†These authors contributed equally.
Speech therapy has been widely used as an essential therapy for compensatory articulation errors in nonsyndromic cleft lip and palate patients. We sought to identify potential biomarkers of nonsyndromic cleft lip and palate children after speech rehabilitation based on resting-state fMRI and graph theory techniques. We scanned 28 nonsyndromic cleft lip and palate and 28 typically developing children for resting-state fMRI on a 3T MRI scanner. Functional networks were constructed, and their topological properties were obtained for assessing between-group differences (two-sample t-tests). Also, language clear degree scale scores were obtained for correlation analysis with the topological features in nonsyndromic cleft lip and palate patients. Significant between-group differences of local properties were detected in brain regions involved in higher-order language and social cognition. There were no significant correlations between topological feature differences and language clear degree scale scores in nonsyndromic cleft lip and palate patients. Graph theory provided valuable insight into the neurobiological mechanisms of speech rehabilitation in nonsyndromic cleft lip and palate patients. The global network features, small-world index, nodal clustering coefficient, and nodal shortest path length may represent potential imaging biomarkers for the estimation of effective speech rehabilitation.
Cleft lip and palate (CLP) can be divided into two types, syndromic CLP and nonsyndromic CLP (NSCLP), according to whether it is part of a well-known syndrome. NSCLP accounts for 70% of CLP with unknown etiology. Speech and resonance disorders due to velopharyngeal insufficiency (VPI), including hypernasality, nasal emission, and compensatory articulation errors, are the most common complications of NSCLP, with incidence rates ranging from 22% to 92% (Ruiter et al., 2009). Even following the physical management of VPI through surgery, 5%-50% of patients with CLP still suffer from compensatory articulation errors (Priester and Goorhuis-Brouwer, 2008; Taib et al., 2015). Therefore, speech therapy is often needed to correct abnormal placement that develops to compensate for VPI. Speech therapy involves establishing appropriate articulation placement and patterns with oral airflow using visual, auditory, and kinesthetic cues for feedback, and finally using principles of motor learning and motor memory for carryover into connected speech (Maas et al., 2008). However, the neuroplastic mechanisms stimulated by speech therapy in children with NSCLP are still unknown.
Neuroimaging studies have confirmed both neural structure and function abnormalities in CLP patients. Most of the previous studies of CLP children (age 7-17) have detected decreased brain volume both at the regional and whole-brain levels, indicating abnormal brain development in regions and measures including the ventral frontal cortex (Boes et al., 2007), subcortical grey matter and cerebral white matter (Adamson et al., 2014), total white matter, especially the frontal and occipital lobes (Van der Plas et al., 2010), cerebellum (Conrad et al., 2009), cerebrum and cerebellum preferential frontal lobe and subcortical nuclei (Nopoulos et al., 2007). However, two studies have reported abnormally increased cerebral volumes. Nopoulos et al. (2010) observed an enlarged volume of the right ventromedial prefrontal cortex (vmPFC), with the higher hyperactivity/impulsivity/inattention score in the boys with CLP, which indicated pathological enlargement of the vmPFC. Adamson et al. (2014) reported abnormally increased cerebral cortex grey matter volumes in children with NSCLP, which suggested an immature or modified developmental trajectory. Functionally, using the fMRI technique, Becker et al. (2008) found abnormal alterations of brain activity in CLP patients. A lexical processing task-related fMRI study in children with unilateral CLP revealed delayed and elongated blood-oxygen-level-dependent (BOLD) response in brain regions such as the cerebrum, prefrontal cortex, cingulate gyrus, right precuneus, and right temporal gyrus (Becker et al., 2008).
Moreover, a recent subvocalization task fMRI study in adult CLP patients following palatoplasty and speech rehabilitation documented similar functional activation patterns between the CLP group and healthy controls, except for an increased activation in the left hippocampus (Zhang et al., 2017). Overall, these structural and functional studies indicate that the pathological state of the cleft in children (age 7-17) impaired brain development and function, especially in the language-related and cognitive brain areas. We hypothesized that after successful surgery, the appropriate speech therapy would induce neuroplasticity in the NSCLP children during the process of correcting speech and resonance errors. It is currently unclear whether there are critical patterns in the functional networks of the developing brains of CLP children following speech rehabilitation.
The resting-state fMRI (rs-fMRI) technique has been broadly applied in speech studies, such as bilingualism (Berken et al., 2016), developmental stuttering (Yang et al., 2016), and speech apraxia (New et al., 2015). Graph theory is a method that assesses the topological properties of network organizations from rs-fMRI data for both healthy subjects and clinical patients (Medaglia, 2017). Based on resting-state BOLD signals characterizing physiological information on spontaneous brain function (Biswal et al., 1995), the small-worldness and local and global metrics of resting-state brain networks can be assessed through graph theory. The small-world network has an optimum equilibrium between local specialization and global integration to process information (Latora and Marchiori, 2001). The local parameters include nodal shortest path length and nodal clustering coefficient. The nodal shortest path length may ensure active integration and fast information transmissions across remote nodes (Wang et al., 2009). The nodal clustering coefficient is used to estimate nodal participation within local information integration (Medaglia, 2017). Small-worldness,local and global metrics have been identified not only in neurological diseases, such as Alzheimer’s disease (Zhang et al., 2015) but also in healthy people, such as with the comprehension of sign language (Liu et al., 2017). Therefore, the current work proposes to evaluate the similarities of the topological parameters of resting-state functional networks between speech rehabilitated NSCLP children and healthy controls using graph theory.
A total of 28 children (age = 10.0 ± 2.3 years) with NSCLP and 28 age- and sex-matched typically developing children were recruited for the current study from the Beijing Children’s Hospital and Beijing Stomatological Hospital. 19 NSCLP children had the only palatoplasty, and the other 9 patients had VPI surgery followed by palatoplasty. The patient inclusion criteria were as follows: (1) NSCLP patients started speech therapy in the outpatient department in 3-6 months after a successful pharyngeal closure surgery with age 6-16 (mean 9.5), with a frequency of 30 min/day and 3 times/week, lasting for half a year. Once the Chinese language clear degree scale (CLCDS) scores of patients reached the critical score of 86 (full credit is 100 points, and 86 is considered as a clear line of rehabilitation), the subjects were given MRI scans; (2) right-handed; (3) Chinese as their dominant language; (4) normal vision and hearing (results of auditory brainstem response below 30 dB nHL); (5) Normal intelligence (the results of Full-Scale Intelligence Quotient (FSIQ) using the Chinese Wechsler Intelligence Scale for Children-Ⅳ more than 90). The exclusion criteria of the patients were following: children with clinic diagnoses of (1) dysarthria; (2) velopharyngeal anatomy or structure deficit; (3) speech disorder (CLCDS scores < 86) (4) hearing and/or vision impairments; (5) developmental delays; (6) congenital disorders; (7) other syndromes or possible; and (8) other chronic health diseases.
All MRI images were obtained through the 3.0 T GE MRI system at the Department of Radiology (Beijing Children’s Hospital). For each subject, rs-fMRI and high resolution T1-weighted anatomical images were acquired. By echo planner imaging sequence, resting-state data were obtained with participants maintaining their eyes closed while being wakeful. The scan parameters of echo planner imaging were as follows: TR/TE: 2000/35 ms, FA: 90°, matrix size: 64 × 64, voxel size: 3.5 × 3 .5 × 3.5 mm. Structural images of the brain were acquired by high-resolution 3D spoiled gradient sequence: 164 continuous sagittal slices, TR/TE: 8.196/3.156 ms, FA: 13°, slice thickness: 1 mm, matrix size: 256 × 256, voxel size: 0.47 × 0.47 × 1 mm.
The acquired DICOM data were analyzed with the GRETNA (Wang et al., 2015) and SPM12 toolbox (http://www.fil.ion.ucl.ac.uk/spm/) based on MATLAB. Briefly, resting-state data were pre-processed with the following measures: (1) the first ten time points were deleted; (2) correction of slice timing for the time offsets between slices; (3) rigid body correction for head motion; (4) spatial normalization for MNI space, 3 × 3 × 3 mm resample with children’s EPI template; (5) linearly detrend; (6) 0.01 ~ 0.10 Hz filtering; (7) linear regression for the head movement, white matter, CSF and global signals. No subjects were removed due to significant head motion (3 mm and/or 3°) during the scan.
Using the GRETNA toolbox, the resting-state brain networks of all subjects were constructed. First, all subjects’ 200 × 200 temporal correlation matrices were obtained through calculating the correlation coefficient (Pearson) of the temporal series between every couple of the 200 brain regions (Harvard-Oxford Cortical Structural Atlas) for every subject (Craddock et al., 2012). During this procedure, the mean temporal series were computed by averaging all voxels’ temporal series from each brain region. The between-region correlation coefficient values were considered as the weighted edges for the graph. Thus, a weighted function connection matrix (200 × 200) was acquired for every subject. For examining the properties of resting-state brain networks based on the function connection matrix, a series of threshold values were applied to provide the same number of edges of each graph. Our study adopted 0.05-0.5 threshold values (step = 0.01), and the parameters of the related graphs at every threshold value were assessed (Zhang et al., 2011).
All the subjects’ topological parameters of the resting-state brain networks were estimated with the GRETNA software. The topological parameters of the network were assessed as follows:
2.5.1 Local node metrics
Local node metrics included nodal shortest path length and nodal clustering coefficient. The nodal shortest path length is the average distance between a given brain region and the rest of all brain regions, describing the capability of information transmission in parallel (Wang et al., 2009). The nodal clustering coefficient is defined as the existing connections of the brain region’s first neighbors divided by all their possible connections, which measures the brain region’s ability to participate in local information integration (Medaglia, 2017).
2.5.2 Global network metrics
Global network metrics included the characteristic path length (Lp), network clustering coefficient (Cp), network global efficiency (Eg), and local efficiency (Eloc) (Wang et al., 2015). Lp is the average shortest path lengths through all possible couples of brain regions of the network, and Cp is the average clustering coefficient of all brain regions of the resting-state network (Watts and Strogatz, 1998). Eg is defined as the average inverse shortest path length, describing the average minimum travel length between brain regions in the resting-state network; Eloc is the averaging subgraphs’ global efficiencies, and the subgraph consists of a given brain region’s close neighbors (Latora and Marchiori, 2001). The weighted Cp and Eloc indicate the segregation of the functional brain network, and the Lp and Eg imply the integration of the functional brain network.
2.5.3 Small-world parameters
The network clustering coefficient (Cp) and characteristic path length (Lp) were compared with the clustering coefficient and shortest path length of a set of random networks (n = 1000), providing a normalized clustering coefficient (γ) and normalized path length (λ), respectively. The small-world index (σ) is the ratio of γ to λ (σ > 1 for small-worldness), evaluating the balance of network integration and segregation (Watts and Strogatz, 1998).
The areas under the curve (AUCs) of topological features, which were integral over the whole density range, were computed. Using age and sex as covariates, statistical assessment of the between-group differences of topological properties and their AUCs were performed by using a series of two-tailed two-sample t-tests. Also, the correlations of the topological parameters with the CLCDS score were calculated. In addition, the false discovery rate (FDR) correction (corrected P < 0.02) was applied for multiple comparison corrections.
The resting-state networks were constructed, and the local, global scale and small-world parameters were evaluated for both NSCLP patients and healthy controls over the density range of 0.05-0.50 (step = 0.01).
The mean nodal values of the two groups were projected onto the cortical surface (see Fig. 1). Compared with healthy controls, NSCLP patients exhibited lower nodal shortest path length detected in the left frontal pole, right occipital pole, and bilateral cingulate gyrus (see Table 1, Fig. 2A and 3A-D). They demonstrated a higher nodal clustering coefficient located in the left frontal pole, superior frontal gyrus, cingulate gyrus, paracingulate gyrus, and bilateral temporal poles (P < 0.05, see Table 1, Fig. 2B and 3E-L).
Reg | Coordinates in RPI | NcpAUC | NlpAUC | Harvard-Oxford Cortical Structural Atlas | ||
---|---|---|---|---|---|---|
x | y | z | t, P | t, P | ||
5 | -7 | 48 | 7 | 2.474, 0.041 | left Paracingulate Gyrus | |
32 | 42 | 12 | -38 | 2.080, 0.040 | right Temporal Pole | |
43 | -40 | 8 | -40 | 2.270, 0.022 | left Temporal Pole | |
46 | 1 | -37 | 31 | -2.020, 0.030 | right Cingulate Gyrus | |
55 | 0 | 18 | 32 | 2.252, 0.003 | -2.206, 0.034 | left Cingulate Gyrus |
91 | 0 | 54 | 25 | 2.591, 0.005 | left Superior Frontal Gyrus | |
133 | -10 | 49 | 39 | 2.591, 0.039 | left Frontal Pole | |
142 | 17 | -88 | 21 | -2.106, 0.034 | right Occipital Pole | |
167 | -27 | 54 | 22 | 2.077, 0.019 | -2.109, 0.029 | left Frontal Pole |
183 | -28 | 59 | 4 | 2.312, 0.037 | left Frontal Pole |
Reg is the code of the Harvard-Oxford Cortical Structural Atlas. Ncp: nodal clustering coefficient, Nlp: nodal shortest path length. AUC: area under the curve.
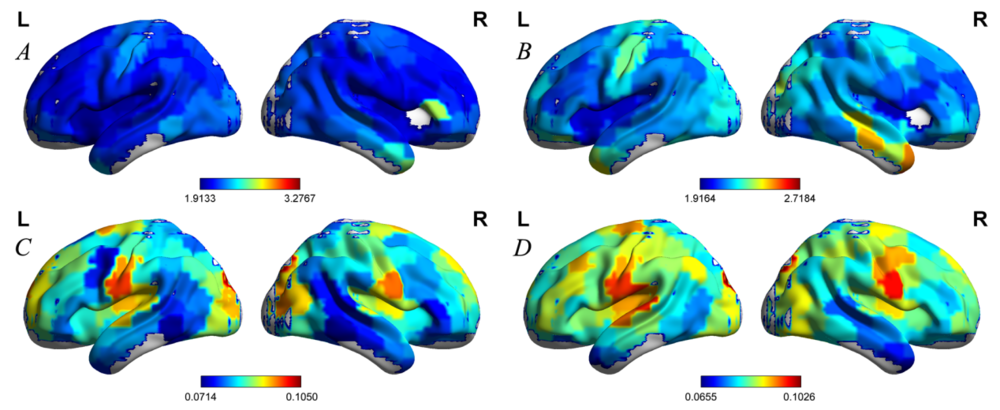
The projection of nodal values onto the cortical surface. A and B indicated the mean nodal shortest path length of the NSCLP patients and healthy controls, respectively. C and D indicated the mean nodal clustering coefficient of the NSCLP patients and healthy controls, respectively.
Note. The color represented the nodal values.
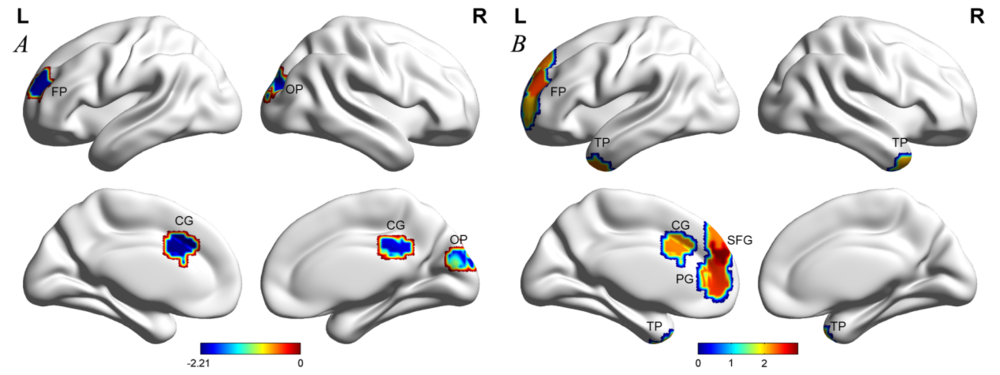
The projection of significant between-group differences in the AUC of the nodal parameters. (A) Shortest path length; (B) Clustering coefficient.
Note. Color represented T values. They were corrected for age and gender. Two-sample two-tailed t-test. AUC: area under the curve. FP: frontal pole, OP: occipital pole, SFG: superior frontal gyrus, PG: paracingulate gyrus, CG: cingulate gyrus, TP: temporal pole.
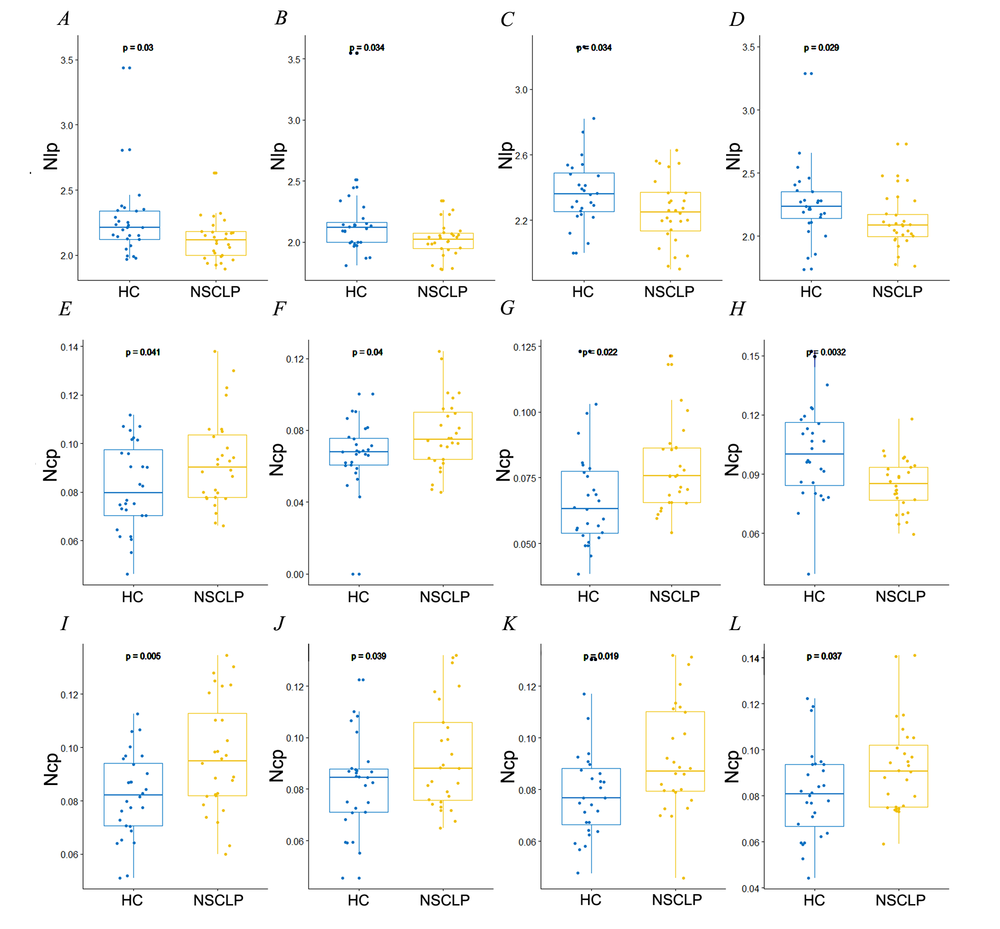
Significant differences in nodal shortest path length (A-D) and cluster coefficient (E-L) between the NSCLP patients and healthy controls. Nlp: nodal shortest path length, Ncp: nodal clustering coefficient. (A) Right cingulate gyrus; (B) Left cingulate gyrus; (C) Right occipital pole; (D) Left frontal pole; (E) Left paracingulate gyrus; (F) Right temporal pole; (G) Left temporal pole; (H) Left cingulate gyrus; (I) Left superior frontal gyrus; (J) Left frontal pole (Reg 133); (K) Left frontal pole (Reg 167); (L) Left frontal pole (Reg 183).
Note. HC: healthy controls, NSCLP: NSCLP patients. They were corrected for age and gender. Two-sample t-test, P < 0.05.
There were no significant between-group differences for CpAUC, LpAUC, EgAUC, and ElocAUC network values, or Cp, Lp, Eg, and Eloc values of all threshold network (FDR correction P < 0.02, see Fig. 4A-D).
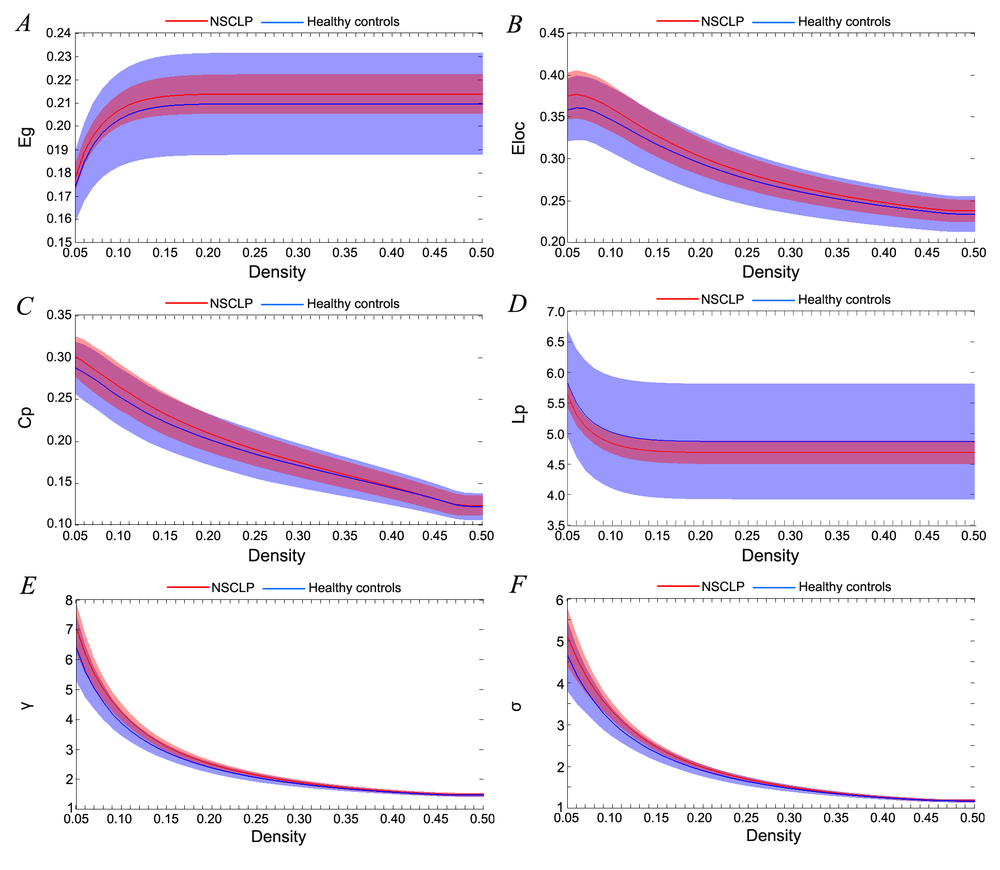
A (Eg), B (Eloc), C (Cp), and D (Lp) showed no significant between-group differences. E (γ, 0.05-0.17) and F (σ, 0.06-0.24) showed significant between-group differences.
Note. Shaded areas represented the standard deviation of the mean. They were corrected for age and gender. Two-sample two-tailed t-test. FDR correction P < 0.02.
At all threshold values, the brain networks of both groups demonstrated a small-world organization over the whole range of threshold values (σ > 1, see Fig. 4F). Furthermore, a two-sample two-tailed t-test indicated there were higher σ (density 0.06-0.24), γ (density 0.05-0.17), σAUC (t = 3.33, P = 0.0018) and γAUC (t = 3.64, P = 0.0008) in speech rehabilitated NSCLP patients (σ and γ FDR correction P < 0.02, see Fig. 4E-F and Fig 5).
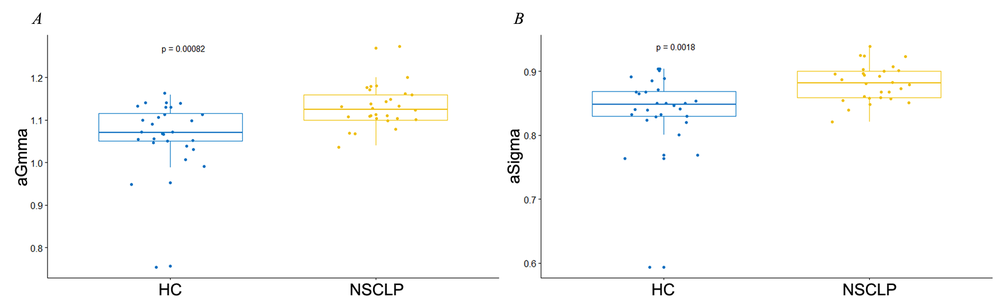
The between-group differences of the AUC of the Gamma (γ) and Sigma (σ). (A) aGamma: the AUC of the γ (γAUC); (B) aSigma: the AUC of the σ (σAUC).
Note. HC: healthy controls, NSCLP: NSCLP patients. The columns and error bars corresponded to the mean values and their standard errors. They were corrected for age and gender. Two-sample two-tailed t-test. AUC: area under the curve.
The mean value of CLCDS was 91.6 (standard deviation 4.0) in the speech rehabilitated NSCLP children. Based on using the between-group differences, topological parameters of the nodes showed no statistical relationship with CLCDS scores (FDR correction P < 0.02).
This the first study to use rs-fMRI to investigate the topological structure of resting-state networks in NSCLP patients following speech rehabilitation. The significant findings can be generalized as follows: (i) the differences of local node metrics were mostly affected in brain regions associated with higher-order language and social cognition; (ii) for the global network metrics, there were no significant between-group differences among Cp, Lp, Eg, and Eloc; (iii) brain networks of the speech rehabilitated NSCLP group exhibited different small-world topology and higher σ and γ values compared with healthy controls.
Our results demonstrate that local metric differences were observed in brain regions associated with higher-order language and social cognition. The decreased nodal shortest path length and increased nodal clustering coefficients were located in the left frontal pole and cingulate gyrus in NSCLP patients following speech rehabilitation. However, a significantly decreased volume of the frontal lobe has been reported in children with CLP (Boes et al., 2007; Nopoulos et al., 2007; Van der Plas et al., 2010). Delayed and elongated BOLD responses have been identified in the prefrontal cortex and cingulate gyrus of CLP children in a lexical processing task-related fMRI study (Becker et al., 2008). Both structural and functional MRI studies have confirmed that there were abnormal structures and functions in the frontal lobe and cingulate gyrus in CLP children. The left frontal pole is associated with integrating information across diverse levels and effective goal-directed behavior (Orr et al., 2015).
Furthermore, the frontal pole participates in the system for “driving of speech” (Kinoshita et al., 2015) and human social cognitive function (Boes et al., 2007). The cingulate gyrus is involved in action-outcome learning (Rolls, 2015) and assessing the spatial- context- and personal-relevance of sensory information (Bar and Aminoff, 2003). Our results indicate that the different local metrics of the left frontal pole and cingulate gyrus in speech rehabilitated NSCLP patients describe the enhanced ability of local information propagation and integration of language and cognitive functions (Medaglia, 2017), which may be related to speech training in CLP patients. Zhang et al. (2017) found increased activation only in the left hippocampus for the adult CLP patients after palatoplasty and speech rehabilitation. The cingulate gyrus carries information from the hippocampus, which facilitates memory, navigation, and cognition and participates in stable and correct speech production (Lisman et al., 2017). The strengthened functional connection between the hippocampus and cingulate gyrus (describing decreased nodal shortest path length and increased nodal clustering coefficient) may mediate the speech rehabilitation process, consistent with the present results.
The right occipital pole exhibited decreased nodal shortest path length in the speech rehabilitated NSCLP patients. The volume of the occipital lobes was particularly low in the CLP children (Van der Plas et al., 2010), which implied that the defect had a significant effect on development. The occipital pole, the most posterior part of the occipital lobe, is in the ventral visual pathway (Freud et al., 2016) and reading circuitry (Price, 2000; Wandell and Le, 2017), and Amedi et al. (2004) found that the occipital pole is associated with verbal processing. The results suggest that the integration of reading and verbal processing was strengthened, which may be induced by speech therapy in CLP children.
We also observed increased nodal clustering coefficient at the left superior frontal and paracingulate gyrus and right temporal pole in the speech rehabilitated NSCLP children compared with healthy controls. However, these brain regions are smaller in volume or exhibit delayed and elongated BOLD response in CLP children (Becker et al., 2008; Nopoulos et al., 2007; Van der Plas et al., 2010), which suggests structural and functional abnormalities in these brain regions. The left superior frontal gyrus is involved in the dorsal stream of phonological processing (Fujii et al., 2016). The left paracingulate gyrus is associated with positive memories (Vogt, 2014). Furthermore, the temporal pole is a significant language center involved in semantic and syntactic processing (Mandonnet et al., 2007). The results indicate that local information integration increased at the left superior frontal and paracingulate gyrus and right temporal pole in the speech rehabilitated NSCLP patients, which may be associated with local information integration of language processing during the speech therapy, including visual, tactile, auditory and action feedback (Pamplona et al., 2017; Yano et al., 2015).
Surprisingly, compared with healthy controls, speech rehabilitated CLP children showed no significant differences in the global network metrics of Cp, Lp, Eg, and Eloc. A subvocalization task fMRI study reported similar functional activation patterns between the adult CLP patients after palatoplasty and speech rehabilitation and healthy controls (Zhang et al., 2017), which is consistent with the present results. Previous studies suggest that network efficiency (Eg and Eloc) is often disrupted by changes in path length (Bassett and Bullmore, 2009), and cognitive function might rely on distant connections (Lp) (Markov et al., 2013). Furthermore, Bassett and Bullmore (2009) concluded that functional network topological properties are often abnormal in clinical disorders. Our results indicate that the functional integration and differentiation of speech rehabilitated CLP patients were similar to healthy controls, which were consistent with the typical clinical speech data for children with NSCLP, based on the CLCDS.
Small-worldness was detected in both groups (σ > 1), which implies the optimum equilibrium of network segregation and integration, consistent with Crone’s study of chronic disorders of consciousness (Crone et al., 2014). Furthermore, compared with healthy controls, the brain networks of NSCLP children exhibited increased γ and σ. The increased γ value in NSCLP children after speech correction indicated more efficiency for local information transfer, which suggested a stronger local specialization for speech training (Watts and Strogatz, 1998). However, there were no significant between-group differences in normalized characteristic path length (λ), which reflected the long-distance connection of the network and described the information transmission and integration capacity of the network. The transmission and integration of information are the foundations of cognitive processing. Therefore, the NSCLP-related λ value might reflect the restructuring of neuronal integration among distant regions that were associated with rehabilitative cognitive functions (measured with CLCDS scores). The functional connection of language-related brain regions decreases in developmental stuttering (Yang et al., 2016) and speech apraxia (New et al., 2015) but increases in bilingualism (Berken et al., 2016), which support the present data. The increased σ value (σ = γ/λ) in rehabilitated CLP patients reflects a more economical parallel information transfer and the optimum equilibrium of functional specialization and integration compared with healthy controls (Wang et al., 2009). Wolff et al. (2017) demonstrated that cognitive load is associated with a small-world index. The increased small-world index may be caused by increased nodal clustering coefficient and decreased nodal shortest path length in higher-order language and social cognition brain regions, which strengthen local function connections. In the present study, the difference in the small-world index indicates that the balance in the NSCLP networks was not the same as in healthy controls, making their networks more in favor of efficiency for local phonologic information transfer attaching to a new balance. Speech therapy established a familiar but non-identical language network that included a strengthened relationship between language-related functional areas with different cognitive processing roles such as vision, hearing, and behavior.
The present study has some limitations. First, the number of subjects in both groups was relatively small, and replication will be needed to make meaningful conclusions related to areas where differences were identified. Second, more work is needed on the diversity of the topological functional network organization in CLP children without surgical or speech therapy. Third, we maximized the exclusion of inherently auditory, visual, intellectual, and syndromic effects on the brain, and the acquired effect of pathological speech correction was considered the main factor. However, we could not control for all inherent and acquired factors, such as genetic and other environmental factors. Fourth, longitudinal studies of the alterations identified in language-related areas and networks in rehabilitated CLP patients should be conducted.
Compared with healthy controls, speech rehabilitated CLP children exhibited similar global topological features and an increased small-world index. Also, nodal metric differences were identified in brain regions associated with higher-order language and social cognition.
BR: Methodology, Software, Formal analysis, Writing, and Original Draft. HC: Writing - Review & Editing, Project administration. YF: Validation, Data Curation. WZ: Investigation Resources. RC: Funding acquisition. YP: Supervision.
Research approval was acquired from the Beijing Children’s Hospital ethical committee (IEC- C-028-A10-V.05), and signed informed consent was obtained for all subjects.
This research was supported in part by the National Natural Science Foundation of China (81671651), Beijing Municipal Administration of Hospitals Clinical Medicine Development of Special Funding Support (code XMLX201714), and the Cultivate Plan of Beijing Municipal Administration of Hospital (PX2018047).
The authors declare that they have no conflicts of interest.