This is a perspective on some theoretical studies obtained in the framework of the dissipative quantum model of brain. The formation of long range neuronal correlations is described in terms of quantum field theory mechanisms operating in systems with a huge number of degrees of freedom. Memory states are constructed through the condensation in the lowest energy state of quanta associated to the long range correlations. Many properties derived from such a modeling are discussed, also in relation with classical/quantum modeling interplay. The brain flexibility in responding to incoming inputs producing novel correlation patterns is attributed to the chaotic character of trajectories or paths through the memory states. A relevant role in the model is played by the fact that the brain is permanently open to its environment. The brain/mind activity is thus described in the formalism of dissipative systems, also accounting for the formation of the meanings of the information carried by the perceptual experiences. A recent novel description of criticality in brain activity during dreaming, meditation and non-ordinary brain states is briefly mentioned. In the model, it is proposed that consciousness finds its origin in the permanent dialog or interaction of the brain with its environment. Although a long way has been done, much work is still necessary to understand the extraordinary functional properties of brain.
In 1991 Walter Freeman was observing that his laboratory observations were showing that the study of the single neuron and the microscopic approach dominating the research in neuroscience could not fully account for the processes involved in perception and its elaboration by brain. Rather it was clear that such brain activities could be understood only provided that collective dynamical activity of a myriads of neuronal cells would be analyzed and such macroscopic contributions would be considered together with the microscopic results (Freeman, 1991). On the other hand, Ricciardi and Umezawa (1967), considering the state of the research in neuroscience and on the basis of the experimentally successful results obtained in the quantum field theory (QFT) of solid state and high energy physics were asking whether the behavior of the single nervous cell would be really essential in determining the functional brain activity. Such a doubt, they were arguing, arises since if this would be the case, many pathological behaviors in brain functioning would be currently observed, which instead are not. As they were remarking, the observations of long-range correlations among many regions of the brain arising as simultaneous responses to external stimuli cannot be explained by classical physics models, which then suggests that adopting a quantum model, similar to those successfully used in many-body physics, could be useful in brain studies.
These statements by Freeman (1991), Umezawa and Ricciardi, and similar ones by Lashley (1942), and Pribram (1972) show how clear was their level of awareness of the crisis in neuroscience studies, notwithstanding the enormous progresses in the research of molecular biochemistry and cell specific properties obtained in those same years, and the reader is strongly advised to read the above mentioned statements in the original words by Freeman, and Ricciardi and Umezawa (1967), which therefore I report as footnotes1,2 (1Freeman (1991): “My own group’s studies, carried out over more than 30 years at the University of California at Berkeley, suggest that perception cannot be understood solely by examining properties of individual neurons, a microscopic approach that currently dominates neuroscience research. We have found that perception depends on the simultaneous, cooperative activity of millions of neurons spread throughout expanses of the cortex. Such global activity can be identified, measured and explained only if one adopts a macroscopic view alongside the microscopic one.” 2Ricciardi and Umezawa (1967): “First of all, at which level should the brain be studied and described? In other words, is it essential to know the behavior in time of any single neuron in order to understand the behavior of natural brains? Probably the answer is negative. The behavior of any single neuron should not be significant for the functioning of the whole brain, otherwise higher and higher degree of malfunctioning should he observed,…the activity of any single neuron is not significant, but rather the patterns of activity of clusters of them; what is important is only a quantity somehow related to the activity of the whole cluster, which does not change appreciably as function of the number of alive neurons belonging to that cluster…the existence of similar and almost simultaneous responses in several regions of the brain (a kind of long-range correlation) to a particular stimulation technique does not find any explanation in terms of activity of the single nerve cells: new non-classical mechanisms have to be looked for…it is strongly suggestive of a quantum model. In other terms, one can try to look for specific dynamical mechanisms (already known in physics of many degrees of freedom) which can satisfy the essential requirements of the observed functioning of the brain.”).
On the basis of these premises, considering that brain is in a permanent interaction with its surrounding world (the environment), in 1995, such a dissipative characteristics of the brain dynamics was formally described, which led to the dissipative quantum model of brain (DQMB) (Vitiello, 1995, 2001).
In the following Sections, by resorting to published results, I discuss few aspects of the DQMB. In Section 2, I review the Ricciardi and Umezawa (RU) model and the DQMB. The motivation for presenting the main features of these models is in the fact that it has been there that the dynamical formalism of QFT has been originally used for the study of brain activity and therefore the understanding of the subsequent discussion in the paper requires the knowledge of the conceptual and formal frame in which those models have been formulated. The “red thread” linking the various considered aspects is indeed the study of the dynamical cooperative activity of millions of neurons, as stressed by Freeman and above recalled, which must complement the study of the properties of individual neurons, by itself absolutely necessary but not sufficient for the comprehension of the complex functional behavior of brain. Within and consistently with such a dynamical vision, in Section 3, the concepts of coherence, phase transition, criticality, chaotic trajectories, the construction of “meanings” associated to perceptual experiences, will be discussed. Section 4 is devoted to conclusive remarks.
I will briefly comment on some aspects of the QFT formalism which might be not familiar to the QFT non-specialist reader. An useful introduction fully relying on qualitative presentation can be found in the references (Vitiello, 2001, 2018).
Let me shortly present distinctive features of the RU model and DQMB useful for the better understanding of the following discussion. Then I will consider the interplay between brain and mind and the formation of meanings out of perceptual experiences through which the brain collects information about its surrounding environment.
As said in the Introduction, the unifying thread linking the various parts of this work finds its concrete realization in the QFT study of collective modes, the Nambu-Goldstone boson condensation and the associated long range correlation waves (see below). Therefore the different aspects and processes described in the following find their unitary origin in these genuine QFT dynamical features. This is not in contrast, but rather it complements the more widely followed approach focused on the properties of individual nervous cells.
The key mechanism in the RU model and in its extension to the dissipative model is the one of spontaneous breakdown of symmetry (SBS) in QFT.
Suppose that continuous transformations forming a group, say
The order parameter acts as a label for the infinite number of physically inequivalent spaces of states (unitarily inequivalent representations) existing in QFT (in contrast, the state spaces in quantum mechanics (QM) are physically (unitarily) equivalent). A transition through inequivalent state spaces is called a “phase transition”. This is a critical process since different state spaces are, as said, unitarily inequivalent. Criticality thus characterizes phase transition processes.
In the RU model, the brain state is supposed to be shifted to a new one prompted by a newly coming external stimulus. This produces SBS and the “recording” process is achieved through coherent condensation of the NG modes. The order parameter represents the “code” (label) of the information carried by the stimulus. The effective mass of the NG modes due to boundary effects is very small (NG modes are quasi-massless), thus their associated long range correlation waves extend over large distances forming extended correlated patterns. This means that a huge number of neurons participate to memory recording resulting from such long range correlations, rather than a small number of nervous cells belonging to a quite small domain. This provides a protection of memories against local destructive actions on few individual neurons, as indeed observed.
The memory recollection mechanism is induced by a stimulus that can be not identical to the one causing recording, but similar to it. Such a similar stimulus may indeed excite the condensed NG modes (with a little expense of energy since these modes are quasi-massless) and the memory is “consciously” felt during the excitation time. The excited mode has finite life-time, and the recall activity is experienced as a flashing (temporary) activity, as indeed commonly experienced.
The model presents other interesting features fitting with observations. For example, it accounts for the memory stability in the presence of the brain intense ongoing electrochemical activity, the interaction of the memory state, which, due to coherence, is a macroscopic quantum state, with the classical level of biochemical activity, etc. For brevity, however, I do not report them here. See Ricciardi and Umezawa, 1967; Stuart et al., 1978, 1979; Vitiello, 2001 for a more complete presentation.
I finally recall that in the RU model and DQMB neurons and glia cells are described as classical systems. Jibu and Yasue (1995) and Vitiello (1995) identify the quantum variables with the vibrational modes of the water molecular electrical dipoles and the NG with the dipole correlation quanta, called dipole wave quanta (dwq). One important limitation of the RU model consists in the very limited memory capacity. Once a memory has been recorded, any successive stimulus produces a new condensation process, overprinting on the previously recorded memory, which thus is lost. The solution of the overprinting problem is obtained in DQMB by considering dissipation (Vitiello, 1995).
As said, in the RU model and DQMB, the external stimulus causes the SBS of the water molecular electrical dipoles, which is the rotational spherical symmetry (the symmetry group
The brain state before the recording of the information is then distinct from the brain state after information has been recorded. Thus, time-reversal symmetry is broken and the arrow of time appears, i.e. irreversibility, typical of dissipative systems.
In QFT in order to study an open, dissipative system one needs to include also the thermal bath (environment) as to study the closed system
The memory state
Let the NG mode, i.e. the dipole wave quanta (dwq), be denoted by
One can show (Vitiello, 1995) that for any
The condensate at
The set
In conclusion, we may have a huge number of distinct information, with distinct codes
Note that unitary inequivalence may be spoiled by the finiteness of the system size (Vitiello, 1995; Alfinito and Vitiello, 2000). We may have then possible transitions among differently coded vacua, producing “association” of memories, following a path of memories, a phenomenon commonly experienced (see also Section 3 on the association of memories). A recent novel discussion of the dynamic criticality in brain has been presented in (Re and Vitiello, 2020a, b). It has been indeed observed that the criticality of phase transitions is also enhanced in sleeping, meditation, under psychoactive substances, etc., whenever the openness of the brain is reduced. In such cases, a flow of images from memories may be experienced like in dreams and non-ordinary brain states (Re and Vitiello, 2020a, b). This is consistent with the proposal, within the DQMB, that the consciousness act lives in the dialog of the self with its Double (Vitiello, 1995, 2001). The physical grounds for the consciousness phenomenon are in the dissipative character of the brain dynamics and the coherent structure of the brain states. Partial or total lack of these conditions may lead to unconscious brain states.
Also note that the dwq effective mass, implied by the finiteness of the system size (boundary effects), introduces an excitation energy threshold, so that recall of memory requires energy equal or higher than the threshold one, and this may be then experienced as a “difficulty in recalling”. On the other side, it also guaranties a memory protection and stability mechanism against energy fluctuations (Vitiello, 1995).
The ground state of the brain at a time
In the DQMB, the observed formation of neuron assemblies in amplitude modulated (AM) synchronously oscillating patterns, in the beta and gamma frequency range, is described in terms of coherent condensation of dwq in the system ground state.
In this way the input associated ground state form an attractor and the landscape of the attractors (Abraham et al., 1978; Hilborn, 1994) constitutes the record of the brain perceptual experience. Any new perceptual stimulus either places the sensory system in an existing basin of attraction in the attractor landscape, selected by generalization (eliminating unnecessary details) and abstraction (association to a category), either, in the absence of the conveniently recognized attractor, generates a new attractor. This last one is not trivially added to the pre-existing attractor landscape; its introduction induces the general reorganization of the landscape.
In both cases, the perceptual experience acquires a meaningful value within the brain perceptual history. Knowledge is thus constructed as accretion of meanings, not just of bare information. Memory then appears to be memory of meanings, not of information. Observations suggest indeed that the brain combines through the action-perception cycles perceptual inputs with its past experiences, within its own expectations and intentionality frame, thus making the perceptual stimulus meaningful to its own individual experience. As Freeman observes3 (3“The result is a meaning-laden perception, a gestalt, that is unique to each individual. For a dog, the recognition of the scent of a fox may carry the memory of food and expectation of a meal. For a rabbit, the same scent may arouse memories of chase and fear of attack.” (Freeman, 1991)), the same input, e.g. an olfactory input, may be associated to different meanings in different subjects.
In its effort aimed to its most harmonious to-be-in-the-world, which is the essence of the aesthetic experience according to Desideri (Desideri, 1998; Vitiello, 2015a), and is described, e.g., in the Merleau-Ponty phenomenology (Merleau-Ponty, 1945), brain acts into the world by testing hypotheses formulated in the action-perception cycle and projecting them backward in time in the attempt of matching them with past experiences. In the dissipative model, cerebral activity (matter) is responsible of actions in forward time, while their mirrored in time images come from and control perception (this is mind, awareness).
It is thus the Double (the mind) that produces hypotheses on the world by constructing images (Freeman and Vitiello, 2016). However, brain activity and mental activity are definitely, permanently entangled, without any possibility of separation or disentanglement. Brain-mind do not represent a dual manifestation of a basic entity. They are one single dynamical entity.
I close this Section by recalling that ECoG provides evidence in sensory cortices of phase modulated patterns in the form of phase cones with outward or inward cyclic pulsations with or without rotational gradients (vortices). One thus observes converging (imploding) and diverging (exploding) wave packets (Freeman, 1991; Vitiello, 2015b; Freeman and Quiroga, 2013), corresponding thus to forward and backward time evolution of phase cone as
Let me now discuss the brain chaotic dynamics (Pessa and Vitiello, 2003, 2004) within the DQMB.
In brain functional activity, collections of millions of neurons (neuron assemblies) are observed to have chaotic behavior in their transitions from a synchronous oscillatory pattern to another one (Freeman, 1991). Since 1987 Walter Freeman and his collaborators have stressed that such a chaotic behavior is a characterizing feature of brain activity4 (4In Freeman words “The brain transforms sensory messages into conscious perceptions almost instantly. Chaotic, collective activity involving millions of neurons seems essential for such rapid recognition.”…“Our studies have led us as well to the discovery in the brain of chaos-complex behavior that seems random but actually has some hidden order. The chaos is evident in the tendency of vast collections of neurons to shift abruptly and simultaneously from one complex activity pattern to another in response to the smallest of inputs…This changeability is a prime characteristic of many chaotic systems… In fact, we propose it is the very property that makes perception possible. We also speculate that chaos underlies the ability of the brain to respond flexibly to the outside world and to generate novel activity patterns, including those that are experienced as fresh ideas….” (Freeman, 1991).) (Skarda and Freeman, 1987; Freeman, 1991) and their discovery has been subsequently confirmed and analyzed (Tsuda, 2001).
The set of states
Explicitly, the state
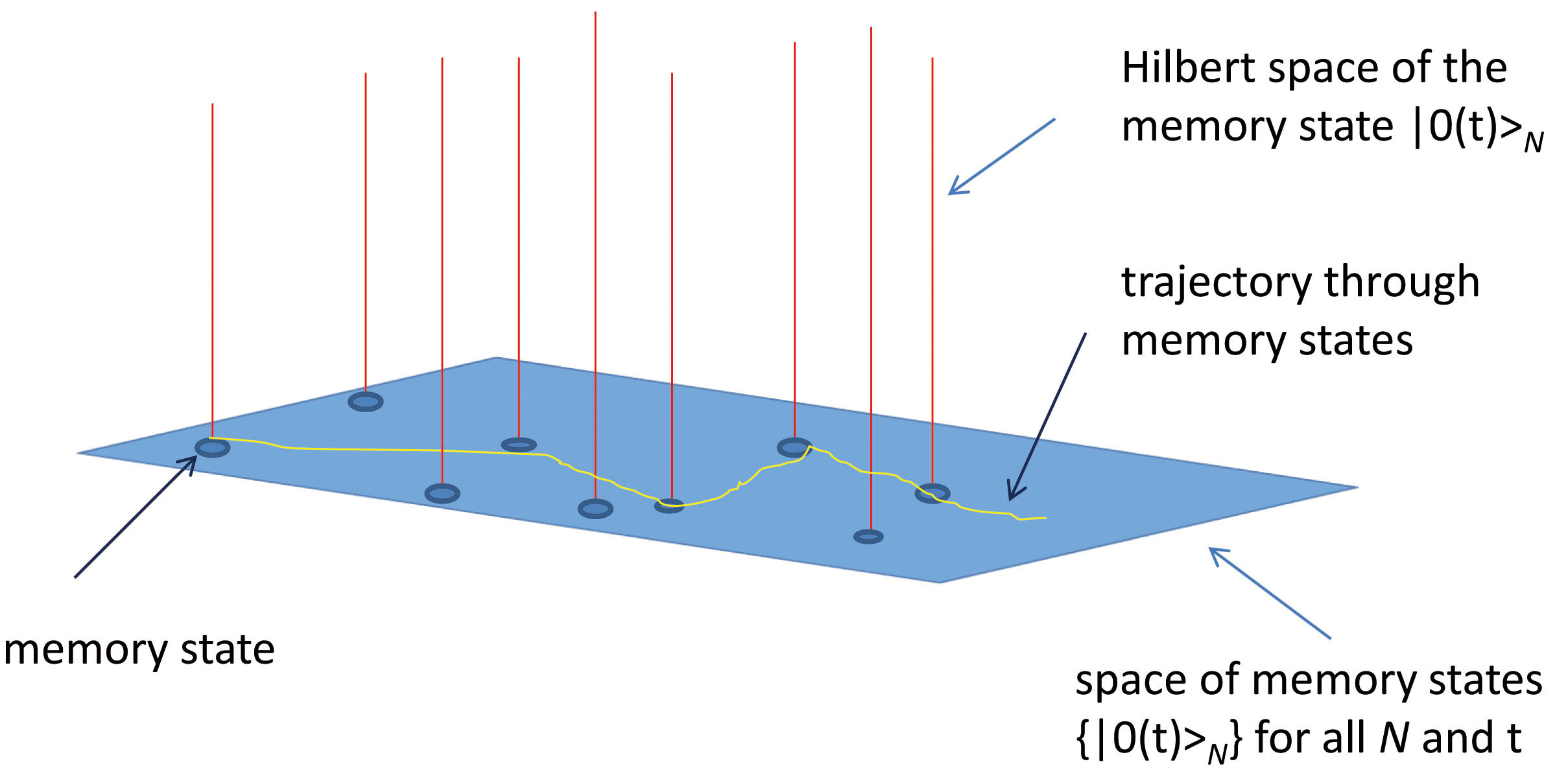
Schematic view of the space of the representations (set of Hilbert spaces) whose lowest energy (vacuum) state is the memory state
For
The difference between the components
Consider two sets
Suppose now that for small
Note that
We have seen that a central role in the RU model and DQMB is played by coherence. Memory states are indeed generalized
Using field theory in brain studies was already suggested in the forties by Lashley (1942), who reporting his laboratory observations was noticing the dominant role of masses of excitations and field activity in brain, almost indipendently from the particular nerve cells. However, only in 1967, brain was modeled as a many-body (QFT) system in the RU field theory model, further developed by Stuart et al. (1978, 1979), Sivakami and Srinivasan (1983), Jibu and Yasue (1995), Jibu et al. (1996). Finally, in 1995 the RU model was extended into the DQMB by considering the brain dissipative dynamics (Vitiello, 1995). Further studies of the brain dissipative dynamics have been pursued in the following years (Alfinito and Vitiello, 2000; Pessa and Vitiello, 1999, 2003, 2004; Vitiello, 2001, 2015a, 2015b; Freeman and Vitiello, 2006; Capolupo et al., 2013, 2017; Freeman et al., 2015; Re and Vitiello, 2020a, b) (for a review see Vitiello, 2017, 2018).
The relation between brain activity and mind activity has been discussed in the previous Sections and it has been stressed that brains construct “meanings” out of the perceptual inputs (information). Then, it has been shown that, in agreement with experimental observations (Freeman, 1990, 1996, 2000), trajectories in memory space exhibit chaotic behavior. Since tiny differences in the external inputs generate divergent, chaotic memory paths, high resolution in the perception of similar stimuli is obtained. Also, codes which are different only for some of their components forming a zero-measure set, may be recognized as being identical codes, which might turn to be useful, e.g., in pattern recognition in the presence of small perturbations or distortions.
Note that the stability of the entanglement between the
As mentioned in Section 2, criticality plays indeed a relevant role in brain activity. It is interesting that criticality has been found to be a specific feature of cellular (self-)organization, to the point that critical dynamic processes describe the same cell life (Pietruszka and Olszewska, 2020). In the case of the DQMB, criticality is intrinsic to the brain dynamics since we have seen it continuously undergoes phase transitions described by chaotic classical trajectories. Moreover, coherent patterns of molecular dipole fields may be driven, perturbed or changed by, e.g., a change of temperature, or a fluctuation around the critical temperature
In this connection, it is much interesting that a scale-free behavior (fractal self-similarity) has been observed in brain activity, e.g. obtaining log-log linear plots of PSD (Power Spectral Density) vs frequency, with fractal dimension depending on operational boundary conditions (Freeman et al., 2008; Freeman and Vitiello, 2009; Gireesh and Plenz, 2008). Similar signatures of fractal self-similarity with linear log-log PSD/frequency plots have been also observed in the study of ionic fluxes in living cell (specifically extracellular ionic fluxes for the ensemble of elongating pollen tubes of some plant cells), and have been related to criticality and coherent dynamics (Pietruszka and Olszewska, 2020). It is remarkable in fact that an isomorphism has been shown to exist between fractal structures and coherent states (Vitiello, 2012), so that the general feature of coherence in the DQMB finds a further consistent support in these observations of scale-free behaviors.
Finally, I observe that coherence plays in the dissipative quantum model the role of bridging two different approaches in brain studies: in the first approach, followed by the majority of researchers in neuroscience, a wide number of brain components are studied in their individual properties and theoretical and experimental data about each of them are accumulated. In the second approach the mathematical modeling tries to derive general macroscopic functional properties, usually out of reach of the first approach, from the microscopic dynamics of the brain system components. This second approach is complementary, not alternative to the first one. QFT investigates the basic dynamics underlying the rich phenomenology of the biochemical activity of neural cellular components.
There is then the question of whether a purely classical physics approach cannot do “the same trick” as QFT when it comes to the activity of clusters of neurons. The answer is that classical modeling so far has not provided the derivation of some macroscopic features of brain activity starting from the analysis at the microscopic level, such as, but not only, long range dynamical correlations waves spanning almost simultaneously large neuronal domains.
It is of course welcome any classical modeling able to reach such a task. However, commenting his experimental observations, Lashley (1942) tells us that: “Here is the dilemma. Nerve impulses are transmitted (…) from cell to cell through defined cell connections. Yet all behavior seems to be determined by masses of excitations (…) within general fields of activity, without reference to particular nerve cells (…). What kind of nervous organization can ever account for patterns of excitations without well-defined and specialized channels of cellular communication? The problem is almost universal in the activity of the nervous system”. About twentyfive years later, in 1967, the problem was still there. As already mentioned in the Introduction (cf. also the footnote 2), Ricciardi and Umezawa (1967) write: “…what is important is only a quantity somehow related to the activity of the whole cluster, which does not change appreciably as function of the number of alive neurons belonging to that cluster…the existence of similar and almost simultaneous responses in several regions of the brain (a kind of long-range correlation) to a particular stimulation technique does not find any explanation in terms of activity of the single nerve cells: new non-classical mechanisms have to be looked for…it is strongly suggestive of a quantum model. In other terms, one can try to look for specific dynamical mechanisms (already known in physics of many degrees of freedom) which can satisfy the essential requirements of the observed functioning of the brain”. They thus say with the words of Physics what Lashley said as neuroscientist.
The problem is therefore the formulation of a model able to explain the dynamical formation of long range correlations acting as collective modes as to provide “almost simultaneous responses in several regions of the brain” to a stimulation technique, able to “satisfy the essential requirements of the observed functioning of the brain”.
The available tools are the ones provided by many-body physics (QFT). They are the only ones we have when dealing with molecular charge distributions, such as electric dipole distributions characterizing all the molecules and bio-molecules involved in nervous cells. The quantum variables in the RU and the DQMB are in fact the vibrational modes of molecular electrical dipoles. In these models, as already remarked in Section 2, neurons and glia cells are indeed considered to be classical systems (Ricciardi and Umezawa, 1967; Jibu and Yasue, 1995; Vitiello, 1995). In this respect the RU model and the DQMB are drastically different from other quantum brain models proposed in the literature (for a general review see Atmanspacher (2020)).
As remarked in previous Sections, in QFT there exist infinitely many unitarily inequivalent state spaces (representations) and this fact offers the possibility of the SBS mechanism out of which long range correlation waves (and the associated NG quanta, the dwq) are dynamically generated. These are genuine QFT features, not existing in known classical models. Of course, since the aim is the understanding of the dynamical emergence of brain macroscopic activity out of its microscopic constituents, there is no competition between quantum and classical modeling. Any possible classical modeling able to provide the derivation, not just a description, of brain functional activity is mostly welcome. In the meantime, we adopt the tools which are today available and experimentally tested in a wide range of physical phenomena, including quantum behaviors in biological systems (Cao et al., 2020) and quantum matter-wave interference of large polypeptide bio-molecules recently observed (Shayeghi et al., 2020).
Apart the mentioned long range correlation waves almost simultaneously spanning large regions of the brain, the huge memory capacity, the dynamical protection of memories against reciprocal interference, the chaotic classical nature of the trajectory in memory space, the protection of memories against local destructive actions on few individual neurons, the stability mechanism against energy fluctuations, already mentioned in previous Sections, I will not insist here in listing further published results of the dissipative model of brain in agreement with experimental observations, which the interested reader may find in the literature (see, e.g., Freeman and Vitiello, 2010; Vitiello, 2016).
Thanks to all the peer reviewers and editors for their opinions and suggestions.
The authors declare no conflict of interest.