- Academic Editor
†These authors contributed equally.
Background: Uterine corpus endometrial carcinoma (UCEC) is a prevalent gynaecologic malignancy. It has been demonstrated that the immune cell infiltration (ICI) pattern plays a critical role in the tumour progression of UCEC. Methods: To further investigate the immune microenvironment landscape of UCEC, we analysed the gene expression data of 539 UCEC patients from The Cancer Genome Atlas (TCGA) database using CIBERSORT and ESTIMATE for consensus clustering of immune cells. We used the limma package to compare differentially expressed genes (DEGs) among ICI patterns and constructed a prognostic model using Cox regression to calculate the risk score of UCEC patients. The immunophenoscore was downloaded to explore the immunotherapeutic effect between low- and high-risk score patients. Finally, the tumour mutation burden (TMB) was calculated using the somatic mutation data. Results: We identified two different immune infiltration patterns in 539 UCEC samples, the immune-desert and immune-inflamed phenotypes, which had distinct prognostic and biological features. We obtained 29 DEGs to construct the ICI-related prognostic model and established a four ICI-related gene-based prognostic model comprising LINC01871, CXCL13, IGKJ5, and LINC01281. The risk score was associated with distinct clinical outcomes, ICI, and immunotherapeutic effects. Patients with a low risk score had higher effective immune cells, which could be classified into the immune-inflamed phenotype. Additionally, patients with a low risk score had a significantly higher immunophenoscore, suggesting a better immunotherapeutic outcome. Finally, TMB was confirmed to be associated with prognosis, which was synergistic with the risk score. Conclusions: This study comprehensively analysed the ICI pattern in UCEC patients and established a four ICI-related gene-based prognostic model to predict prognosis and guide precise immunotherapy strategies.
Uterine corpus endometrial cancer (UCEC) is a prevalent type of malignant tumour in gynaecology. According to global cancer statistics in 2020, the incidence of endometrial cancer was 4.5%, ranking sixth among women’s cancers, and its morbidity and mortality increase annually [1]. In 2020, it was estimated that there would be 65,620 new cases and 12,590 deaths [2]. In recent years, the incidence and mortality of UCEC have risen rapidly, particularly in association with obesity and diabetes [3]. The poor prognosis of UCEC is related to both the molecular subtype and clinical stage. In 2013, The Cancer Genome Atlas (TCGA) Research Network classified UCEC into four molecular subtypes based on genomic and transcriptomic data: polymerase epsilob (POLE) ultramutated, microsatellite instability hypermutated (MSI-H), copy-number low (CNL) and copy-number high (CNH). The POLE ultramutated subtype was found to have a better prognosis, whereas the CNH subtype had the poorest outcome [4]. Patients diagnosed at later stages are more likely to experience recurrence and metastasis and have a poor prognosis. The 5-year survival rate for relapsed patients is 68%, while that for metastatic patients is only 17% [5]. Although traditional treatments, such as surgical resection, chemotherapy, and radiotherapy, have been greatly improved, overall survival (OS) has been not significantly improved. Therefore, a novel prognosis prediction and therapeutic response assessment method are urgently needed to facilitate precision and individualized treatment.
The tumour microenvironment (TME) consists of an extracellular matrix secreted by tumour cells, stromal cells, endothelial cells, immune cells, and tumour-associated cells. The high heterogeneity of TME phenotypes and cancer cell genotypes is one of the factors contributing to clinical treatment sensitivities and outcomes among tumour patients [6]. TME components interact with tumour cells and regulate their growth and development. In recent years, increasing evidence has shown that the occurrence, development, and metastasis of malignant tumours are associated with the TME [7, 8, 9]. The infiltration of immune cells and their interaction with cancer cells form a unique tumour immune microenvironment (TIME) [10]. The immune cell infiltration (ICI) in the TIME plays a decisive role in patient prognosis. Many studies have shown that there is significant ICI in UCEC tissue, and these immune cells are involved in tumour development [11]. It has been demonstrated that patients with high infiltration of CD8+ T cells and CD45RO+ T cells have a better prognosis in UCEC, whereas patients with high infiltration of tumour-associated macrophages have a worse prognosis [12]. Moreover, ICI has also been reported to play an important role in immunotherapy for UCEC [13, 14]. Therefore, a deeper understanding of the landscape of ICI in UCEC may improve the precision of prognosis prediction and reveal optimal treatment strategies.
In this study, we explored the ICI landscape of 539 UCEC samples from the TCGA database. We aimed to identify immune-associated subtypes by unsupervised clustering of 22 infiltrating immune cells in UCEC and constructed a prognostic risk model based on the differentially expressed genes (DEGs) among the ICI subgroups. By analysing the correlation between risk score and molecular subtype, clinical stage, immune score, immune checkpoint, and tumour mutation burden (TMB), we aim to predict which UCEC patients may benefit from immunotherapy. In summary, our results imply that ICI is of great help for tumour immunotherapy, and our ICI-related prognostic model can provide a basis for selecting precision immunotherapy strategies in UCEC patients. The design and procedures are shown in Fig. 1.
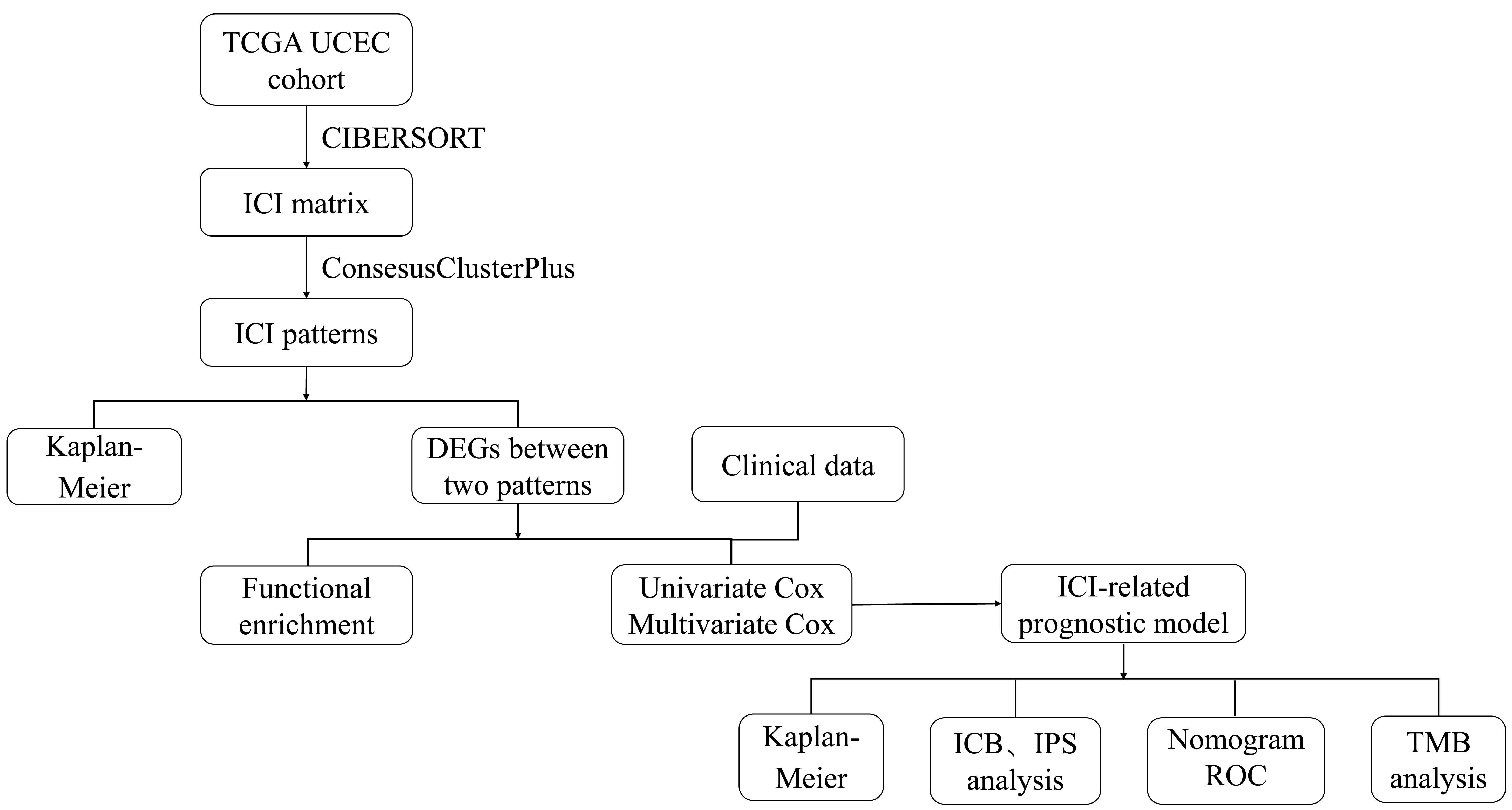
The workflow of the study. TCGA, The Cancer Genome Atlas; UCEC, uterine corpus endometrial carcinoma; ICI, immune cell infiltration; DEGs, differentially expressed genes; ICB, immune checkpoint blockade; IPS, immunophenoscore; TMB, tumour mutation burden; ROC, receiver operating characteristic.
We obtained the transcriptomic data and corresponding clinical characteristics of UCECs from the TCGA database, consisting of a total of 583 UCEC samples. We excluded normal samples, duplicate samples, and samples with missing survival times, leaving us with 539 samples for analysis. The transcriptomic profiles of TCGA-UCEC in the fragments per kilobase per million (FPKM) were transformed into transcripts per kilobase million (TPMs).
We applied the CIBERSORT package (http://cibersort.stanford.edu/) to obtain a fraction matrix of ICI using the TPM data, which estimated the abundances of 22 tumour infiltration immune cells (TIICs) [15]. The ESTIMATE algorithm was used to infer the tumour purity and calculate the immune score and stromal score. Additionally, single-sample gene set enrichment analysis (ssGSEA) was performed on UCEC samples using the GSVA package (http://www.bioconductor.org/) based on the expression levels of immune-related markers. We then used the “ConsensusClusterPlus” package (http://www.bioconductor.org/) to divide the UCEC samples into two subgroups. To ensure the stability of the classification, the analysis process was repeated 1000 times using the unsupervised clustering “Pam” method. We used the “pheatmap” R package (https://cran.rstudio.com) to draw the heatmap.
We divided UCEC samples into two ICI clusters based on the consensus clustering
algorithm. Differentially expressed genes (DEGs) between the different ICI
subgroups were identified by using the “limma” package. The significance
cut-off was set as an adjusted p value
The “survival” package was used to perform univariate Cox regression analysis
to identify DEGs related to the OS of UCEC patients. Then, we conducted
multivariate Cox regression to construct an ICI-related prognostic risk model,
and the risk score was calculated by the following formula for each UCEC patient.
Risk score =
According to the molecular classification method based on TCGA [16], we divided the 539 UCEC patients into four molecular subtypes: POLE, MSI-H, CNL and CNH. To investigate the correlation between the risk score and molecular subtypes, we generated box plots using GraphPad Prism v8.0.2 software (GraphPad Software, Inc., San Diego, CA, USA) and performed unpaired t tests to determine statistical significance. We used the same method to examine the relationship between risk score and clinical stage, survival status, and age groups.
We divided the 539 UCEC patients into two groups based on the median risk score. To explore the correlation between the risk score and TIME, we used the Wilcox test and drew box plots to demonstrate the difference in TIME between the low- and high-risk groups.
Research has demonstrated that the expression levels of certain key genes related to immune checkpoint blockade (ICB) may be correlated with the clinical outcomes of immune checkpoint inhibitor blockade therapy. To investigate the potential role of the risk score in immunotherapy, we analysed the correlation between the risk score and the expression levels of ICB-related genes. Here, we focus on the six key ICB genes, namely, PD-L1 (CD274), PD-1 (PDCD1) [17], PD-L2 (PDCD1LG2), TIM-3 (HAVCR2), CTLA-4 [18], and IDO1 [19], that have been reported in previous studies. Additionally, we further compared the correlation between nine inflammation-related genes (TNF, TBX2, PRF1, IFNG, CD8A, CLCL9, CXCL10, GZMA, and GZMB) [20] and the risk score. To evaluate the potential impact of immunotherapy predicted by the risk score, we also analysed the correlation between the immunophenoscore (IPS) and the risk score of UCEC patients. The IPS data for UCEC patients are available for download from The Cancer Immunome Atlas (TCIA).
To enhance the interpretability of the prognostic risk model and facilitate patient evaluation, we developed a nomogram using the “rms” R package, which integrates the risk score and age to estimate OS at 1, 3, and 5 years. The nomogram assigns a score to each factor based on their respective contribution to the outcome variable and sums them to obtain the total score. To validate the prognostic value of the nomogram, we used calibration and receiver operating characteristic (ROC) curves.
To reveal the intrinsic link between the TIME and somatic mutations, we conducted an analysis to assess the correlation between TMB and risk score. Somatic mutation data were obtained from the TCGA database, and the “maftools” package (http://www.bioconductor.org/) was utilized to calculate the number of somatic nonsynonymous point mutations in each sample, which corresponded to the TMB. Furthermore, we generated separate waterfall plots for the low- and high-risk groups to visually display the distribution of gene mutations among patients in each risk score group.
All statistical analyses were conducted using R version 4.1.0 (University of Auckland, Auckland, New Zealand). The fraction of 22 TIICs was calculated using the “CIBERSORT” package in R. The Wilcoxon test was used to compare differences between two groups. DEGs were identified using the “limma” package in R. Survival analysis was performed by the Kaplan-Meier (K-M) method, and comparisons between groups were made using the log-rank test. The “survivalROC” and “timeROC” packages were used to generate the ROC curve. GSEA was performed using GSEA software version 4.1.0 (Broad Institute, Inc., Cambridge, MA, USA).
To further analyse the TIME in UCEC, we calculated the level of 22 immune cells in a total of 539 samples. Based on the ICI profile, the 539 samples were classified into different subtypes using the “ConsensusClusterPlus” package. To ensure sample equilibrium and clustering stability, we chose k = 2 (Supplementary Fig. 1), resulting in the division of samples into two ICI patterns, ICI Cluster A (329 samples) and ICI Cluster B (210 samples). We drew a comprehensive heatmap to further describe and explore the relationship between ICI patterns and clinical phenotypes, including age and survival status (Fig. 2A). Furthermore, we conducted Kaplan‒Meier survival analysis to compare the prognosis of the two ICI subgroups and found that ICI Cluster B had a better prognosis than ICI Cluster A, although the difference was not statistically significant (p = 0.31, Fig. 2B).
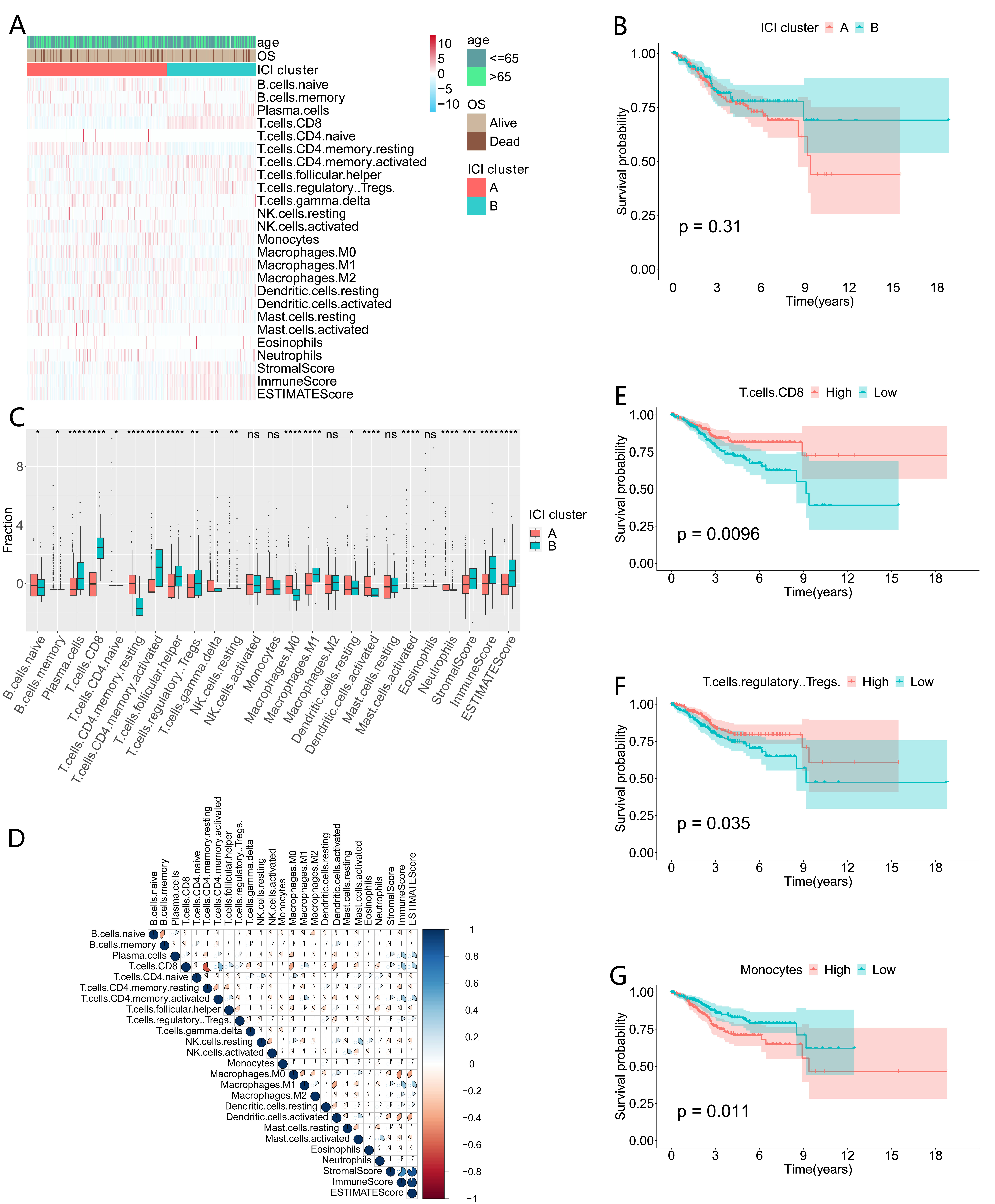
The landscape of ICI in UCEC. (A) Heatmap of immune cells in UCEC
patients. (B) K-M curves for the OS of UCEC patients in two ICI clusters. (C) The
levels of immune cells, immune scores, and stromal scores in two ICI clusters.
(D) Correlation of immune cells and immune scores. (E–G) OS analysis between
patients with different infiltration levels of immune cells. (E) CD8+ T cells.
(F) Regulatory T cells. (G) Monocytes. *p
To further investigate the association between immune scores and infiltrating immune cells, we compared the TIME between the two clusters. Our analysis revealed that ICI Cluster B displayed higher levels of plasma cells, CD8+ T cells, activated state CD4+ T cells, follicular helper T cells, regulatory T cells, and M1 macrophages, as well as high stromal and immune scores. These findings are consistent with previous studies showing that the abundance of these immune cells significantly correlates with antitumour immune status [21]. Furthermore, we compared the correlations between immune cells and the immune score, stromal score, and ESTIMATE score (Fig. 2C,D). Among these immune cells, plasma cells, CD8+ T cells, activated-state CD4+ T cells, regulatory T cells, and M1 macrophages were significantly and positively correlated with immune scores. Therefore, we defined ICI Cluster B as immunoinflammatory. In contrast, most immune cell infiltrates were significantly lower in ICI Cluster A, except for resting-state CD4+ T cells, M0 macrophages, activated-state dendritic cells, higher levels of neutrophils, and lower stromal and immune scores. Resting-state CD4+ T cells, M0 macrophages, and activated-state dendritic cells were significantly and negatively correlated with immune scores. Therefore, ICI Cluster A can be considered the immune desert type.
To further assess the value of ICI subgroups in predicting prognosis, we conducted Kaplan‒Meier survival analysis based on the different ICI subgroups. The results showed that regulatory T cells and CD8+ T cells enriched in ICI Cluster B were associated with a longer survival time and a good prognosis (Fig. 2E,F). In contrast, a high level of monocytes, which were enriched in ICI Cluster A, showed a poor prognosis (Fig. 2G).
Furthermore, we compared the expression levels of six key immune checkpoint blocking genes (PD-1, CTLA-4, TIM-3, IDO1, PD-L1, and PD-L2) in the two ICI clusters (Fig. 3). Our analysis revealed that the expression levels of ICB-related genes were significantly higher in ICI Cluster B than in ICI Cluster A, suggesting that ICI cluster B may be more suitable for immunotherapy.
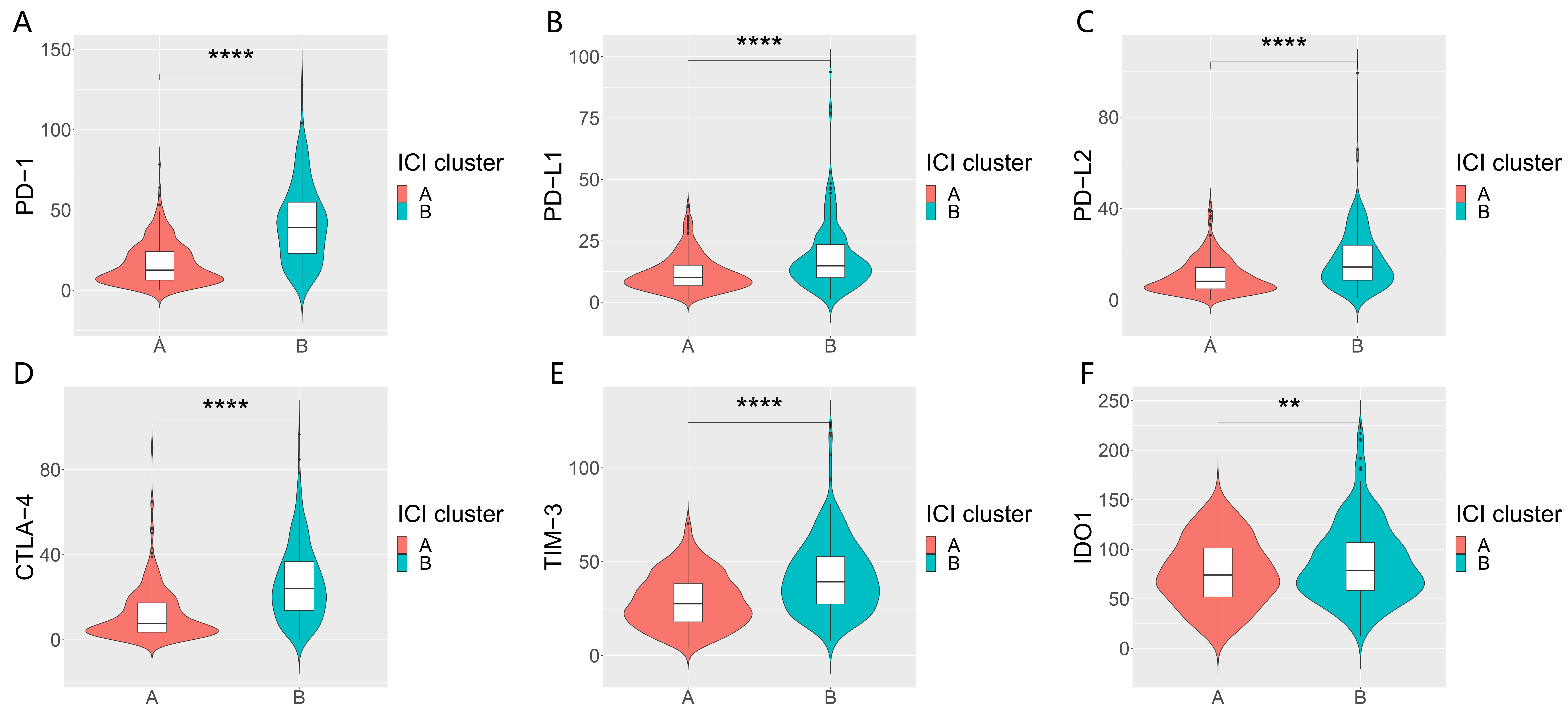
The expression levels of six ICB genes in two ICI clusters. (A)
PD-1. (B) PD-L1. (C) PD-L2. (D) CTLA-4. (E)
TIM-3. (F) IDO1. **p
Using the limma package, we identified 29 DEGs between the two ICI clusters,
which were defined as ICI-related genes (Table 1). We performed GO enrichment
analysis with the ICI-related genes and found that most biological functions were
related to the immune response, such as immunoglobulin production, humoral immune
response, immunoglobulin complex, and immunoglobulin receptor binding
(Supplementary Fig. 2). We then performed univariate Cox and
multivariate Cox regression analyses to identify the ICI-related genes associated
with OS and to establish a prognostic risk model. Finally, an optimal prognostic
model was obtained: risk score = (0.9842
Gene | logFC | AveExpr | t | p value | adj.p.Val | B |
IFNG | 0.690138 | 4.619117 | 13.77466 | 3.43 |
3.16 |
73.68758 |
LINC02446 | 0.642649 | 4.549493 | 12.79338 | 6.62 |
2.16 |
63.93433 |
LINC01871 | 0.536552 | 4.811053 | 12.56105 | 6.49 |
1.63 |
61.68009 |
RTP5 | 0.510671 | 4.106001 | 12.02844 | 1.11 |
1.81 |
56.59841 |
CXCL13 | 0.564699 | 5.388636 | 11.63992 | 4.38 |
5.64 |
52.97128 |
LINC01281 | 0.51517 | 4.183521 | 11.33138 | 7.71 |
8.70 |
50.14149 |
IGLC6 | 0.555786 | 4.302542 | 10.47021 | 1.79 |
1.31 |
42.49629 |
IGKV1.8 | 0.612746 | 4.640868 | 9.63965 | 2.16 |
1.11 |
35.50488 |
IGKV1D.12 | 0.537981 | 4.131287 | 9.47581 | 8.36 |
3.86 |
34.17319 |
IGLV3.1 | 0.547847 | 5.174836 | 9.304659 | 3.38 |
1.38 |
32.79948 |
IGLC7 | 0.587786 | 4.819901 | 9.109792 | 1.62 |
6.01 |
31.25746 |
IGHV1.24 | 0.552303 | 5.045554 | 8.980839 | 4.51 |
1.55 |
30.25016 |
IGKV1.12 | 0.512237 | 4.152039 | 8.842155 | 1.34 |
4.24 |
29.17869 |
IGKV1.9 | 0.529306 | 5.143034 | 8.767901 | 2.39 |
7.12 |
28.6101 |
IGKV1D.16 | 0.517931 | 4.363243 | 8.593826 | 9.17 |
2.40 |
27.29129 |
IGHV3.20 | 0.550608 | 4.550816 | 8.549534 | 1.29 |
3.22 |
26.95891 |
IGLV1.36 | 0.535547 | 4.607999 | 8.54105 | 1.37 |
3.40 |
26.89539 |
IGKJ5 | 0.520335 | 4.383708 | 8.474194 | 2.28 |
5.53 |
26.39655 |
IGHV2.70 | 0.571032 | 4.700231 | 8.465907 | 2.43 |
5.84 |
26.33492 |
IGLV7.43 | 0.517652 | 4.898322 | 8.364099 | 5.23 |
1.23 |
25.58161 |
IGHV1.69D | 0.527484 | 5.041317 | 8.31834 | 7.37 |
1.66 |
25.2453 |
IGLV3.27 | 0.539226 | 4.720764 | 8.213847 | 1.60 |
3.38 |
24.48266 |
IGKV1.17 | 0.511528 | 5.01979 | 8.088337 | 4.04 |
8.00 |
23.57654 |
IGKV1.16 | 0.503234 | 5.066696 | 8.022742 | 6.51 |
1.26 |
23.10732 |
IGHV1.3 | 0.515697 | 4.404484 | 7.997288 | 7.84 |
1.48 |
22.92604 |
IGLV9.49 | 0.531905 | 4.728355 | 7.870241 | 1.96 |
3.51 |
22.02805 |
IGHV1.69 | 0.511032 | 4.838856 | 7.788804 | 3.50 |
5.95 |
21.45843 |
IGLV4.60 | 0.520134 | 4.652707 | 7.764731 | 4.16 |
6.93 |
21.29095 |
IGKV2D.29 | 0.510528 | 4.673165 | 7.564385 | 1.70 |
2.51 |
19.91324 |
logFC, log(fold change).
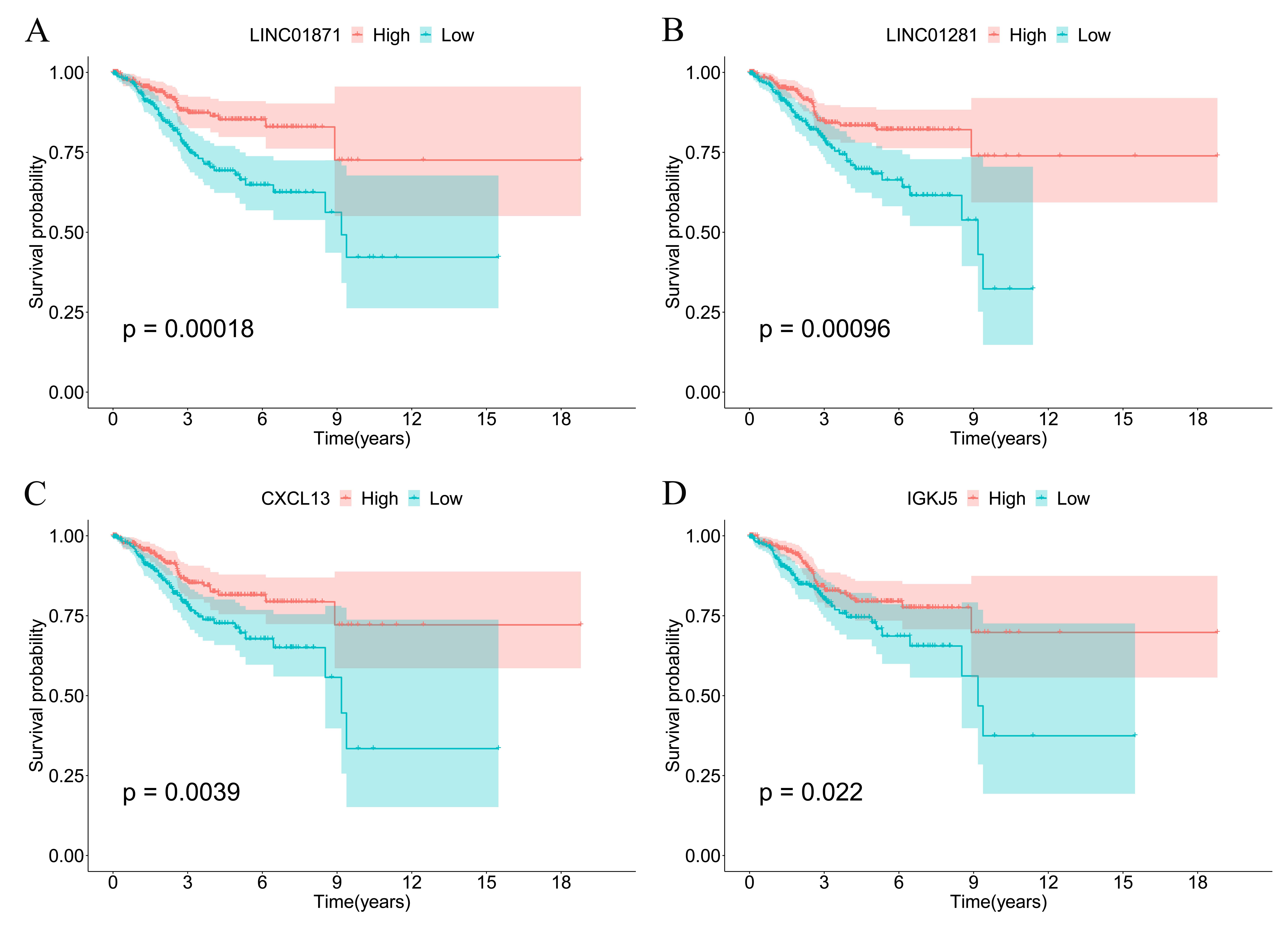
Kaplan‒Meier OS curves of four ICI-related genes. (A) LINC01871. (B) LINC01281. (C) CXCL13. (D) IGKJ5. Patients with high expression of ICI-related genes showed a good prognosis (red curves), while patients with low expression of ICI-related genes showed a poor prognosis (blue curves).
To further validate the prognostic role of risk scores, risk curves and scatter
plots were constructed according to the survival status of each sample (Fig. 5A,B). The risk curves and scatter plots visualized the risk scores and survival
status of TCGA-UCEC patients, illustrating that patient death was closely
associated with high-risk scores. Patients were divided into high- and low-risk
groups with the median risk score as the cut-off. The Kaplan‒Meier survival curve
showed that the low-risk group had a longer survival time (p
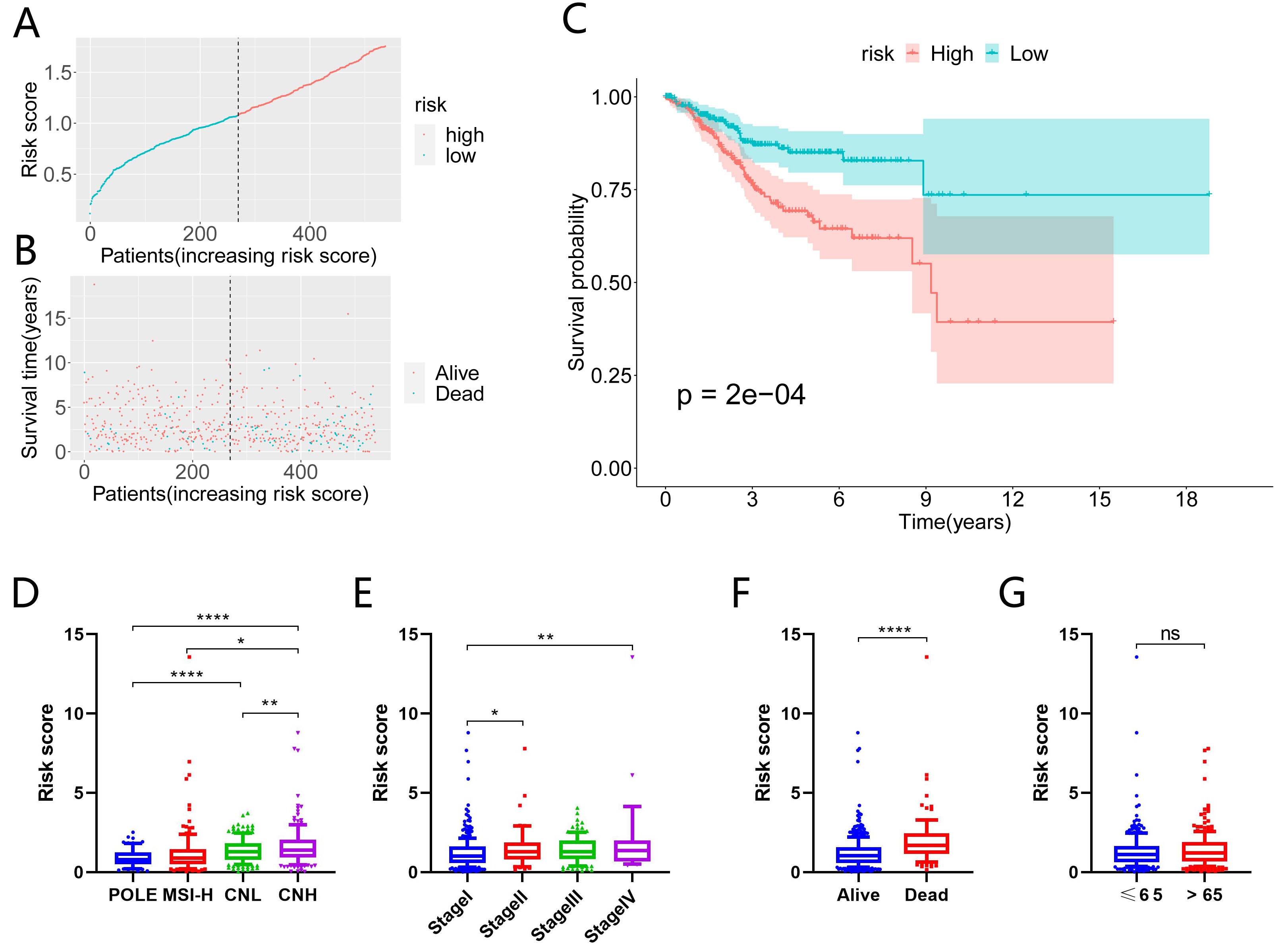
Evaluation of the ICI-related prognostic model. (A) Distribution of the
risk score for each patient. (B) Survival status for each patient. Red points
correspond to surviving patients, and blue points correspond to deceased
patients. (C) Survival analysis between patients with different risk scores. (D)
Risk score in different molecular subgroups. (E) Risk score in different clinical
stages. (F) Risk score in the surviving and deceased groups. (G) Risk score in
different age groups. *p
As the risk score was calculated by the ICI-related prognostic model, we further explored the differences in the tumour immune environment between the high- and low-risk groups. Our results showed that the risk score was negatively correlated with the level of various immune cells closely related to the antitumour immune response, including plasma cells, CD8+ T cells, activated state CD4+ T cells, follicular helper T cells, regulatory T cells, and M1 macrophages (Fig. 6A). Patients with low risk scores had higher stromal scores, immune scores, and ESTIMATE scores than patients with high risk scores (Fig. 6B). The ssGSEA results showed that both ICI and immune signal enrichment were significantly higher in the low-risk group than in the high-risk group (Fig. 6C). The heatmap exhibited the enrichment of the immune features of the patients in the high-risk and low-risk groups correspondingly (Fig. 6D). Based on these results, the high-risk and low-risk groups exhibited significantly different ICI phenotypes. Patients with low risk scores showed an immune-inflamed phenotype with a large number of immune cell infiltrates, while patients with high risk scores showed an immune desert phenotype with less immune infiltration. These findings indicated that the risk score was closely related to the TIME and may play an important role in immunotherapy.
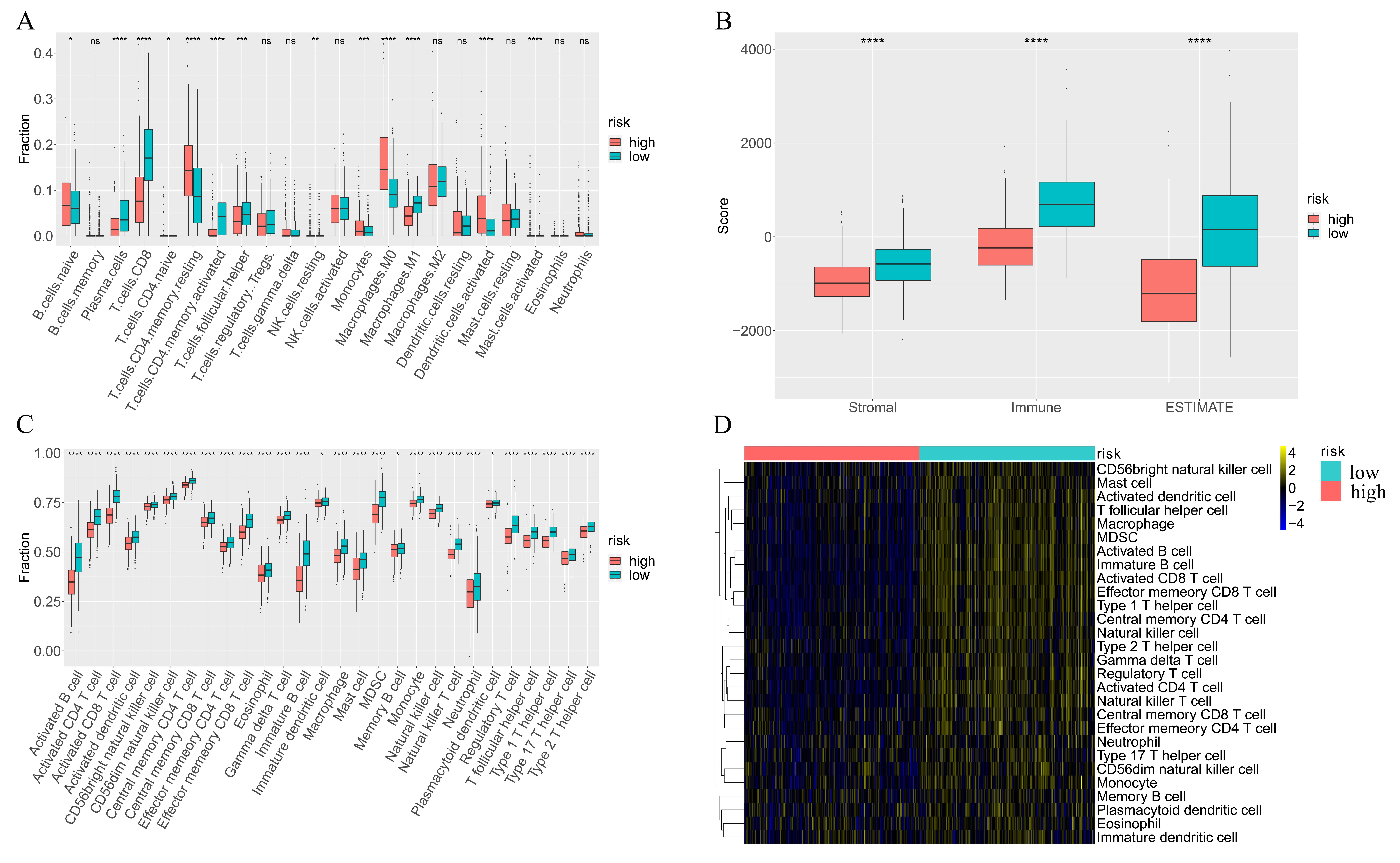
Association between the risk score and TIME characteristics. (A) Immune cell infiltrating abundances in the low- and high-risk score groups. (B)
Differences in stromal score, immune score, and ESTIMATE score between the low-
and high-risk score groups. (C) Difference in immune functions between the low-
and high-risk score groups. (D) Heatmap of immune cell infiltrating abundances
between the low- and high-risk score groups. *p
In this study, we analysed ICB-related genes and inflammation-related genes to assess tolerance and immunoreactivity in patients with different risk scores (Fig. 7A). Almost all genes were significantly upregulated in patients with a low risk score, except for TBX2 and TNF. These results imply that patients with a low risk score have better tolerability and immunoreactivity. To further reveal the potential role of the risk score in the ICB treatment of UCEC patients, we examined the correlation between the six key ICB genes and the risk score and drew pie charts (Fig. 7B). We found that the expression of all six genes was significantly negatively correlated with the risk score (Fig. 7C–H). These results suggest that the risk score may play an important role in predicting the response to ICB treatment in UCEC patients.
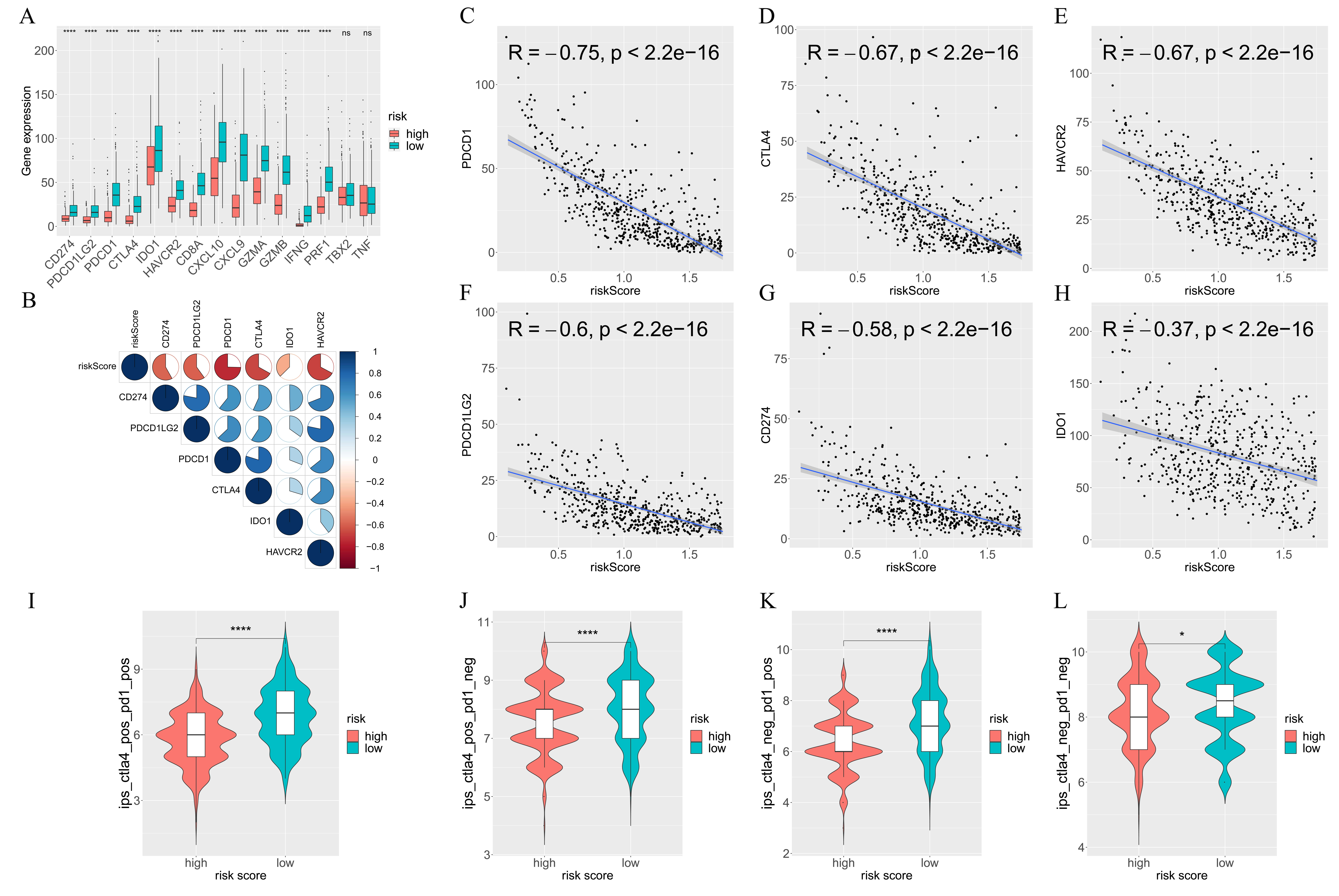
Correlation of risk score with immunotherapy. (A) Differences in the
expression levels of ICB-relevant genes (CD274, PDCD1LG2,
PDCD1, CTLA4, IDO1, and HAVCR2) and
inflammation-related genes (CD8A, CXCL10, CXCL9,
GZMA, GZMB, IFNG, PRF1, TBX2, and
TNF) between the low- and high-risk score groups. (B–H) Correlation
between the risk score and immune check inhibitors. (I–L) Difference in the IPS
score between the low- and high-risk score groups. *p
Two subtypes of IPS, IPS-PD1-positive and IPS-CTLA4-positive, were used as proxies for immunotherapy response in UCEC patients to assess the effect of immunotherapy predicted by risk scores (Fig. 7I–L). Our results showed that the IPS-CTLA4 and PD1 blockade score, IPS-CTLA4 blockade score, IPS-PD1 blockade score, and IPS score were significantly higher in the low-risk group. This indicates that patients with low risk scores are more suitable for immunotherapy.
We constructed a nomogram to predict the 1-, 3-, and 5-year OS of UCEC samples using the risk score and age (Fig. 8A). To validate the performance of the prognostic nomogram, we plotted calibration curves (Fig. 8B–D). Furthermore, we drew an ROC curve to further evaluate the constructed prognostic model, and the area under curve (AUC) values of 1-, 3-, and 5-year OS were 0.642, 0.658, and 0.716, respectively (Fig. 8E). These results indicate that the model had high sensitivity and specificity.
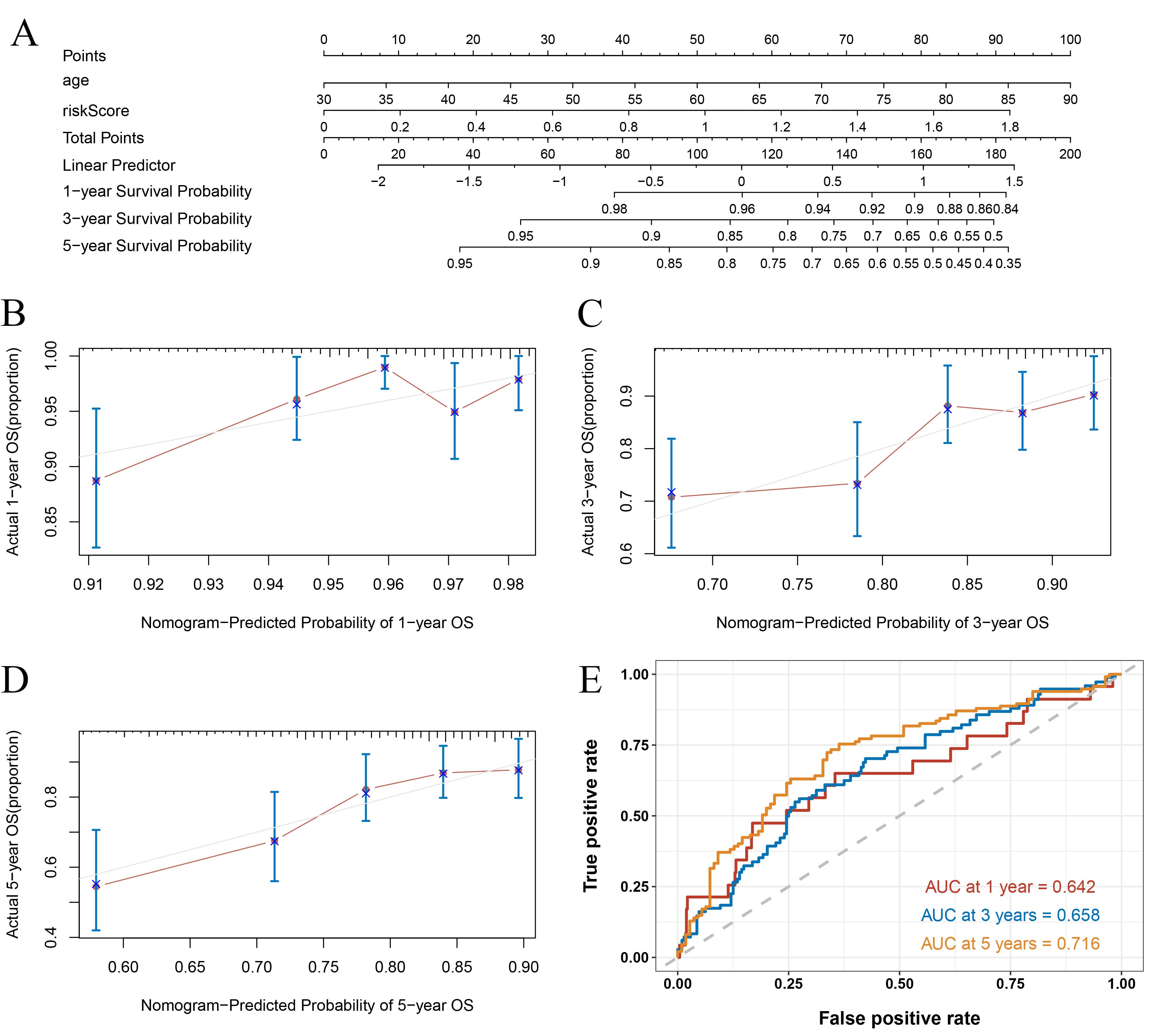
Evaluation of the risk score in prognosis. (A) A nomogram was constructed using age and risk score to predict the OS of UCEC patients. (B) 1-year nomogram calibration curves. (C) 3-year nomogram calibration curves. (D) 5-year nomogram calibration curves. (E) ROC curves for 1-, 3-, and 5-year survival. OS, overall survival; UCEC, uterine corpus endometrial carcinoma; ROC, receiver operating characteristic; AUC, area under curve.
It has been reported that high levels of TMB are associated with high infiltration of CD8+ T cells [22]. CD8+ T cells recognize tumour neoantigens and induce an immune response to destroy tumour cells. Therefore, TMB may serve as a prognostic factor for the effectiveness of the response to antitumour immunotherapy. In our study, we assessed the TMB levels in patients with both low and high risk scores and found that patients with a low risk score had a higher TMB (Fig. 9A). Additionally, our correlation analysis revealed a significant negative correlation between TMB and the risk score (Fig. 9B), indicating that a lower risk score may be associated with higher TMB and thus with a better response to antitumour immunotherapy.
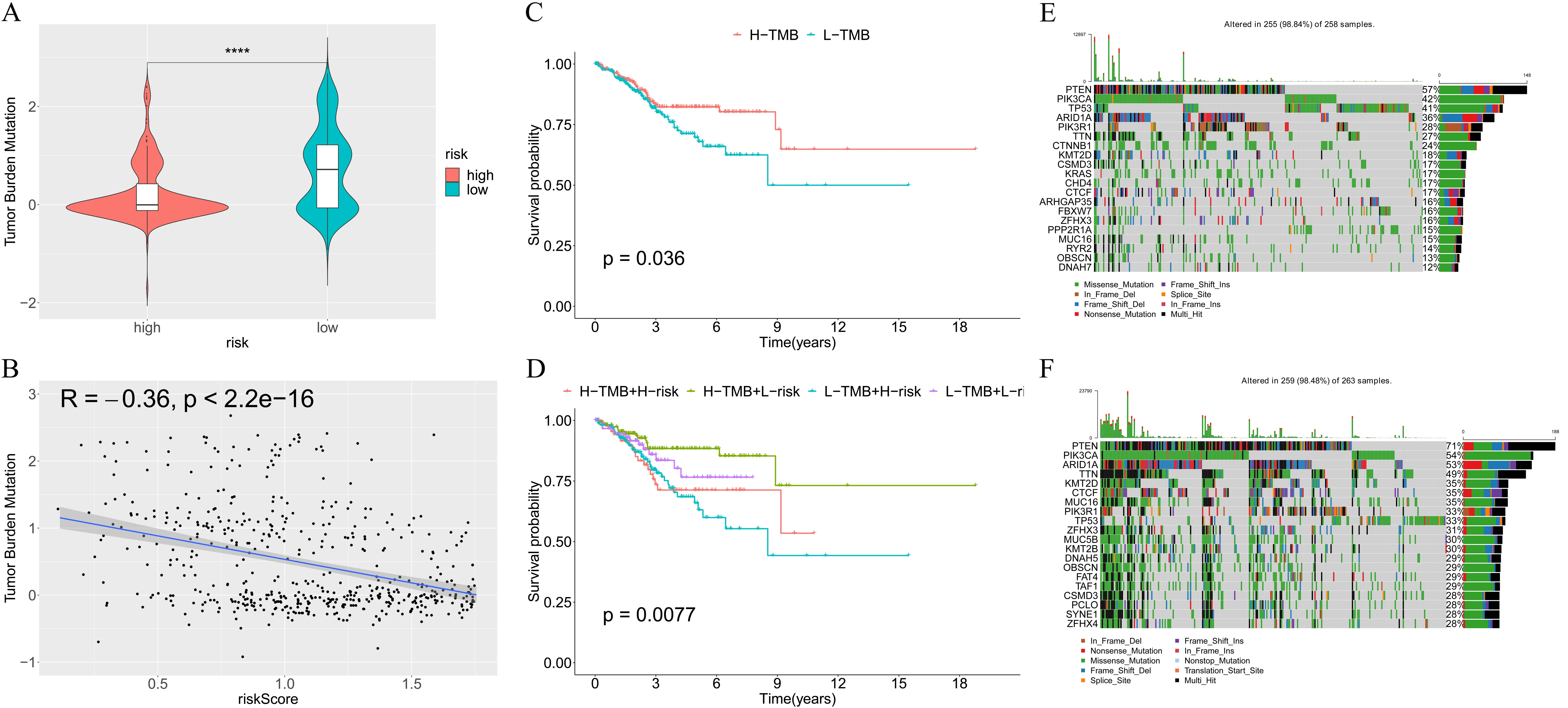
Correlation between the risk score and TMB. (A) Difference in TMB
between the low- and high-risk score groups. (B) Scatterplots depicting the
negative correlation between risk score and TMB. (C) Survival analyses for
patients with low and high TMB using K-M curves. (D) Survival analyses for
patients stratified by both the risk score and TMB using K-M curves. The
waterfall plot of tumour somatic mutations was constructed using the high-risk
score (E) and low-risk score (F). ****p
Then, we divided the patients into two groups based on their TMB levels. Kaplan‒Meier survival analysis showed that patients with high TMB had a greater probability of survival (Fig. 9C). To further assess the prognostic value of the risk score and TMB, we evaluated the synergistic effect of these two factors in predicting the survival of patients with UCEC (Fig. 9D). Patients with both a high TMB and low risk score exhibited the best survival probability, while patients with a low TMB and high risk score had the worst survival probability. These findings suggest that the risk score may serve as an independent prognostic factor and may be utilized to predict the clinical outcome of antitumour immunotherapy.
We also analysed the distribution of mutations between the low- and high-risk groups. The integrated somatic mutation landscape was used to visualize the mutation patterns of the top 20 most frequently mutated driver genes (Fig. 9E,F). The mutation profile of significantly mutated genes showed a higher mutation rate of TP53 (41% vs. 33%) in the high-risk group and a higher mutation rate of TTN (49% vs. 27%) and KMT2D (35% vs. 18%) in the low-risk group. These findings provide deeper insight into the intrinsic link between the risk score and somatic mutations in UCEC immunotherapy.
Endometrial cancer is a prevalent morbidity and malignant tumour in females. Its occurrence and development are regulated by various mechanisms, many of which are related to the TME [23]. The high heterogeneity of tumour microenvironmental phenotypes and cancer cell genotypes leads to different clinical treatment sensitivities and distinct clinical outcomes. Therefore, we need to seek a new treatment strategy to increase host antitumour immunity during esophageal cancer (EC) treatment. Immune-based antitumour therapy has made significant breakthroughs in a variety of cancers [24], and studies have demonstrated that infiltrating immune cells have an important impact on tumour progression and antitumour immunity [25, 26]. This study aimed to analyse the immune microenvironment of UCEC through omics data and construct the first ICI-related prognostic model to monitor the prognosis and response to immunotherapy in UCEC patients.
Previous studies have shown that the level of immune cells associated with immune activation affects the clinical outcome of immunotherapy [27, 28]. This study verified that the prognosis of UCEC is related to the immune microenvironment and found that patients with high levels of CD8+ T cells and regulatory T cells have a better survival. Furthermore, the immune microenvironment of UCEC can be divided into two different types: immune-inflamed phenotype and immune-desert phenotype. We can calculate the immune scores of different phenotypes to predict the prognosis of UCEC. This is the first study to establish immune microenvironment typing of UCEC, which is expected to be used in immunotherapy.
This study divided the 539 samples into two groups with distinct immune phenotypes. The K-M curves showed that ICI Cluster B had better OS than ICI Cluster A, although this difference was not statistically significant. This may be due to the limitation of the sample size. ICI Cluster B had high levels of multiple immune cells, including CD8+ T cells, regulatory T cells, activated state CD4+ T cells, follicular helper T cells, and M1 macrophages, with high stromal and immune scores. These scores have been reported to be significantly correlated with antitumour immune status [18]. Therefore, we defined ICI Cluster B as an immune-inflamed phenotype. However, we defined ICI Cluster A as an immune-desert phenotype, with low stromal and immune scores.
In addition, we calculated the DEGs between ICI Cluster B and ICI Cluster A to
obtain ICI-related genes. The results of the GO enrichment analysis based on the
DEGs were mainly related to the immune response, demonstrating that the DEGs are
associated with immunotherapy. We then constructed a prognostic model to
calculate the risk score of UCEC patients based on four ICI-related genes
(LINC01871, LINC01281, CXCL13, and IGKJ5).
K-M survival analysis showed that the expression of the four genes was
significantly associated with a longer survival time. LINC01871 has been used to
predict the prognosis of various cancers, including breast cancer, cervical
cancer, and endometrial cancer [29, 30, 31]. Guihai Zhang et al. [32]
identified 4 lncRNAs, including LINC01281, to predict the prognosis of laryngeal
cancer. Jing Ye et al. [33] reported that cervical cancer patients with
high levels of LINC01281 had better survival. Many studies have shown that CXCL13is related to prognosis. For example, Liangliang Liao et al. [34]
identified three prognostic markers, including CXCL13, in colon adenocarcinoma
(COAD). Sidsel C Lindgaard et al. [35] reported that CXCL13, together
with IL-6, PDCD1, and TNFRSF12A, can be used to discriminate patients with OS
For the first time, we constructed an ICI-related prognostic model for UCEC and calculated the risk score using the gene expression levels. The risk score is not only related to survival time but also closely related to the immune microenvironment, which may guide the efficacy of immunotherapy. To explore the role of the risk score, we divided the patients into low- and high-risk groups using the median score as the cut-off. We observed that the low-risk group had better survival, indicating that the risk score may serve as a prognostic marker. We also compared the ICI patterns between the two groups, and the results showed that the low-risk group had a high stromal score, immune score and almost all immune-relevant signatures. These findings suggest that the low-risk group may be an immune-inflamed phenotype, and the risk score may serve as an immunosuppressive indicator. Moreover, we discovered that the risk score was significantly and negatively correlated with the expression of six ICB-related genes, indicating that patients with a low risk score might be more sensitive to immunotherapy. The immunophenoscore analysis showed that the low-risk score group had statistically higher IPS-CTLA4 and PD1 scores, suggesting that patients with a low risk score had a better response to CTLA4 and PD1 therapy. These findings signify that the risk score might serve as a powerful prognostic tool for UCEC patients.
Endometrial cancer can be effectively cured when diagnosed at an early stage. However, the treatment options become limited once the cancer reaches an advanced or metastatic stage. Tumours with high mutation rates and mismatch repair deficiency (dMMR) are more responsive to immunotherapy likely due to the increased expression of neoantigens that can be recognized by the immune system. Recent clinical studies have shown that immune checkpoint inhibitors, such as PD-1/PD-L1 inhibitors, have a significant effect on chemotherapy-resistant metastatic EC, especially in tumours with dMMR [36, 37, 38]. However, the relationship between tumour cell mutations and the immune microenvironment in immunotherapy is not yet clear. In this study, we aimed to establish an appropriate evaluation system for the TIME and develop a prognostic model. Our results showed that the POLE and MSI-H subtypes had lower risk scores, indicating that patients with the POLE and MSI-H subtypes may be more suitable for immunotherapy, while those with the CNH type had the highest risk score. These patients may require combination therapy for better treatment outcomes. These results were consistent with the molecular typing of endometrial cancer, where tumours with high mutation rates had the lowest risk score for ICI, indicating the potential for effective immunotherapy [38]. Our findings may aid in the clinical decision-making process for immunotherapy, but further experimental and clinical validation is needed.
Tumour mutation burden has been reported to be a predictive indicator of sensitivity to antitumour immunotherapy in various studies [39, 40]. We calculated the TMB using somatic mutation data, and the results showed that TMB was significantly and negatively correlated with the risk score. Patients with a high TMB had better survival in UCEC, which was consistent with previous reports [41]. The synergistic effect of the TMB and risk score showed that patients with low risk scores and a high TMB had the best survival. All the above results demonstrate that the prognostic model may predict the benefit of immunotherapy in UCEC.
In conclusion, 539 UCEC samples were classified into two ICI patterns. Different ICI patterns had a distinct immune microenvironment, so we defined them as “immune-inflamed” and “immune-desert” phenotypes. Moreover, we established an ICI-related prognostic model to predict the prognosis of UCEC patients based on the DEGs between the two ICI patterns. The correlation between the risk score and expression of ICB genes was used to estimate the treatment effect of UCEC patients. Finally, we demonstrated the synergistic effect between the risk score and TMB in predicting prognosis. The ICI-related prognostic model needs further experimental verification and is expected to be used to direct precision immunotherapeutic strategies.
All data used in this study were acquired from The Cancer Genome Atlas (TCGA) portal: TCGA-UCEC (https://portal.gdc.cancer.gov).
HZ, LS, XN, and QW designed the research study. HZ and FL performed the data analysis. FL, WH, SZ, and JC constructed the figures. FL and HZ wrote the manuscript. LS, XN, and QW reviewed and edited the manuscript. All authors contributed to editorial changes in the manuscript. All authors have participated sufficiently in the work and agreed to be accountable for all aspects of the work.All authors contributed to the article and approved the final version.
Not applicable.
We thank the TCGA working groups for generously sharing their data.
This research was supported by the National Natural Science Foundation of China (Grant No. 81872103), Shanghai Municipal Health Commission Funds (Grant No. 20204Y0274 and 202140339) and Innovation-oriented Science and Technology Grant from NHC Key Lab of Reproduction Regulation (Grant No. CX2022-03).
The authors declare no conflict of interest.
Publisher’s Note: IMR Press stays neutral with regard to jurisdictional claims in published maps and institutional affiliations.