Academic Editor: Jerome L. Fleg
Background: There are almost 2 million adult patients with congenital
heart disease in China, and the number of moderate and severe patients is
increasing. However, few studies have investigated the risk of serious adverse
events (SAE) after catheterization among them. The aim of this study was to
identify risk factors for SAE related to cardiac catheterization and to provide
the risk scoring model for predicting SAE. Methods: A total of 690
patients with moderate and severe adult patients with congenital heart disease
(ACHD) who underwent cardiac catheterization in Wuhan Asian Heart Hospital
Affiliated to Wuhan University of Science and Technology from January 2018 to
January 2022 were retrospectively collected and subsequently divided into a
modeling group and a verification group. A univariate analysis was performed on
the identified SAE risk factors, and then significant factors were included in
the multivariate logistic regression model to screen for independent predictors
of SAE. The receiver operating characteristic curve (ROC) and the Hosmer-Lemeshow
test were used to evaluate the discrimination and calibration of the model,
respectively. Results: A SAE occurred in 69 (10.0%) of the 690
catheterization procedures meeting inclusion criteria. The established SAE risk
calculation formula was logit(p) = –6.134 + 0.992
Congenital heart disease is defined as impaired formation of the heart and great vessels during embryonic period, or unclosed passage after birth, resulting in abnormal structure or function of the heart or great vessels [1]. With the advancement of pediatric cardiology, more and more children with congenital heart disease can successfully survive to adulthood [2]. There are almost 50 million adult patients with congenital heart disease (ACHD) worldwide [3], and the number in China increases rapidly, reaching about 2 million [4]. Cardiac catheterization has been widely applied to treat ACHD patients due to its advantages such as smaller incisions and fewer complications. However, studies have showed that the occurrence rate of serious adverse events (SAE) for ACHD patients underwent cardiac catheterization is as high as 24%, and compared with patients of mild ACHD, moderate and severe patients are more complex and have a higher chance of suffering from SAE after cardiac catheterization [5].
The risk prediction model can guide medical staff to carry out individual prevention and treatment measures [6]. Over the past 10 years several risk prediction models for ACHD patients after cardiac catheterization had been constructed [7, 8, 9]. However, there has been far less research conducted on SAE among Chinese patients with moderate and severe ACHD. Moreover, the application value of those models for Chinese patients has not been confirmed. The aim of this study is to develop and validate the SAE risk prediction model for Chinese moderate and severe ACHD patients, so as to help clinicians early identify the high-risk ACHD patients and provide timely prevention and treatment for them.
Our study was approved by the Medical Ethics Committee of Wuhan University of Science and Technology (reference number: 2022116). The data of all moderate and severe ACHD patients who underwent cardiac catheterization in Wuhan Asian Heart Hospital from January 2018 to January 2022 were retrospectively collected via the hospital electronic medical record system.
Inclusion Criteria: patients with congenital heart disease diagnosed by
echocardiography; patients aged
Among 690 patients included, 483 cases from January 2018 to December 2020 were used as the derivation cohort, while 207 cases from January 2021 to January 2022 were used as the validation cohort.
A SAE was defined as any adverse event causing mortality, permanent morbidity, need for further interventions, or extended length of stay [11] (Supplementary Table 2). SAE information recorded in the electronic medical record system included: event name, brief narrative description, identification time, symptoms, diagnostic auxiliary examinations, and handling measures.
There were 44 risk factors screened in our study: (1) General conditions: age, gender, height, weight, body mass index (BMI), heart rate (HR), systolic blood pressure (SBP), smoking or drinking history; hospital sources; (2) Complications: hypertension, diabetes, coronary heart disease, heart failure, cerebrovascular disease, chronic obstructive pulmonary disease (COPD), pulmonary artery hypertension, cyanosis, anemia; (3) Procedure-related indicators: disease severity, type of catheterization, procedure risk, access location, degree of surgical anesthesia, American Society of Anesthesiology (ASA) score. Procedure risk categories were devised based on the CRISP 9 and C3PO risk categories [12, 13] (Supplementary Table 3); (4) Laboratory examinations: N-terminal Pro-B-type Natriuretic Peptide (NT-proBNP), potassium determination, magnesium determination, calcium determination, cardiac troponin I (cTnI), uric acid (UA), triglyceride (TG), serum total cholesterol (TC), low-density lipoprotein cholesterol (LDL-C), high-density lipoprotein cholesterol (HDL-C), serum creatinine (Scr), serum urea (Urea), aspartate transferase (AST), lactate dehydrogenase (LDH), red blood cell (RBC), neutrophils (NEUT), hemoglobin (HB), high-sensitivity C-reactive protein (hs-CRP), and plasma D-dimer (D-D).
Data were analyzed using SPSS 26.0 software (IBM, Armonk, NY, USA). Data did not
conform to normal distribution after inspected, so Mann-Whitney U test and
Pearson chi-square test were used. Variables with statistical significance in
univariate analysis were included in binary logistic regression analysis, and
independent risk factors were screened by stepwise forward method for
establishing risk prediction model. To eliminate the influence of extreme values
on the regression results, continuous variables were dichotomously transformed
using the cut-off value corresponding to the receiver operating characteristic
(ROC) curve [14]. The discrimination of the risk prediction model was tested by
the area under the ROC curve (AUC), and AUC
Four methods were used to compare the application value between our model and
CRISA model [7]: (1) –2log likelihood ratio (N2LL); (2) Akaike Information
Criterion (AIC), defined as N2LL + (2
Among 690 moderate and severe ACHD patients who underwent cardiac catheterization, 236 (34.2%) were males and 454 (65.8%) were females, aged from 18–81 years with an average age of 44 years. The most common diagnosis was partial or complete atrioventricular septal defect (n = 226, 32.8%), followed by moderate and large patent ductus arteriosus (n = 86, 12.5%), aortic sinus aneurysm/fistula (n = 42, 6.1%), and congenital heart disease associated with pulmonary vascular disease (n = 37, 5.4%), as shown in Table 1. 529 (76.7%) procedures were diagnostic cardiac catheterization combined with interventional therapy and 161 (23.3%) procedures were isolated cardiac catheterization. Interventional procedures were most commonly closure (n = 371, 70.1%), followed by percutaneous transluminal angioplasty or stenting (n = 53, 10.0%), balloon valvuloplasty (n = 41, 7.8%), embolization (n = 38, 7.2%), and combined intervention for other complex malformations (n = 26, 4.9%).
Type of congenital heart disease | n (%) | |
---|---|---|
Moderate | ||
Anomalous pulmonary venous connection | 18 (2.6) | |
Anomalous coronary artery arising from the pulmonary artery | 16 (2.3) | |
Anomalous coronary artery arising from the opposite sinus | 19 (2.8) | |
Aortic stenosis — subvalvular or supravalvular | 11 (1.6) | |
Partial or complete atrioventricular septal defect | 226 (32.8) | |
Secondary atrial septal defect | 25 (3.6) | |
Coarctation of the aorta | 16 (2.3) | |
Double chambered right ventricle | 22 (3.2) | |
Unrepaired moderate and large patent ductus arteriosus | 86 (12.5) | |
Moderate or severe pulmonary stenosis | 33 (4.8) | |
Sinus of Valsalva aneurysm/fistula | 42 (6.1) | |
Sinus venosus defect | 7 (1.0) | |
Ventricular septal defect with associated anomalies | 32 (4.6) | |
Severe | ||
Congenital Heart Disease Associated with Pulmonary Vascular Disease | 37 (5.4) | |
Cyanotic congenital heart disease | 26 (3.8) | |
Double-outlet ventricle | 21 (3.0) | |
Interrupted aortic arch | 3 (0.4) | |
Pulmonary atresia | 13 (1.9) | |
Transposition of the great arteries | 13 (1.9) | |
Univentricular heart | 12 (1.7) | |
Truncus arteriosus | 7 (1.0) | |
Other complex atrioventricular conduction abnormalities and anomalous ventricular arterial connections | 5 (0.7) |
A total of 69 (10.0%) patients occurred postoperative SAE, of which 16 (2.3%) patients had two or more SAEs. The SAEs included arrhythmia requiring pharmacologic intervention (n = 23, 3.3%), pericardial effusion requiring surgical intervention or pericardial drainage (n = 12, 1.7%), pulmonary hemorrhag (n = 10, 1.4%), infection (n = 8, 1.2%), retroperitoneal hematoma (n = 8, 1.2%), arteriovenous fistula requiring surgical or transcatheter intervention (n = 6, 0.9%), secondary thoracotomy for hemostasis (n = 5, 0.7%), need for medicine or mechanical hemodynamic support (n = 4, 0.6%), unplanned transfusion (n = 4, 0.6%), anaphylactic reaction (n = 3, 0.4%), renal compromise (n = 3, 0.4%), pseudoaneurysm requiring surgical or transcatheter intervention (n = 3, 0.4%), complete heart block (n = 2, 0.3%), sudden cardiac arrest within 24 hours after operation (n = 2, 0.3%), death related to procedural complication (n = 2, 0.3%), hemothorax requiring thoracentesis (n = 1, 0.1%) and coronary artery thrombosis (n = 1, 0.1%).
There were significant differences in 12 variables between SAE group and non-SAE
group, including BMI, NT-proBNP, LDH, cTnI, Urea, NEUT, heart failure, pulmonary
artery hypertension, severity of congenital heart disease, procedure type, ASA
score, and access location (p
Variable | non-SAE (n = 432) | SAE (n = 51) | p-value | |
Age (years) |
43 (22) | 44 (25) | 0.310 | |
Gender |
0.164 | |||
Male | 152 (35.2) | 23 (45.1) | ||
Female | 280 (64.8) | 28 (54.9) | ||
Height (cm) |
1.60 (0.12) | 1.61 (0.15) | 0.120 | |
Weight (kg) |
57.0 (13.7) | 55.7 (19.0) | 0.180 | |
BMI (kg/m |
22.4 (4.7) | 21.0 (5.7) | 0.010 | |
HR (beats/min) |
77 (14) | 78 (22) | 0.300 | |
SP (mmHg) |
118 (19) | 112 (27) | 0.148 | |
Hospital sources |
0.309 | |||
Outpatient service | 363 (84.0) | 40 (78.4) | ||
Emergency department | 69 (16.0) | 11 (21.6) | ||
Smoking history |
0.508 | |||
Yes | 27 (6.3) | 2 (3.9) | ||
No | 405 (93.7) | 49 (96.1) | ||
Alcohol history |
0.335 | |||
Yes | 27 (6.3) | 5 (9.8) | ||
No | 405 (93.7) | 46 (90.2) | ||
Diabetes |
0.255 | |||
Yes | 15 (3.5) | 4 (7.8) | ||
No | 417 (96.5) | 47 (92.2) | ||
Hypertension |
0.280 | |||
Yes | 46 (10.6) | 8 (15.7) | ||
No | 386 (89.4) | 43 (84.3) | ||
Coronary heart disease |
0.060 | |||
Yes | 59 (13.7) | 12 (23.5) | ||
No | 373 (86.3) | 39 (76.5) | ||
Heart failure |
0.012 | |||
Yes | 14 (3.2) | 6 (11.8) | ||
No | 418 (96.8) | 45(88.2) | ||
Cerebrovascular disease |
0.602 | |||
Yes | 20 (4.6) | 1 (2.0) | ||
No | 412 (95.4) | 50 (98.0) | ||
COPD |
0.361 | |||
Yes | 3 (0.7) | 1 (2.0) | ||
No | 429 (99.3) | 50 (98.0) | ||
Pulmonary artery hypertension |
0.002 | |||
Yes | 150 (34.7) | 29 (56.9) | ||
No | 282 (65.3) | 22 (43.1) | ||
Cyanosis |
0.056 | |||
Yes | 12 (2.8) | 4 (7.8) | ||
No | 420 (97.2) | 47 (92.2) | ||
Anemia |
0.253 | |||
Yes | 9 (2.1) | 3 (5.8) | ||
No | 423 (97.9) | 49 (94.2) | ||
Disease Severity |
||||
Moderate | 343 (79.4) | 28 (54.9) | ||
Severe | 89 (20.6) | 23 (45.1) | ||
Procedure type |
0.019 | |||
Diagnostic | 94 (21.8) | 4 (7.8) | ||
Diagnostic and interventional | 338 (78.2) | 47 (92.2) | ||
Procedure risk category |
0.214 | |||
Mild | 248 (57.4) | 26 (51.0) | ||
Moderate | 97 (22.5) | 17 (33.3) | ||
Severe | 87 (20.1) | 8 (15.7) | ||
Degree of anesthesia |
0.459 | |||
Local anesthesia | 406 (94.0) | 46 (90.2) | ||
General anesthesia | 26 (6.0) | 5 (9.8) | ||
ASA score |
||||
1–2 | 382 (88.4) | 30 (58.8) | ||
3 | 35 (8.1) | 5 (9.8) | ||
4–5 | 15 (3.5) | 16 (31.4) | ||
Access location |
0.014 | |||
Arterial | 15 (3.5) | 6 (11.8) | ||
Venous | 283 (65.5) | 36 (70.6) | ||
Both | 134 (31.0) | 9 (17.6) | ||
Potassium (mmol/L) |
3.68 (0.34) | 3.67 (0.53) | 0.675 | |
Magnesium (mmol/L) |
0.83 (0.08) | 0.82 (0.07) | 0.465 | |
Calcium (mmol/L) |
2.31 (0.23) | 2.28 (0.22) | 0.194 | |
NT-proBNP (pg/mL) |
54.22 (126.67) | 242.9 (1044.78) | ||
cTnI (mg/L) |
0.004 (0.01) | 0.006 (0.05) | 0.007 | |
AST (U/L) |
19.1 (7.3) | 19.1 (12.3) | 0.296 | |
LDH (U/L) |
163 (47) | 189 (70) | ||
Scr ( |
66 (16) | 69 (19) | 0.098 | |
Urea (mmol/L) |
4.96 (1.76) | 5.40 (2.96) | 0.041 | |
UA (mmol/L) |
303 (117) | 323 (144) | 0.056 | |
TG (mmol/L) |
1.21 (0.56) | 1.21 (0.61) | 0.420 | |
TC (mmol/L) |
3.16 (1.66) | 3.24 (1.50) | 0.180 | |
HDL-C (mmol/L) |
1.35 (0.45) | 1.33 (0.32) | 0.735 | |
LDL-C (mmol/L) |
2.42 (0.58) | 2.31 (0.99) | 0.945 | |
RBC (10 |
4.4 (0.7) | 4.2 (0.5) | 0.183 | |
NEUT (%) |
58 (14) | 63 (17) | 0.001 | |
HB (g/L) |
132 (24) | 133 (29) | 0.881 | |
Hs-CRP (mg/L) |
0.68 (1.12) | 0.76 (1.9) | 0.411 | |
D-D (mg/L) |
0.21 (0.16) | 0.22 (0.20) | 0.523 | |
Abbreviations: SAE, serious adverse event; BMI, body mass index; HR, heart rate;
SBP, systolic blood pressure; COPD, chronic obstructive pulmonary disease; ASA,
American Society of Anesthesiology; NT-proBNP, N-terminal pro-B-type natriuretic
peptide; cTnI, cardiac troponin I; AST, aspartate transferase; LDH, lactate
dehydrogenase; Scr, serum creatinine; Urea, serum urea; UA, uric acid; TG,
triglycerides; TC, serum total cholesterol; LDL-C, low-density lipoprotein
cholesterol; HDL-C, high-density lipoprotein cholesterol; RBC, red blood cells;
NEUT, neutrophil; HB, hemoglobin; hs-CRP, high-sensitivity C-reactive protein;
D-D, plasma D-dimer. |
Multivariate analysis results showed that significant predictors of SAE included
disease severity, procedure type, pulmonary artery hypertension, cTnI, and
NT-proBNP (Table 3). The final established SAE risk calculation formula was
logit(p) = –6.134 + 0.992
Variable | B | SE | Wald | p | OR | 95% CI | |
---|---|---|---|---|---|---|---|
Lower limit | Upper limit | ||||||
Pulmonary artery hypertension | 0.992 | 0.393 | 6.381 | 0.012 | 2.696 | 1.249 | 5.818 |
Disease Severity | 1.459 | 0.375 | 15.130 | 4.301 | 2.062 | 8.970 | |
Procedure type | 2.324 | 0.600 | 14.981 | 10.217 | 3.149 | 33.148 | |
cTnI | 1.436 | 0.400 | 12.893 | 4.205 | 1.920 | 9.208 | |
NT-proBNP | 1.537 | 0.382 | 16.226 | 4.652 | 2.202 | 9.830 | |
Constant | –6.134 | 0.725 | 71.533 | 0.002 | - | - | |
Abbreviations: B (Beta), Regression coefficient; SE, standard error; NT-proBNP, N-terminal pro-B-type natriuretic peptide; cTnI, cardiac troponin I; -, not applicable; CI, confidence interval; OR, odds ratio. |
We converted the model into a scoring system. The weight of the predictor with
the smallest
Variable | Points assigned | ||
Pulmonary artery hypertension | |||
No | 0 | 0 | |
Yes | 0.992 | 1 | |
Disease Severity | |||
Moderate | 0 | 0 | |
Severe | 1.459 | 1 | |
Procedure type | |||
Diagnostic | 0 | 0 | |
Diagnostic and interventional | 2.324 | 2 | |
cTnI | |||
0 | 0 | ||
1.436 | 1 | ||
NT-proBNP | |||
0 | 0 | ||
1.537 | 2 | ||
Total score | - | 0–7 | |
Abbreviations: SAE, serious adverse event; |
The C-statistic for the incidence of SAE in the derivation and validation
cohorts was 0.840 (95% CI, 0.779–0.901) and 0.911 (95% CI, 0.850–0.973),
respectively, indicating that the model had a good degree of discrimination
(shown in Fig. 1 and Fig. 2). In addition, the model showed good calibration,
according to the p values of the Hosmer-Lemeshow goodness-of-fit test in
the modeling group (
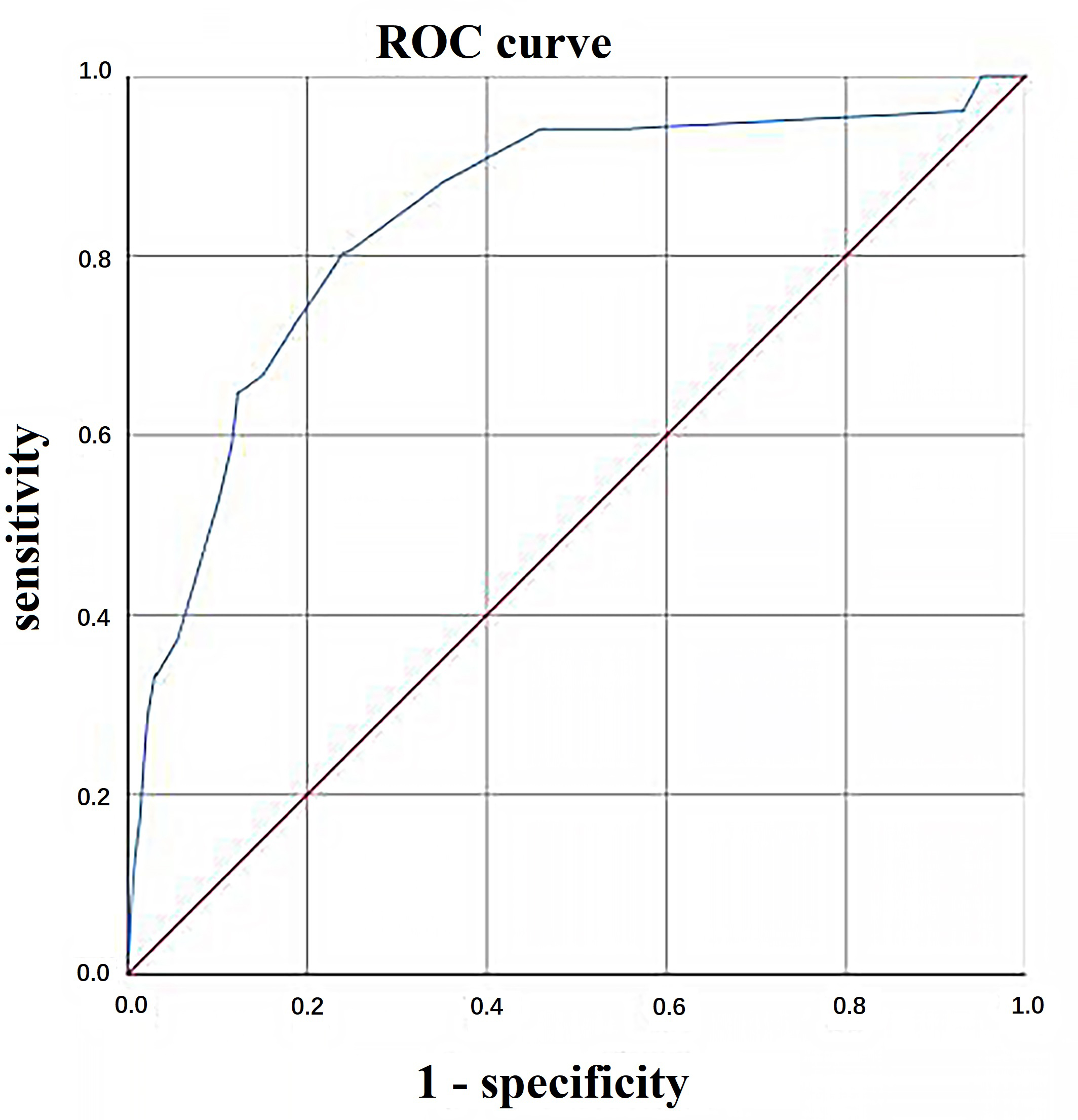
Area under the ROC curve (AUC) plots for prediction model fitted on development sample. ROC, receiver operating characteristic.
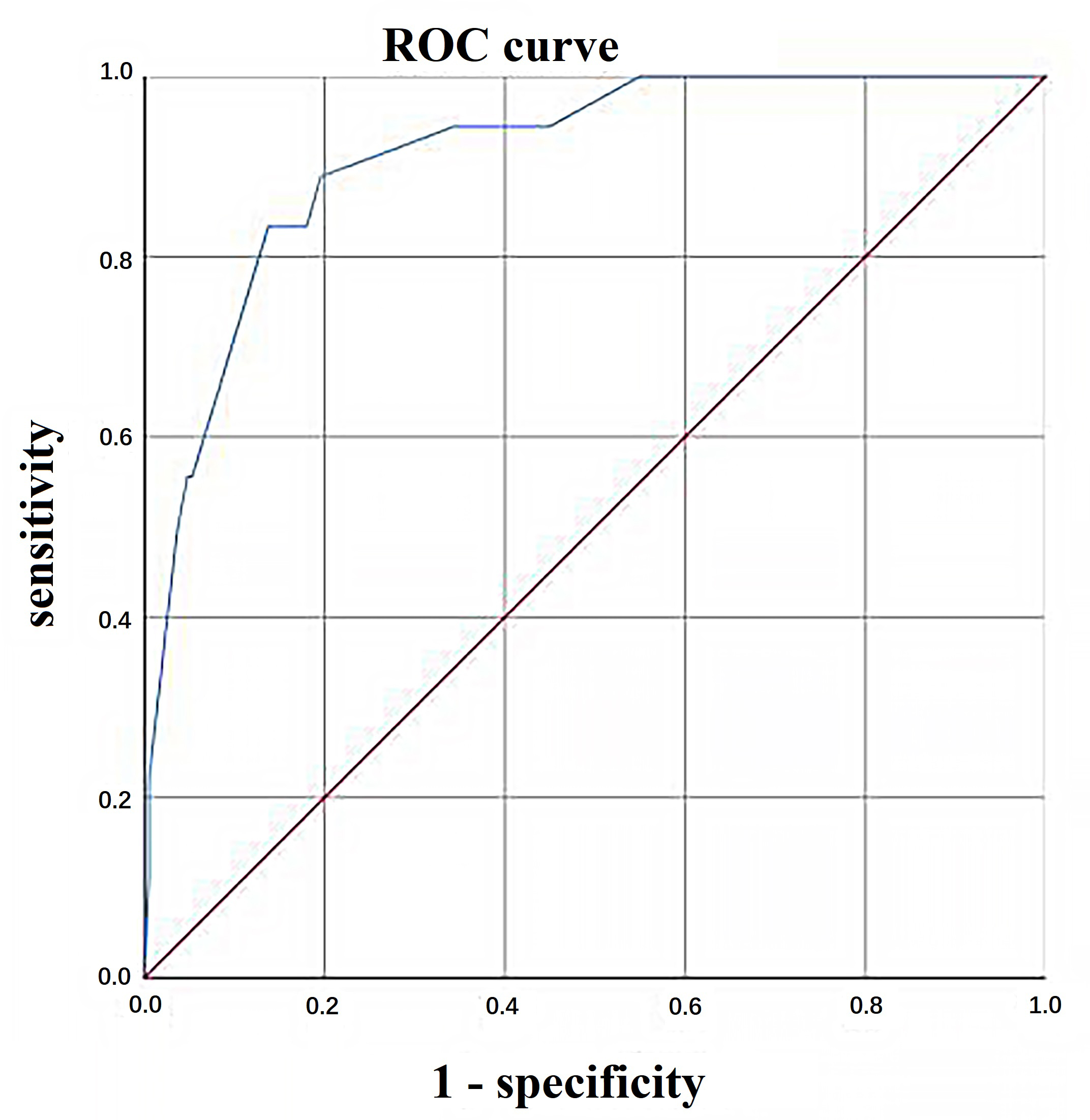
Area under the ROC curve (AUC) plots for prediction model fitted on validation sample. ROC, receiver operating characteristic.
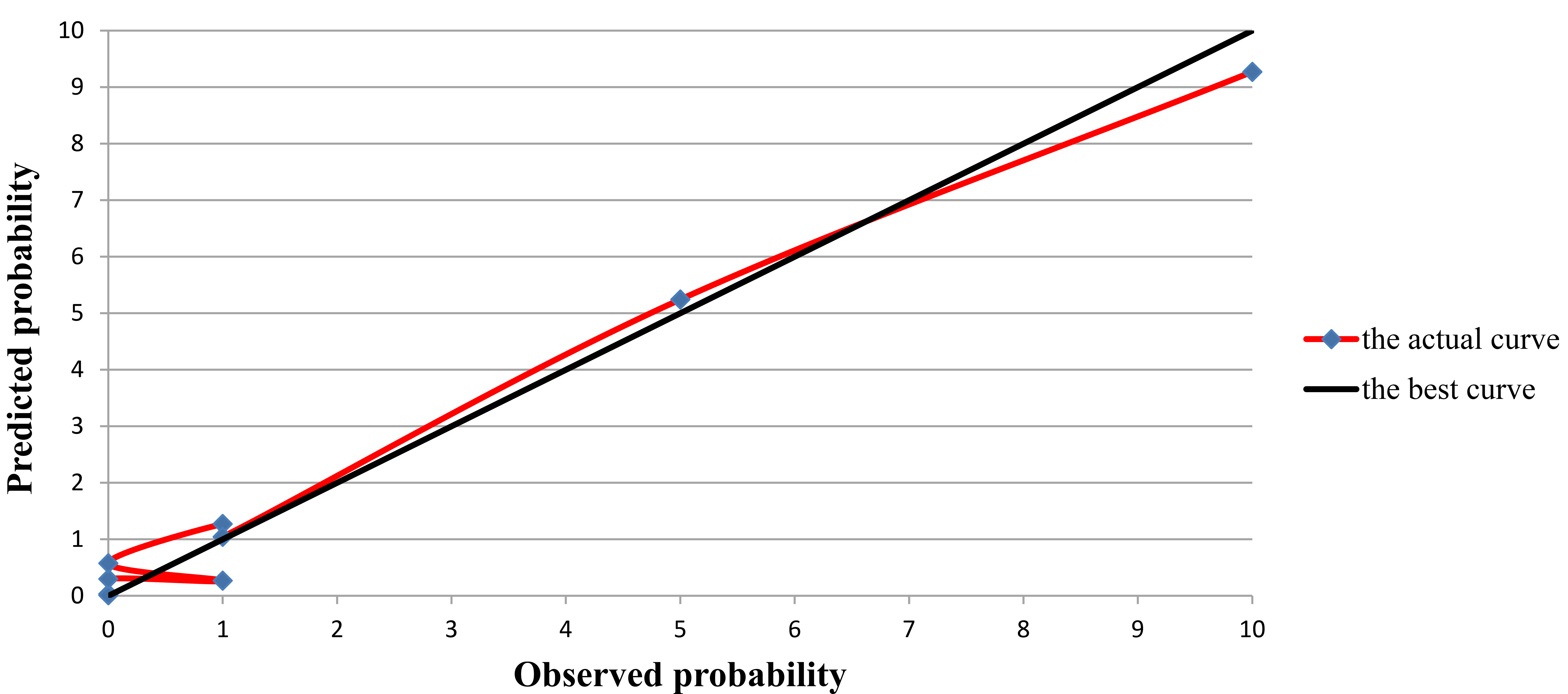
Calibration curve for predicting the probability of SAE occurrence and the actual probability of SAE occurrence after cardiac catheterization in moderate and severe ACHD patients (The black line is the best curve and the red line is the actual curve).
To further simplify the SAE risk assessment model, the derivation cohort was
divided into two groups: SAE low-risk group (0–3 points) and SAE high-risk group
(4–7 points) according to the optimal cut-off value of 3.5 in the ROC curve of
the scoring model [14]. In the derivation cohort, the incidence of SAE observed
in patients in the low-risk and high-risk groups was 3.6% (13/358) and 30.4%
(38/125), respectively, and the difference between the two groups was
statistically significant (
Compared with CRISA model, we found that our risk prediction model had better application value for its lower N2LL, AIC, BIC, and higher AUC (Table 5).
Risk prediction model | N2LL | AIC | BIC | AUC |
---|---|---|---|---|
CRISA model | 92 | –532 | –502 | 0.777 |
Developed model | 78 | –563 | –543 | 0.911 |
Abbreviations: CRISA, Catheterization RISk in Adult patients; AIC, Akaike’s Information Criteria; AUC, Area under the receiver operator curve; BIC, Schwarz’s Bayes Information Criteria; N2LL, –2log Likelihood (an assessment for model fit). |
Studies have shown that there are more than 1 million ACHD patients in the
United States and Canada, and the number of moderate and severe ACHD patients
increases rapidly [17]. Although the survival rate of ACHD is improved due to the
development of cardiac catheterization techniques, serious complications and
other unplanned adverse events after surgery are not rare, such as malignant
arrhythmia and stroke, which will affect the patients’ clinical outcome, and
sequentially increase the economic burden on them. In this study, we found a 10%
incidence of SAE after catheterization in 690 patients with moderate and severe
ACHD. In addition, we successfully developed and validated a risk prediction
model for SAE and the model showed good discrimination (c statistic = 0.911) and
calibration ability (
Up to now there has not been a SAE risk prediction tool for ACHD patients after cardiac catheterization in China. Many studies focused on the risk factors of ACHD and postoperative complications [18, 19, 20]. There were some risk prediction models developed in western countries.
Taggart NW et al. [7] developed a CRISA risk prediction model to predict the overall risk of SAE for ACHD patients with cardiac catheterizations, in which eight risk factors were included. Compared with this model, our model was more comprehensive, including not only above risk factors, but also other indicators such as past history and laboratory tests. Furthermore, it was worth noting that in CRISA model the types of procedure were classified into three categories: diagnostic, interventional and hybrid procedure. However, we found there was no separate interventional catheterization in China, because patients usually underwent intervention followed diagnostic procedure. Moreover, our research showed that our model was superior than the CRISA model in the comparison of model complexity and fit (Table 5).
Stefanescu Schmidt et al. [8] constructed a risk prediction model for
major adverse events (MAE) after ACHD catheterization. Three features
distinguished our model from their model. First of all, in the validation of the
model, our model had a larger C-statistic (0.911
Learn et al. [9] developed the model of congenital heart disease adjustment for risk method for adults with congenital heart disease (CHARM-ACHD), and claimed that adults underwent cardiac catheterization in pediatric hospitals in the past had fewer adverse events (4%). The model was included hemodynamic vulnerability indicators. However, in China, not every ACHD patient but severe patients are implemented comprehensive hemodynamic monitoring in ICU. Moreover, the application value of this model had not been validated in a separate cohort.
The SAE risk prediction model we constructed including three preoperative variables of pulmonary artery hypertension, NT-proBNP, cTnI, and two procedural variables of procedure type and disease severity. Pulmonary artery hypertension was a relatively common complication of congenital heart disease and associated with the size and nature of cardiac defects as well as environmental and genetic factors, accounting for approximately 10% of adult cases [21]. Compared with ACHD patients without pulmonary artery hypertension, pulmonary artery hypertension patients had a 2-fold increase in all-cause mortality and a 3-fold increase in the incidence of heart failure and arrhythmia, which somewhat increased the difficulty of catheterization procedures, such patients had a greater risk of postoperative SAE, and even experienced clinical deterioration after catheterization [22]. Type of procedure significantly predicted SAE, and our study found that the risk of SAE following diagnostic and interventional procedure was 2.324 times higher than diagnostic catheterization alone. At present, interventional therapy is widely used for ACHD patients to close intracardiac shunts, relieve obstructive valvular disease, stent stenotic vessels, replace and repair dysfunctional valves [23]. Compared with diagnostic catheterization, it will cause greater physical damage and a higher risk of postoperative complications.
Our result showed that patients with severe congenital heart disease had 1.459 times higher risk of SAE than moderate patients, which suggested that severity of ACHD was an important risk factor. In addition, our model included two biomarkers: NT-proBNP and cTnI. Preoperative NT-proBNP levels can be used as a marker to evaluate the hemodynamic and functional status of patients. Gessler P et al. [24] proposed that higher NT-proBNP was associated with ventricular dysfunction and ventricular volume overload in patients with ACHD. cTnI was a specific and sensitive marker [25], and Immer FF et al. [26] found that the maximum cTnI value within the first 24 hours of cardiac surgery can predict the serious postoperative complications, as well as the duration of intensive care treatment.
In our study, we confirmed that our model had good discrimination and calibration, and established simple risk stratification aimed at providing personalized risk counseling to patients before cardiac catheterization. Although the risks of catheterization may vary by random events, hospitals or surgeons, the risk score had a strong risk prediction power. Due to the intuitive and quantitative advantages of risk stratification, medical staff can use it to assess patients at high risk of SAE, so that personalized treatment can be adopted accordingly.
There were some limitations in this study. Firstly, the model included the overall SAE risk of moderate and severe ACHD patients after cardiac catheterization, lacking of the ability to predict risk of a specific kind of SAE. Secondly, the sample was limited to one hospital, therefore the model needs to be further validated in multiple center patients. Thirdly, we did not assess SAE risk of discharged patients but mainly focused on those of inpatients. Consequently, some potential factors may be omitted for the sake of focus.
A total of 690 moderate and severe ACHD patients who underwent cardiac catheterization were analyzed to identify procedural risk factors. We provided a prediction model for the risk of SAE after cardiac catheterization with favorable discrimination and calibration. The risk score scale developed based on the model could predict high-risk patients and allow medical providers to implement individualized prevention when cardiac catheterization is performed in ACHD patients.
The data that support the findings of this study are available from [Wuhan Asian Heart Hospital] but restrictions apply to the availability of these data, which were used under license for the current study, and so are not publicly available. Data are however available from the authors upon reasonable request and with permission of [Wuhan Asian Heart Hospital].
JH and YZ participated in the conception and design of the study, as well as data analysis and manuscript writing. WZ designed the research study and performed the research. JL and PP analyzed the data. All authors contributed to editorial changes in the manuscript. All authors read and approved the final manuscript.
The study was approved by the Ethics Committee of Wuhan University of Science and Technology (reference number: 2022116). All procedures involving human participants were in accordance with the Declaration of Helsinki. Written informed consent from participants was not required for the study according to the local guidelines.
Not applicable.
This research received no external funding.
The authors declare no conflict of interest.