†These authors contributed equally.
Academic Editors: Vladimir Jakovljevic, Dragan M. Djuric and Brian Tomlinson
Background: Coronary heart disease is a highly prevalent inflammatory disease caused by coronary atherosclerosis. Numerous studies have revealed that perivascular adipose tissue is closely associated with atherosclerosis. Here, we conducted a comprehensive analysis of long non-coding RNAs and mRNAs differentially expressed in perivascular adipose tissue in patients with coronary heart disease. Methods: We conducted Gene Ontology term and Kyoto Encyclopedia of Genes and Genomes pathway enrichment analyses of the differentially expressed genes. Furthermore, single sample gene set enrichment analysis, immune infiltration analysis, and co-expression analysis of differentially expressed long non-coding RNAs and immune gene sets were performed. Finally, the starBase and miRTarBase databases were used to construct a competing endogenous RNA network. Results: The results show that aortic perivascular adipose tissue has higher inflammation and immune infiltration levels in patients with coronary heart disease. Dysregulated long non-coding RNAs may be related to immunity, inflammation, and hypoxia. Conclusions: The findings of this study provide new insights into atherosclerosis and coronary heart disease.
Coronary heart disease (CHD), a common cardiovascular disease, is one of the major causes of death worldwide [1, 2]. Atherosclerosis (AS) is the common pathological basis of CHD, and its etiology is complex, involving endothelial cell dysfunction, immune response, oxidative stress, and other mechanisms [3, 4, 5]. Many studies in recent years have discovered that Perivascular adipose tissue (PVAT) dysfunction is implicated in the onset and progression of AS via a paracrine or endocrine pathway [6, 7]. PVAT covers the majority of blood vessels, including large arteries and veins, as well as small and resistive vessels, under physiological conditions, PVAT exhibits anti-inflammatory roles and is important in vasodilation regulation [8]. However, under pathophysiological situations such as obesity, PVAT becomes dysfunctional and promotes the infiltration of inflammatory immune cells and local oxidative stress, thereby leading to the dysfunction of the underlying vascular smooth muscle cells and endothelial cells [8, 9, 10]. Hence, dysfunctional PVAT promotes cardiovascular disease progression.
So far, many studies have found substantial variations in gene expression in
PVAT at sites of AS [11, 12, 13]. Tang et al. [14] found that Ribosomal Protein S3A (RPS3A)
expression is decreased in the epicardial adipose tissue of CHD patients and the
PVAT of ApoE
However, current studies in PVAT in the field of CHD or AS have mainly focused on genes encoding proteins, with few studies on non-coding RNAs. There is mounting evidence that non-coding RNAs play a prominent role in the development of cardiovascular diseases [16, 17, 18]. Several studies have demonstrated different expression profiles of lncRNAs during CHD [19, 20]. In this study, we analyzed the differentially expressed mRNAs and lncRNAs in patients with CHD based on the study of Mazzotta et al. [13]. And through the method of bioinformatics analysis to speculate the possible role of these unknown function lncRNAs in patients with CHD. This study provides new insights into AS and CHD and lays the foundation for the development of targeted therapies.
Gene Expression Omnibus (GEO) (https://www.ncbi.nlm.nih.gov/geo/) is a
functional public genomic data repository. We downloaded the gene expression
microarray datasets GSE152326 and GSE21545 from the GEO database. The GSE152326
dataset contains data of 10 perivascular adipose tissue samples collected from
the proximal aorta and left IMA (LIMA) of 5 patients during coronary artery
bypass surgery [13]. Clinical features of included CHD patients can be seen in
Supplementary Table 1. The GSE21545 dataset contains data of 97
peripheral blood mononuclear cells (PBMC) samples collected from patients with AS
[21]. Clinical features of 97 patients with AS can be seen in
Supplementary Table 2. Datasets were annotated using Perl 5 (version
30). The probe IDs were replaced with the Entrez ID or gene symbol. The median
expression level of all probes was analyzed if more than one probe corresponded
to one gene. For GSE152326, we re-annotated the gene types using HGNC BioMart
(https://biomart.genenames.org/) and obtained the expression profiles, including
4387 lncRNAs and 18365 protein-coding genes. Differentially expressed genes
(DEGs) between the proximal aorta PVAT and LIMA PVAT were identified using the
Limma package in R (version 3.6.3). The threshold for statistical significance
was set as
Gene Ontology (GO) term functional annotation and Kyoto Encyclopedia of Genes and Genomes (KEGG) pathway enrichment analyses were
conducted using the “Cluster Profiler” package in R [22]. Sangerbox 3.0
(http://vip.sangerbox.com/home.html) an online bioinformatics visualization
platform for visualizing results. Results with p
Single sample gene set enrichment analysis (ssGSEA) was performed on immune cell types in samples from the GSE152326 dataset
using the GSVA R package (version 3.6.3) [26]. A meta-gene for each immune cell
type was scored based on the ssGSEA score, according to the method of Zhang
et al. [27]. Downloaded cell markers for 22 immune cells including B
cells, macrophages, and natural killer cells from the cell-specific marker gene
database PanglaoDB (https://panglaodb.se) [28]. The
Wilcoxon test was used to evaluate the differences between the proximal aorta and
LIMA artery groups. The results were visualized using Sangerbox 3.0, a free
online platform for data analysis (http://vip.sangerbox.com/home.html). Results
with p
Immune-related genes were retrieved from the Molecular Signatures Database
(MSigDB) (http://www.gsea-msigdb.org/gsea/index.jsp), including two gene sets,
immune response (M19817) and immune system process (M13664). Thereafter,
immune-related lncRNAs were defined based on the correlation analysis between the
mRNA expression level and lncRNA expression data (
The 97 PBMC samples from GSE21545 were used for prognosis analysis as the method of Liu et al. [30]. The 97 patients with AS were followed for an average of 44 months, and the ischemic events were defined as myocardial infarctions or ischemic strokes. Based on the expression value of each lncRNA in the microarray, we divided the patients into two groups and examined their prognosis. The prognosis of patients in each group was estimated by Kaplan-Meier, and the comparison of survival prognosis between the two groups was performed by log-rank test. To select the optimal expression cutoff for the most significant patient group, we grouped all 20th to 80th percentile expression values, tested for significant differences in survival outcomes between groups, and derived the lowest log-rank p value. Sangerbox 3.0 (http://vip.sangerbox.com/home.html) was used for statistics and visualization.
We predicted the subcellular localization of other lncRNAs by using the tool lncLocator (https://www.csbio.sjtu.edu.cn/bioinf/lncLocator). lncRNA sequences were obtained from the Ensembl (http://asia.ensembl.org/index.html) or UCSC (http://genome.ucsc.edu/) database. If one lncRNA gene symbol encoded multiple transcripts, we selected the ensemble canonical transcript sequence. The final results were analyzed and presented using GraphPad Prism 8 software (version 8.0; GraphPad Software, San Diego, CA, USA).
lncRNA–miRNA interactions were predicted using starBase v2.0 database
(http://starbase.sysu.edu.cn/index.php) [31]. The lncRNA–miRNA pair that
satisfies CLIP-Data
Human umbilical vein endothelial cells (HUVECs) were purchased from Sciencell
(USA, Cat. # 8000) and cultured in endothelial cell culture medium (ECM)
(Sciencell, USA, Cat. # 1001) with 5% fetal bovine serum, 1% endothelial cell
growth, and 1% antibiotic solution at 37 °C with 5% CO
We used the RNASimple Total RNA Kit (Cat. # DP419; Tiangen, China) to isolate
the total RNA according to the manufacturer’s instructions. The cDNA was prepared
using the Primescript RT Master Kit (RR036A; Takara, Japan) according to the
manufacturer’s instructions, and then quantitative real-time polymerase chain
reaction (qPCR) was carried out using the TB Green Premix Ex Taq™
II (RR820A; Takara, Japan).
Gene | Primer sequence | |
ICAM1 | Forward | 5′-AGCGGCTGACGTGTGCAGTAAT-3′ |
Reverse | 5′-TCTGAGACCTCTGGCTTCGTCA-3′ | |
VCAM1 | Forward | 5′-GATTCTGTGCCCACAGTAAGGC-3′ |
Reverse | 5′-TGGTCACAGAGCCACCTTCTTG-3′ | |
GAS5 | Forward | 5′-AAGCCTAACTCAAGCCATT-3′ |
Reverse | 5′-TTACCAGGAGCAGAACCA-3′ | |
H19 | Forward | 5′-GACAGGAGAGCAGAGACT-3′ |
Reverse | 5′-GCAGCGAGACTCCAGGAA-3′ | |
MIR22HG | Forward | 5′-GAGCCGCAGTAGTTCTTC-3′ |
Reverse | 5′-TCAATCCAGCCAGTGTCT-3′ | |
LINC01091 | Forward | 5′-GATCTGCTGTTAGAGGAGA-3′ |
Reverse | 5′-ATTTGCAGATGAAGTGATAC-3′ | |
Forward | 5′-CACCATTGGCAATGAGCGGTTC-3′ | |
Reverse | 5′-AGGTCTTTGCGGATGTCCACGT-3′ |
The gene expression levels in the PVAT of five subjects with CHD were analyzed
using a microarray; 643 genes were significantly differentially expressed between
the proximal aorta and LIMA as a result (p
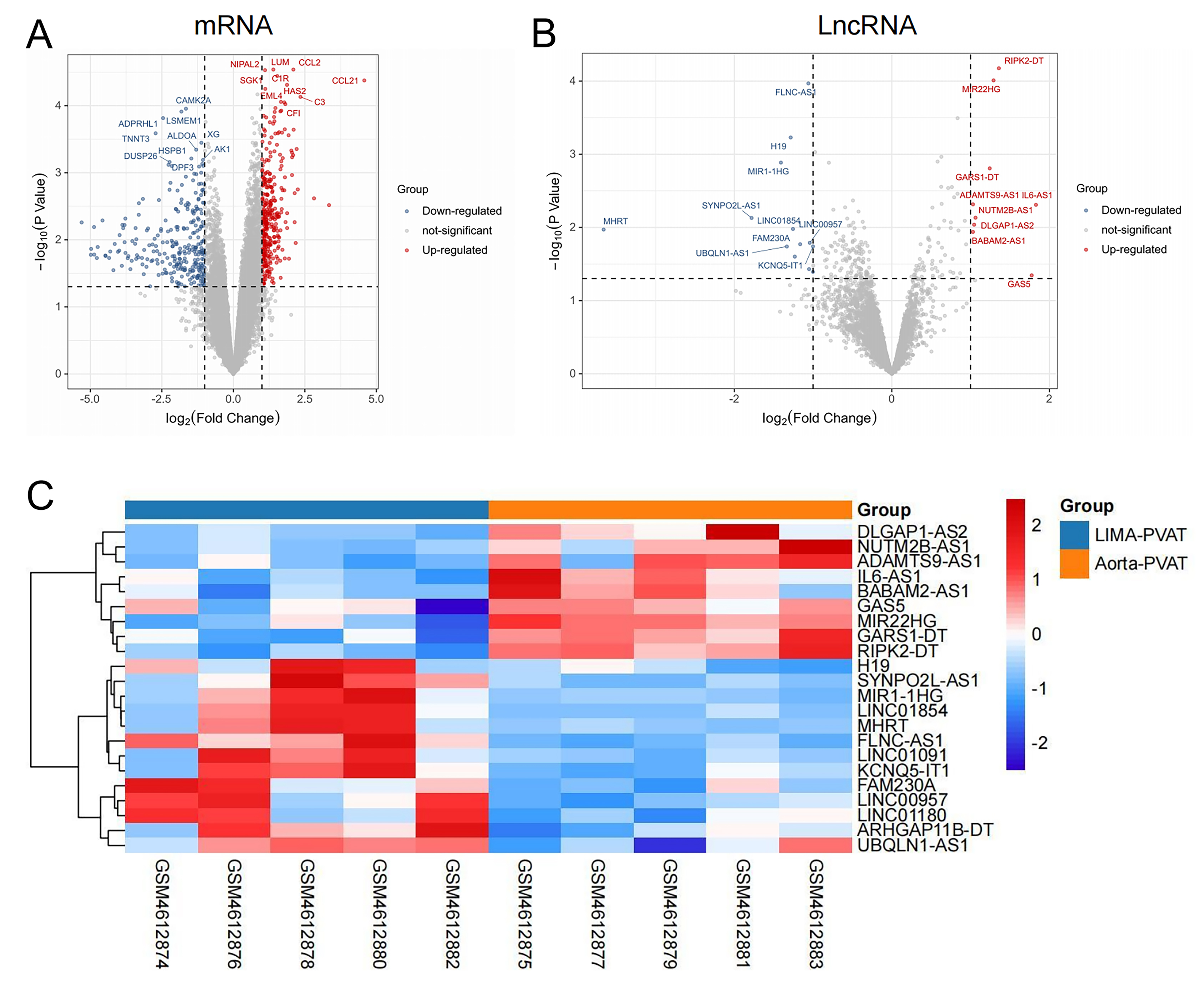
Differentially expressed genes in aortic and left internal mammary
artery (LIMA) perivascular adipose tissue (PVAT) in patients with coronary heart
disease (CHD). (A) Volcano plots of differentially expressed mRNAs. (B) Volcano
plots of differentially expressed lncRNAs. (C) Heatmap of differentially
expressed lncRNAs.
We then systematically assessed the potential biological functions of the DEGs
using the GO term and KEGG pathway enrichment analyses. The following GO terms in
the biological process (BP) category were enriched by the upregulated DEGs:
immune system process, immune response, regulation of immune system processes,
and response to cytokine (Fig. 2A). Consistent with these findings, the
upregulated KEGG pathway enrichment analysis revealed that several immune-related
pathways were enriched in aortic PVAT, including antigen processing and
presentation, complement and coagulation cascades, and Th17 cell differentiation
(Fig. 2B). In addition, several inflammation-related signaling pathways such as
the tumor necrosis factor (TNF) signaling pathway, nuclear factor kappa-B (NF-
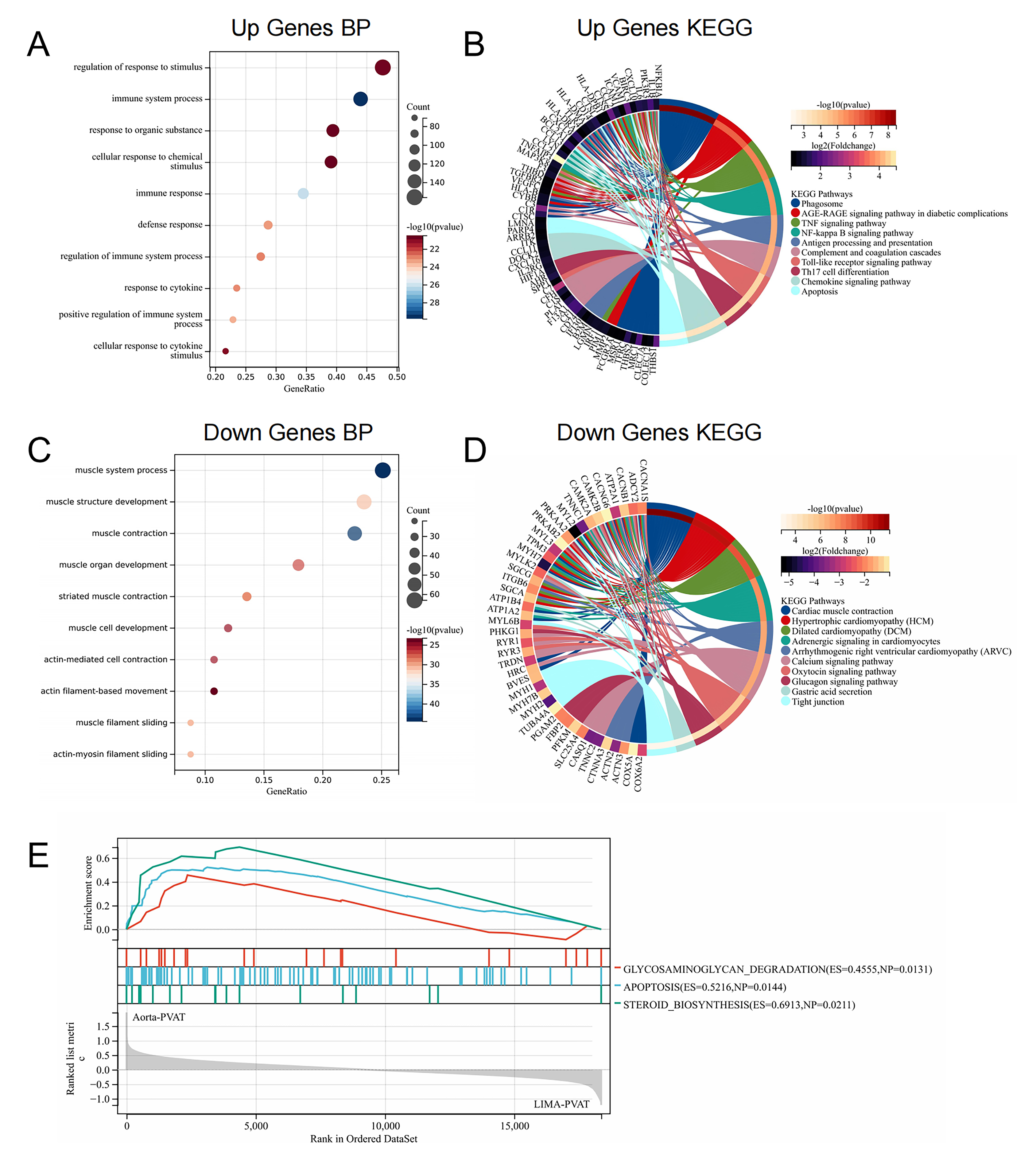
Enrichment analysis of differentially expressed genes. (A)
Biological processes enriched by upregulated genes. (B) Kyoto Encyclopedia of
Genes and Genomes (KEGG) pathway analysis of upregulated genes enrichment. (C)
Biological processes enriched by downregulated genes. (D) KEGG pathways enriched
by downregulated genes. (E) Upregulated GSEA in aortic perivascular adipose
tissue (PVAT) in patients with CHD. Pathway enrichment analysis results with
p
As the DEGs identified suggested that immune processes play an important role in PVAT dysregulation, we analyzed the infiltration level of immune cells in the PVAT of the aorta and LIMA using ssGSEA (Fig. 3). Notably, aortic PVAT had higher ssGSEA scores than LIMA PVAT. The ssGSEA scores of basophils, macrophages, mast cells, and cytotoxic T cells were significantly increased in aortic PVAT (Fig. 3B). Subsequently, we explored the potential relationships between the immunocytes using Pearson’s correlation analysis. Among all immunocytes, there was a strong correlation between ssGSEA scores of B and T cell subsets. There was a strong positive correlation between macrophages and eosinophils, a strong correlation between neutrophils and monocytes, and a negative correlation between cytotoxic T cells and mast cells (Fig. 3C).
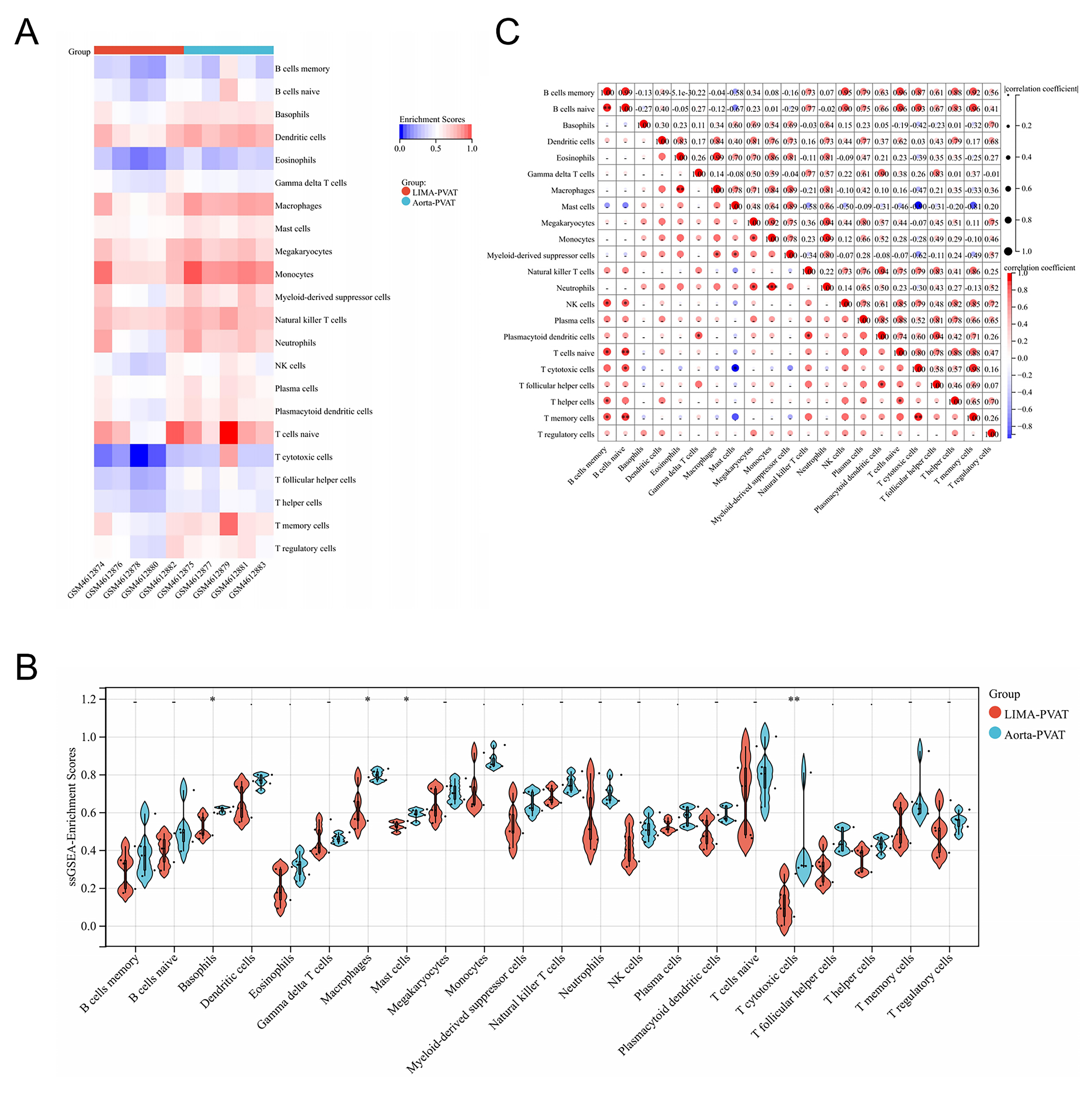
Analysis of immune cell infiltration of perivascular adipose tissue
(PVAT) in patients with coronary heart disease (CHD). (A) Heatmap of
single-sample gene set enrichment analysis (ssGSEA) enrichment scores of ten
samples. (B) Compositional differences of 22 immunocytes between aortic PVAT and
LIMA PVAT. (C) Correlation matrix of 22 immunocytes proportions. The Wilcoxon
test was used to evaluate the differences between the groups. The correlation
between immune cell ssGSEA scores was calculated using Pearson’s method. *
p
The above results suggest that the immune process is very important, so we wondered whether these differentially expressed lncRNAs are also related to immunity. To screen lncRNAs that may be related to immunity, we first calculated the correlation coefficients between the expression of lncRNAs and immune-related genes in the chip. Subsequently, we selected lncRNAs with a high correlation coefficient to construct immune-related lncRNA networks (Fig. 4). We identified nine lncRNAs corresponding to immune-related genes. Thereafter, we determined the enriched KEGG pathways of the mRNAs that were strongly correlated with immune-related lncRNAs. The results showed that genes co-expressed with FAM230A, UBQLN1-AS1, and BABAM2-AS1 were mainly enriched in cytokine-cytokine receptor-related pathways, as well as inflammation and immune-related pathways. Genes co-expressed with DLGAP-AS2 were enriched in some pathways that regulate T cell differentiation, such as that of Th1, Th2, and Th17. The genes co-expressed with LINC01180, ADAMTS9-AS1, and NUTMB-AS1 were enriched in the inflammatory and cell adhesion pathways. The genes co-expressed with IL6-AS1 were enriched in the B cell receptor pathway in addition to some pathways related to inflammation or cytokines. The genes co-expressed with GAS5 were mainly enriched in cellular signaling pathways related to inflammation and hypoxic injury.
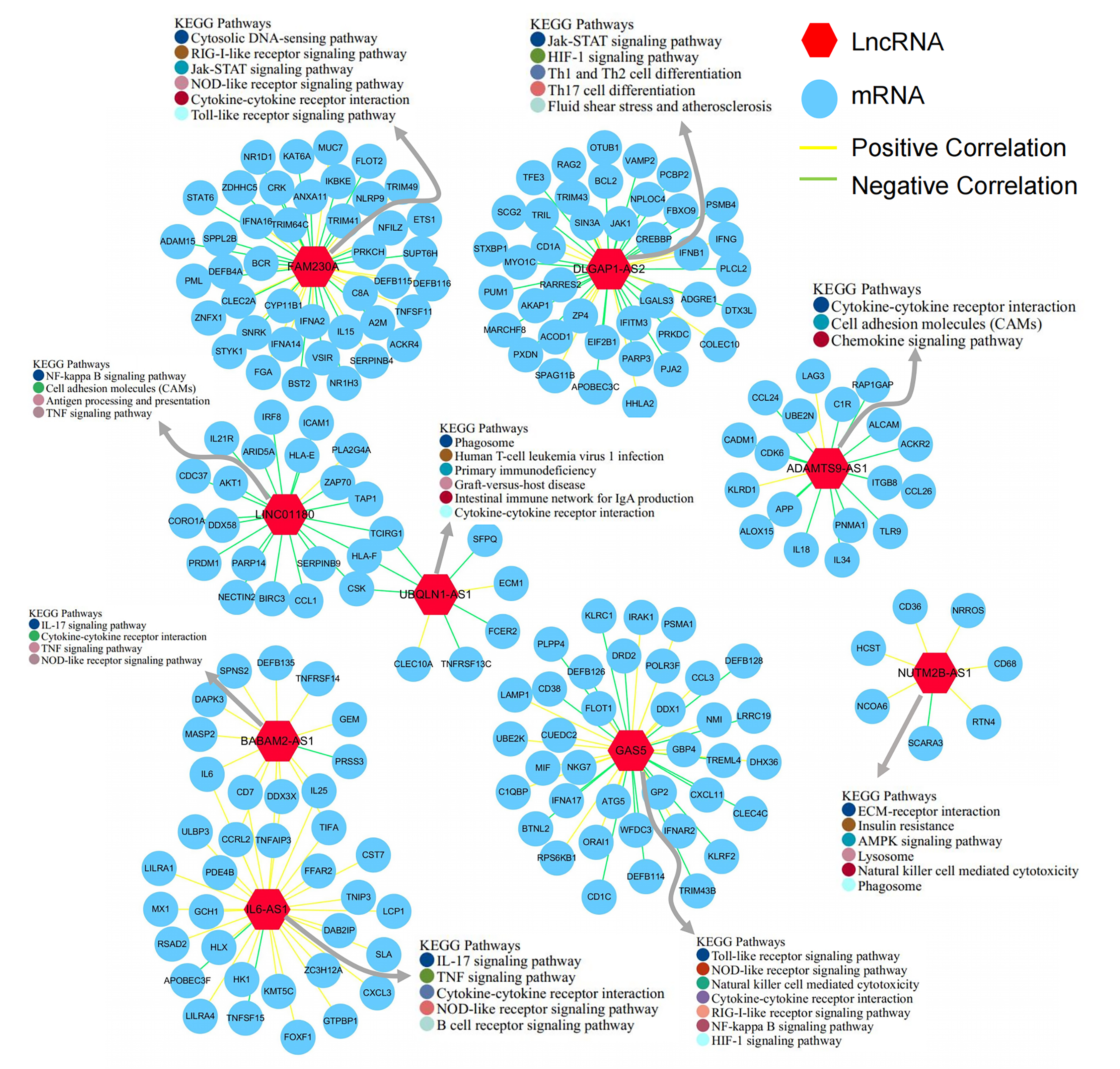
Analysis of immune-related lncRNAs. Co-expression network of
lncRNAs associated with immune genes, and Kyoto Encyclopedia of Genes and Genomes
(KEGG) pathway enrichment analyses of lncRNAs in the co-expression network. The
KEGG pathway enrichment analysis results with p
Subsequently, we analyzed the prognostic value for ischemic events of these
immune-related lncRNAs in microarray data containing PBMCs of 97 patients with AS
(Fig. 5). The results showed that LINC01180 may be a protective factor
in AS (p
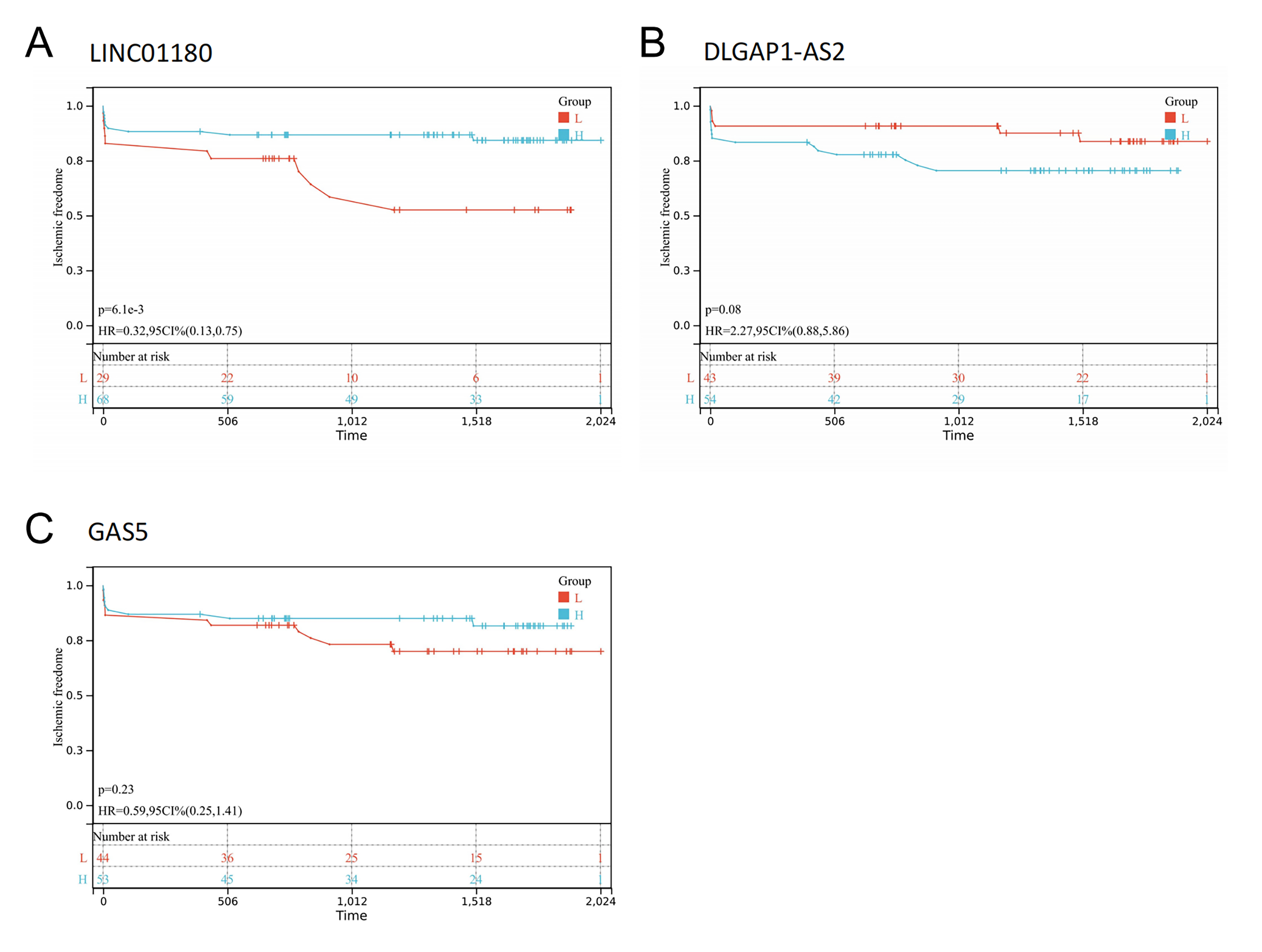
Prognosis analysis of immune-related lncRNAs. (A) Kaplan-Meier survival curves of LINC01180. (B) Kaplan-Meier survival curves of DLGAP1-AS2. (C) Kaplan-Meier survival curves of GAS5. The p-value was calculated using the log-rank test.
As lncRNA functions are closely associated with their subcellular localization [34]. We investigated the positions of the remaining 13 lncRNAs. Database prediction results showed that FLNC-AS1 and ARHGAP11B-DT localized to the nucleus, MIR1-1HG localized to exosomes, and the others localized to the cytoplasm or cytosol (Fig. 6A). Based on these results, we hypothesized that these lncRNAs participate in CHD or AS progression by acting as ceRNAs. To test this hypothesis, we constructed a ceRNA network based on the starBase and miRTarBase databases, comprising 3 lncRNAs, 40 miRNAs, and 50 mRNAs (Fig. 6B).
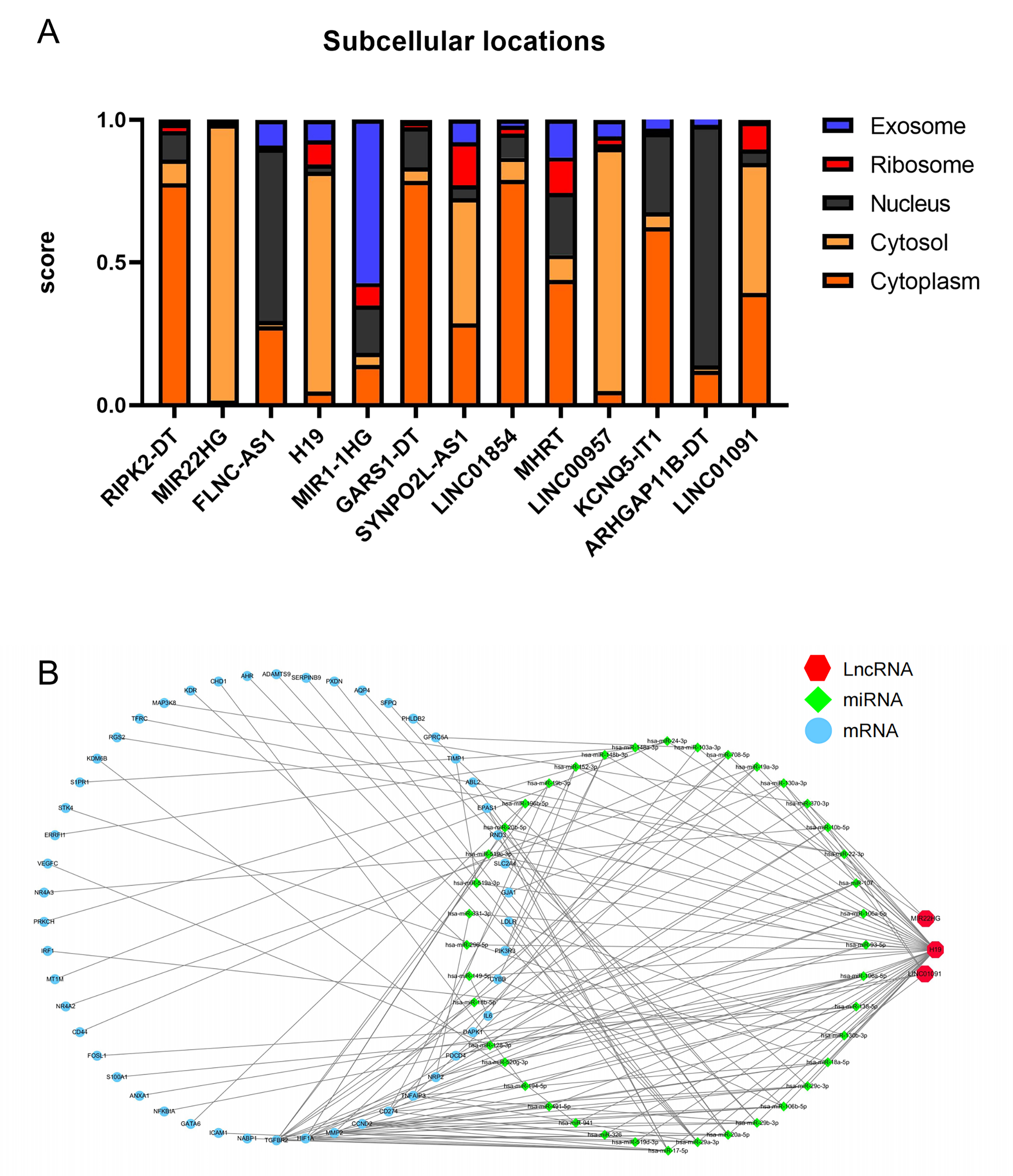
Construction of a ceRNA network. (A) Results of immune-unrelated lncRNAs’ cytoplasmic-nuclear localizations. (B) A lncRNA-associated ceRNA networks network.
lncRNA-associated ceRNA networks can influence the regulation of the related mRNA-encoding genes. By constructing the ceRNA network, the functions of the lncRNAs can be inferred. The KEGG pathway enrichment analysis was performed on the genes in the identified networks, and several pathways were found to be significantly enriched (Fig. 7). The KEGG pathway enrichment analysis suggested that the TNF signaling pathway, hypoxia inducible factor-1 (HIF-1) signaling pathway, FoxO signaling pathway, PI3K-Akt signaling pathway, and other signaling pathways may associated with the occurrence and development of CHD and AS (Fig. 7A). Notably, IL-6 and PI3K participate in several of these signaling pathways, indicating that they may be potential therapeutic targets for patients with AS (Fig. 7B,C,D).
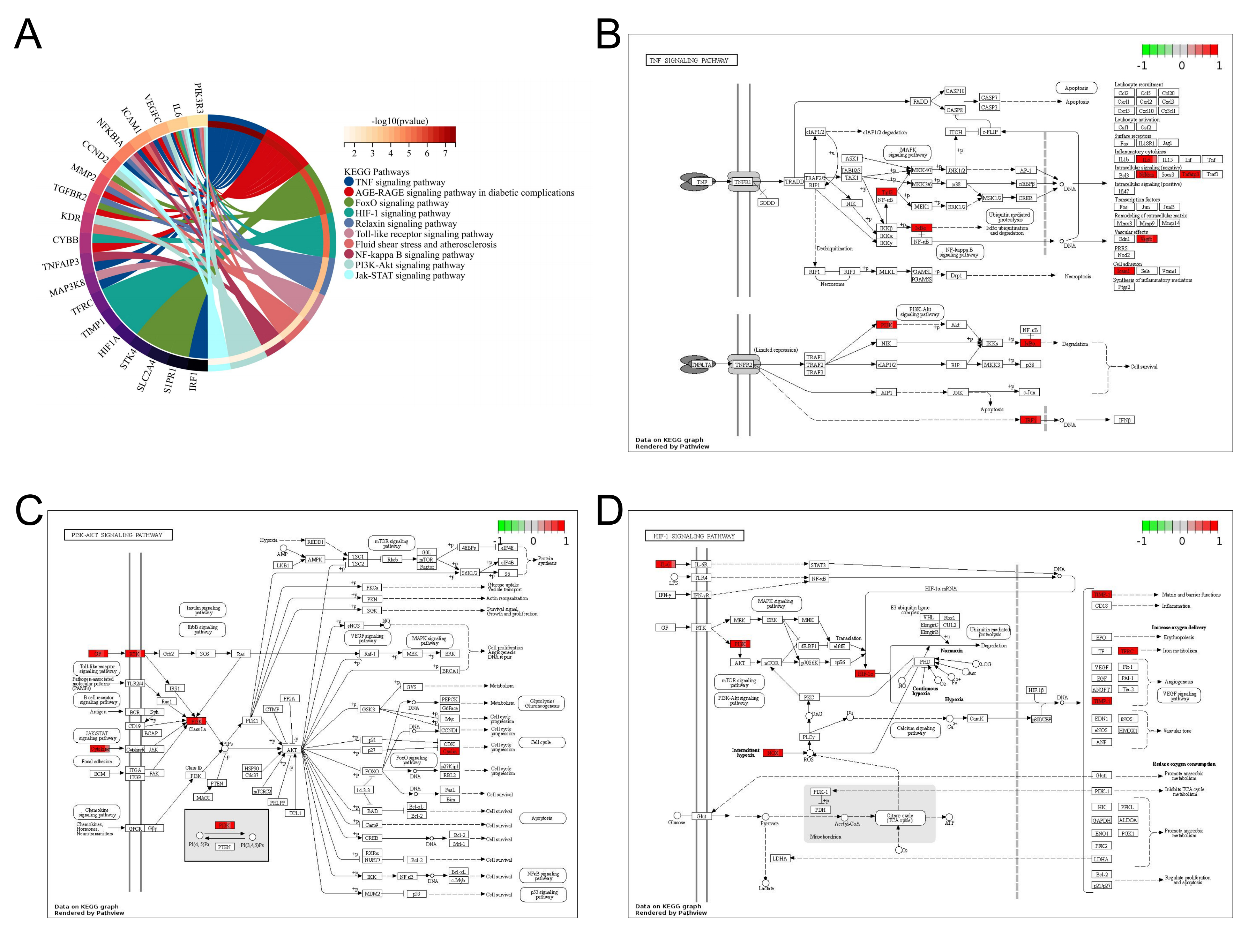
Enrichment analysis of mRNAs in the ceRNA network. (A) Kyoto
Encyclopedia of Genes and Genomes (KEGG) pathway enrichment analysis of mRNAs in
the ceRNA network. (B, C, D) Visualization of mRNAs present in the ceRNA network
in the TNF, PI3K-Akt, and HIF1 signaling pathways. KEGG pathway enrichment
analysis results with p
Finally, we used qPCR to validate the levels of the lncRNAs selected above (Fig. 8). ICAM1 and VCAM1 were significantly upregulated in HUVECs after hypoxia treatment (Fig. 8B). The results showed that GAS5, H19, and MIR22HG were upregulated in HUVECs after hypoxia treatment, whereas LINC01091 was downregulated (Fig. 8C).
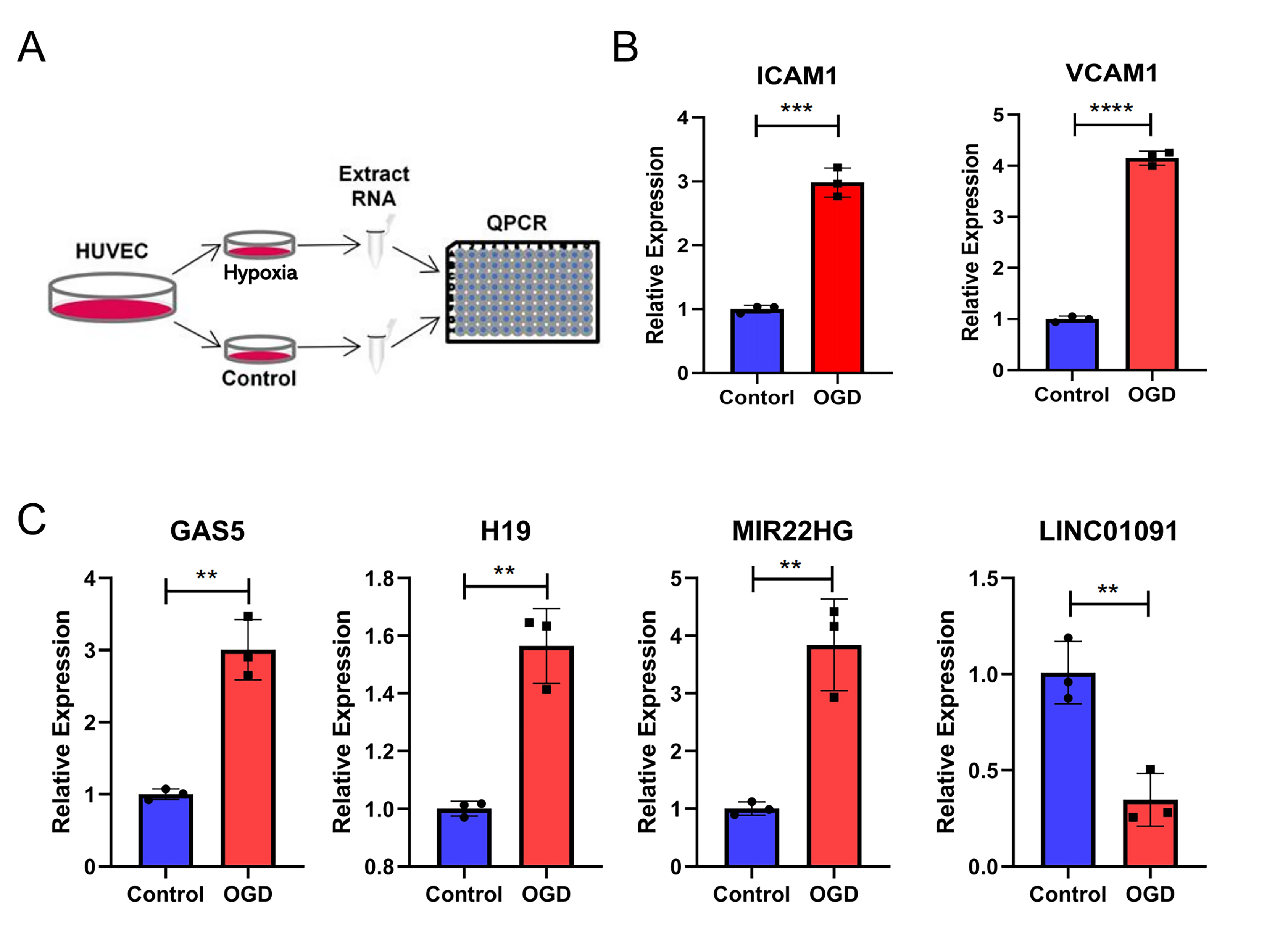
Verification of lncRNA levels in hypoxia-treated endothelial
cells by qPCR. (A) The experimental design of this study. (B) The expression of
ICAM1 and VCAM1 in human umbilical vein endothelial cells
(HUVECs) with hypoxia treatment. (C) The expression of GAS5,
H19, MIR22HG, and LINC01091 in HUVECs with hypoxia
treatment. Student’s t-test was used to compare the relative expression
differences between the groups. * p
Based on the chip data published by Mazzotta et al. [13], we did a more in-depth analysis. According to Mazzotta et al. [13], aortic PVAT from patients with CHD shows a higher degree of inflammation than LIMA PVAT, with higher levels of inflammatory factors such as IL-1, MCP-1, and IL-6, which positively correlate with the Gensini score. Our results showed that aortic PVAT in patients with CHD might be accompanied by higher immune cell infiltration. The expression of nine lncRNAs strongly correlated with the expression of immune-related genes, including FAM230A, NUTM2B-AS1, GAS5, ADAMTS9-AS1, BABAM2-AS1, DLGAP1-AS2, IL6-AS1, LINC01180, and UBQLN1-AS1. The differential expression of H19, LINC01091, and MIR22HG in patients with CHD may be related to oxidative stress and hypoxia. Hence, the aforementioned lncRNAs may be associated with AS and CHD and should be further investigated.
Recently, with the application of flow cytometry, immunofluorescence staining, and single-cell sequencing, our understanding of the diversity of immune cells in PVAT has considerably improved. As listed in Table 2 (Ref. [35, 36, 37, 38, 39, 40, 41]), PVAT mainly consists of T cells, B cells, NK cells, macrophages, neutrophils, dendritic cells, eosinophils, and mast cells [35, 36, 37, 38, 39, 40, 41]. Numerous studies have demonstrated that PVAT secretes various cytokines and plays an important role in multiple cardiovascular pathophysiological processes by inducing immune cell infiltration or directly affecting smooth muscle cells and vascular endothelial cells [9, 42]. Srikakulapu et al. [36] discovered that most B cells in and around the aorta are derived from PVAT, with a substantial proportion of these B cells belonging to the B-1 subgroup, and that local IgM production may play a role in AS protection. Numaguchi et al. [43] compared PVAT around the coronary artery (CA-PVAT) and the internal thoracic artery (ITA-PVAT) and observed that M1 macrophages increased more in CA-PVAT than in ITA-PVAT, whereas the ratio of M2 to M1 macrophages decreased. Therefore, it is necessary to explore the infiltration of immune cells in PVAT in disease states.
Author | Immune cell type | Sample source | Method | References |
Farias-Itao et al. | B cells; T cells; macrophages | Human | immunohistochemistry | [35] |
Srikakulapu et al. | B cells; T cells | Human; Mouse | Flow cytometry | [36] |
Withers et al. | Eosinophils | Mouse | Flow cytometry | [37] |
Sagan et al. | B cells; T cells; Macrophages; Granulocytes; NK cells; Dendritic cells | Human | Flow cytometry | [38] |
Kumar et al. | B cells; T cells; Macrophages; NK cells; Mast cells; Neutrophils | Rats | Flow cytometry | [39] |
Nosalski et al. | B cells; T cells; Macrophages; NK cells | Rats | Flow cytometry | [40] |
da Silva et al. | B cells; T cells; M1 Macrophages; M2 Macrophages | Mouse | Flow cytometry | [41] |
The score of immune cells in each sample can be calculated by combining chip
data and bioinformatic analysis methods [44]. We evaluated the infiltration
levels of immune cells using the ssGSEA and revealed significant differences
(p
In addition to immunity and inflammation, epigenetics plays an important role in AS [50]. In our study, we constructed a co-expression network to identify lncRNAs that may be related to immunity. Yi et al. [51] revealed that IL6-AS1 is upregulated in chronic obstructive pulmonary disease and promotes the expression of inflammatory factors, particularly IL-6. This is consistent with the results obtained from our analysis that IL6-AS1 may affect the secretion of IL-6 through the TNF signaling pathway (Fig. 4). GAS5 has also been studied extensively, and some of these studies have also shown that GAS5 is involved in the regulatory process of immune cells, and thus affect disease progression. Ahmad et al. [52] found that GAS5 plays a key role in macrophage differentiation, polarization, and regulation of innate functions including antigen processing and phagocytosis. Li et al. [53] found that GAS5 inhibited Th17 differentiation by promoting TRAF6 mediated ubiquitination of STAT3, thereby alleviating immune thrombocytopenia. These lncRNAs exhibit functions well beyond the regulation of immune processes. In addition to being related to immunity, GAS5 has also been reported to regulate cellular inflammation, lipid uptake, and other processes [54, 55, 56]. In the data set GSE21545, we found that LINC01180 was associated with the instability of AS plaques through univariate Cox regression analysis. The high expression of LINC01180 in PBMCs of patients with AS seems to have a protective effect and should be explored in future studies. Therefore, our findings can provide a foundation for future research on the functions and downstream mechanisms of these lncRNAs.
In recent years, the role of several lncRNA–miRNA–mRNA axes are thought to play a role in the pathogenesis of cardiovascular diseases [57, 58, 59]. In this study, we constructed a lncRNA-associated ceRNA network. The KEGG pathway enrichment analyses of the protein-coding genes in the network revealed that they are primarily involved in response to hypoxia and oxidative stress (Fig. 7A). Ramirez et al. [10], have demonstrated that although adipocytes are primarily present in the PVAT, infiltrating immune cells may become more important under hypoxic and inflammatory conditions. The HIF signaling pathway is the most used for cells to respond to hypoxia, studies have shown that this signaling pathway is involved in the development of the heart, AS and myocardial ischemia [60]. In endothelial cells, smooth muscle cells, macrophages, and other cells, the HIF signaling pathway plays an important regulatory role in hypoxic injury [60]. The janus kinase-signal transducer and activator of transcription (JAK-STAT) signaling pathway is the alternative pathway of the second messenger system, through which chemical signals from outside the cell are transmitted to the cell to induce DNA transcription and intracellular activity, and involved in regulating inflammation [61]. The PI3K/Akt signaling pathway is also closely related to endothelial cell dysfunction and AS [62]. Therefore, lncRNAs in the ceRNA network may participate in the regulation of cardiovascular disease by regulating intracellular inflammation and hypoxia response, which should be studied further. Our results further illustrate the important role of PVAT in the occurrence and development of CHD and AS.
AS is a complex pathological process, and its pathogenesis remains unclear, clinicopathological examinations show that endothelial cells in CVD patients have severe structural and functional damage, indicating that vascular endothelial cells play an important role in the occurrence and development of AS [63]. Therefore, we verified the expression of these lncRNAs related to inflammation and hypoxia by constructing a model of hypoxia endothelial cell injury. A previous study revealed that the expression of GAS5 was increased in ox-LDL-treated THP-1 cells and that GAS5 knockout could potentially promote the reverse transport of cholesterol, inhibit intracellular lipid accumulation, and prevent AS progression [55]. Li et al. [64] revealed that the expression of GAS5 increased in atherosclerotic mouse plaques, and GAS5 knockout promoted endothelial cell proliferation, reduced apoptosis, and improved AS. Chen et al. [65] revealed that GAS5 is related to the aging of smooth muscle and plays a role in the pathological process of AS. H19, a lncRNA that was among the first to be discovered and studied, has also been reported to be involved in cardiovascular diseases, CHD, and AS. Zhang et al. [66] showed that plasma H19 was elevated in patients with CHD, and its level could serve as an independent predictor of CAD. Hobuss et al. [67] found that H19 is upregulated in the acute phase after cardiac ischemia in mice, and in vitro cellular experiments found that hypoxia leads to upregulation of H19 in several cardiac cell types. Cao et al. [68] found that H19 expression was elevated in human atherosclerotic plaques and oxidized-LDL (ox-LDL) treated HUVECs, and that H19 knockdown inhibited ox-LDL induced HUVEC inflammation, apoptosis, and oxidative stress.
Taken together, our findings suggest an important regulatory role for
GAS5 and H19 in AS and CHD, some of which were mechanistically
explored in endothelial cells. Our findings are consistent with previously
published studies, GAS5 and H19 expression were found to be
elevated in the endothelial cell hypoxia injury model. In addition, the
relationship among MIR22HG and LINC01091 and cardiovascular and
endothelial cells has not been reported in the literature. MIR22HG has
been reported to be dysregulated in various tumors [69]. However, the
relationship between MIR22HG and cardiovascular diseases is unclear. A
previous study reported that the expression of MIR22HG is upregulated in
the hypoxic myocardium, thus aggravating hypoxic injury of cardiomyocytes through
NF-
Although lncRNAs have been discovered to be significant regulators of cardiovascular disease, our understanding of the role of these molecules in cardiovascular disease has not yet been extensively investigated [71, 72]. In this study, Bioinformatics was used to investigate the differentially expressed lncRNAs in CHD patients, and to speculate their roles. Some of these lncRNAs, such as GAS5, H19, and IL6-AS1, were compatible with earlier research, indicating that our bioinformatics approach yielded relatively credible results. Finally, our results can provide research ideas for lncRNA studies in AS and CHD. Inevitably, our study has some limitations. First, the number of samples of the source chip was relatively small; therefore, follow-up experimental verification is necessary. Second, the control group was the LIMA PVAT of the same patient instead of the PVAT of a normal human aorta. If the PVAT of a normal human aorta can be obtained as a control, more information may be obtained; however, as stated by the contributor of the chip, due to the particularity of this tissue, it is difficult to obtain it only by performing thoracotomy. LIMA is the blood vessel often selected for coronary artery bypass surgery. Therefore, comparing the aortic PVAT and LIMA PVTA could also partially address the problem. Finally, many of our analyses were based on bioinformatic methods. Some of the lncRNAs mentioned above that may be related to immunity are obtained based on bioinformatics analysis without experimental support and, therefore, need to be verified by flow cytometry or single-cell sequencing and experiments.
In conclusion, through a comprehensive analysis of lncRNAs differentially expressed across different PVAT in patients with CHD, we characterized several lncRNAs that may be related to immunity. We also constructed lncRNA-associated ceRNA networks. These findings further expand our understanding of the pathogenesis of CHD and AS and provide new insights into the function of PVAT in patients with AS and CHD.
CHD, Coronary heart disease; AS, Atherosclerosis; lncRNA, long non-coding RNA; ceRNA, competing endogenous RNA; BP, Biological Process; DEG, Differentially expressed genes; ECM, Endothelial cell culture medium; FDR, False discovery rate; GEO, Gene Expression Omnibus; GO, Gene Ontology; HUVEC, Human umbilical vein endothelial cells; Qpcr, Quantitative real-time polymerase chain reaction; IMA, Internal mammary artery; KEGG, Kyoto Encyclopedia of Genes and Genomes; LIMA, Left internal mammary artery; PVAT, Perivascular adipose tissue.
XX participated in the study design, organized and analyzed the data, and wrote the manuscript. SYW participated in the data analysis and revised the manuscript. JR participated in data sorting and analysis. JX participated in the results and discussion. The other authors participated in study design and conceptualization. YG supervised the study and revised the manuscript. KYL, NL, KL, SLW, and WL contributed to editorial changes in the manuscript. XX, SYW, JR, JX, KYL, NL, KL, SLW, WL, and YG read and approved the final manuscript.
The authors are accountable for all aspects of the work in ensuring that questions related to the accuracy or integrity of any part of the work are appropriately investigated and resolved.
We acknowledge the GEO database for providing its platforms and contributors for uploading their valuable datasets. We would also like to thank Huiqing Wang and Xue Jiang for their valuable comments and help during the revision of the manuscript.
This research was funded by the National Natural Science Foundation of China (grant number 81873495); Heart Failure Center Research Fund of Fujian Provincial Hospital (supported by Fujian Provincial Department of Finance); Natural Science Foundation of Fujian Province (grant number 2018J01242); Fujian Provincial Health Commission Youth Key Talents Project (Key Category) (grant number 2014-ZQN-ZD-2).
The authors declare no conflict of interest.
Publisher’s Note: IMR Press stays neutral with regard to jurisdictional claims in published maps and institutional affiliations.