†These authors contributed equally.
Academic Editor: Hsien-Yuan Lane
This is an open access article under the CC BY 4.0 license.
As a non-invasive detection method and an advanced imaging method, magnetic resonance imaging (MRI) has been widely used in the research of schizophrenia. Although a large number of neuroimaging studies have confirmed that MRI can display abnormal brain phenotypes in patients with schizophrenia, no valid uniform standard has been established for its clinical application. On the basis of previous evidence, we argue that MRI is an important tool throughout the whole clinical course of schizophrenia. The purpose of this commentary is to systematically describe the role of MRI in schizophrenia and to provide references for its clinical application.
Magnetic resonance imaging (MRI), a non-invasive and advanced imaging method, has been widely applied in schizophrenia research since 1984 [1]. MRI is able to provide multi-parameter and multi-level information about brain anatomy, function, and metabolism [2]. Recently, with the developments of resting-state functional MRI (fMRI), structural magnetic resonance imaging (sMRI), diffusion weighted imaging (DWI), magnetic resonance spectroscopy (MRS), and other sequences, alterations of brain structure and function could be displayed more comprehensively and accurately. An increasing body of evidence suggests that MRI has the ability to display both structural and functional brain abnormalities in patients with schizophrenia [2, 3, 4]. Patients with schizophrenia exhibit widespread thinner cortical thickness and smaller subcortical volumes as compared with healthy controls as well as increased heterogeneity in frontotemporal thickness and hippocampal volumes [5]. A review associated with structural brain changes in schizophrenia summarizes conclusions from previous longitudinal MRI studies demonstrating that the cortical gray matter exhibit progressive thinning, especially in the superior and inferior frontal regions [6]. Brain structural and functional network connectivity disturbances in patients with schizophrenia are highly concentrated in hub regions, especially the frontal lobe [7]. The overlap between structural changes and functional abnormalities in drug-free patients was systematically summarized through 15 sMRI studies and 16 fMRI studies, primarily in areas related to default mode and auditory networks, including frontotemporal regions, bilateral medial posterior cingulate/paracingulate gyrus, bilateral insula, basal ganglia and left cerebellum [8].
Although such important neuroimaging studies have been conducted, the clinical application of MRI in schizophrenia has not yet formed an effective and unified standard and lacks significant consensus. Therefore, the present commentary is being conducted to further our understanding of the utility of MRI throughout the whole clinical process and to provide a reference for the application of MRI in schizophrenia. We argue that MRI is a powerful tool for monitoring the clinical course of schizophrenia with several important applications (Fig. 1): predicting transition from clinical high risk to schizophrenia, providing evidence of macro-scale neural mechanisms, delving into the nature of symptoms, facilitating diagnosis and subtyping, predicting treatment response, detecting psychopharmacological effects, and guiding neuro-navigation of neuro-stimulation, thereby managing very-late-onset schizophrenia-like psychosis.
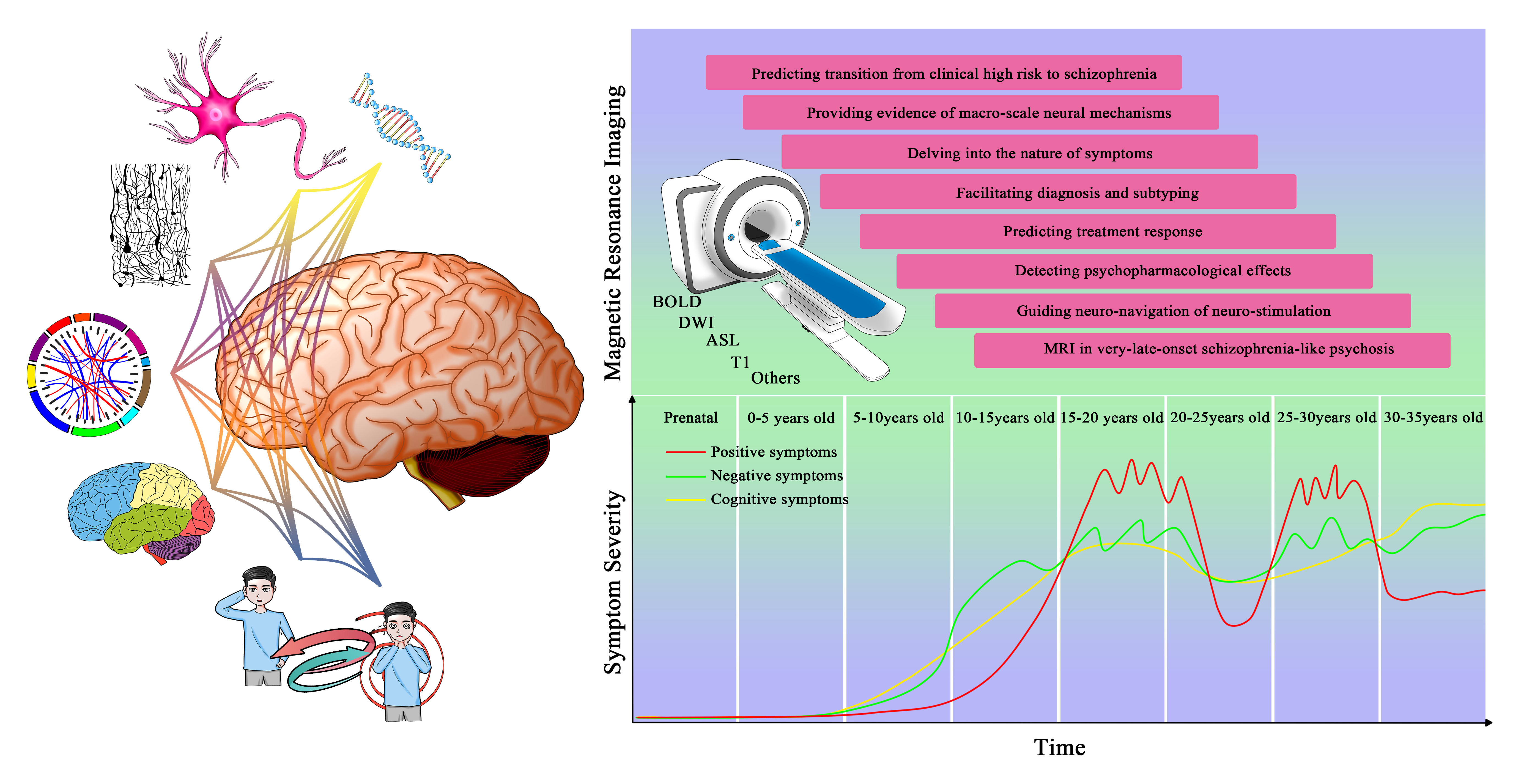
Magnetic resonance imaging throughout the clinical course of schizophrenia.
The clinical high risk (CHR) paradigm is a framework used to study potential mechanisms of psychiatric susceptibility [9]. Effective identification of patients at CHR status [10] can promote early intervention and reduce the transition to schizophrenia. MRI plays a crucial role in the discrimination of individuals at CHR by revealing baseline brain differences associated with the future transition to schizophrenia.
Resting state arterial spin labeling (ASL) scans [10] revealed a higher level of cerebral blood flow in regions within the default mode network in first-degree relatives of schizophrenia versus controls. Their cerebral blood flow in the left middle temporal gyrus was higher than that of the schizophrenia group. Both patients and their relatives exhibited dysconnectivity patterns. In an fMRI study, first-degree relatives revealed abnormal connectivity primarily localized to the connections from the bilateral anterior cingulate cortex (ACC) to the right hippocampus compared with healthy controls [11]. Using multimodal fMRI and diffusion tensor imaging (DTI) scanning, it was suggested that both schizophrenia and relatives showed aberrant functional connectivity of the ventral corticostriatal circuitry. Relatives showed reduced connectivity between the striatum, the right ACC, and the left precuneus. Structural abnormalities were only detected in schizophrenia [12]. Longitudinally, a higher rate of prefrontal cortical thinning was observed in youth at CHR who transitioned to schizophrenia [13]. A sMRI study included 1792 individuals at CHR and 1377 healthy controls demonstrated that widespread regional lower cortical thickness may serve as a biomarker for identifying individuals at CHR [9]. More longitudinal studies with a larger sample size are warranted to prove previous findings in future studies.
Over the past few years, advances in MRI techniques have increasingly improved our ability to detect brain structural and functional deficits associated with schizophrenia at macro-scale levels [7]. The relationship between whole-brain network level structural connectivity alterations and micro-structural abnormalities was demonstrated by extracting magnetization transfer ratio from magnetization transfer imaging data and reconstructing macro-scale connectivity from DWI data [14]. Reconstruction of macro-scale brain connectome in first-episode schizophrenia patients by combining DWI and fMRI data revealed that connectome rich club structures exhibited significant impairment in medication-naïve patients, which provides further evidence that brain connectivity disruption may play an important role in the early stage of schizophrenia pathophysiology [15]. A recent fMRI study combining connectome gradient and stepwise connectivity analysis demonstrated abnormal compression of sensorimotor-to-transmoda cortical hierarchy organization in schizophrenia, providing new insight into the pathological relationship between early sensory and cognitive impairment [16].
Due to the continuous progress of MRI-related technology, the multi-level research on MRI has become more specific and comprehensive. All in all, future MRI-related neural studies will provide more in-depth insight into the macro-scale neural mechanism underlying the clinical symptoms of schizophrenia and possess the capacity to present more adequate imaging evidence.
By exploring the macro-scale neural mechanisms and the links between macro-structure and functional changes, we may increasingly reveal the relationship between brain phenotype changes and symptoms.
Schizophrenia usually shows positive symptoms such as delusion, hallucination, and thinking disorder, and negative symptoms including decline of social function and adaptability. Auditory verbal hallucinations (AVHs), one of the essential diagnostic symptoms of schizophrenia, occurs in approximately 60–80% of patients, and may also appear in people with other neuropsychiatric disorders and even general populations [17, 18]. This symptom can cause great distress because of its negative content and uncontrolled nature. It has been found that the AVHs in schizophrenia might be associated with hyperconnectivity and abnormal network function between putamen and prefrontal cortex regions [19]. AVHs-related brain activity has also been found within resting-state networks involved in auditory processing, language production/monitoring, and sensory information filtering in schizophrenic patients with AVHs [20]. Specifically, the auditory cortex, dorsal border, insula, putamen, dorsolateral prefrontal cortex, angular gyrus, anterior cuneus, and cochlea [20] were found to be abnormally disrupted.
Based on the excellent visualization of brain phenotype by MRI, several hypotheses have been proposed regarding brain dysfunction in AVHs. For instance, the self-monitoring hypothesis suggests that AVHs is a consequence of failure to inhibit self-generated inner speech, which involves dysconnectivity between the Broca area and temporal cortex. According to the memory intrusion hypothesis, AVHs is a result of activating unpleasant memories which usually contain judgmental voices, and activates the hippocampus. Simultaneously, AVHs may be associated with hyperactivity in the auditory cortex (bottom-up processing) and erroneous modulation from the anterior cingulate cortex, insula, and other brain regions (top-down processing). Previous studies have established the linkage between hippocampal morphometry, negative symptoms, and cognitive impairment. The increased hippocampal activity was revealed to be positively associated with negative symptoms while negatively associated with cognitive impairment [21]. The relationship between hippocampal volume and negative symptoms in individuals with adolescent onset schizophrenia was negatively correlated, which was indirectly mediated by verbal memory impairment [22]. It has been proposed that the negative symptom of indifference is due to abnormality of the subcortical prefrontal cortex, which is implicated in motivation and goal-directed behavior. Stereotyped thinking is negatively correlated with the volume of the right amygdala, and the activation of the amygdala is positively correlated with the severity of face recognition dullness [23].
Since the symptoms of schizophrenia are complex, they often cannot be explained by changes in individual brain structures but rather involve deficits in the coordination between several cortical and subcortical structures. Therefore, MRI is a powerful tool for probing the neurobiological underpinnings of the disease.
Currently, the diagnosis of schizophrenia is made solely on the basis of the psychiatrist’s subjective assessment. Objective and specific neuroimage biomarkers are urgently needed to facilitate faster treatment success [24]. By extracting functional connectivity features using a radiomics strategy, the diagnosis accuracy was 87.09% for patients with schizophrenia and was superiorly validated in the replication data set [25].
Due to the instability of the sub diagnosis of various schizophrenia, the latest DSM-5 had cancelled the subclassification of schizophrenia and emphasized the dimension of the disease instead. However, characterized by persistent primary negative symptoms, the “deficit syndrome. of schizophrenia has been proven to be a relatively stable clinical subtype, which has diagnostic significance and helps to eliminate clinical heterogeneity. In a clinical study applying MRI imaging, it was found that the right lower longitudinal tract, right arcuate tract, and left uncinate tract were fractured in patients with deficit schizophrenia compared with the non-deficient and normal subjects [26, 27]. Moreover, sMRI technology can advance the identification of neuroanatomical differentiation of schizophrenia by displaying general brain patterns consistent with the clinical staging model of schizophrenia, demonstrating the huge potential in discriminating schizophrenia subtypes [27]. Future MRI research on schizophrenia will focus on developing more accurate and specific neuroimaging biomarkers [28], thereby improving the level of early screening and precise treatment of schizophrenia.
The development of predictive biomarkers is a key challenge for schizophrenia. In the early stage of schizophrenia, MRI has the ability to predict the response to antipsychotic drugs [29]. The application of MRI may be of significance in selecting appropriate treatment regimens, evaluating the efficacy of drug therapy, and predicting prognosis. Imaging features that can be used to construct biomarkers of disease include T1 structural image features, DTI reconstructed white matter tract features, bold signal derived functional features, graph theory indicators, metabolic features, and so on [30]. Multi-modal fusion can combine the advantages of various single modes of imaging while overcoming its disadvantages. One model combining both inter-hemispheric functional connectivity features and structural features included cortical thickness, surface area, gray matter regional volume, mean curvature, metric distortion, and sulcal depth in the right precuneus, cuneus, and inferior parietal lobule achieves superior predicting accuracy for early antipsychotic treatment response in schizophrenia [31]. The radiomics method combines artificial intelligence technology and imaging technology such as MRI to extract quantitative imaging features in a high-throughput way. This enables us to conduct an objective quantitative study of mental illness without causing harm to patients and is expected to predict curative effects as well as treatment guidance.
Electroconvulsive therapy (ECT), originally based on the hypothesis of antagonistic relationship between schizophrenia and epilepsy, applies electrical current induced convulsions to alleviate symptoms of major psychosis including depression and schizophrenia [32]. Due to the development of MRI techniques, the progress of psychiatric diagnosis, and the standardization of ECT management, the underlying mechanism of ECT has become clearer and its efficacy has been recognized. Thus ECT related research has been revived in the past few decades. Recent clinical trials have focused on the mechanism of modified ECT and regarded it as adjuvant therapy, besides antipsychotic drug therapy [33]. The application of MRI can not only show the changes in brain structure and function after ECT treatment but also predict the effectiveness of ECT therapy through multidimensional combination analysis. The results show that structural and functional connectivity of the limbic system has changed, including hippocampus, amygdala, etc. [34], as well as insula [35] and thalamus [36], and may consist of part of the mechanism. Changes in these regions based on MRI multi-parameter models are proposed to predict its efficacy [37]. In the future, studies with a larger sample size, stricter control, and longer period of follow-up may produce uncompromised evidence for theoretical research and clinical application of ECT.
Although there is currently no clear MRI imaging standard for assessing the therapeutic effect of antischizophrenic drugs, MRI’s suggestive role in clinical treatment has been internationally recognized. There is also a lot of related research devoted to verifying and exploring relevant standards. One clinical study observed and documented intervention responses to early schizophrenia in MRI images. They measured the amplitude of low-frequency fluctuation (ALFF) and found that compared with non-responders, the baseline ALFF in the left posterior gyrus/inferior parietal lobule increased in responders [38]. In monitoring the effect of drug intervention on schizophrenia, another study found that the clustering coefficient and local network efficiency of patients were lower than those of healthy controls in fMRI. However, this abnormality was alleviated after drug treatment.
MRI can explore alterations of brain phenotypes before and after any treatment from the perspectives of brain structure, function, and metabolism, but in neuro-stimulation it has the added benefit of being able to plan the intervention itself. The application of neurostimulation techniques such as repetitive transcranial magnetic stimulation (rTMS) is a new method that has shown promise. In the current clinical practice, antipsychotics in the treatment of schizophrenia are typically effective for positive symptoms and have little or no effect on cognitive impairments [39]. rTMS does not appear to share this limitation, having promising results in both symptom areas possibly due to an ability to enhance neuroplasticity and remodel connectivity [40]. Low-frequency rTMS stimulation of the left hemispheric temporoparietal junction (TPJ) may improve the auditory hallucination symptoms of schizophrenia. Dysfunction in the dorsolateral prefrontal cortex (DLPFC) of people with schizophrenia is associated with alterations in cognitive function [41], an area of particular interest when neurostimulation techniques are used to investigate schizophrenia. Some studies have shown that rTMS can improve the negative symptoms of schizophrenia by restoring the network connection between DLPFC and the cerebellum. After delivering rTMS stimulation to selectively restore cerebellar-DLPFC network connectivity, the severity of negative symptoms in patients with schizophrenia was improved [42]. Nevertheless, DLPFC is a relatively broad region, which is not easily targeted during rTMS treatment. Therefore, the use of MRI for neuronavigation during the procedure may be useful to precisely locate the target, thereby maximizing the therapeutic potential of nerve stimulation technology.
Very-late-onset schizophrenia-like psychosis is one of the most severe geriatric mental disorders. Because of complicated clinical manifestations, poor treatment response, and pharmacotherapeutic adverse effects, treatment strategies and methods for very-late-onset schizophrenia-like psychosis are limited. At present, the definition of late-onset age for very-late-onset schizophrenia-like psychosis is still controversial. Further research is needed [43], but we have every reason to believe that MRI’s potential in the diagnosis and treatment of this disease extends to this subset of patients.
In recent years, artificial intelligence technologies such as machine learning can objectively model mental imaging data and extract quantitative indicators reflecting the brain structure and functional characteristics of schizophrenia patients, which has brought new opportunities for the application of MRI in schizophrenia. Radiomics, an emerging and effective method for quantitative analysis and prediction using medical imaging big data, is considered to be a bridge between medical imaging and individualized medicine [44]. Combined with artificial intelligence technology, the use of radiomics strategies to extract MRI features to find biomarkers of schizophrenia, facilitate diagnosis, and predict treatment response is feasible and promising. Meanwhile, the trans-scale and multi-omics approaches combining genes and imaging in schizophrenia facilitates the elucidation of gene-related pathogenesis and the exploration of potential biomarkers [45]. Alterations in genes at the molecular level may underlie effects on brain function and structural connectivity, as well as gray and white matter volume and density. The introduction of genomics has provided insight into the many possible genetic and biological variants in the pathogenesis of schizophrenia.
In exploring brain volume changes in schizophrenia, antipsychotic medication intake and cannabis use are the main confounding factors [46]. In a longitudinal study comparing brain volumes of patients who stopped taking medication and of those who did not, MRI results showed a decrease in the nucleus accumbens and putamen volume in patients who stopped taking medication. They showed increased volume in those who continued taking medication. This suggests that stopping the medicine may reverse the effects [47]. A sMRI study observed brain changes in patients with schizophrenia who continued to use cannabis during follow-up. The results showed a significant decrease in gray matter volume associated with cannabis use [48].
Among the contents listed in the article, relevant studies have proved and expounded the far-reaching positive significance and valuable practical experience of MRI in the whole process of screening, diagnosis, and treatment of schizophrenia. In the future, MRI may be widely used in early screening of schizophrenia, neuronavigation for rTMS, evaluation of anti-schizophrenia drug treatment and intervention effects, and prediction of ECT efficacy.
Despite such importance, MRI is currently used only as a reference or hint for schizophrenia research and no diagnostic criteria for schizophrenia based on MRI have been formed. It is worth noting that there are still various limitations and deficiencies in the research. Due to the widespread clinical heterogeneity, a larger sample size is required in future studies to increase statistical power. Furthermore, most studies focus on baseline cross-sectional data and do not reflect progressive changes in schizophrenia brain phenotype. More longitudinal studies are warranted to explore the effects of confounding factors in patients with schizophrenia. The application of MRI in schizophrenia will require continued exploration and improvements in research methodology. It is our hope that this review will aid in these efforts.
DW, FC, and LBC conceptualized the manuscript. SWZ, YC, ZK, XFL, JWF, YWG, XYW, YFF, XC, XSW, PZ, and LBC wrote the first draft of the manuscript. All authors contributed to editorial changes in the manuscript. All authors read and approved the final manuscript.
Not applicable.
Thanks to all the peer reviewers for their opinions and suggestions.
This work was supported by Shanghai Sailing Program (21YF1402400), National Natural Science Foundation of China (82102138), Health Care Project of Chinese PLA General Hospital (2020ZD05), Basic Research Reinforcement Project (2021-JCJQ-JJ-1079), Innovative Project of Chinese PLA General Hospital (CX19028), Project funded by China Postdoctoral Science Foundation (2020M683739), and the Fourth Military Medical University (2019CYJH).
The authors declare no conflict of interest.
Publisher’s Note: IMR Press stays neutral with regard to jurisdictional claims in published maps and institutional affiliations.