- Academic Editor
†These authors contributed equally.
Background: Prolonged length of stay (LOS) following targeted
temperature management (TTM) administered after cardiac arrest may affect
healthcare plans and expenditures. This study identified risk factors for
prolonged LOS in patients with cardiac arrest receiving TTM and explored the
association between LOS and neurological outcomes after TTM. Methods:
The retrospective cohort consisted of 571 non-traumatic cardiac arrest patients
aged 18 years or older, treated with cardiopulmonary resuscitation (CPR), had a
Glasgow Coma Scale score
Sudden cardiac arrest is among the leading causes of premature death and
disability worldwide [1, 2]. Cardiac arrest imposes a considerable clinical and
economic burden on the healthcare system, society, families, and individual
patients, including direct and indirect medical and nonmedical care costs, such
as financial losses resulting from the loss of productivity. In the United
States, the annual and lifetime economic productivity losses resulting from
out-of-hospital cardiac arrest (OHCA) were estimated at US
Many deaths associated with cardiac arrest occur before arrival at the hospital, and most patients hospitalized after the restoration of spontaneous circulation (ROSC) do not survive discharge [1, 6]. Those discharged with strong functional recovery are among the minority [1, 6, 7]. Despite the scientific debate, to reduce adverse outcomes associated with cardiac arrest, targeted temperature management (TTM) has been introduced to improve neurological recovery in patients with cardiac arrest [8, 9, 10, 11]. A longer length of stay (LOS) and the use of TTM or cardiovascular interventions after arrest significantly affect medical expenditure [4, 5]. LOS is a significant determinant of post-care costs, and identifying the independent predictors of LOS can help improve resource allocation and cost-effectiveness. Furthermore, longer LOS may reduce the quality and efficiency of healthcare. Prolonged LOS may affect the capacity of hospital beds and the availability of healthcare personnel to accommodate patients, which may decrease the healthcare quality and deny other patients access to inpatient care [12, 13, 14]. Extended hospital stays may also result in patients being more susceptible to hospital-acquired infections and lead to less favorable outcomes [12, 15]. In addition, the devices and procedures used to achieve TTM incorporate many complex protocols [16]. Significant heterogeneity exists in patients who received TTM [10, 16, 17]. The interventions and medications used in TTM vary greatly depending on the patient’s condition, and there may be different complications after TTM that require additional treatments [6, 10, 16, 18]. These variabilities may result in prolonged LOS for patients undergoing TTM. In addition to potentially increasing the time spent in the hospital, TTM may delay critical decision-making regarding whether to continue active treatment or withdraw care [19]. There have been many reports discussing and establishing the predictions of neurological outcomes of TTM [20, 21, 22, 23, 24, 25]. No study has determined the relationship between TTM outcomes and LOS in hospitals after cardiac arrest.
The present study identified risk factors for prolonged LOS in patients undergoing TTM, explored the association between neurological outcomes after TTM and LOS, and developed a predictive model to estimate the risk of prolonged LOS in patients with cardiac arrest before the application of TTM.
Clinical data from medical records obtained from the Taiwan Network of Targeted Temperature Management for Cardiac Arrest (TIMECARD) registry were retrospectively reviewed [20, 26]. The TIMECARD registry is a nationwide multi-center registry project conducted between January 2014 and September 2019 in nine medical centers in Taiwan [20, 22, 26]. Each participating hospital reported its patient-level data by using an online case report form. All electronic medical data were anonymized.
We included patients who were aged 18 years or more, experienced a nontraumatic
cardiac arrest event that occurred inside or outside the hospital, were treated
with cardiopulmonary resuscitation (CPR), had a Glasgow Coma Scale score of
We excluded patients who experienced uncontrollable bleeding or intracranial
hemorrhage; had impaired consciousness before cardiac arrest, as indicated by a
Cerebral Performance Category (CPC) score of
All eligible patients were treated in accordance with the TTM protocol following the consensus scientific statement issued by the Taiwan Society of Emergency and Critical Care Medicine [26]. TTM is designed to maintain a patient’s temperature within the target range with minimal variation. Depending on the clinical protocol of each participating hospital, TTM can be applied using cold saline infusion, cooling blankets, intravenous cooling catheters, extracorporeal membrane oxygenation, or in combination with other therapies [20, 26]. All protocols maintain the patient’s temperature at the target level for at least 24 h and rewarm them to normal body temperature at a relatively slow rate [20]. Patient registration data were recorded using the latest Utstein resuscitation registration template [26, 27]. Variables were retrieved from archived patients’ registries and analyzed, including baseline demographic data, comorbidities, cardiac arrest etiology, TTM method, complications, and outcomes. TTM-related complications were defined as complications that occurred within 7 days of undergoing TTM. LOS for patients with OHCA was calculated as the time from admission to hospital discharge. LOS for patients with in-hospital cardiac arrest (IHCA) was calculated as the time from the occurrence of the cardiac arrest event to discharge. We defined prolonged LOS as hospital stays beyond the 75th quartile of the entire cohort [28, 29].
A CPC score of 1 to 2 (conscious and alert with adequate or moderate cerebral performance) at discharge was considered a favorable neurological outcome; an unfavorable outcome was defined as a CPC score of 3 to 5 (severe neurological disability, persistent vegetative state, or death) [23, 26, 27].
Categorical variables are described as numbers and percentages. As appropriate,
continuous variables are presented as means
To predict prolonged LOS, we developed logistic regression (LR)-based models by using patient information at the time of TTM application, post-TTM, and at discharge. Variables were included in LR analysis, and the effects of relevant variables are reported as odds ratios and 95% confidence intervals.
In model 1, we used patients’ age, sex, event type, prearrest CPC, initial cardiac arrest rhythm, CPR duration, comorbidities, and etiology as predictors, representing the prolonged LOS prediction prior to TTM (Fig. 1). Model 2 contains all the pre-TTM variables in model 1, the maintenance model, and complications of TTM, which represents the prediction after TTM. Model 3 includes all of the aforementioned predictors and the CPC score at discharge, representing the prediction at discharge. The predicted outcome was whether prolonged LOS followed treatment with TTM (Fig. 1). We performed receiver operating characteristic curve analysis and calculated the area under the curve (AUC) to evaluate the level of discrimination of the models. All statistical analyses were performed using STATISTICA v13.3 (TIBCO Software, Tulsa, OK, USA).
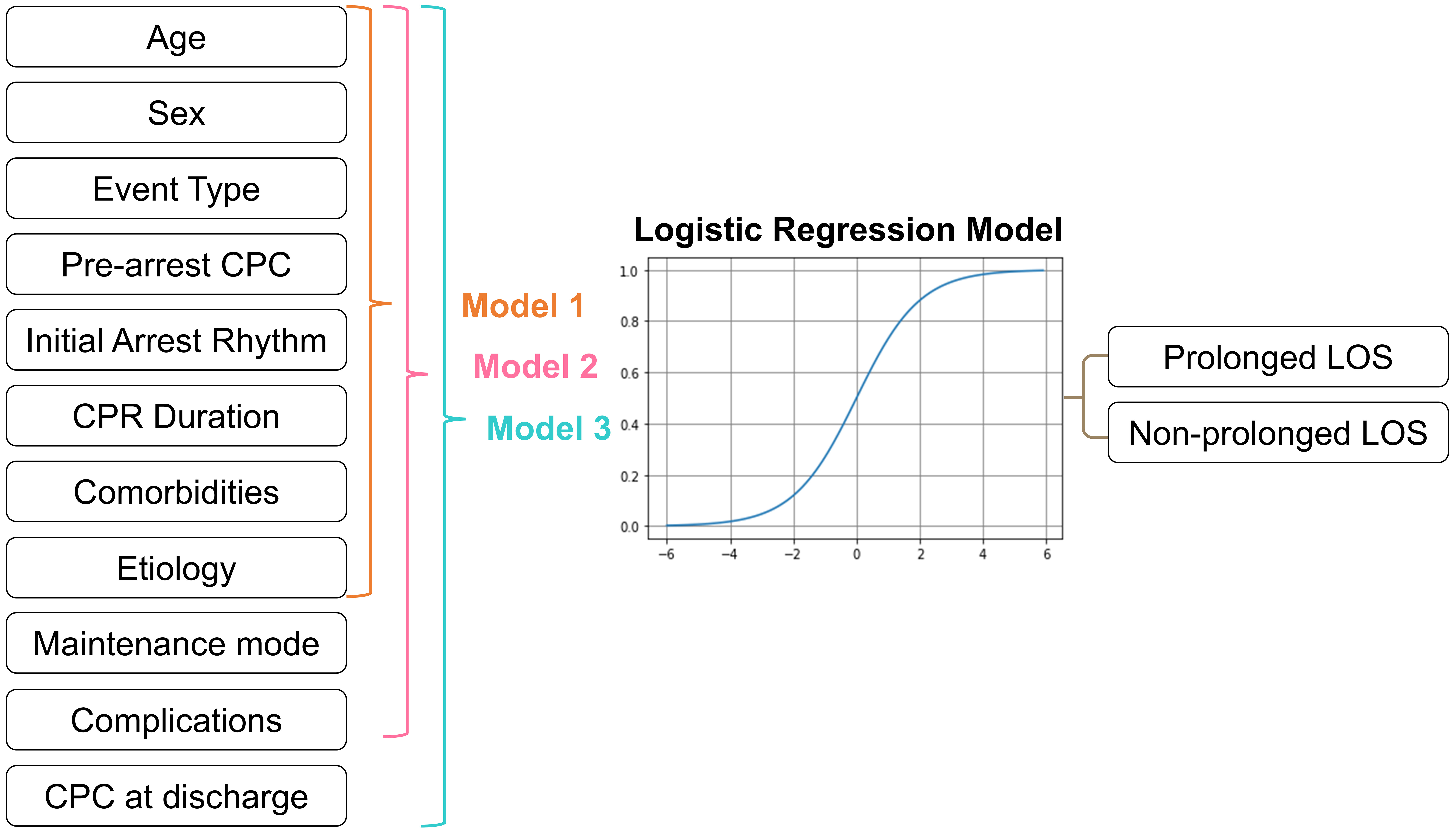
Logistic regression models predicting prolonged LOS for patients who underwent TTM. Graphical representation of the predictors and outcomes used in the LR models for predicting prolonged LOS before the initiation of TTM (model 1), after TTM (model 2), and at discharge (model 3). CPC, Cerebral Performance Category; CPR, cardiopulmonary resuscitation; LOS, length of stay; LR, logistic regression; TTM, targeted temperature management.
A total of 580 patients were registered in the TIMECARD database during the
study period. Nine patients without documented discharge dates from the hospital
were excluded from the analysis. A total of 571 patients with cardiac arrest who
received TTM (195 women and 376 men; mean age: 64.7
Variable | Entire cohort (n = 571) | Length of stay (LOS) in the hospital | p-value | ||
Prolonged (n = 146) | Non-prolonged (n = 425) | ||||
LOS (days) | 17 (9–35) | 54 (42–69.75) | 13 (6–20) | ||
Age (years) | 64.7 |
64.8 |
64.6 |
0.881 | |
Female, n (%) | 195 (34.2) | 48 (32.9) | 147 (34.6) | 0.762 | |
Event type, n (%) | 0.0001* | ||||
OHCA | 463 (81.1) | 102 (69.9) | 361 (84.9) | ||
IHCA | 108 (18.9) | 44 (30.1) | 64 (15.1) | ||
Prearrest CPC score | 1.29 |
1.37 |
1.27 |
0.087 | |
Initial cardiac arrest rhythm, n (%) | 0.886 | ||||
VF/Pulseless VT | 209 (36.6) | 54 (37.0) | 155 (36.5) | ||
Pulseless electrical activity | 138 (24.2) | 37 (25.3) | 101 (23.8) | ||
Asystole | 224 (39.2) | 55 (37.7) | 169 (39.8) | ||
CPR duration (min)* | 24.0 |
21.3 |
24.9 |
0.020* | |
Etiology | 0.201 | ||||
Cardiac | 301 (52.7) | 74 (50.7) | 227 (53.4) | ||
Asphyxia | 116 (20.3) | 27 (18.5) | 89 (20.9) | ||
Sepsis | 59 (10.3) | 16 (11.0) | 43 (10.1) | ||
Electrolyte imbalance or acidosis | 21 (3.7) | 9 (6.2) | 12 (2.8) | ||
Other medical causes | 25 (4.4) | 10 (6.8) | 15 (3.5) | ||
Other nonmedical causes | 49 (8.6) | 10 (6.8) | 39 (9.2) | ||
Comorbidities, n (%) | |||||
Hypertension | 323 (56.6) | 87 (59.6) | 236 (55.5) | 0.439 | |
Diabetes mellitus | 236 (41.3) | 63 (43.2) | 173 (40.7) | 0.627 | |
Coronary artery disease | 152 (26.6) | 45 (30.8) | 107 (25.2) | 0.194 | |
Heart failure | 109 (19.1) | 39 (26.7) | 70 (16.5) | 0.010* | |
Arrhythmia | 71 (12.4) | 20 (13.7) | 51 (12.0) | 0.565 | |
COPD or asthma | 62 (10.9) | 25 (17.1) | 37 (8.7) | 0.008* | |
Chronic kidney disease | 105 (18.4) | 36 (24.7) | 69 (16.2) | 0.026* | |
ESRD with dialysis | 70 (12.3) | 16 (11.0) | 54 (12.7) | 0.662 | |
Hepatic insufficiency | 18 (3.2) | 5 (3.4) | 13 (3.1) | 0.788 | |
Previous cerebral vascular disease | 75 (13.1) | 21 (14.4) | 54 (12.7) | 0.670 | |
Hyperlipidemia | 106 (18.6) | 24 (16.4) | 82 (19.3) | 0.537 | |
Malignancy | 72 (12.6) | 22 (15.1) | 50 (11.8) | 0.313 | |
Maintenance mode, n (%) | 0.774 | ||||
Arctic Sun cold blanket | 271 (47.5) | 73 (50.0) | 198 (46.6) | ||
Traditional cold blanket | 242 (42.4) | 57 (39.0) | 185 (43.5) | ||
ECMO | 41 (7.2) | 12 (8.2) | 29 (6.8) | ||
Icy catheter | 17 (3) | 4 (2.7) | 13 (3.1) | ||
Complications, n (%) | |||||
Bleeding | 156 (27.3) | 37 (25.3) | 119 (28.0) | 0.591 | |
Arrhythmia | 237 (41.5) | 54 (37.0) | 183 (43.1) | 0.207 | |
Serious infection | 257 (45.0) | 65 (44.5) | 192 (45.2) | 0.923 | |
Seizure | 158 (27.7) | 55 (37.7) | 103 (24.2) | 0.003* | |
Hypokalemia | 363 (63.6) | 86 (58.9) | 277 (65.2) | 0.195 | |
Hypoglycemia | 61 (10.7) | 14 (9.6) | 47 (11.1) | 0.756 | |
Outcome measures | |||||
CPC score at discharge | 5 (3–5) | 4 (3–5) | 5 (4–5) | 0.0004* | |
Survival to discharge, n (%) | 233 (40.8) | 104 (71.2) | 129 (30.4) | ||
Favorable neurological outcome, n (%) | 119 (20.9) | 30 (20.5) | 89 (20.9) | 1.000 | |
COPD, chronic obstructive pulmonary disease; CPC, Cerebral Performance Category;
ECMO, extracorporeal membrane oxygenation; ESRD, End-stage renal disease; IHCA,
in-hospital cardiac arrest; LOS, length of stay; OHCA, out-of-hospital cardiac
arrest; OR, odds ratio; VF, ventricular fibrillation; VT, ventricular
tachycardia.
*p-value |
In the cohort, a significantly higher proportion of the patients with IHCA had
prolonged LOS than did those with OHCA (40.7% IHCA patients with prolonged LOS
vs. 22% OHCA patients, p = 0.0001; Table 1). The median LOS for the
patients with IHCA and OHCA were 25 (IQR: 11–52.75) and 16 (IQR: 9–31) days,
respectively (p = 0.01). The patients with prolonged LOS had a shorter
CPR duration and higher prevalence rates of heart failure, chronic obstructive
pulmonary disease (COPD) or asthma, and chronic kidney disease compared with
those without prolonged LOS. The patients with COPD or asthma had a longer mean
LOS (39.4
The factors in Table 1 were included in multivariate analyses to compare the
prolonged LOS and non-prolonged LOS groups according to the three models in Fig. 1; Table 2 summarizes the variables with a p-value of
Variable | Crude odds ratio (95% CI) | Adjusted odds ratio (95% CI) |
---|---|---|
Model 1 | ||
Event type, IHCA | 2.43 (1.56, 3.79)* | 2.16 (1.29, 3.64)* |
COPD or asthma | 2.17 (1.25, 3.74)* | 2.35 (1.27, 4.36)* |
Model 2 | ||
Event type, IHCA | 2.43 (1.56, 3.79)* | 2.14 (1.25, 3.66)* |
COPD or asthma | 2.17 (1.25, 3.74)* | 2.46 (1.30, 4.64)* |
Hyperlipidemia | 1.22 (0.74, 2.00) | 1.89 (1.03, 3.50)* |
Seizure | 1.89 (1.26, 2.82)* | 2.25 (1.43, 3.53)* |
Model 3 | ||
Event type, IHCA | 2.43 (1.56, 3.79)* | 2.42 (1.29, 4.54)* |
Heart failure | 1.85 (1.18, 2.89)* | 2.66 (1.30, 4.64)* |
Seizure | 1.89 (1.26, 2.82)* | 1.96 (1.13, 3.41)* |
CPC at discharge, 1 | 1.66 (0.90, 3.09) | 2.97 (1.35, 6.55)* |
CPC at discharge, 2 | 4.56 (2.00, 10.40)* | 6.48 (2.49, 16.9)* |
CPC at discharge, 3 | 16.66 (7.67, 36.18)* | 28.45 (11.62, 69.66)* |
CPC at discharge, 4 | 12.15 (6.94, 21.28)* | 16.62 (8.52, 32.43)* |
CPC at discharge, 5 | 1.00 | 1.00 |
The table summarizes the variables with a p-value of |
We examined associations between neurological outcomes and LOS in patients with
cardiac arrest treated with TTM. A higher proportion of the patients with
prolonged LOS tended to survive discharge than did those with a shorter LOS
(71.2% vs. 30.4%, p
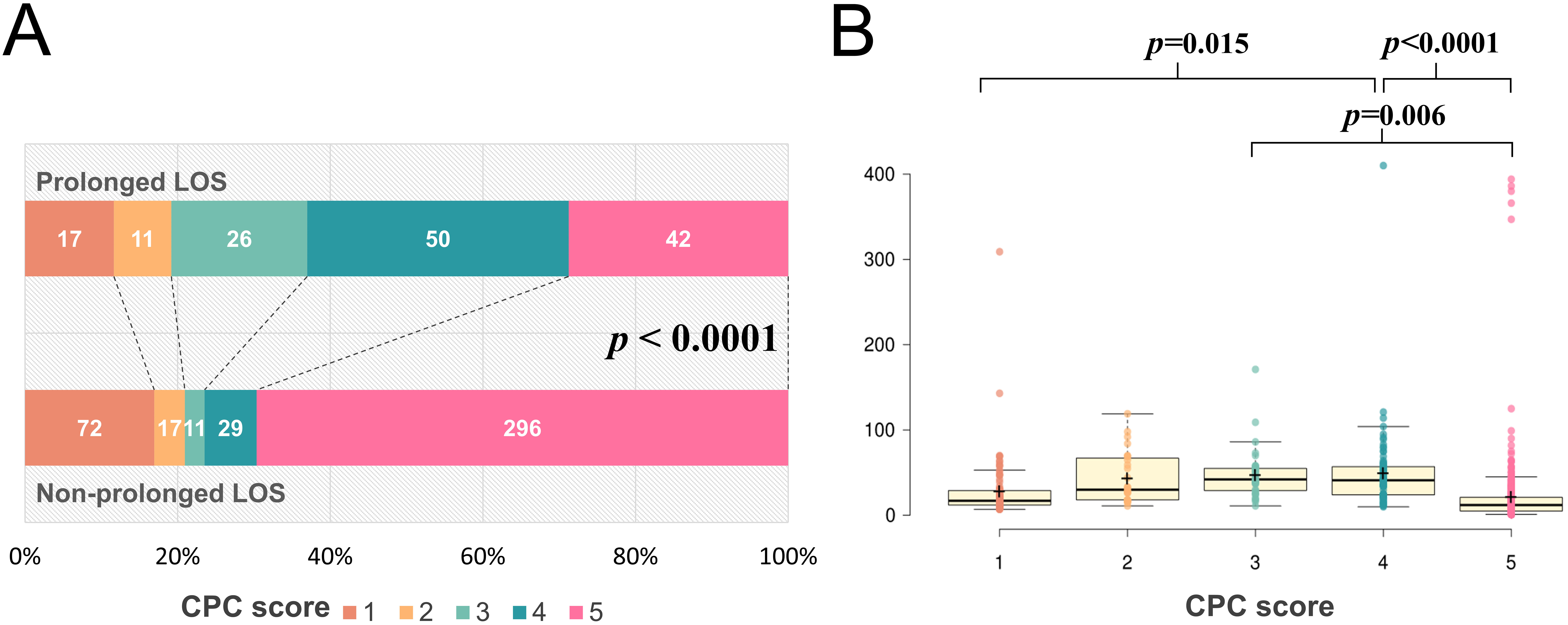
CPC scores and LOS. (A) CPC scores of patients with and without prolonged LOS. The difference in CPC scores at discharge for patients with cardiac arrest who underwent TTM with or without prolonged LOS. Each color-coded bar indicates the number of patients. (B) LOS in days for patients with different CPC scores at discharge. The x-axis represents the LOS in days. Box and dot plots indicate the LOS for patients who underwent TTM with different CPC scores at the time of discharge. Data are presented as raw data and box plots with median, 25% quartile, 75% quartile, and standard deviation. Black crosses indicate the mean LOS. Patients with a CPC score of 1 had a significantly shorter LOS than those with a CPC score of 4; patients with a CPC score of 5 had a shorter LOS than those with a CPC score of 3 or 4. CPC, Cerebral Performance Category; LOS, length of stay; TTM, targeted temperature management.
For the entire cohort, the median time from ROSC to TTM was 4 h 8 min (IQR: 2 h 50
min–6 h 19 min). Most patients (99.6%) showed an improvement in their Glasgow Coma Score (GCS)
between the interval following ROSC and initiating TTM, with
a GCS of 3.5
As presented in Fig. 1, we developed an LR-based model using patient information before and after the application of TTM, and at discharge to predict prolonged LOS. Fig. 3A illustrates the performance of prediction model 1 for the entire cohort, as indicated by an AUC of 0.694. Furthermore, we also calculated the performance of the LR models for predicting prolonged LOS in patients with IHCA (n = 108) and OHCA (n = 463) who received TTM. The LR model 1 with age, sex, prearrest CPC, initial cardiac arrest rhythm, CPR duration, comorbidities, and etiology as predictors achieved an AUC of 0.716 and 0.694 for the patients with IHCA and OHCA, respectively. Model 2 contained maintenance mode, complications of TTM, and the predictors of model 1, achieving an AUC of 0.718 for the entire cohort. The AUCs of model 2 were 0.790 and 0.712 for the patients with IHCA and OHCA, respectively (Fig. 3B). Model 3 included all the predictors of model 2 and CPC score at discharge, achieving AUCs of 0.840, 0.896, and 0.849 for the entire cohort, patients with IHCA, and patients with OHCA, respectively (Fig. 3C). The acceptable model performance demonstrates the model’s potential to assist in the clinical prediction of prolonged LOS for patients who undergo TTM.
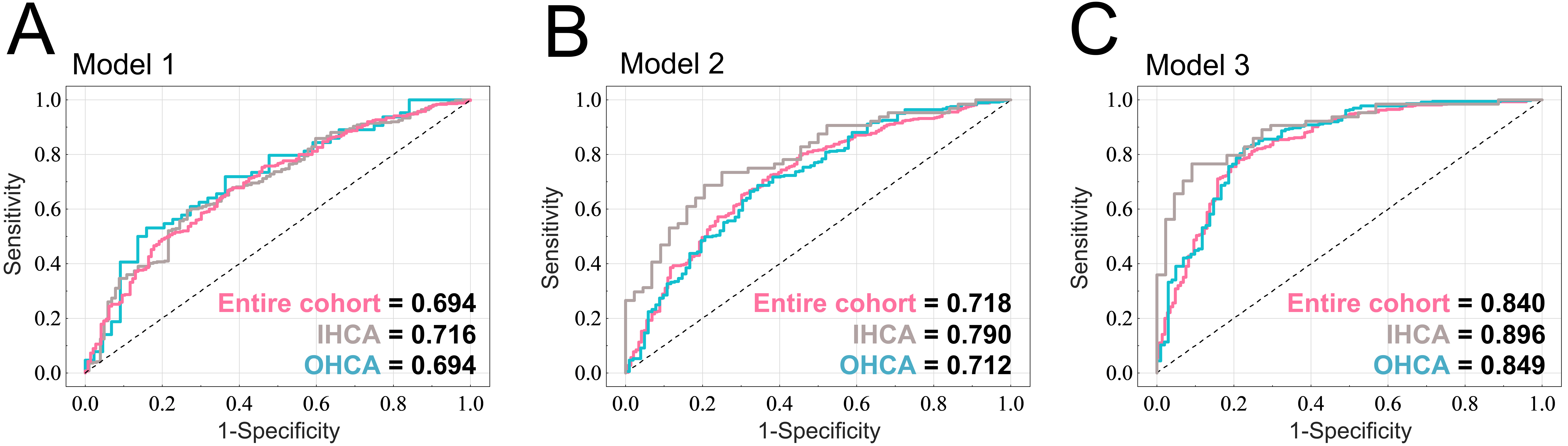
Performance in predicting prolonged LOS for patients who underwent TTM. The ROC curves and AUC values of the (A) model 1, (B) model 2, and (C) model 3 for the entire cohort, for patients with IHCA, and patients with OHCA. Models 1, 2, and 3 represent the prediction of prolonged LOS before TTM, after TTM, and at discharge, respectively. AUC, area under the curve; CPC, Cerebral Performance Category; IHCA, in-hospital cardiac arrest; LOS, length of stay; LR, logistic regression; OHCA, out-of-hospital cardiac arrest; ROC, receiver operating characteristic; TTM, targeted temperature management.
Our findings revealed that, in the current cohort, the median LOS of the patients with cardiac arrest who underwent TTM was 17 days (IQR: 9–35 days). Compared with the patients with OHCA, those with IHCA had a longer LOS. Comorbid heart failure, COPD or asthma, chronic kidney disease, and epilepsy after TTM increase the risk of prolonged hospitalization. The patients who survived cardiac arrest after receiving TTM had longer hospitalization than did those who died, but prolonged LOS was not associated with overall favorable neurological outcomes. At discharge, the patients with a CPC score of 1 or 5 had a significantly shorter LOS, and those with a CPC score of 3 or 4 had a considerably longer LOS. The AUCs of the LR-based prediction model based on patient parameters prior to TTM, after TTM, and at discharge were 0.694, 0718, and 0.840, respectively, indicating that these models exhibited favorable performance in predicting prolonged LOS in the patients with cardiac arrest who received TTM.
TTM was introduced in the 1990s to reduce the metabolic demand on the brain tissue, providing a protective effect for patients after cardiac arrest [30, 31, 32, 33]. However, its effectiveness remains controversial because of the heterogeneity of populations and interventions applied in various studies and the inconsistent results of several trials [34, 35, 36, 37]. Although most discussions have focused on prognoses, our study focused on LOS for patients treated with TTM, which is not linearly correlated with neurological prognosis. The patients with the highest or lowest CPC score had a shorter LOS. By contrast, those who survived to discharge with CPC scores of 3 or 4 had relatively prolonged LOS (Fig. 2B). LOS is an indicator of healthcare resource consumption [5, 38, 39]. A study revealed that the cost of hospitalization for patients who received TTM was 25% more than that for patients who did not receive TTM [5]. Another study comparing historical controls indicated that patients with OHCA who received TTM with unfavorable treatment outcomes (CPC score of 3 or 4) had a longer intensive care unit (ICU) stay and spent more time on ventilators [40]. These results are similar to our findings, and our observations revealed that the patients with a CPC score of 1 or 5 had a shorter LOS. In our study, all the shortest quartiles of hospital stays were those patients who died at discharge. Our results implied that patients with unfavorable prognoses after TTM may require more intensive nursing care and longer hospitalization than did those with favorable outcomes and those who die early after cardiac arrest. On the other hand, sedatives and analgesics are commonly used in patients undergoing TTM. For patients with cardiac arrest, sedatives or neuromuscular blocking agents may be metabolized more slowly [41]. The use and accumulation of these drugs may further cause delayed awakening, thus affecting the prognosis and prolonging LOS in patients who received TTM [16, 18, 41, 42]. In addition to potentially increasing the time spent in the ICU or hospital, TTM may delay critical decision-making regarding whether to continue active treatment, withdraw care, or donate organs [4, 19]. TTM may increase the number of patients who survive cardiac arrest, but these patients may have severely impaired neurological function [34]. Although the patient’s life is prolonged, the subsequent quality of life might be low and potentially costly. Similar to a hypothetical model developed on the basis of the inclusion criteria of the Hypothermia After Cardiac Arrest trial [4], patients who survived but had poor neurological function accounted for the majority of the cost of post-discharge care for patients with cardiac arrest.
In line with the findings of another study [43], our study indicated that the patients with IHCA had a significantly longer LOS after TTM than did patients with OHCA. To our knowledge, no randomized trials of TTM for IHCA have been conducted. A meta-analysis that included six retrospective controlled studies revealed that TTM did not improve survival or neurological function among patients with IHCA [44]. Because the characteristics of patients with IHCA and the treatment received before, during, and after arrest may differ from those of patients with OHCA, the prognosis and LOS after TTM should be evaluated as different entities [37, 43, 45].
In our cohort, receiving TTM and having COPD or asthma as a comorbidity significantly affected LOS. Studies have reported a lower survival rate to hospital discharge and less favorable neurologic outcomes in patients with cardiac arrest comorbid with COPD compared with those without COPD [20, 22, 46, 47]. To our knowledge, no reports on the relationship between COPD or asthma and LOS after TTM have been published. Altered pulmonary compliance resulting from COPD and asthma may lead to respiratory-related comorbidities and complicate treatment applied after TTM, thus affecting neurological outcomes and prolonging LOS [39].
Heart failure is a common comorbidity in patients with sudden cardiac arrest [7, 48]. For patients undergoing TTM, underlying heart failure is associated with reduced survival and a worse prognosis [22, 24]. In our LR model 3, heart failure was demonstrated to be an essential predictor of prolonged LOS at discharge (Table 2). This finding is in line with current knowledge that the characteristics of heart failure include concomitant acute illness and complications, the need for more appropriate treatments, and prolonged hospital stays [1, 49, 50].
In our cohort, 27.7% of the patients developed seizures after TTM and had a significantly longer LOS. The incidence of epilepsy among our patients was similar to that in other reports [51, 52]. Studies have demonstrated an association between seizures and adverse neurologic outcomes in patients after cardiac arrest [41, 51, 52]. The treatment of epilepsy and the use of anesthetic or sedative medications for status epilepticus may result in delayed awakening [53, 54] and prolonged hospitalization of patients with cardiac arrest.
With the continual development of healthcare systems, the management of hospital resources and the ability to predict patients’ LOS are becoming crucial. Our model 3, achieving an AUC of 0.840 to 0.895, provides further relevant knowledge and direction for developing LOS prediction models for patients who receive TTM. With an advanced understanding of patients’ LOS, healthcare teams can make accurate medical decisions, provide information to patients and families regarding expected discharge dates, and design appropriate medical plans [55, 56]. Likewise, LOS is an indicator of the speed of recovery, which can assist patients in the organization and management of their medical budgets. Hospitals can use predicted LOS information to reduce the cost of illness, improve the efficiency of care, and increase the use of resources [55, 56]. Predicting and analyzing factors affecting the LOS of patients with cardiac arrest receiving TTM can improve management and reduce risk factors for patients before and during hospitalization [56].
The correlation between LOS, prognosis, and medical expenditures is multi-factorial and complex. Previous studies have reported that the cost of hospitalization for those patients with cardiac arrest experiencing a CPC of 3–4 was considerably higher than for those with a CPC of 1–2 [39, 57]. Performing early prognostication may help to reduce hospitalization costs. However, when the total long-term costs and benefits for these patients are considered, hospitalization costs are only a small part of the equation. The cost of post-discharge care, rehabilitation, and loss of economic productivity after cardiac arrest can be enormous [2, 3, 4, 5]. Although cardiac arrest patients require considerable cost and resource consumption, this allocation of resources is critical and reasonable when considering the trade-off between inputs and outputs, costs and outcomes in terms of the patient’s long-term survival and quality of life [39, 58, 59].
Our study should be interpreted in the context of the following limitations. First, although this was a multi-center study, the sample size was relatively small. Therefore, a large multi-center cohort with a greater number of patients with different characteristics is required to represent the disease population and validate our results. Second, for patients with IHCA, our registry lacks information about the severity of the disease and events at the time of admission or during hospitalization. The registry also did not have information on whether patients received tracheostomy that required prolonged mechanical ventilation after ROSC. These factors may affect LOS more than the variables reported in the current study. Third, the generalizability of the study may be limited by differences in admission processes and treatment plans among the participating hospitals as well as by the heterogeneity of TTM protocols across the hospitals. Fourth, differences in health insurance, religious affiliation, and socioeconomic status may affect how long a patient remains in the hospital. Our participants were confined to a geographic area and may not be a diverse population. In future studies, different institutions could analyze and develop individualized predictive models based on patient characteristics. Finally, actual medical costs are not reported in the TIMECARD registry. Our study only examined the number of days of hospitalization and could not, therefore, assess the impact of TTM and prognosis on actual medical costs.
Identification of factors associated with prolonged LOS after TTM, especially at different phases of therapy, can provide essential information that can be beneficial for the medical team while making crucial decisions and designing appropriate medical plans for patients. This information acts as a reference for hospital management for the allocation of necessary treatment resources. Likewise, patients’ relatives can use this knowledge to manage and organize their budgets and expectations of illness.
COPD, chronic obstructive pulmonary disease; CPC, Cerebral Performance Category; CPR, cardiopulmonary resuscitation; ECMO, extracorporeal membrane oxygenation; ESRD, End-stage renal disease; IHCA, in-hospital cardiac arrest; LOS, length of stay; LR, logistic regression; OHCA, out-of-hospital cardiac arrest; TTM, Targeted Temperature Management.
The datasets used and analyzed during the current study are available from the corresponding author on reasonable request.
C-CC and LC designed the study. W-TC performed the research and acquired the data. W-TC and C-CC analyzed and interpreted the data and wrote the manuscript. JHBM, C-TH, Y-SC, C-HH, C-HWu, C-HWang, and ST revised the manuscript critically for intellectual content. All authors contributed to editorial changes in the manuscript. All authors read and approved the final manuscript.
The Joint Institutional Review Board of Taipei Medical University (TMU-JIRB Approval No. N201711046) approved the study. The TMU-JIRB approved the waiving of informed consent for this retrospective study involving the secondary analysis of anonymous data.
We thank the reviewers for their thoughtful comments.
This research was funded by Taipei Medical University (TMU111-AE1-B23) to CC Chung.
The authors declare no conflict of interest.
Publisher’s Note: IMR Press stays neutral with regard to jurisdictional claims in published maps and institutional affiliations.