Background: Biomarker detection strategies have, in recent years, been
moving towards nucleic acid-based detection systems in the form of aptamers,
short oligonucleotide sequences which have shown promise in pre-clinical and
research settings. One such aptamer is M5-15, a DNA aptamer raised against human
alpha synuclein (
Biomarker detection has become a critical component in the diagnosis of disease
and has emerged as a critical factor in deciding courses of treatment of
patients. Over 30 years ago, the first monoclonal antibody was developed for
biomarker detection with the first licensed products being deployed in 1986 [1].
Global market value for monoclonal antibodies surpassed
Aptamers are defined as synthetic ligands composed of single stranded RNA or DNA which have unique structures that can be designed to target specific biomolecules with high affinity [9]. The production of aptamers is achieved through a process of systematic evolution of ligands by exponential enrichment (SELEX) and was discovered by three groups independently at the beginning of the 1990s, beginning with RNA aptamers then expanding into DNA and peptide-based aptamers (referred to as affimers) developed today [10, 11, 12]. Nucleic acid-based aptamers are single stranded nucleic acid structures which can form secondary structures by folding in on themselves into thermodynamically stable shapes [13]. These structures consist of double helices, and hair-pin structures of varying sizes governed by the number of mis-match pairing of bases which produce internal loops and three-way helical regions [14]. While SELEX has been used previously for the development of aptamers, there have been movements towards the use of in silico computer-based modelling to streamline the production and design of aptamers [15]. Techniques like M.A.W.S (making aptamers without SELEX) are the most notable recent examples of in silico aptamer development and has been seen as a gold standard for the development of aptamers in silico [16]. The focus of aptamer generation in silico looks to remove some of the initial high costs associated with aptamer selection, as well as looking to produce high affinity aptamers prior to the purchase of reagents needed for in vitro validation of aptamer binding [17].
One of the main advantages of aptamers over their monoclonal antibody
counterparts is tissue penetration, as well as a capability to be raised against
targets that do not elicit an immune response, such as charged ions,
intracellular proteins and vitamins [18]. The use of aptamers has emerged as a
potential detection strategy for a wide number of biomarkers, with particular
interest in the detection of markers for neurological disease [19]. In
particular, alpha synuclein (
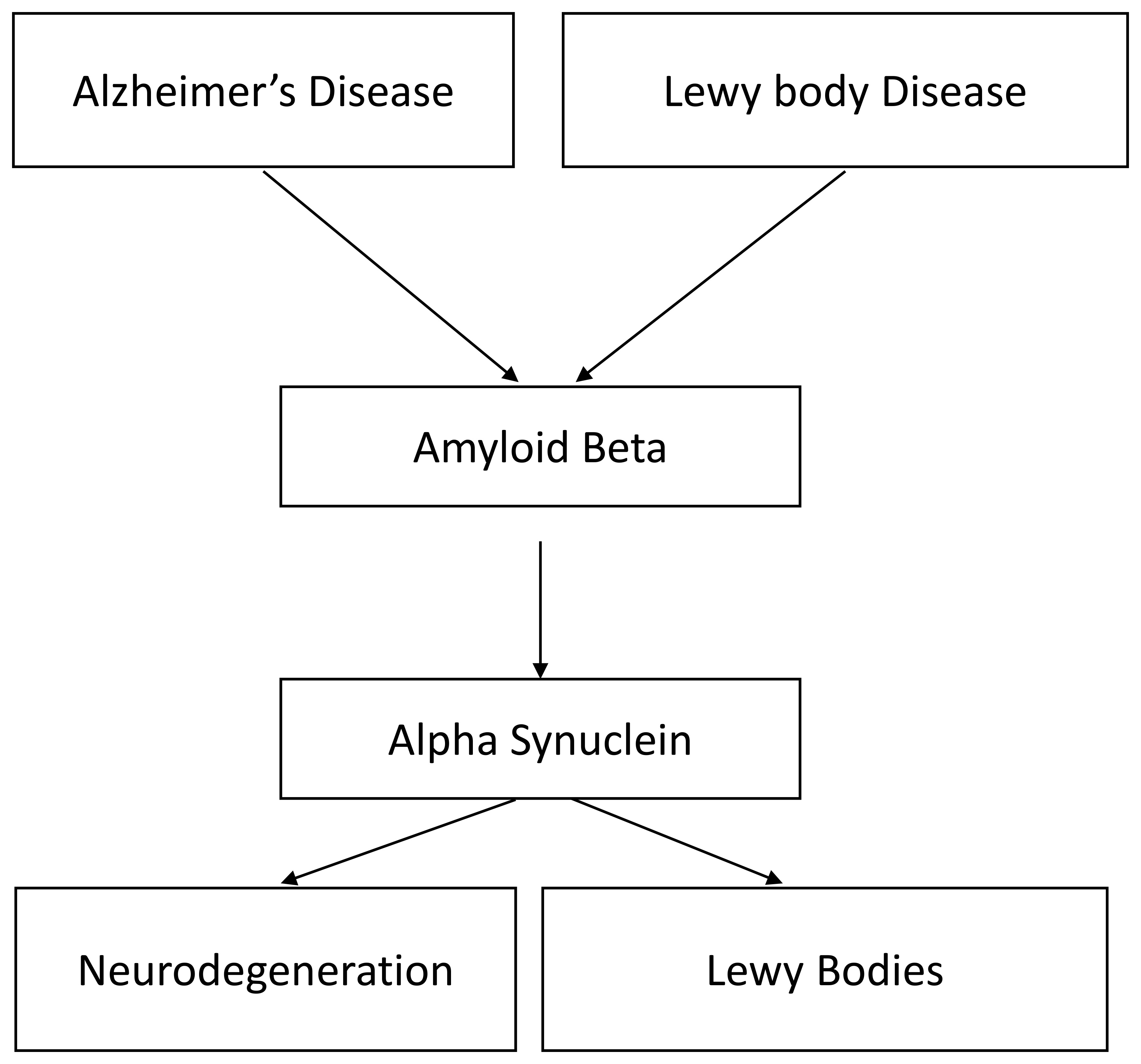
Summary of the interactions in AD. Notably
The link between AD and
The concept of computer aided drug design, and by extension in silico aptamer design, has been gaining traction in recent years, specifically due to its ability to streamline the design of innovative treatment and detection strategies for a wide variety of applications. Advances in computer algorithms capable of predicting the folding characteristics of single stranded DNA, as well as increasing availability of these software, have been critical to the development of in silico methods [26]. The standardised approach to in silico aptamer design is summarised in Fig. 2 (Ref. [27]).
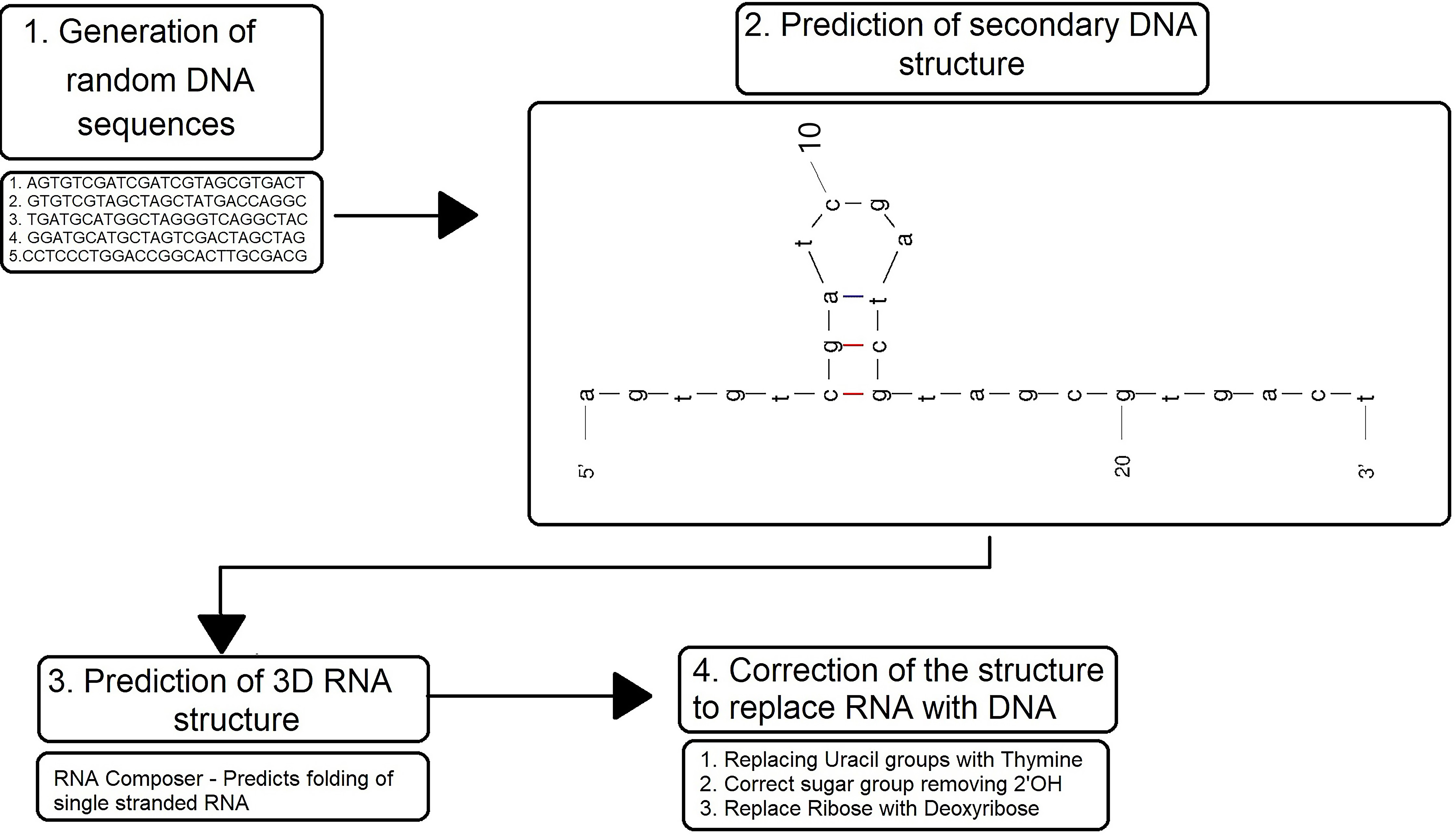
Summary of steps within in silico aptamer design. RNA folding algorithms have proven to be effective at predicting DNA aptamer structure despite minor chemical differences. It is common practice to correct these structures in post. Image based on information contained within [27].
Sequence generation can be upscaled to be comparable with in vitro SELEX, allowing for the screening of an exceptionally large number of sequences. The difficulties come when establishing selection criteria for the computer-generated sequences, and which ones to proceed with in a docking experiment as the large number of sequences make it impractical to dock every aptamer produced. A common approach is to look at the free energy potential of the aptamers secondary structure [26] and secondary structural motifs [28] to screen potential aptamer candidates. The second option is particularly useful when looking at aptamer optimization studies, as the original sequence can be mutated at specific points to see what impact this will have on overall binding.
Following sequence generation, aptamers are then docked to the target molecule
in question. There are a number of programmes which are available for use in this
field, some which are freely available and some requiring a subscription [28].
Irrespective of the programme used, the objective is to create a pose (two
molecules which have formed a complex) with the lowest Gibbs free energy
(
Docking approach | Key characteristic |
Rigid docking - fixed receptor and ligand | Both receptor and ligand bond angles and atomic positioning are fixed. This mimics the traditional “lock and key” theory for ligand/receptor interactions. Is the least resource intensive out of the approaches, but does not take into consideration structure flexibility. It also limits understanding of the solvents impact on docking |
“Soft docking” - rigid receptor, semi-flexible ligand | Small degree of flexibility within a small area for the ligand, the receptor remains fixed |
“Side chain flexibility” - rigid receptor, side chain flexibility only | Majority of the structures (both ligand and receptor) are fixed in position, with only the amino acid side chains able to flex |
Composite receptor modelling - flexible receptor | Combined structural information breaking up larger receptors into smaller subunits. These subunits are then contained within a single docking “box”. Allows for degree of flexibility in the receptor while minimising computer load |
Ensemble docking - Flexible receptor | Generation of a composite receptor structure from multiple components, with each subgroup capable of rotating freely through its structure. Ligand can be either fixed or flexible in these scenarios. Critically in this instance they are not confined to a single “box”, but are made up of multiple components that work independently. This is a processor intensive position and requires time and resources to run effectively |
In silico docking is not without its limitations however, despite the advances in computer modelling and DNA/RNA folding algorithms. Issues with computer processing load [27], particularly with more flexible approaches to docking, as well as the difficulties in accurately mimicking the electrochemical environment these molecules would experience in vivo [30] do mean that at this stage, predictions need to be validated in vitro before definitive conclusions can be made. However, this does not detract from the usefulness of in silico methods for they have the potential to both streamline and improve the aptamer generation process.
In this study, an experimentally produced aptamer was docked alongside an
in silico developed aptamer against human
Aptamer sequences were generated using Python script to create a library of 1000
sequences with an N30 wobble region flanked by constant primer binding regions
(ATAGTCCCATCATTCATT-N30-AGATATTAGCAAGTGTCA). Sequences were retained following
prediction of thermodynamic stability using the DINAMelt server-QuickFold web
server [31]. Retained sequences were required to have negative
Folding temperature | 25 |
Ionic conditions | [Na |
Distance between paired bases | No limit on distance |
Sequence type | Linear |
Maximum possible foldings | 50 |
Secondary structural information for 50 structures, selected based on their
Vienna sequence outputs from MFold were used to assist in the production of 3 dimensional models using RNAcomposer [33]. To account for this being a programme which folds RNA, all thymine residues within the sequences were replaced with uracil groups for the purpose of folding. Vienna sequences were input along with the base sequence data, with the top 50 sequences being run in batch format within RNAcomposer. Structure prediction used default parameters for the purposes of folding.
Protein data bank (PDB) output files of the predicted sequences were converted into mol2 format using CSD-Discovery-Mercury [34]. Structures were then modified to reintroduce the deoxyribose groups, replacing the ribose sugar groups within their structures using PyMol [35] via command line editing within the software (Schrödinger, New York, USA). Commands were set to remove O2’ and HO2’ atoms from all residues and to add a hydrogen to the C2’ atom of each residue. Uracil groups were replaced with thymine groups using the mutagenesis wizard also contained within the PyMol software. Files were then exported back into CSD-Discovery-Mercury to add any hydrogens to the structure. In addition, bond angles and types were corrected as some had been incorrectly modelled during the file conversion process. Of the 50 structures that were originally generated, the top 10 were selected to dock with the target molecule. Primary structural similarity to M5-15 was used as the primary criteria when selecting the aptamer, followed by thermodynamic stability. This is due in part to the nature and composition of free hydrogen linkage points within the short structure determining how the single stranded nucleotide will fold in on itself [36].
Docking experiments were analysed determining the predicted interactions between the components of the final pose. Bond distance, nature of interactions and type of interaction were all documented and analysed. Overall fitness of poses was determined using ChemPLP fitness scoring within CSD-Discovery. Structures were virtually screened in blocks of 10 using LYS 96, O as a central atom and a radius of 81Å, encompassing the target protein completely. All rotatable bonds were fixed to create a rigid dock and search efficiency was set to low/virtual screening. Fitness scoring function was set to ChemScore with no re-scoring function selected.
Binding energies were determined using follow up confirmation docking
experiments using AutoDock Vina. Docking parameters between the programmes were
kept constant. The docking positions generated by CSD-Discovery were used to
inform the choice of grid location for docking in AutoDock Vina. The top 3
aptamers selected from CSD-Discovery were docked against
File name | Modifications to file | Reference |
1xq8 (Human |
No modifications made | [37] |
4ts2 (Spinach RNA aptamer in complex with DFHBI ((5Z)-5-(3,5- | Metal ions (Magnesium and Potassium) removed | [38] |
difluoro-4-hydroxybenzylidene)-2,3-dimethyl-3,5-dihydro-4H-imidazol-4-one), magnesium ions) | Ligands (beta-D-fructofuranose-(2-1)-alpha-D-glucopyranose and Cytidine-5’-Phosphate-2’,3’-cyclic phosphate, (5Z)-5-(3,5-difluoro-4-hydroxybenzylidene)-2,3-dimethyl-3,5-dihydro-4H-imidazol-4-one) removed | |
Hydrogens added |
Top poses from CSD GOLD were used to determine the best aptamers to move forward to docking in Autodock Vina based on a combination of Fitness score, number of hydrogens and average bond length of interactions. Analysis of variance was conducted on the data produced by Autodock Vina to determine any significant changes in binding energies in comparison to M5-15.
The in silico selection of ssDNA aptamers against
Aptamer | Sequence | ΔG kj/mol |
M5-15 | Forward -GTATGGTACGGCGCGGTGGCGGGTGCGTGG- Reverse | –6.39 |
120 | Forward -CGGGTTAGGTGGAAGATCCCACCTATTCCG- Reverse | –8.52 |
123 | Forward -GTGGGGGTCCAGGCCTAGTACTGCTAAGGC- Reverse | –7.70 |
36 | Forward -CATAAGCCCATGCTAGACTTGCGAAAGGGT- Reverse | –7.16 |
TMG-79 | Forward -ACGTTGGTGCATGCCGAAGCCGGCGAATGC- Reverse | –6.96 |
60 | Forward -GGGGGATACTAACCCGTCCCCGGCGAGCGG- Reverse | –6.27 |
54 | Forward -ATAAGGGCCCGTGCCGTTCTACGGTTCCCG- Reverse | –6.22 |
195 | Forward -CCTTTCGGGGGCGGCCCCCCCTTCCGGTAT- Reverse | –5.96 |
116 | Forward -TAACTTTTAAAATCTGTAGAGCAGGTGCGA- Reverse | –5.79 |
125 | Forward -ACGTTTGGGGAAATAGTATCCCCCCAACAT- Reverse | –5.28 |
30 | Forward -CTGAAAGGGGCTGTGCCGCCGGTAATAATA- Reverse | –4.83 |
The known sequence which binds to
Pairwise alignment (Fig. 3) was conducted to determine the sequence homology between each candidate and M5-15. When aligned, TMG-79 was shown to have the largest percentage similarity (51.4%), suggesting a degree of similarity between the structural motifs of TMG-79 and the M5-15 (see Figs. 2,4). Aptamers 123 and 125 both show lower similarities than TMG-79 which would explain structural differences within aptamer 123 and 125 such as the appearance of structural motifs not present in M5-15 and the change in relative position of stem loops.
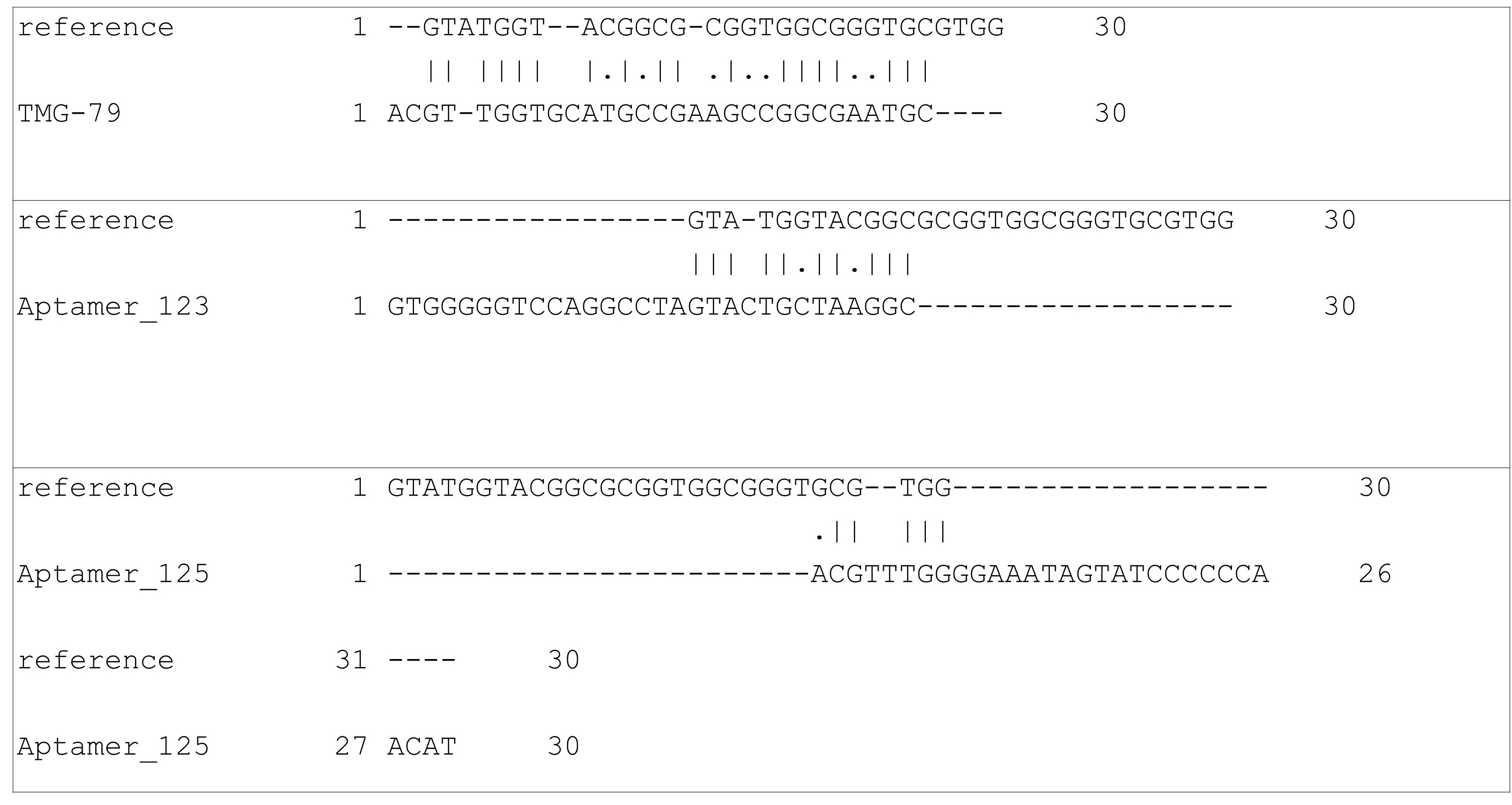
Pairwise alignment of N30 wobble region for top three selected aptamers based on PLP Fitness score against experimentally created aptamer. Similarity: TMG-79 = 51.4%; Aptamer 123 = 20.8%; Aptamer 125 = 9.3%.

Predicted secondary structures for top 10 aptamers based on
GOLD docking results show a large improvement in fitness scores when comparing positive and negative controls to in silico aptamers. TMG-79 PLP fitness (Fig. 5) indicates it could be a candidate for greater binding potential. Further analysis of docking data shows that aptamer complexes for candidates 123 and 125 have a larger number of predicted H bonds with a comparable average bond length (Table 5).
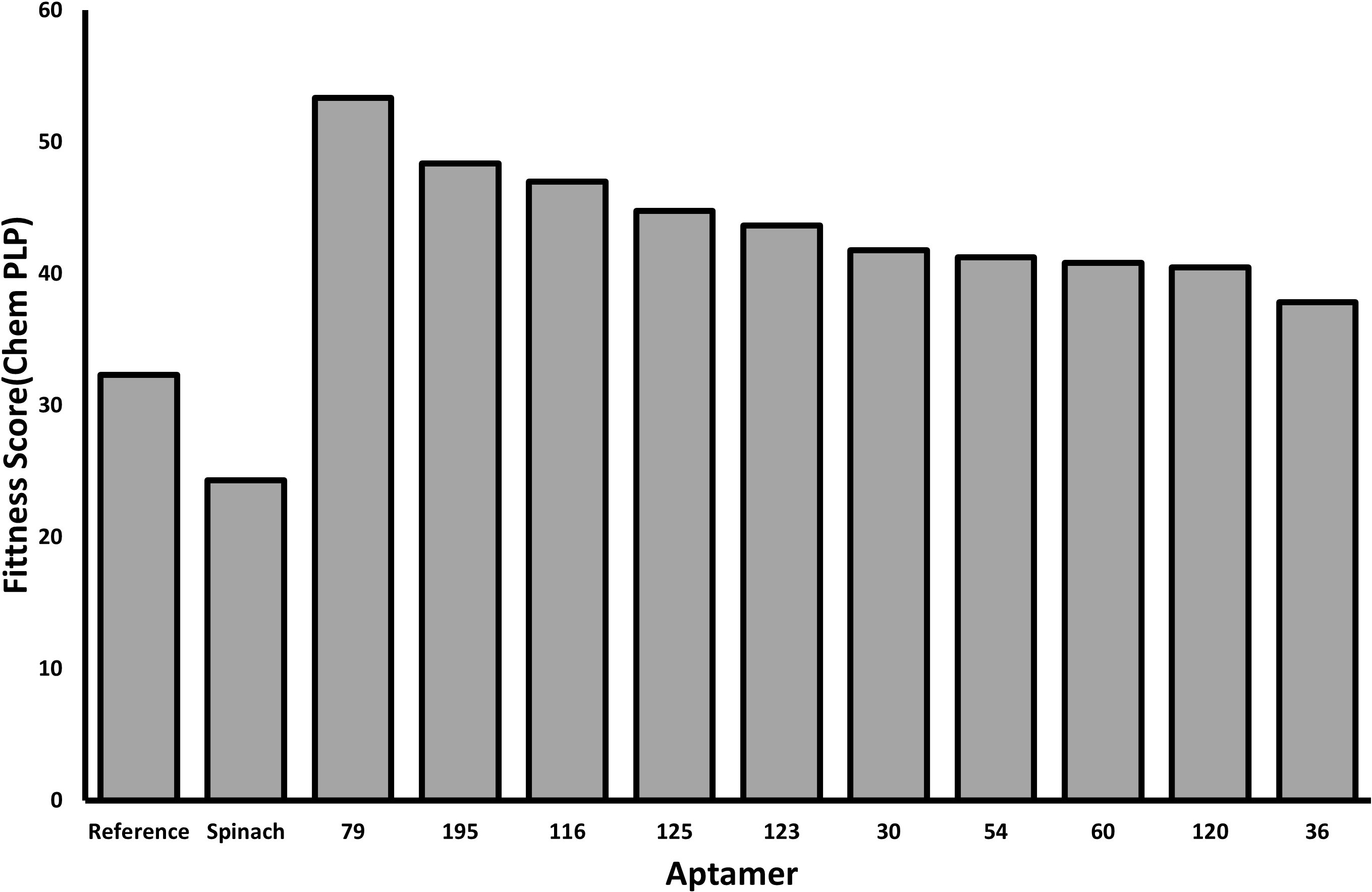
Data showing the ChemPLP fitness score for the top poses of the previously selected aptamer sequences. The reference aptamer and spinach aptamer serve as positive and negative controls, respectively. Each selected aptamer shows a higher docking score for their top pose when compared to the reference aptamer.
Aptamer | PLP. Chemscore. Hbond (3.dp) | Number of H bonds | Average bond length (3.dp) | Top pose binding energy (kcal/mol) | Mean Binding energy (kcal/mol) (2.dp) | Mean dist from rmsd l.b. |
Reference | 0.008 | 2 | 2.948 | –16.8 | –15.90 | 27.312 |
4ts2 | 0.000 | 0 | - | –17.6 | –16.22 | 21.583 |
79 | 2.000 | 2 | 2.884 | –18.7 | –17.77 | 13.801 |
125 | 1.089 | 3 | 2.944 | –17.0 | –16.40 | 16.830 |
123 | 4.976 | 5 | 2.734 | –18.4 | –16.48 | 16.314 |
rmsd, root mean squared distance. |
Despite having a lower fitness score than that of TMG-79, aptamer 123 was predicted to form a larger number of H-bonds making it a promising candidate. This aptamer also appears to show some minor structural similarities to the reference aptamer, particularly when examining its hairpin loop structures. Large variations in ChemPLP score were observed across the poses for each aptamer due to the low search efficiency used for virtual screening.
In addition, follow up experiments using AutoDock Vina demonstrating top pose and average binding affinity for the reference, spinach and the top 3 in silico generated aptamer sequences. Binding free energy was lower for all generated sequences compared to the M5-15, these changes were not statistically significant (TMG-79, p = 0.18; aptamer 123, p = 0.17; aptamer 125, p = 0.42; Spinach, p = 0.18).
Further analysis of the reference aptamer and TMG-79 showed that interactions
occurred mainly in or around the stem loop structural motifs of each aptamer. The
reference aptamer was predicted to form H-bonds at residues LYS97 and LYS21. In
contrast, TMG-79 was predicted to form H-bonds at protein residues LYS80 and
GLN99 suggesting that it may interact in a similar region to the reference
aptamer. Differences in the overall structure of TMG-79 compared to the reference
aptamer support the observation that the interactions are similar, but at
different points within the protein. Aptamer 123, whilst having a lower overall
ChemPLP fitness score, had a greater number of interaction points in these
simulations, importantly with polar side chains of amino acids such as THR81,
suggesting stability of these bonds within the complex. However, given that
ChemPLP score is a summary of critical factors, including binding energy, this
suggests that while there are greater interaction points, these interactions are
less likely to form spontaneously and be stable. Bond distances observed from the
reference aptamer are also greater than in 2 of the 3 aptamers generated, and
mean binding energy predicted greater affinity across all aptamers screened. This
indicates a tighter binding potential with the generated sequences compared to
M5-15. Structural comparisons of the poses suggests that all aptamers bind to a
similar region within
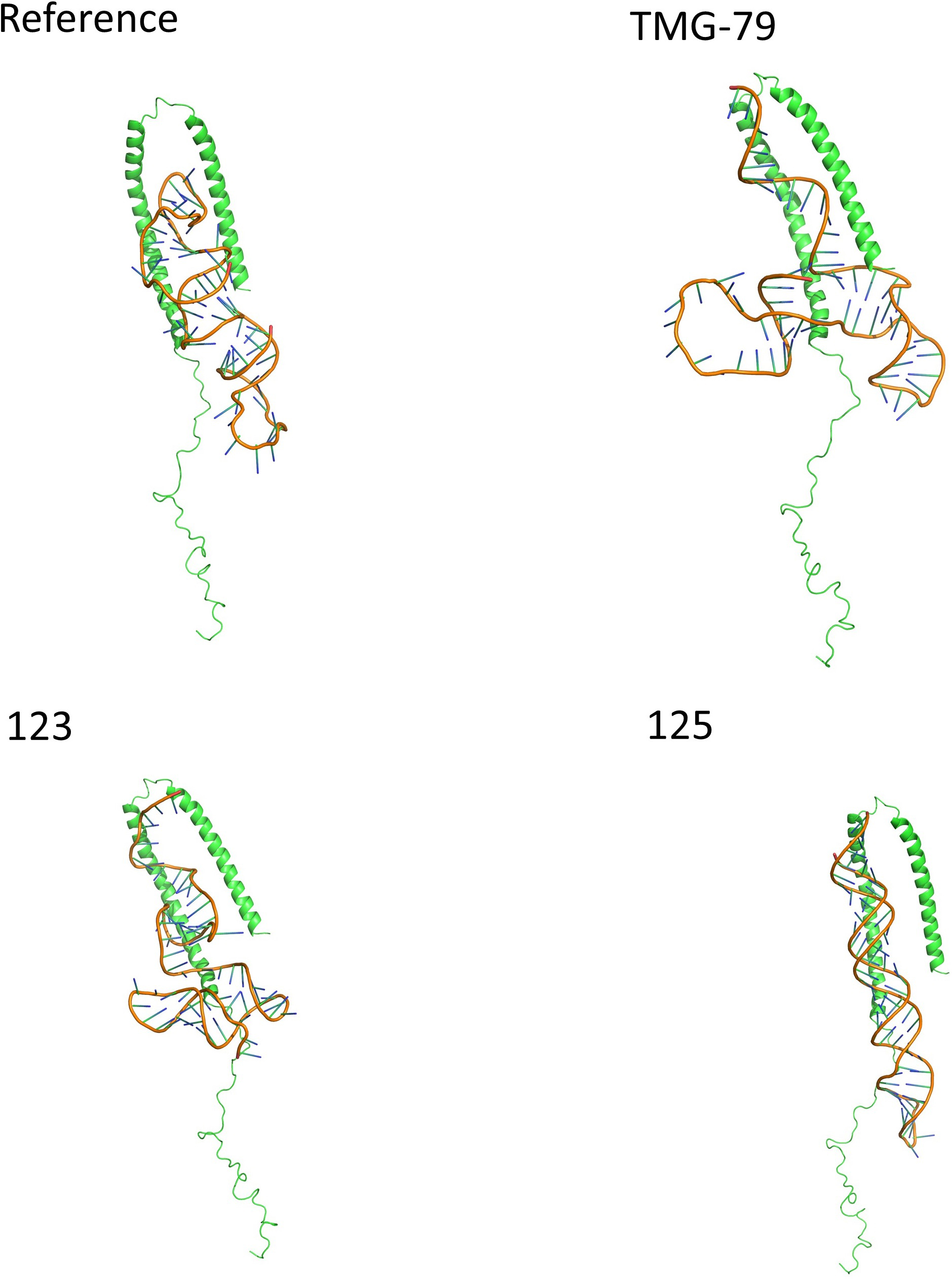
Showing the binding position and orientation of each aptamer in
their top pose when docked against alpha synuclein. Each aptamer is predicted to
bind within the alpha helices of
The purpose of this investigation was to determine if it was possible, based on a template sequence derived experimentally, to improve upon the binding affinity of an aptamer to a given target. While there has been significant advancement in aptamer generation in recent years, any improvements that can be made will naturally be of benefit in the future. As part of this work, the team has managed to generate several potential aptamer candidates which demonstrated higher affinity for the target molecule than the experimentally derived aptamer within our model. Data presented here suggests that it is possible to produce an aptamer which has the potential to be comparable or better than the experimentally derived sequence. While the data presented here does not suggest a significant difference in predicted binding affinity, it does demonstrate that there is a possibility with a greater data set and use of the aptamer in real world settings that the aptamer could either perform as well or outperform the reference aptamer. Analysis of the predicted interactions could shed light on the reasons for the observed increases in affinity, despite this not being statistically significant in this case.
When comparing the reference aptamer interaction with the generated sequences,
there are similarities between the way they interact with
Thr and Lys interactions with DNA are well documented, with Thr interacting predominantly with phosphate backbone groups [41], and Lys predominantly interacting with guanine groups [42]. Specifically, when looking at Lys, it has been reported to interact with oxidized guanine residues (8-Oxo-G). Binding induces conformational change in the 8-oxoguanine DNA glycosylase (Ogg) required for the removal of 8-Oxo-G, an important DNA repair mechanism [42]. While there are no 8-Oxo-G residues present within the structure of the aptamer proposed here, it appears that there is a natural interaction between Lys and guanine. Thr based hydrogen bonding has the highest propensity in highly hydrophilic environments [43], and since the target protein is water-soluble and primarily found in neuronal tissue, there is a suggestion that this interaction may occur in vivo. Following on from this, we should consider the possibility of the predicted Gln, Asn and Ser interactions between the generated aptamer and the target. Gln has been reported previously to have strong association with polar side chains and phosphates contained within the backbone, as well as interacting with the nitrogenous base component of adenosine [41]. Asn is generally associated with base recognition binding, predominantly to adenosine, and has been demonstrated in synthetic zinc finger constructs like 1meyC [44]. These interactions have also been observed in B-DNA binding of transcription factor-like elements [45]. Ser rich proteins are essential pre mRNA splicing factors. They regulate constitutive splicing and influence alternative splicing processes through an extensive and controlled phosphorylation of the Ser residues [46]. Ser residues are highly conserved within the DNA binding domains of bZip transcription factors of Arabidopsis thaliana, and were reported to be in physical contact with DNA [47] specifically providing a binding point through the hydroxymethyl side chain, something that was observed here in our model.
Interestingly, the non-species aptamer 4ts2 demonstrated a greater binding
affinity for the target M5-15 which was an unexpected outcome of the work. This
may be in part due to the very nature of
When considering the structures of the aptamers, it is important to consider the presence and size of key structural motifs within the aptamer. Loops, both external and internal, tend to be much more flexible in nature when compared to standard Watson and Crick paired sections or other loops such as stems and bulges [51]. This inherent flexibility, combined with the relatively small size of aptamers in three-dimensional space, allows for the aptamer to integrate with regions of proteins that larger more complicated structures, like antibodies, are simply incapable of achieving [14]. This has been demonstrated previously by Autiero et al. [52] when looking into RNA aptamer binding to S8 ribosomal proteins. Here the ability of the aptamer to adjust its structure to bind to the target not only improves the binding efficiency of the aptamer but is also a critical property of aptamers that warrants further investigation.
When looking at the reference aptamer and the top aptamer generated as part of
this study, displayed in Fig. 7, the most notable differences between them are
the extension of the stem loop between C7 and G25 and the incorporation of a
greater number of Watson and Crick pairing between G27 and C48 in TMG-79. This
increase in bulge size, and the incorporation of unpaired G44 and A45, gives
greater flexibility to this extended region in comparison to the reference
aptamer’s similar structure between C26 and G39. An additional consideration is
the increase in the number of potential binding points which have been predicted
within TMG-79. This may be due to the increased flexibility of the aptamer
compared to the reference aptamer which, while not being a rigid structure, has a
greater number of structural motifs limiting its flexibility [52]. It is
also important to remember that, while the increase in flexibility is important,
the structural motifs themselves provide unique binding properties which allow
for aptamer/protein interactions. However, there is evidence to suggest that both
stable and unstable secondary motifs are important in aptamer binding. Adachi
et al. [53] demonstrated that their RNA aptamer’s inherent instability
actually promoted tighter binding with higher specificity compared to aptamers
which had more stable structures. This conflicts with Troisi et al.
[54], where the structural motifs interact in a specific fashion to promote
greater affinity for thrombin of NU172. The difference between these studies and
their approach to the development of the aptamer may be down to either
differences in complexity of the proteins or their specific locations. In both
examples presented, they are looking at relatively complex proteins, either
thrombin [54] or interleukin 17 receptor A/F (IL17A/F) inhibition [53]. Within
the second example, the hope is to inhibit formation of IL17A/F, requiring only
to be able to bind to one component to competitively inhibit this function. In
contrast, Troisi et al. [54] identified sequence specific binding to the
thrombin protein which could be significantly reduced based on single amino acid
mutations. The conclusion that can be drawn from this is that location, structure
and the complexity of the proteins involved dictates the requirement for either
rigidity or flexibility within the aptamer’s structure which influences its
ability to bind to its target. Considering the inherent properties of
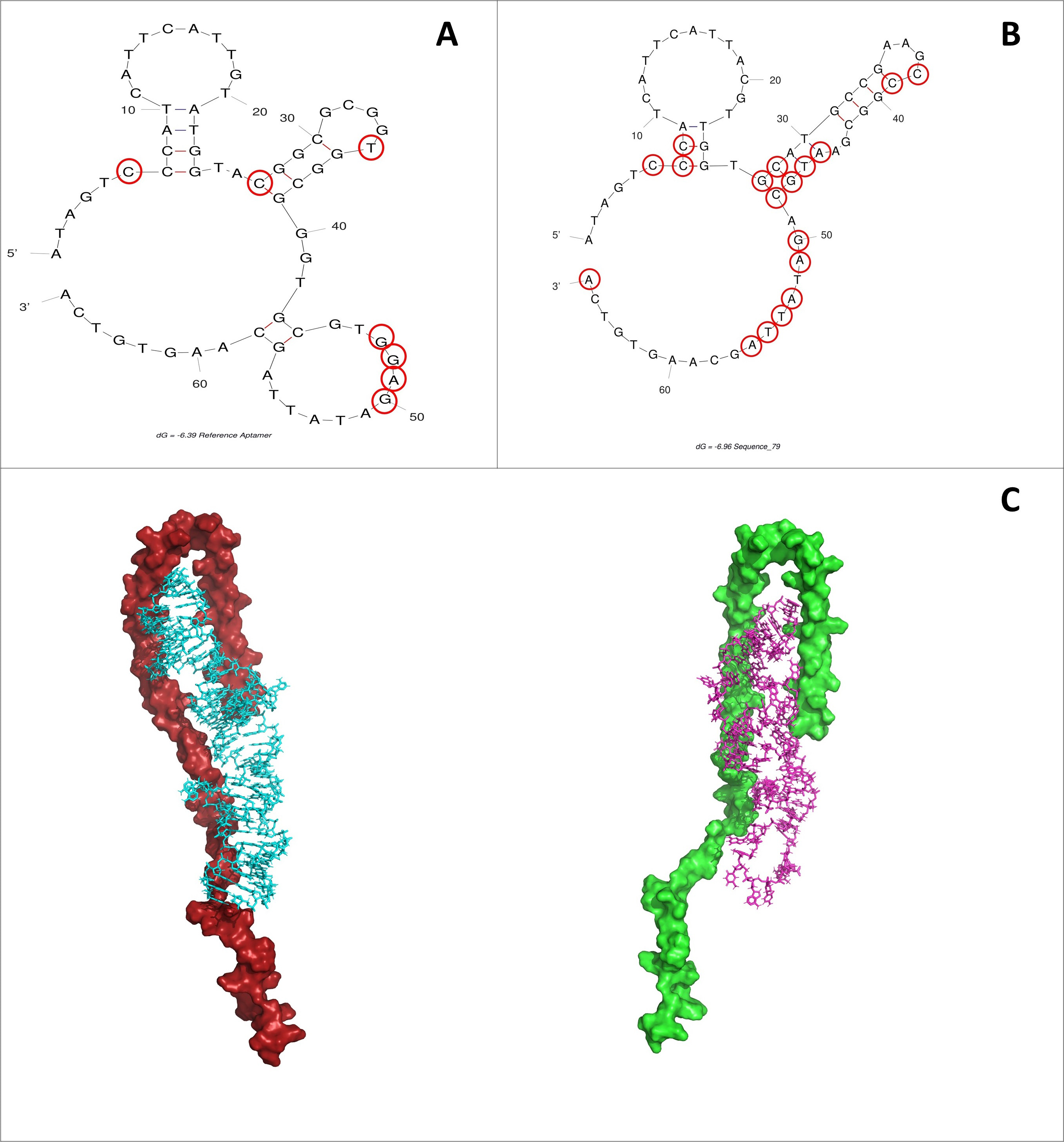
Binding interaction points highlighted in red. (A) M5-15. (B)
TMG-79. (C) While there are similarities between the two aptamer structures
(51.4% sequence homology), the notable difference is the lack of stem loop
between G43 and C58 within the reference aptamer. This change results in a larger
bulge region in TMG-79, allowing for increased flexibility of the overall aptamer
structure. Top pose predictions of 4ts2 (left) and M5-15 (right) against
While these models are based on artificial, computer-generated structures it
does present an interesting case for moving towards a hybrid in silico/in
vitro development strategy for aptamer selection. Within this work we have
demonstrated using in silico methodologies that you can refine the
structures of experimentally derived aptamers to provide a structure which has
the potential to have greater affinity for the target. Predicted interactions, as
well as calculated free energy, suggest that TMG-79 has the potential to be a
more effective means of detecting
All authors contributed to the study conception and design. Model design, python scripting for library generation, docking simulations and data collection was performed by MR, TAI. MR and GDZ assisted in data interpretation and writing of the 1st draft of the manuscript. AFBDA, SD, AAlbrakati, GESB and AAlexiou assisted with final manuscript writing. All authors approve the final version of this manuscript.
Not applicable.
The authors would like to thank the University of Derby for supporting the project. We would like to thank the Taif University Researchers Supporting Program (project number: TURSP -2020/151), Taif University, Saudi Arabia.
This work was supported by Taif University Researchers Supporting Program (project number: TURSP -2020/151), Taif University, Saudi Arabia.
The authors declare no conflict of interest.