-
- Academic Editors
-
-
-
Background: Atrial fibrillation (AF) is a common arrhythmia that can result in adverse cardiovascular outcomes but is often difficult to detect. The use of machine learning (ML) algorithms for detecting AF has become increasingly prevalent in recent years. This study aims to systematically evaluate and summarize the overall diagnostic accuracy of the ML algorithms in detecting AF in electrocardiogram (ECG) signals. Methods: The searched databases included PubMed, Web of Science, Embase, and Google Scholar. The selected studies were subjected to a meta-analysis of diagnostic accuracy to synthesize the sensitivity and specificity. Results: A total of 14 studies were included, and the forest plot of the meta-analysis showed that the pooled sensitivity and specificity were 97% (95% confidence interval [CI]: 0.94–0.99) and 97% (95% CI: 0.95–0.99), respectively. Compared to traditional machine learning (TML) algorithms (sensitivity: 91.5%), deep learning (DL) algorithms (sensitivity: 98.1%) showed superior performance. Using multiple datasets and public datasets alone or in combination demonstrated slightly better performance than using a single dataset and proprietary datasets. Conclusions: ML algorithms are effective for detecting AF from ECGs. DL algorithms, particularly those based on convolutional neural networks (CNN), demonstrate superior performance in AF detection compared to TML algorithms. The integration of ML algorithms can help wearable devices diagnose AF earlier.
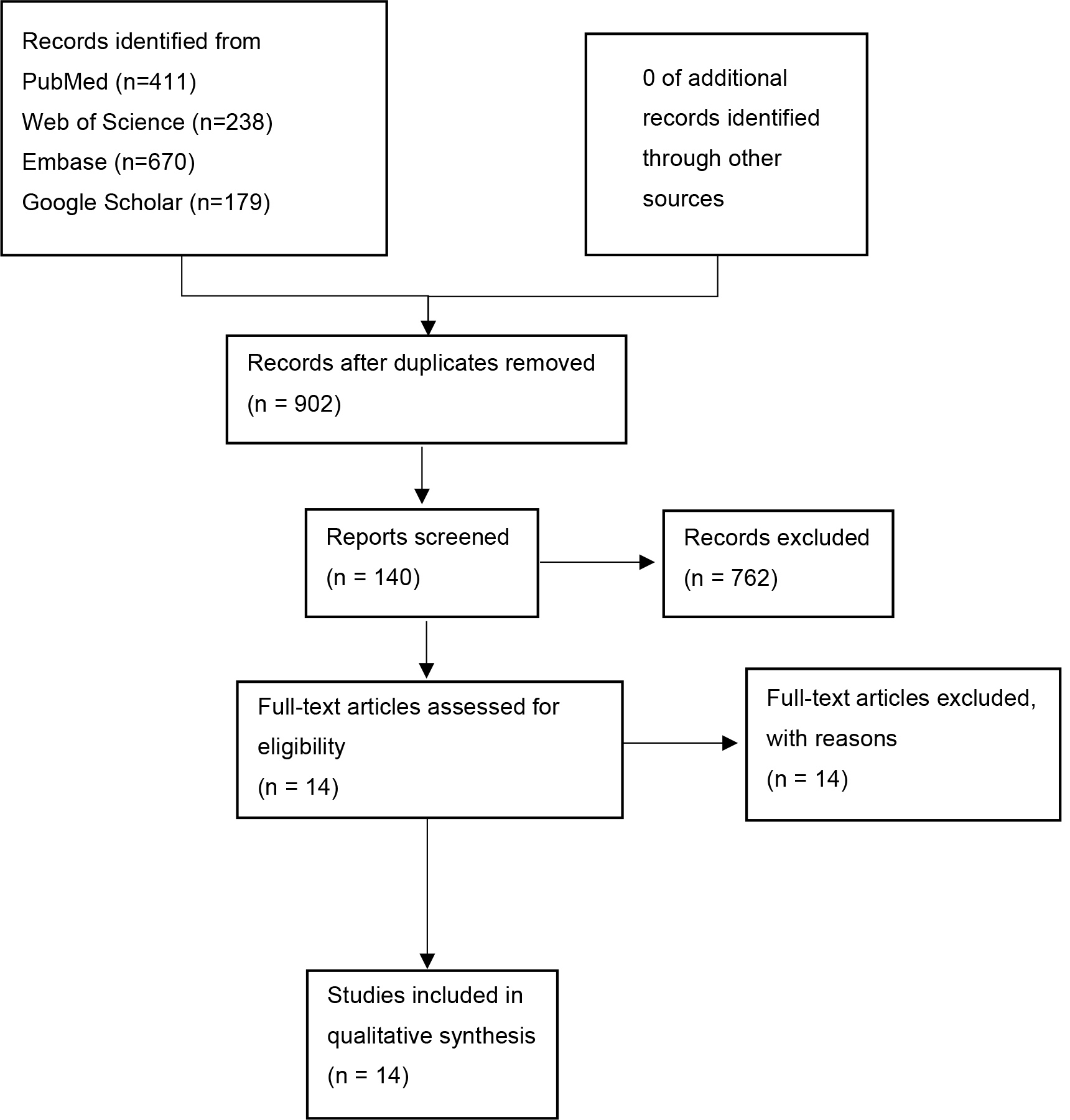