatomical areas have been discussed in several occupational schemes. The studies addressing biomechanical loading on the torso and spine, which are the risk factors for musculoskeletal disorders, are less addressed. Conclusions: Despite the recent interest in investigating the neural mechanisms underlying human motor functioning, assessing the brain signatures of physical tasks performed in naturalistic settings is still limited.
Neuroergonomics, the study of the brain and behavior at work, applies methods and tools from neuroscience and neuroengineering to human factors, ergonomics and engineering for understanding the human brain at work and in everyday life [1, 2, 3]. Traditional domains of human factors and ergonomics are categorized into cognitive, physical, and organizational ergonomics [4]. Cognitive ergonomics is associated with different mental processes such as perception, reasoning, decision making, information processing, and memory. Physical ergonomics is concerned with biomechanical, anthropometric, human anatomical, and physiological characteristics. Progress in neuroergonomics research to date mainly focused on analyzing the neural behavior in the cognitive domain of human activity, while few studies were conducted in the physical domain [3, 5, 6, 7, 8]. Since humans are engaged daily with tasks that require human body or limb movements alongside cognitive processing, integrating both physical and cognitive considerations should be applied in future neuroergonomics studies to understand better the human capabilities and limitations at work [9, 10, 11].
The human brain is composed of over 100 billion neurons that communicate via
electrical signals generating an electrical current, which subsequently creates
wave patterns termed as brain signals [12]. The brain signals measured in hertz
(Hz) including delta (
One limitation in EEG data is the high contamination of non-neural signals termed as “artifacts”. Artifacts create abnormal and irregular signal patterns yielding to data distortion, and thus reducing the signal output quality [20]. Artifacts are categorized into physiological and non-physiological sources [21]. Physiological artifacts occur due to the eye or head movements, glossokinetic movement, muscular, respiratory, cardiac activities, and sweat bridges [22] whereas the non-physiological artifacts arise from the external environments such as movement of electrodes, broken electrodes, bad electrode contact, cable movements, power line, electrical equipment (e.g., cell phones and air conditioner) and the light source that is 60 Hz or above [23, 24]. Considerable research has been devoted to detecting, separate, and isolating the EEG artifacts. Preventing movement artifacts entirely from the data is impossible, but reducing and separating some is applicable [25, 26]. The challenging aspect is not only to remove movement artifacts but also to assess their impact on the results.
EEG indices are reliable indicators that reflect the spontaneous activity in the brain. In this regard, it is essential to explore the research into EEG indices in physical activities. An emerging body of knowledge illustrates the applications of EEG techniques to characterize the brain signatures in several applications, including brain-computer interfaces (BCI) [27], sports [28], human factors [29], neuromarketing [27] and clinical and psychiatric domains [30]. This study follows up on a prospective review by Rahman et al. [31], which discussed the applications of EEG technique in physical activities. Our current study systematically explores the use of EEG indices in quantifying human physical performance to reveal the current state of knowledge about characterizing the neural mechanisms associated with physical tasks based on the predefined research questions as follows:
RQ1: What are the different domains that address the applications of EEG to assess work-related physical activities?
RQ2: What are the dominant EEG indices used to quantify human performance in physical activities?
RQ3: What are the aspects of EEG measurement relevant to physical activities concerning methods of feature extraction, the number of channels, the number of participants and their gender, and methods of artifact removal that have been addressed to date?
RQ4: What are the current limitations in characterizing human physical performance using EEG data?
To the best of our knowledge, there is no comprehensive study that has reviewed the EEG indices used to quantify human performance to the assessment of physical activities. The current study might be helpful for future investigations that aim to evaluate the human brain activity associated with human physical tasks in the context of neuroergonomics.
The present study uses a systematic approach to review the applications of EEG indices used to quantify human performance at work either in the laboratory or real-life settings.
This systematic review was conducted using the updated guidelines for preferred reporting items for systematic reviews and meta-analyses (PRISMA) [32] and a checklist item. The checklist item we included in (Supplementary Material A) consists of 27 item checklists addressing the introduction, methods, results and discussion sections of a systematic review report. It is a crucial part of the PRISMA 2020 protocol as it helps to record the responses with the page number for each item in the checklist(s), which makes the reviewers and readers know what authors did and found, but also to optimize the quality of reporting and make the peer review process more efficient. The Cochrane Collaboration’s method has been used to minimize the risk of bias [33].
The articles in this review were selected after a search of the following databases: IEEE Xplore, SpringerLink, Google Scholar, and Web-of-Science. Some Boolean operators with specific keywords including “EEG” OR “Electroencephalography” AND “physical work” OR “physical task” OR “physical exercise” OR “physical activity” OR “physical movement” OR “movement-related cortical activity”. Conducted searches were not restricted to publication dates. A brief explanation of the strategy we followed for the literature search, including the used data bases, the combined searches with the Boolean operator, and keywords/terms are presented in Supplementary Material B. We further included an example of how we selected some articles from “IEEE Xplore database” according to our criteria of inclusions and exclusions.
Based on the updated PRISMA as shown in (Fig. 1), 830 articles were retrieved from databases. Duplicate studies (n = 130) were removed, and (n = 427) studies were removed as an initial screening based on study title with some exclusions including studies on brain diseases, studies on children or infants, and studies on animals. Therefore, (n = 273) records originally screened for eligibility. After reviewing all abstracts of the remaining articles, (n = 188) were further excluded. To collect all relevant articles during the literature search, the reference lists of the candidate articles (n = 75) were reviewed. Three researchers independently reviewed the 190 full text articles specifically (n = 115 from database and n = 75 from citations search) for inclusion and exclusion criteria. Exclusion criteria were applied to limit the final selection of the relevant studies. To meet the eligibility requirements, we have included published articles with the following criteria: (a) only English language publications; (b) experimental studies on healthy participants; (c) content from peer-reviewed journals, conference publications, textbooks, and reference books, and (d) physical activities representing the biomechanical properties of movements, such as grasping, gripping, finger wrist, elbow, arm, knee and hip movements that may be present during lifting, assembling, carrying, and placement tasks. Articles with the following features were excluded: (a) studies that were not associated with physical tasks; (b) multi-modal studies that do not present EEG results separately; (c) physical activity studies on infants or children; (d) physical activities studies on participants with neural disorders or brain diseases; and (e) physical in vigorous tasks that require high-intensity movements, such as jogging [34, 35], dancing [36], running [37], or jumping [38].
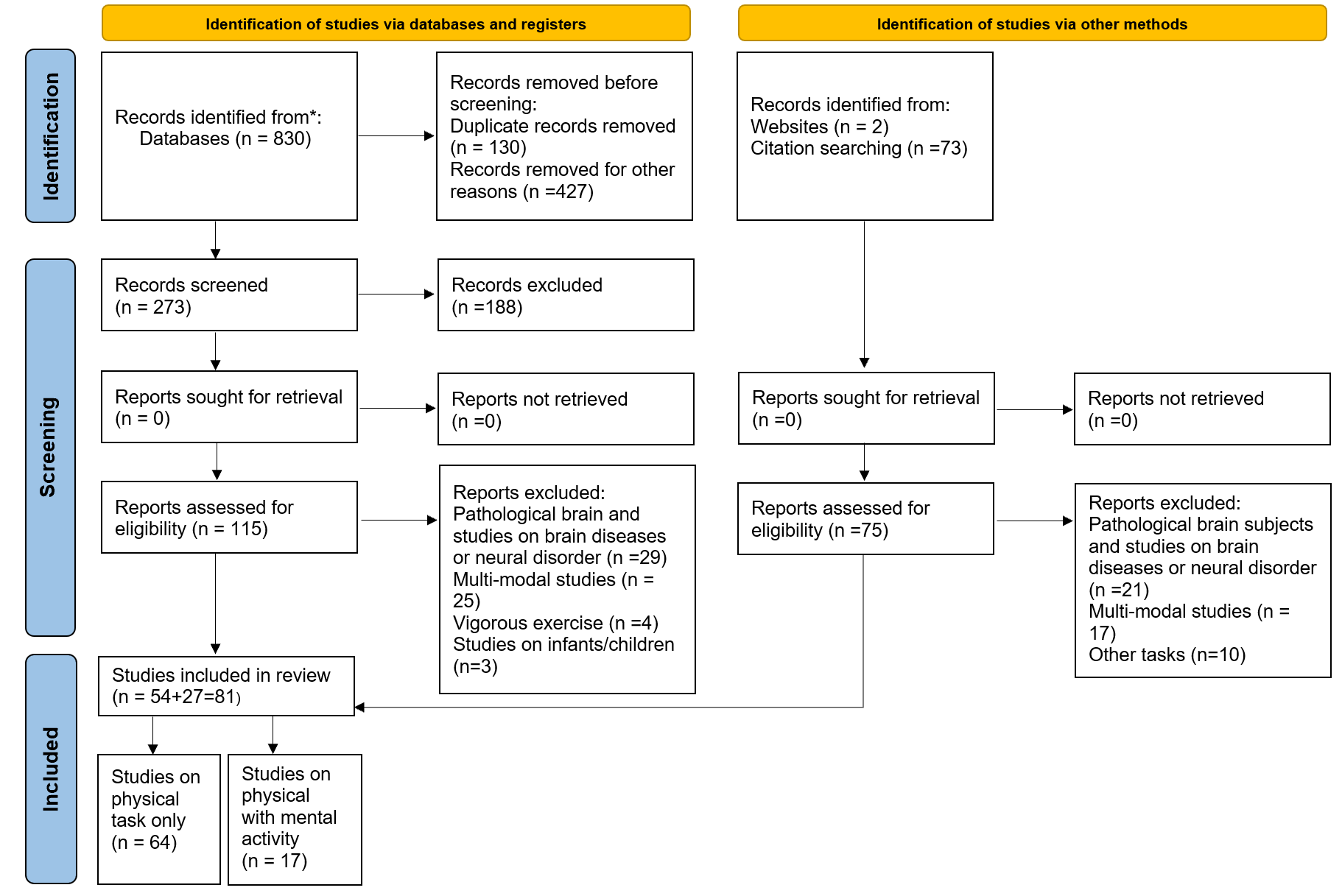
Flowchart of the methodology and process selection according to PRISMA.
Relevant information from the included articles was extracted and is summarized in Supplementary Material C, which illustrates the article reference, author name and year of publication, physiological measurements used, EEG reference used, EEG index analyzed, different domains, different experimental tasks, characteristics of participants in terms of gender and number, the EEG artifact removal method, and EEG feature extraction method.
The selected articles were classified according to the following eight domains: (1) physical, muscular, or neuromuscular fatigue, (2) movement observation, planning, and execution, (3) biomechanical properties (e.g., force, torque), (4) stressful and emotional exhaustion, (5) physical workload, (6) perception of physical effort, (7) motor training and learning, and (8) strength capability. Data extraction and synthesis were independently reviewed by three researchers.
Three researchers independently assessed the quality of the studies. Any
disagreement between the authors was resolved by consensus. The Cochrane
Collaboration’s method [39] was used to assess the risk of bias in each
experiment of the selected studies. The Cochrane Collaboration’s method has six
main domains of bias: (1) random sequence generation, (2) allocation concealment,
(3) blinding of participants and personnel, (4) blinding to outcome assessment,
(5) incomplete outcome data, and (6) selective reporting. The following judgments
were used to assess the quality of the articles: low risk of bias, unclear risk
of bias, or high risk of bias. To evaluate the strength of the evidence, we
applied the standards of the Agency for Healthcare Research and Quality AHRQ
[40]. A good quality article was judged to have a low risk of bias, a fair
quality article was judged to have two unclear risks of bias, and low-quality
article was judged to have a high risk of bias. The overall quality of the
studies was categorized into good, fair, or low, if the number of low-risk
domains was
A total of 81 articles were eligible for the final inclusion in the systematic literature review. The overall search process and the associated quantitative identifications are shown in (Fig. 1). Three researchers screened all papers to ensure the minimum bias, transparency, and keep that we covered a generic scope of everyday settings applications. Furthermore, disagreements between the three authors were resolved by consensus.
Of the 81 studies included in this systematic review, (n = 34) were classified as good quality, (n = 9) were classified as fair quality, and (n = 37) were classified as low quality (Fig. 2). The explanation behind this finding is that all studies across the six domains have a high frequency of unclear risk levels. The reviewed studies confirmed that EEG indices are sensitive to fluctuations during physical activity. The articles in the current review have been categorized into (1) physical activity experiments only, and (2) physical activity experiments with mental components. Generally, 64 (80%) of the reviewed articles investigated brain activity during physical activity only, whereas 17 articles (20%) reported on the combined physical and mental activities.
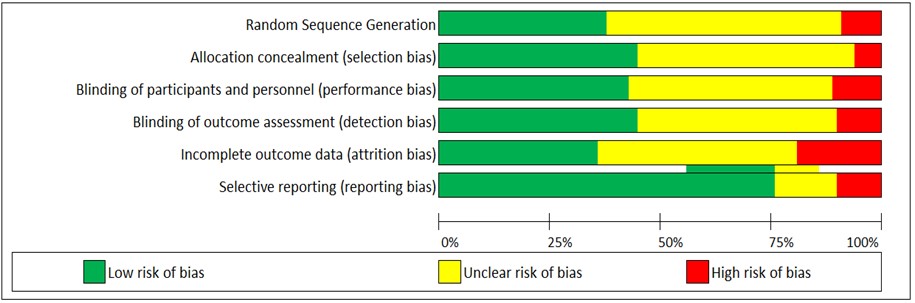
Assessment of risk of bias using the Cochrane collaboration’s tool.
The experimental studies have been categorized into physical tasks only, and studies on physical and mental tasks. Physical tasks were categorized into upper body, lower body, upper and lower body, and stressful and emotional exhaustion. The studies of physical tasks with mental components were grouped to analyze (1) the effect of the physical and mental activity on EEG, and (2) the effect of the physical activity on cognitive processes (Fig. 3).
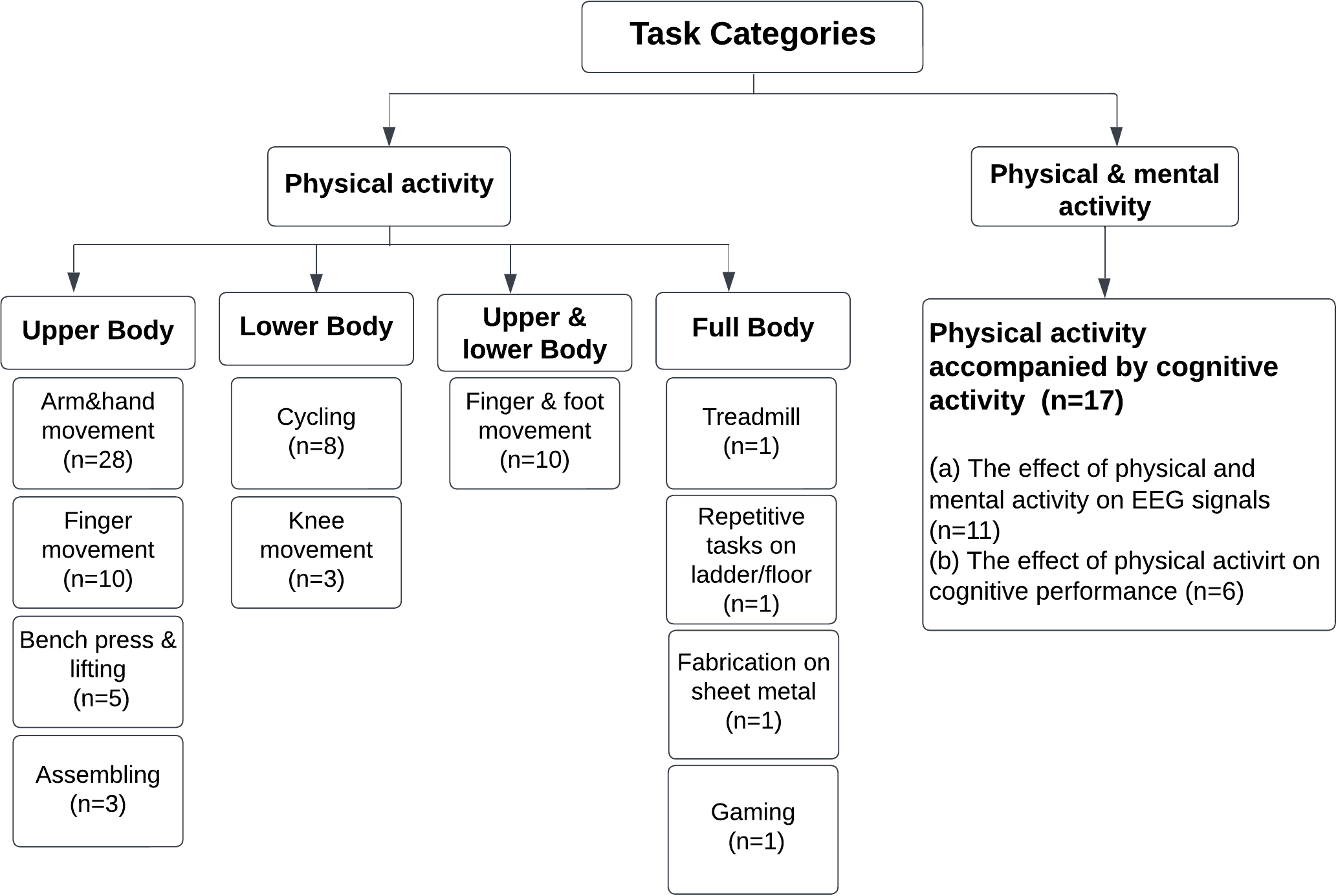
Task Categorization of the reviewed article’s tasks.
The taxonomy of different domains in physical activities (Fig. 4) includes physical fatigue task (n = 22), followed by observation, preparation, and execution (n = 14), workload (n = 7), force and torque (n = 6), stressful and emotional exhaustion (n = 6), perception of effort (n = 5), motor training and learning (n = 4), and perception of risk (n = 1), addressing research question 1.
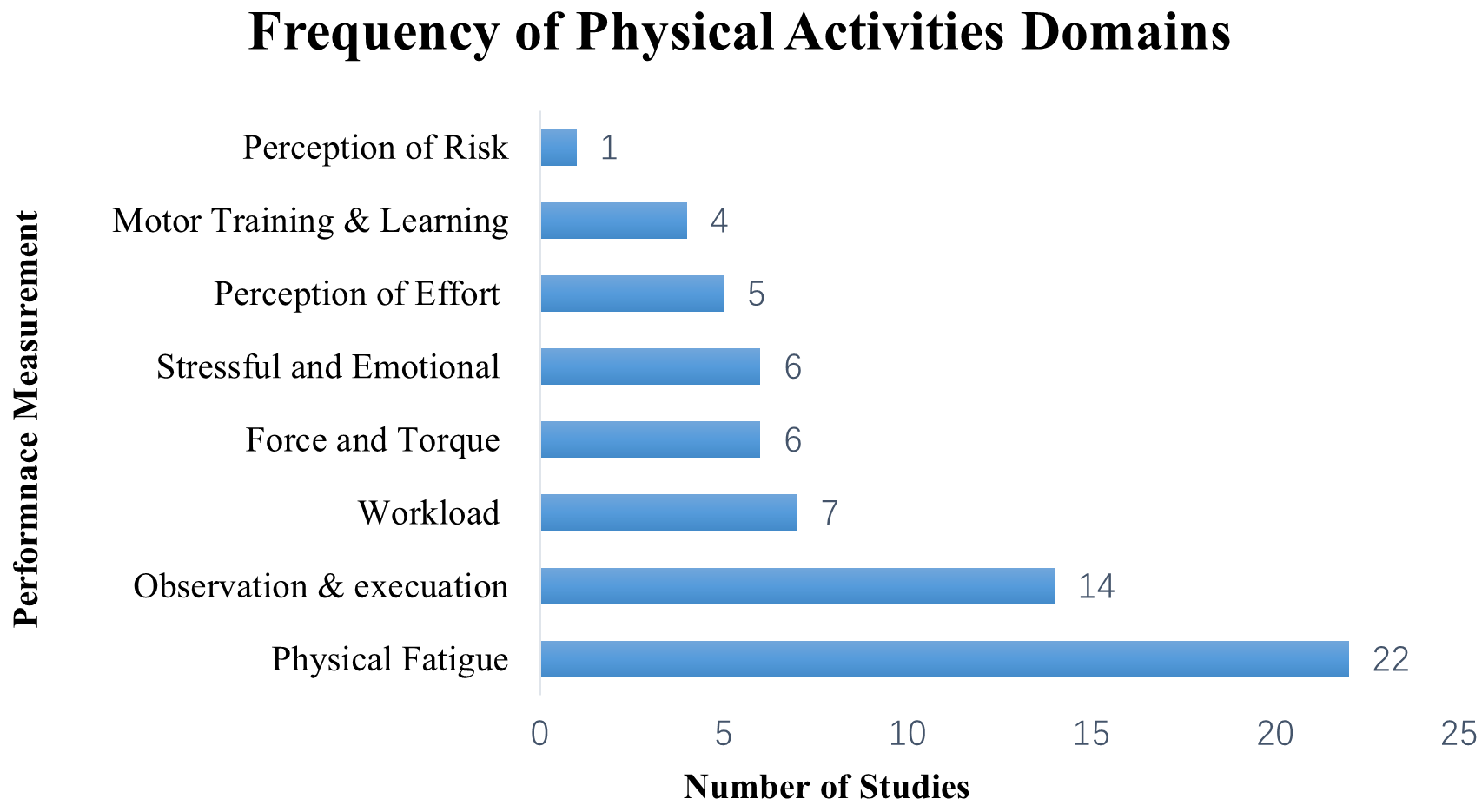
Taxonomy of different domains in physical activities.
Generally, 68% of the reviewed articles used a traditional linear analysis approach to analyze EEG signals in physical activity studies. Numerous studies (n = 43) have applied the power of frequency methods including power spectrum density (PSD), event-related desynchronization and synchronization (ERD/ERS), the ratio of powers, and peak alpha frequency (PAF), Root Mean Square (RMS), and the ratio of powers (Fig. 5), followed by the event-related potentials (ERP) components (n = 25). Some studies have combined the frequency of powers with the ERp components (n = 7). Few studies combined both linear and nonlinear methods (n = 3). The synchronization between the connectivity of the pairs EEG electrodes was discussed in two studies. However, the nonlinear methods such as fractural dimension (FD) and largest lyapunov exponents (L1) have been used in few studies. EEG coherence was found in only one study, addressing research question 2.
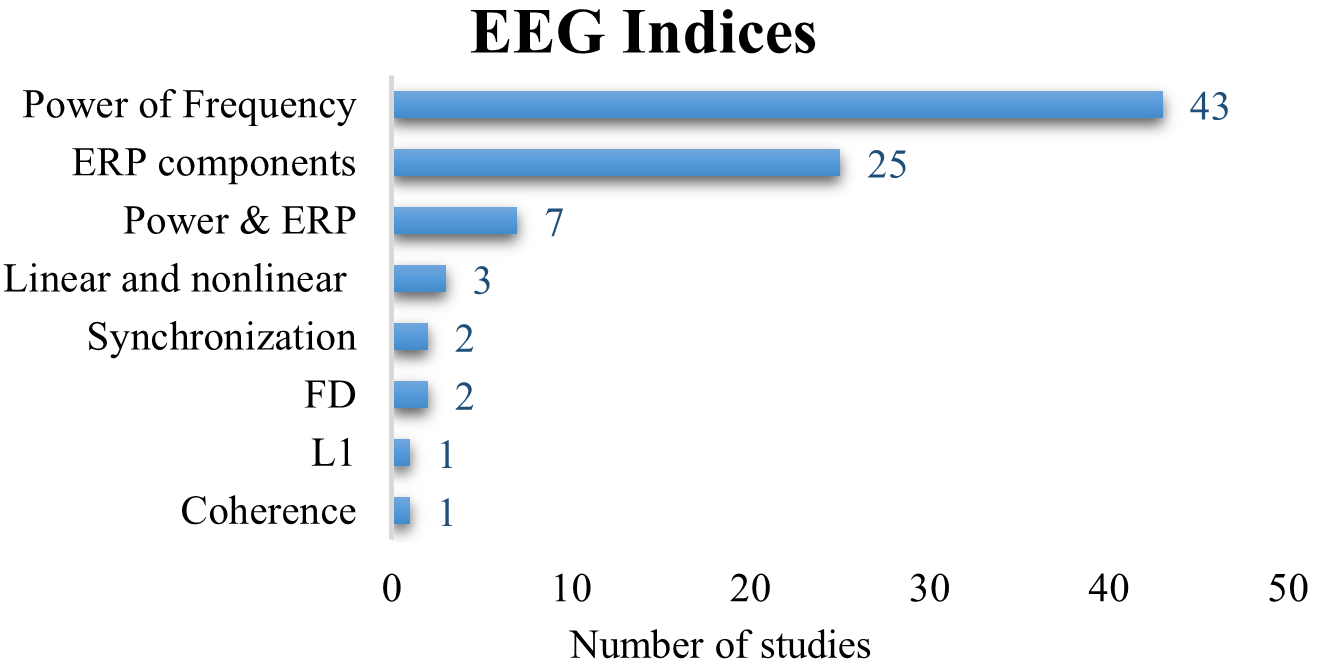
The frequency of the used EEG indices (event-related potential [ERP], fractural dimension [FD], largest lyapunov exponents [L1]).
The aspects of EEG measurement relevant to physical activities include the methods of feature extraction, the number of channels, the number of participants and their gender, and methods of artifact removal that have been addressed, this section addressing research question 3.
Since PSD is the most used EEG analysis method, the Fourier transform has been widely used for feature extraction from the power spectrum.
A critical aspect of any EEG study is the selection of the number of recording
electrodes. Two recommendations were made in the literature. The first is to
reduce the number of electrodes (i.e.,
The demographic distribution of the studies included healthy male and female participants. Of these, 27 studies engaged males, and three studies employed females, and 51 reported participation of subjects of both genders. The dimensions of the experimental samples are listed in Supplementary Material C. Most reviewed studies had a higher number of male participants than females (n = 25).
The raw EEG signals in physical tasks can be contaminated with artifacts due to body movements, including the neck and muscle activity. Therefore, several artifact removal methods have been developed and applied including (1) visual checking (n = 48); (2) filters such as bandpass filters, low pass filter, high pass filter, notch filter, spatial filters, Butterworth filter, moving average filters, infinite impulse response filter (FIR), and infinite impulse response (IIR) (n = 44); (3) bad channel rejection (n = 38); (4) power line removal (n = 12); (5) regression methods (n = 3); (6) blind source separation including independent component analysis (ICA) and principal component analysis (PCA) (n = 35); (6) artifact subspace reconstruction method (ASR) (n = 4); and (7) automatic artifacts rejections software including EEGLAB [51], NeuroScan’s software [52], and Fieldtrip [53]. Visual checking and a bandpass filter were the most frequently used methods.
This section discusses the effect of the following eight application domains including (1) physical or muscular fatigue, (2) movement observation, planning, and execution, (3) biomechanical properties (e.g., force, torque), (4) stressful and emotional exhaustion, (5) physical workload, (6) perception of effort, (7) motor training and learning, (8) strength capability. We focus on the EEG analysis methods used to characterize human performance in physical work. These methods are categorized into the time domain, frequency domain, time-frequency domain, and nonlinear methods. The Time-domain analysis methods include EEG components analysis known as event-related potential (ERP); the frequency domain method is known as spectral analysis, including power spectrum density (PSD), event-related desynchronization and synchronization (ERD/ERS), the ratio of powers, and peak alpha frequency (PAF); the time-frequency domain methods include the wavelet transform and Hilbert-Huang transform. Finally, nonlinear methods which are suitable to analyze the non-stationary, dynamic, and nonlinear EEG time series [54], including, for example, assessing the level of chaos in time-series data by using the concepts of entropy, fractural dimension (FD), largest Lyapunov exponents (L1), or Lempel-Ziv complexity (LZC) [55].
Fatigue is a multidimensional concept that combines psychological and physiological aspects and results in vigilance deterioration, reduces the wellness to exert effort, and declines in physiological capabilities [56, 57]. Fatigue is categorized into physical, muscular, mental, and visual fatigue. Physical fatigue is a temporary inability of the muscle to perform the optimal work or expected force [58]. The notion of physical fatigue is much broader than muscular fatigue. The term physical fatigue is more complex than muscular fatigue since it includes the interactions between muscular and other several central factors [59]. During a physical task, not only the human muscles become fatigued, but also the central nervous system is affected. As defined by Zadry et al. [60], “If the muscles begin to fatigue, the brain also begins to fatigue”. Liu et al. [61] demonstrated that the human brain avoids fatigue by shifting the brain activities toward the right anterior and inferior hemispheres, which means the brain requires more resources to complete the task when fatigue occurs. Accordingly, understanding the neuromuscular fatigue by analyzing the coherence between EEG signals (i.e., brain) and electromyography (EMG) signal (i.e., muscles) [62, 63, 64, 65] became an exciting area of neuroergonomics research.
It was reported in the literature that after completion of the physically demanding and fatiguing task, the PSD of theta activity in the posterior brain region has increased, which is an interesting phenomenon since mental fatigue has been also associated with an increase in the PSD of theta activity in the frontal brain region [66, 67]. A study by Ng and Raveendran et al. [68] found an increase in the PSD of lower alpha and beta activity at the left motor cortex, whereas delta activity did not show any significant change. They concluded that beta activity is associated with motor control. Furthermore, Baumeister et al. [69] reported a reduction in the lower and upper alpha activity in the frontal cortex of the brain during a knee joint reproduction task which might be an indication to the existence of physical fatigue.
Physical fatigue has also been assessed by the ratio of EEG power. Aryal
et al. [59] reported an increase in the ratio of the power of
(
The PSD of EEG frequencies have been applied as an input parameter for estimating physical fatigue. For example, Jain et al. [73] used the PSD of delta, theta, alpha, and beta activity for detecting muscular fatigue with the help of an Auto-Regression (AR) model. Abdul-latif et al. [70] combined the EEG ratio index along with both the heart rate and skin temperature data and used these as an input parameter to the Boosted tree, decision trees, and Support Vector Machine (SVM) with kernel function algorithm. It has been suggested that the above knowledge will help in the development of the smart warning alarm systems that can prevent the occurrence of physical fatigue by monitoring changes in the task error rates [73], or task precision [69]. Another exciting study proved that hyperthermia could be an independent cause of brain fatigue but not muscle fatigue during a cycling task [74]. Thus, high body temperature inhabits the adequate neural drive to the muscles. A linear increase in the alpha/beta index over the frontal, motor, visual cortex was reported under the condition of hyperthermia (40 °C) compared to normal temperature levels (18 °C). Whereas the root mean square, amplitude, and median spectral frequency of EMG did not change, the index of PSD alpha/beta reflected the sensation of the core temperature [74, 75]. A similar study investigated a decline in beta activity and a higher level of alpha/beta index in the frontal cortex during hyperthermia (42 °C), demonstrating a reduction of arousal level in the frontal cortex due to exercise in relation to the core temperature (i.e., cognitive fatigue) [76]. Périard et al. [77] found that an increase in alpha and beta activity is localized in the brain primary somatosensory and motor cortices during exercise under the controlled and hypoxic conditions, and increase in beta activity due to hot temperature. The connectivity between EEG electrode pairs has also been analyzed after computing the spectrum power of EEG [78] and it was shown that the muscle fatigue strengthens the functional connectivity in the left motor cortex to maintain the same level of force.
The motor-related cortical potential (MRCP) is an ERP component that is locked to the initiation of movement [79]. MRCP has been extensively used to reflect the magnitude of the neural activity before and after physical task by using three components: (1) Bereitschaftspotential (BP) or readiness potential (RP), which is a slow negative segment that occurs before the movement within 2 seconds in the pre-supplementary motor area [80]; (2) Motor potential (MP), which is a negative potential following the RP approximately 150 ms before voluntary movement; (3) Movement Monitoring Potential (MMP), which is a complex negative-positive potential following the onset of a given voluntary motor [81]. The RP, MP, and MMP potentials are associated with movement planning or preparation, movement execution, and performance control, respectively [81, 82].
An increase in the RP values at the supplementary motor area (SMA) (Cz, C3, and C4 electrodes) was found with a small level of physiological fatigue during the exertion of highly repetitive forces on the construction tasks [83]. Furthermore, when fatigue occurred during the grasping task, an increase in the MP amplitude at precentral and contralateral, and an increase in BP amplitude at precentral were also observed [84]. The above studies indicate that the brain accomplishes the required level of contraction force by increasing the cortical motor activity over the supplementary motor and contralateral sensorimotor areas. In a simple repetitive unilateral button press task, a negative amplitude of post-movement potential PMP over the supplementary motor and contralateral sensorimotor areas was reported with the onset of muscular fatigue [85]. However, different changes in the MRCP were observed in a large group of muscles on different tasks, leading to the conclusion that one should analyze the similar levels of forces exerted by the same muscle groups when comparing related neurophysiological brain responses [86].
Combining the PSD and MRCP to compare fatigue between the sustained and preparation phases of maximal voluntary contraction (MVC) showed no change for PSD in the preparation phase, but a significant reduction in the sustained phase of the contraction force. It was also demonstrated that MRCP-negative potential (NP) slightly changes in the preparation phase [87] above the left sensorimotor brain area [61].
For nonlinear method indices, a higher value of FD was reported during a fatigued handgrip contraction task comparing to the resting state [88], whereas L1 reduced with fatigue [89]. Few studies have combined the PSD frequency along with non-linear methods to assess the effect of physical fatigue states. The PSD of alpha, beta, and gamma, along with the sample measures of entropy, were used with and without a magnetic stimulation to study the effect of physical fatigue and the impact of different force levels [42]. Muscular fatigue was also accompanied by an increase in the alpha activity in C3, and C4 brain areas, with no changes in the PSD of the beta and gamma signal.
Muscular fatigue can be defined as the temporary inability of the muscle to reach the MVC [90]. The PSD of gamma (in C3, C4, Cz, Pz, and Fz), and beta (for C3) were significantly higher in 50% MVC,75% MVC than in 25% MVC during the fatigued state [91]. A direct relationship between the force exerted and the amplitude of MRCP exists [92]. For instance, as the force levels increase, the Bp during a repetitive hand contraction task increases [93] Also, the negative slope of MRCP is highly correlated with joint forces [94]. Moreover, the amplitude of the RP increases when both the force production and the rate of force development torque increase [81]. This means that the more force is required, the more neurons are being recruited by the brain. However, during a finger movement task, when the force level increases, the amplitude of MRCP components, mainly BP and MP, decreases [95]. Finally, Schillings et al. [83] found no significant changes in RP signal due to the repetitive forceful grip contraction task.
Negative emotions that occur in the workplace can affect human performance and increase the probability of errors and injuries. A considerable number of studies have addressed the psychosocial problems, including emotional exhaustion, stress, and burnout at work using subjective measurements, physical and biometrics measurements such as EMG, skin temperature, electrodermal activity, heart rate variability, or the variation between heartbeats. However, studying brain signals might provide rich information on quantifying human psychosocial conditions [96]. The frontal lobe is known as the emotion control center [97]. However, recent studies found evidence of the activation of the motor cortex area under stressful working conditions [96, 98]. After performing the stressful physical work, the PSD of the beta band in the right hemisphere was higher than that in the left hemisphere [99]. Other investigations of stressful occupation conditions have been conducted to determine the worker arousal and valence levels. The authors reported different working conditions with distinct hazard levels, for which they developed mathematical equations based on the PSD of the alpha and beta ratio to quantify the arousal and valence levels. The results revealed that workers’ emotions were negatively influenced by a poor working environment [100]. Time pressure, defined as “the difference between the amount of available time and the amount of time required to solve a task” [101], is another significant factor that affects human performance. During a time pressure visuomotor task, an increase in the frontal midline theta activity and gamma activity in different regions was found [101]. The RMS of the alpha band increased with the time-stress of the task [102]. The results also demonstrated the high occurrence of the errors in time pressure conditions, indicating mental fatigue associated with time stress. This research area will open new insights into workplace design by avoiding negative emotions and hazardous environments to maintain workers’ well-being.
The brain is activated before the task execution, a time where there is no muscle movement [103]. Still, the person remains aware of what is going to be performed in the future (i.e., task observation) [82]. The task observation is activated by the mirror neurons [104], mainly in the motor cortex and the posterior frontal cortex [105]. An essential and relevant parameter in this respect is derived from EEG signal power is an ERD/ERS. A reduction in power is called event-related desynchronization (ERD), while its increase is referred to as event-related synchronization (ERS) [106]. During the motor task observation, imagery, and execution, the ERD/ERS can be found in alpha, mu, and beta activity [107]. It should be noted that the alpha ERD is non-task specific, while the upper alpha ERD is a task-specific [108]. A similar phenomenon has been observed in beta ERD [109]. The mu rhythm, which was first introduced by Gastaut et al. [110], falls between 8 and 12 Hz over the motor cortex, mainly in Cz, C3, and C4 EEG electrodes. The mu has been extensively used during both the task observation and execution [111] since it is suppressed during and after motor action [110]. A reduction in the amplitude of mu rhythm was observed during the observation of a precision grip contrasted to a simple hand extension [112]. The reduction of mu rhythm reflects desynchronization, indicating that the brain became more active, processing the observation of precision grip task. Furthermore, Babiloni et al. [113] and Muthukumaraswamy et al. [112] found an increase in the alpha ERD in the primary sensorimotor during the preparation and execution of movement tasks. The alpha ERD showed an asymmetric pattern in the preparation of finger and foot movement [114]. However, different behaviors of lower and upper alpha ERD/ERS was found after performing the movement task. Calmels et al. [115] found a higher power of ERD in alpha and beta for the pre-movement than in post-movement. Zaepffel et al. [116] detected an increase in beta ERD during a cueing task, followed by a reduction in beta ERD during movement preparation and execution. Finally, Nakayashiki et al. [107] found that the mu and beta ERD is insensitive to kinetics conditions in an isometric contraction hand grasping task, but they appear sensitive to the kinematics conditions. Cochin [105] demonstrated a reduction in alpha PSD during the observation and execution of finger movements. Significant changes in PSD were found over the right sensorimotor regions of the brain during the left arm movement. In contrast, dominant changes in the PSD over the left sensorimotor regions were obtained during the right arm movement [117]. Furthermore, ERD for alpha activity was slightly higher over the contralateral central brain regions and, secondarily, the ipsilateral motor and mesial regions compared to ERD for the beta activity. Overall, the spectral coherence for both alpha and beta signals was reduced during arm movement and during the execution of grasping and reaching [118]. Similarly, Fallani et al. [119] found an increase in the alpha partially-directed coherence during the movement preparation, which implies a higher exchange of information in the cortical brain regions of interest when performing the subsequent movements. Different ERD patterns appeared during motor imagery and execution. Less significant mu ERD was found at contralateral during motor execution compared to ipsilateral movements [120]. The MRCP and mu ERD reflect different aspects of sensorimotor cortical processes [113]. In particular, alpha ERD reflects changes in the cortical sensorimotor areas, whereas MRPs increased in task supplementary motor area and contralateral primary sensorimotor. However, negative potential MRCP in motor preparation tasks demonstrated minimal changes [87].
A study revealed that FDs of EEG signals increased linearly with handgrip force during the holding and the movement, with no significant change nor correlation during the preparation period of the movement task [121]. Yang et al. [122] investigated the EEG time-dependent EEG source strength during the preparation, execution, and sustaining phases of isometric hand exertions. Results demonstrated a reduction in the nonlinear source strength during the sustaining phase but an increase during the preparation phase. Similarly, there was an increase in the alpha partial directed coherence during the movement preparation, which reflects the higher exchange of information for movement execution [47]. A Bayesian model was applied to investigate the effect of the coupling strength during motor execution and motor imagery. A higher strength coupling was found between the dorsolateral prefrontal cortex to the pre-motor cortex during motor execution than during motor imagery. However, the coupling strength of the pre-motor cortex to the supplementary motor area and the primary motor cortex to the pre-motor cortex was higher in the motor imagery than in motor execution [120].
The rate of perceived exertion (the perception of effort) is defined as “the conscious awareness of the central motor command sent to the active muscles” [123]. Guoa et al. [124] demonstrated that the amplitude of MRCPs and the subjective ratings increase with the increase of muscle fatigue in the primary motor area and prefrontal cortex of the brain. Slobounov et al. [125] found an increase in MRCP in frontal, central, and parietal cortical areas associated with the development rate of force. Furthermore, they found that the amplitude of the early MRCP component increased with the perception of effort, whereas the MMP increased with force level. De Moree et al. [123] showed a significant correlation between the amplitude of MRCP and the perception of effort. Two years later, the authors studied the effect of caffeine intake and time spent on the task over the perception of effort [126]. Results revealed a reduction in MRCP amplitude and the Borg Rating of Perceived Exertion after caffeine intake in the premotor and motor cortex, whereas the time spent on task was linked to an increment in the amplitude of MRCP. Nybo and Nielsen [74] found that the frontal cortex, mainly the values of PSD of alpha/beta in the F3 location, are the best predictors of the rate of perceived exertion. Therefore, since the perceived exertion is associated with cortical activity, new perspectives have opened for developing rehabilitation technologies and progress in BCI. A study by Comani et al. [127] found a predominant frontal-motor coupling in the alpha band and a frontal-occipital coupling in the beta band during a cycling task.
Generally, human performance can be improved through practice and training. Evaluating motor learning based on neural changes has been a challenging area for sports medication, rehabilitation, and kinematic prediction in the neuroergonomics area [128]. Practice reduces the theta ERS in the frontal area, indicating the deterioration in the attention after training. This reflects the easiness of the task after training [129]. Therefore, it has been suggested to use the alpha ERD neurofeedback to train the frontal alpha rhythms to produce a strong ERD for better human performance [130]. The MRCP, ERD, ERS were found to change with motor training. For instance, Jochumsen et al. [131] compared the MRCP, ERD/ERS for an alpha, mu, and beta activity between single and multiple training sessions. An increase in the amplitude of MRCP after a single training session was observed, while a reduction was found after multiple training sessions. The ERD/ERS for only the beta band showed a significant increase after the single training only. The amplitude and latency of the ERP increase after training in the premotor cortex [132], indicating that the motor cortex (neuron network) changes after training and practice.
Finally, the Gamma Band Activity (GBA) was used to quantify the EEG signals in the motor cortex after a hand movement training task [133]. Results showed a significant increase in GBA after performing the hand moving task. These findings demonstrate that the EEG indices are useful for monitoring the cortical changes in the motor learning processes.
Aljuaid and Karwowski [134] investigated the neural signatures of manual material lifting tasks, including the relationship between the maximum acceptable weight of lift and the EEG signals. Significant differences in PSD under different lifting frequencies were observed, mainly in the frontal, central, and parietal brain regions. The EEG signals during the isokinetic and isometric strength tests for both arms and legs were also studied. The observed levels of PSD of alpha, beta, and gamma for isometric arm strength were significantly lower than those in the isometric leg strength test in the frontal, central, and partial brain regions.
The human workload is defined as the ratio between human capacity and task demand [135]. It is a multidimensional concept reflecting human mental ability, physical limitations, task difficulty, task engagement, and effort [136]. The workload can be classified into mental workload (i.e., monitoring, attention, and decision-making) and physical workload (i.e., pushing, carrying, lifting, and handling). Despite wide-spread automation, many tasks performed in the manufacturing sector, such as assembly-line activities and the hospitality industry, require significant physical effort. Many EEG studies have demonstrated that a high level of workload activates more brain regions than low workload [137]. For instance, in a light assembly task, the PSD of alpha is higher at a low workload task than at a high workload task [138]. The PSD levels of alpha in the Fz-Pz channel are higher at a low workload than at a high workload. The PSD of alpha for the O1-O2 channel is lower at low workload than the high workload [60]. Other studies have found a positive correlation between the normalized PSD of EEG and workload [139, 140]. Engchuan et al. [141] reported an increase in the PSD of beta and gamma signals in a weight pressing task. Furthermore, the PSD of beta activity increased with the exercise duration, while the PSD of alpha decreased [142]. Different task difficulty levels activation different topological brain regions. In a simple hand movement task, changes were found in sensorimotor areas by a reduction in the PSD of alpha and beta. In a complex hand movement task, changes were observed in the right frontal, prefrontal, posterior parietal, and left temporal areas [143]. Enders et al. [144] reported a significant increase in the PSD of EEG in the frontal area of the cortex during physical exercise and demonstrated the connectivity between parietal areas and motor areas during exercise. The amplitude of the ERP component, namely, P300, increases with the exercise frequency [145]. The location of changes in the cortical activities before, during, and after a physical task has also been an area of considerable interest. A study of EEG density using low-resolution electromagnetic tomography (LORETA) demonstrated that the motor cortex activity is elevated with high levels of physical effort intensity [146]. Porter et al. [147] reported an increase in the PSD of theta activity of the cortical region when combining both physical and mental exertion tasks.
As stated by Mehta [11], “The human action is orchestrated by the mind (brain) and body interactions”. In naturalistic work conditions, several cognitive skills such as attention, decision making, perception, and working memory, as well as physical abilities, are required to perform a task. For assessing human performance at work, both physical and cognitive behavior must be considered [148]. High cognitive demand influences the physical work, and vice versa [149]. Our literature search discovered 16 studies that reported EEG activity during the combined physical and cognitive work process components.
Smith et al. [150] have addressed the effect of both the mental and physical efforts on the attention level. After the mental effort, an increase in the PSD of theta was found. Additionally, they reported an increase in PSD for alpha and lower beta after the physical effort. The study concluded that mental effort deteriorates the alertness level, while physical effort increases the need for attention. According to Jagannath and Balasubramanian [151], an increase in the PSD of theta, alpha, and the ratio of (alpha+theta)/beta activity, with a reduction in beta activity, are signs of the attention deterioration due to physical and mental fatigue during a driving task. Another study analyzed the attention levels of human operators while handling boxes and solving cognitive riddles [67]. The PSD of theta and alpha activity was used to quantify the cognitive state, while the amplitude of the P300 component was used to characterize the physical tasks. The results demonstrated a dominant increase in the PSD of theta and alpha activity and the amplitude of N2 during a cognitive task, whereas the amplitude of P300 was reduced during the physical task. Two years later, the same authors replaced the solving of cognitive riddles task with the Simon task [152]. Results revealed an increase in the PSD of alpha activity with time spent on a task, reflecting an increase in mental fatigue and motivation reduction.
Mijović et al. [153] compared two different age groups of participants in a manual assembly task to analyze the effect of age on attention performance. They found a lower level of PSD alpha in the older group than the young group, indicating that mental fatigue is more pronounced at an older age. Since workers’ attention can be enhanced by providing instructions, Zink et al. [154] experimented with a ‘go’ or ‘not to go’ conditions. They reported a reduction in the amplitude of P300 during the ‘go’ condition, which demonstrates an increase in the cognitive load due to movement, and the deterioration of attention. This phenomenon was also confirmed by Yagi et al. [155]. However, Mijović et al. [153] reported the contrary results. Porter et al. [147] found an increase in cortical activity when combining both physical and mental exertion tasks. An increase in the PSD of theta activity and a partial correlation were found in the frontal brain region. Similarly, Shaw et al. [156] observed changes in the mental workload during dual-task walking. Finally, Sengupta et al. [57, 157] studied the synchronization between different brain regions during physical, mental, and visual fatiguing tasks using the spectrum power of EEG. They reported an increase in the brain horizontal visibility graph-based synchronization in the electrode pairs related to parietal and occipital brain areas under the condition existence fatigue. Effect of physical activity on cognitive processes.
To assess the mental workload, Albuquerque et al. [158] investigated different physical activities using three EEG indices, namely, the PSD for nine frequency bands, the amplitude modulation rate of change, and phase coherence. During a medium level of physical activity, the amplitude modulation features become more important in the parietal brain region, while in the high physical activity, the magnitude coherence becomes more important in other brain regions. A significant difference between high and low mental workload states across the three levels of physical activity was found from the EEG features. These results demonstrated that the increase in the intensity of the physical activity elicits more mental resources, increasing the individual’s drowsiness.
In a manufacturing processes task, Ma et al. [148] have monitored the
operator’s mental and physical workload during a production line operation.
Comparing an improved and non-improved workstation designs, the EEG features
mainly PSD of theta, and sensory-motor response (12–15 Hz) showed higher values
in the non-improved design indicating the presence of mental workload. Another
study by Xu et al. [159] has addressed the effect of physical activity
on mental fatigue. The spectral coherence value (SCV), Lempel-Ziv complexity
(LZC), and wavelet packet energy (WPE) were used to characterize the mental
fatigue during a cycling task. SCV was used to estimate the functional
connectivity between two pairs of EEG electrodes. LZC was used to detect the
complexity of EEG signals, whereas WPE was used to decompose the EEG power into
frequency bands. The study revealed that physical activity increases the mental
fatigue by (a) a reduction in the relative energy in beta (E
Exercise intensity significantly affects the attention level. The amplitude of contingent negative variation (CNV) decreased after high-intensity tasks compared to medium intensity, whereas the relative power of theta activity increased after the high-intensity exercise compared to medium intensity [161]. Furthermore, exercise intensity significantly alters information processing in the brain A reduction in the amplitude of P300 was observed after high-intensity physical tasks compared to medium intensity tasks. An inverted-U shape was also proposed to model the interaction between vigilance and the amplitude of CNV. A similar trend has been observed for the relationship between the amplitude of the P300 signal and exercise intensity [162]. A new perspective to understand the perception of risk using a new hybrid kinematic-EEG method has been proposed by Wang et al. [163] during the execution of construction tasks.
This section is addressing research question 4, the current review includes studies with randomized controlled trials. However, studies considering non-randomized controlled trials were not considered included in the current review. We encourage future systematic reviews to consider both randomized controlled trials and non-randomized controlled trials to assess the methodological quality.
The application of EEG indices has advanced our knowledge in characterizing the brain activity relevant to human physical activity at work. Previous studies focused on traditional linear methods; for instance, PSD was highly used to characterize the effects of fatigue, while ERD/ERS was applied during planning, observation, and movement execution. Moreover, the MRCP was frequently applied in studying the biomechanical properties of the human body, such as force and torque. Since EEG data are complex and contain dynamic information from the brain, they should also be analyzed using nonlinear methods derived from nonlinear dynamical systems and chaos theory [164, 165, 166].
The number of studies on physical tasks with mental activities is significantly less than the number of studies dealing only with physical tasks. However, contemporary ergonomic assessment of the workplace requires the evaluation of physical tasks with a large variety of cognitive components. Since cognitive demands affect physical capabilities and physical demands affect cognitive processing, future research in neuroergonomics should address the interrelationship between physical activities and cognitive functions.
The recent technological innovations of EEG systems in portability, power storage, and wireless design allows experiments to be conducted in natural working environments [23]. However, our review revealed that most of the reported studies were focused on controlled laboratory experiments due to the low signal to noise ratio in controlled laboratory conditions. Few studies have investigated neural signatures under real workplace conditions, such as the construction sites [59, 98, 167] or manufacturing tasks [148]. The use of EEG outside a controlled laboratory will rely on several additional factors, such as new class of dry electrodes [168, 169, 170, 171, 172], sophisticated and intelligent algorithms for online motion artifact correction, device shielding, and integrated computational power that should be considered in future studies. Until now, significant number of studies are using conventional EEG systems with limited portability and long preparation time. Future studies should use ecologically friendly EEG systems with less preparation time, mobility, wireless, and even disposable headset to overcome the laboratory controlled conditions [173]. A number of challenges lie ahead for improving the signal to noise ratio including using active EEG electrodes [174]. Another challenge inherent to the collection of EEG data in the real-world application is a relative loss of experimental control [175].
Most of the reviewed EEG studies covered the body areas such as upper limbs, mainly finger movement, handgrip, and hand grasping tasks. Tasks that require the activity of the shoulder, such as those of overhead drilling operation or sewing machine operators, have been poorly addressed. Moreover, typical assembly and manual load handling tasks that are still commonly performed as essential components of many jobs in manufacturing, shipping, healthcare, hospitality, and other service-related industries have not been studied. A few studies dealt with lower limb activities such as cycling. The neural signatures of tasks that involve the torso, spine, and lumbar area, which are essential for the prevention of work-related musculoskeletal disorders, should also be investigated in the future studies.
EEG data are spatiotemporal with an excellent temporal resolution but poor spatial resolution. The EEG electrode reference and volume conduction significantly influence spatial resolution. Therefore, localizing the EEG source to correlate the activity of the brain regions using current source density (i.e., Laplacian ([176])) or LORETA has provided successful results in reducing the volume conduction and improving the EEG spatial resolution [177]. Selecting the best reference is still a debate [72, 178, 179]. Four studies have applied EEG source localization methods in physical tasks, including the assessment of voluntary muscle contraction tasks [122], muscle fatigue [61] , motor execution, and imaginary [120] and exercise intensity [180]. The methods for assessing the human strength capabilities and perception of physical effort using EEG data are needed.
The selection of the number of recording electrodes is an open/raises research question. Several studies characterized the EEG information from the individual electrode source point of view, neglecting the integration and segregation between EEG electrodes and their interactions. Connectivity between brain regions provides useful information regarding the functions of the human brain, an emerging field of study known as connectome [181]. Connectome studies have been widely applied in cognitive neuroscience studies but to a minimal extent in the domain of physiological neuroergonomics [47, 117, 128, 182, 183, 184, 185, 186]. One recent study has applied graph theory approach modelling the force exertion levels during a physical task [187]. Results of global network characteristics showed different network topological properties associated with different force exertion levels.
All the reviewed articles did not explicitly take into consideration the hybrid of EEG technique with other neurophysiological. Combining multiple neurophysiological techniques might provide an innovative approach that synthesize the advantages of each technique while overcomes the limitations. For instance, combining EEG and fNIRS is one of the promising methods since it improves both temporal and spatial resolution [188, 189], which is crucial in diverse of applications including BCI and motor imagery [190, 191, 192, 193], and cognitive workload [194]. Although, the integration is very promising still few researchers have explored this integration [195].
This systematic review demonstrates the use of EEG indices that are relevant and useful to the field of physical activities at both laboratory and real-world settings. Our review demonstrates that EEG indices are reliable and sensitive indicators for quantifying the neurophysiological changes associated with a variety of work-related physical activities, motor learning, and psychosocial conditions. The findings from 81 experimental studies established that EEG studies have primarily relied on linear methods mainly the power spectrum density. Consequently, the Fourier transform has been widely used as feature extraction method. Most studies focused on evaluating the brain activity associated with muscular fatigue task using few EEG channels. The upper anatomical body areas have been addressed in most of the reviewed articles whereas the torso, spine, and lumber, which are the risk factors for musculoskeletal disorders are less addressed. Mapping brain patterns during physical activities is an open challenge to understand the role of functional brain networks at work. Soon, the application of advanced mathematical algorithms to analyze EEG data should help develop adaptive systems that are capable of monitoring the human physical states to prevent fatigue and excessive physical workload. Furthermore, detective systems and prediction models to monitor the learning process should help estimate the outcomes that might promote and facilitate the training and learning processes at work and everyday activities.
LI conducted the literature search and prepared the initial draft of the paper. LI and WK have contributed to the conceptualizing and designing the study, data extraction, and study selection. PAH, RT, and RFS contributed to the statistical analysis, interpretation, and discussion of the data. WK supervised all aspects of manuscript preparation, revision, editing, and final intellectual content. All authors contributed to editorial changes in the manuscript. All authors read and approved the final manuscript. All authors have participated sufficiently in work and agreed to be accountable for all aspects of the work.
Not applicable.
We thank to all the peer reviewers for their opinions and suggestions.
This research received no external funding.
The authors declare no conflict of interest.
Publisher’s Note: IMR Press stays neutral with regard to jurisdictional claims in published maps and institutional affiliations.