- Academic Editor
Background: Breast cancer is the most universal malignancy worldwide.
Getting regular screening tests to detect early breast cancer is the surest way
to reduce breast cancer deaths. The purpose of this study was to explore the
predictors of breast cancer screening
behavior among Chinese women using the protection motivation theory (PMT).
Methods: This cross-sectional study included 895 women from eastern
China. Data were collected using an online questionnaire that included
sociodemographic information, PMT theoretical construction, and breast cancer
screening behavior. Structural equation modeling was used to test predictive
relations among the PMT model variables related to breast cancer screening
behavior. Results: The results showed that response
efficiency (
One of the most prevalent cancers in women, breast cancer poses a major risk to the life and health of women, with morbidity and mortality rates among female malignancies ranking first and fifth, respectively, globally [1, 2]. The International Agency for Research on Cancer reported, according to a report in 2020, an estimated 2,300,000 new cases (11.7%) and 685,000 deaths (15.5%) of breast cancer [2]. Compared with other female malignancies, China has the highest incidence of breast cancer, accounting for 19.9% of newly diagnosed breast cancer cases and 9.9% of global breast cancer deaths [2]. The burden of breast cancer is also progressively rising each year as a result of rapid economic development and lifestyle changes, posing significant threats to China’s public health security [3, 4].
Fortunately, routine screening can identify breast cancer in its early-stage, significantly reduce the health consequences of breast cancer, and reduce unnecessary deaths [5, 6]. Mammography, breast self-examination (BSE), and clinical breast examination (CBE) are broadly used screening strategies for breast cancer [7]. A systematic review in 2015 estimated that early effective screening can reduce breast cancer mortality by 20% [8]. According to the National Center for Health Statistics in the United States, breast cancer mortality has continued to decline over the past two decades, which is largely attributed to earlier detection through screening and treatment [9]. Despite mounting clinical evidence of the benefits of early screening, data have demonstrated that breast cancer screening rates in Chinese women are disturbingly low [10, 11]. A study conducted in 2010 indicated that among Chinese women aged 18–107 years, 21.7% had been screened for breast cancer [12]. In China in 2013, a large-scale nationwide survey shows in women aged 35–69 years, only 22.5% of people accept the breast cancer screening [13]. Studies have confirmed that lack of breast cancer knowledge, lack of recognition of the consequence of screening, cost and time constraints, history of breast disease, and negative attitudes are barriers to screening [10, 11, 14, 15]. According to other studies, low participation in screening behavior is predominantly the result of individual decision-making processes. This is influenced by women’s attitudes toward breast cancer screening as well as by screening enablers and barriers [16, 17]. Consequently, it is necessary to investigate which psychological drivers determine women’s engagement in breast cancer screening behavior, and to establish targeted intervention strategies.
The theory of healthy behavior, constructed from psychological and social perspectives, has a major implication for understanding the understanding and prediction of breast cancer screening behavior. As a theoretical model concerned with health behavior decision making, the health belief model (HBM) has been frequently used to evaluate and explain the adoption of breast cancer screening [18]. The HBM argues that six constructs predict health-protective behavior: perceived susceptibility, perceived severity, perceived benefits, perceived barriers, cues to action, and self-efficacy [19]. Previous studies have shown that intervention programs guided by the HBM could help encourage screening participation among women [18, 20]. However, a significant limitation of the HBM is that this theory does not consider the role of the environmental context in adopting a desired health behavior [21]. This underscores the need for a new theory.
Protection motivation theory (PMT) is a promising alternative to the HBM. Developed by Rogers and based on an earlier HBM [22]. The PMT incorporates an individual’s cognitive process into an integrated theoretical structure to explain behavioral motivation and behavior. The PMT posits that health protection behavior under the influence of personal protection motivation, and personal protection motivation is by participating in health behavior motivations to assess [22]. In accordance with PMT, the motivation is the closest and most significant predictor of health-protective behavior, deriving from two psychological processes: threat appraisal and coping appraisal [23]. The fear generated in these two stages can directly influence protection motivation and behavior. Specifically, with the improvement of threat appraisal and response appraisal, an individual’s protection motivation will increase, which will further increase the likelihood of adopting protective behavior. Threat appraisal measures an individual’s perception of the threat to health events or certain behaviors through perceived risk, severity, and fear arousal. Perceived risk reflects the likelihood of being affected by a potential threat [23]. Perceived severity reflects the perception of the degree of a potential threat. Fear arousal refers to the degree of worry affected by a potential threat. Similarly, to evaluate by response efficiency, response cost, and self-efficacy to assess an individual’s ability to deal with and avoid the threat of danger. Response efficiency reflects the perceived effectiveness of a specific preventative behavior. Response costs reflect the perceived costs of adapting to the recommended behavior. Self-efficacy refers to an individual’s perception of their ability to implement preventative behavior [23].
The PMT is an effective framework that has been successfully utilized to predict cancer-preventing behaviors [24, 25, 26]. Li et al. [24], for instance, applied PMT to predict participation in cervical cancer screening. They concluded that PMT subconstructs are important in predicting cervical cancer screening behaviors. Roozbahani et al. [25] investigated skin cancer prevention behaviors based on PMT. They discovered that response efficacy was the strongest kind of protection motivation, and protection motivation was closely related to skin cancer-preventive behaviors. A small-scale study conducted by Zhang et al. [26] indicated the importance of three PMT subconstructs (self-efficacy, perceived severity, and response cost) in predicting the motivation of Chinese women to participate in breast cancer screening. The findings of this study tender useful preliminary data for an in-depth study of Chinese women’s breast cancer screening behavior based on the PMT. In addition, previous studies in Iran have indicated that PMT are highly important in predicting breast cancer screening [27, 28, 29]. The findings from these studies showed that breast cancer screening behaviors were significantly influenced by several PMT subconstructs, including perceived severity, self-efficacy, response efficacy, and perceived cost. However, Chinese women have different values, religious beliefs, cultural backgrounds, health concepts, and health-seeking behaviors, and few studies have implemented the PMT to predict breast cancer screening behavior in Chinese women.
In addition, previous study has interpreted that breast cancer screening utilization is influenced by female’s socioeconomic status (SES) [30]. SES is the position of an individual or group in a class society, and it is a comprehensive reflection of indicators such as occupation, education level, and income [31]. The study has found that low SES is a negative factor that affects individuals’ self-health assessment and behavior [32].
Therefore, based on PMT, we designed a hypothesis model with the introduction of SES (Fig. 1) to determine the influencing factors of women’s breast cancer screening behavior and the relationship between influencing factors. The hypotheses of this study are as follows.
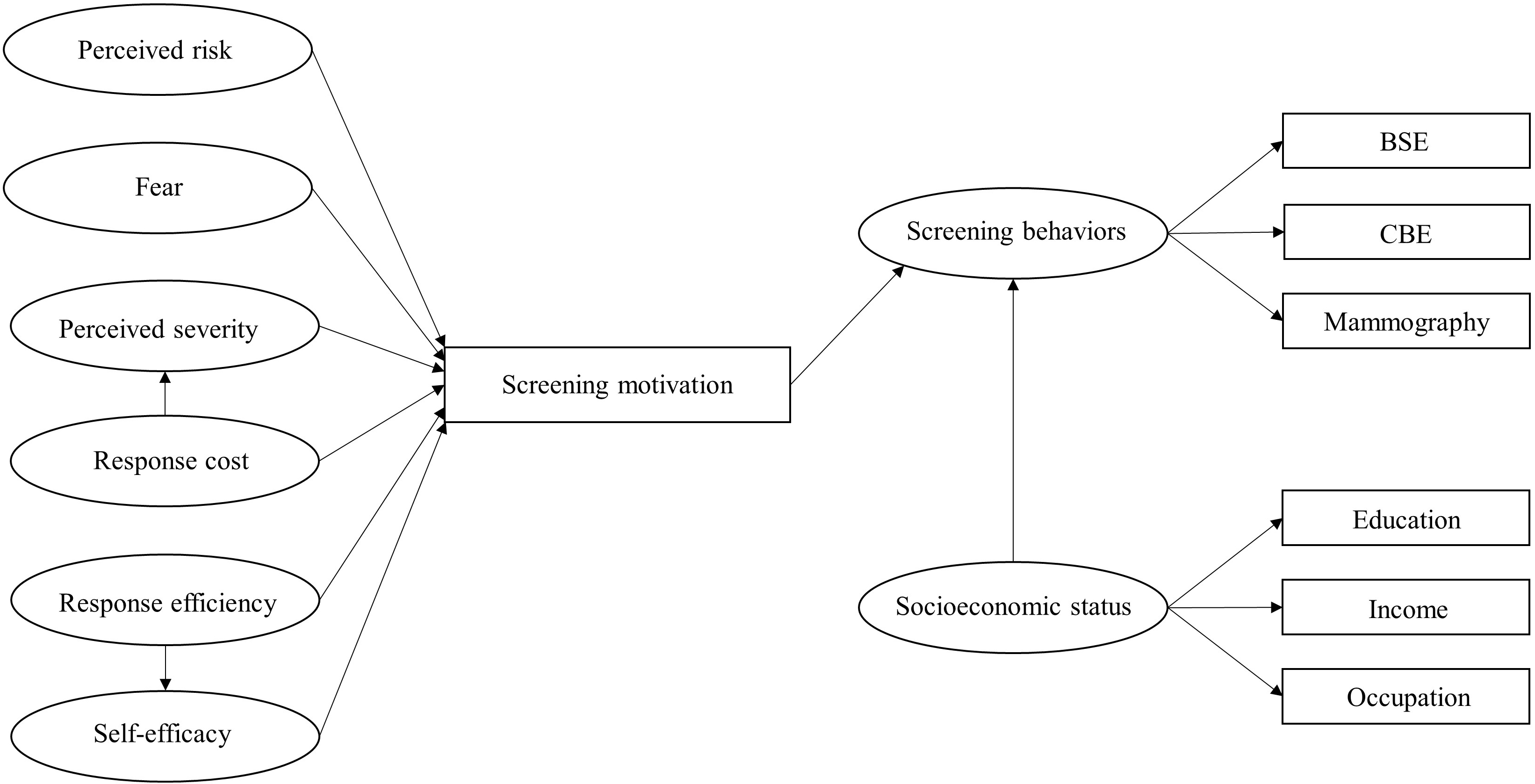
Hypothesis model. The rectangle represents the observed variable and the ellipse represents the latent variable. BSE, breast self-examination; CBE, clinical breast examination.
• PMT substructure influences breast screening behavior in women.
• SES influences breast screening behavior in women.
• There are interconnections between PMT substructures.
The purpose of this study is to identify a set of predictors of breast cancer screening behavior among Chinese women based on the PMT and to identify the associations between SES and screening behavior. Our results provide theoretical basis for professionals, and help health systems to customize effective breast screening intervention strategies. At the social level, this study contributes to the improvement of breast screening coverage.
This work was approved by the Ethics Committee of Bengbu Medical College (2021–070).
This cross-sectional survey used a convenience sampling method and data
collection was actualized between April and May 2020, the study was conducted in
August 2020. We published an anonymous online questionnaire through WeChat 8.0.11
(Tencent, Shenzhen, Guangdong, China), and each participant only had a unique ID
to respond. If participants leave the questionnaire page or stop answering for
more than 15 minutes, the questionnaire will be automatically collected. All
eligible participants completed the questionnaire independently after voluntarily
providing oral informed consent. The inclusion criteria were as follows: (1) the
place of residence was Anhui Province, East China; (2) at least 18 years of age;
(3) women without a history of breast cancer; (4) no reading and behavior
disorders. Exclusion criteria were: (1) excessive data missing (
The authors did not access information that identified individual participants during or after completing the anonymous online questionnaire. The average time participants took to complete the questionnaire was approximately 10–15 minutes. A total of 956 questionnaires were distributed. After excluding 61 questionnaires with missing data, 895 valid questionnaires were collected, with an effective response rate of 93.6%.
A single population ratio statistical formula was used to calculate the required sample size:
At the 95% confidence level, the corresponding critical value was Z = 1.96. According to a previous study, the p value was 53.6%. After considering a 10% non-response rate and 5% error, the required sample size was 572. By increasing the nonresponse rate by 20%, the minimum required sample size was 715. The sample size obtained was larger than the minimum requirement, which increased the credibility of the results.
The original questionnaire was customized based on the relevant literature and our previous research [15, 33, 34]. The items were validated by experts from academics from field. The questionnaire was pre-tested on a random sample of community women. According to the pre-test results and expert opinions, we adjusted and improved the questionnaire.
The questionnaire consisted of three sections: sociodemographic information, PMT theoretical constructs, and breast cancer screening behavior. Social demographic information included age, marital status, occupation, education level, and annual family income. The participants were also asked about whether to accept a breast health education. Education, occupation, and annual family income were combined into a latent variable called “SES”.
The second section of the questionnaire was designed as a list of questions to measure PMT subconstructs including perceived risk with 3 items (e.g., “Are more likely to develop breast cancer than others”), perceived severity with 6 items (e.g., “Once you get breast cancer, your life changes dramatically”), fear arousal with 3 items (e.g., “The thought of getting breast cancer makes you nervous”), response efficiency with 3 items (e.g., “You can recognize normal and abnormal changes in your body”), response cost with 3 items (e.g., “Breast cancer screening is a waste of time”), self-efficacy with 3 items (e.g., “You can stick to regular breast self-exams if you try”), and also screening motivation.
All PMT model items, except screening motivations, were measured on a five-point Likert Scale, with a score of 1–5 indicating “strongly disagree” to “strongly agree”.
Screening motivation was evaluated by asking participants, ‘Would you be willing to take part in breast cancer screening in the near future?’ The response options were “Yes” and “No”.
Breast cancer screening behavior (3 items) was surveyed on a five-point scale of 1 to 5 indicating “Never” to “Always”.
The Kaiser-Meyer Olkin and Cronbach’s alphas were 0.815 and 0.742, respectively.
The data were analyzed using IBM SPSS Statistics 25.0 (International Business Machines Corporation, Armonk, NY, USA) and IBM SPSS Amos 24.0 (International Business Machines Corporation, Armonk, NY, USA).
The individuals’ basic demographic traits were examined using descriptive
statistical methods. We employed factor analysis and Cronbach’s alphas to
evaluate the efficacy and dependability of the PMT assessment methods. We used
the Kolmogorov-Smirnov test for normality testing and exploratory factor analysis
to screen the variables in the model construction. The principal component method
and maximum variance orthogonal rotation were used for the factor analysis. The
factors with characteristic roots
Table 1 shows that the participants’ average age was 28.4 years old, most of them lived in rural areas (51.28%), most had a bachelor’s or college degree (85.15%), and most women were unmarried (71.17%). Most participants had never received health education on breast cancer prevention (61.34%). In terms of breast screening behavior, 50.4% of the participants had undergone a breast self-examination. Only 13.2% and 5.8% of the patients had been exposed to clinical breast examinations and mammography, respectively (Table 2).
Variable | Frequency | Percent (%) | |
Age in years | |||
18–30 | 652 | 72.85 | |
31–40 | 44 | 4.92 | |
41–50 | 118 | 13.18 | |
51–60 | 80 | 8.94 | |
1 | 0.11 | ||
Birthplace | |||
Urban area | 436 | 48.72 | |
Rural area | 459 | 51.28 | |
Occupation | |||
No occupation | 42 | 4.69 | |
Low professional respect | 580 | 64.81 | |
Medium professional respect | 132 | 14.75 | |
High professional respect | 141 | 15.75 | |
Education | |||
Primary schools or below | 5 | 0.55 | |
Secondary school or technical secondary school | 73 | 8.15 | |
Bachelor or junior college | 762 | 85.15 | |
Master or doctor | 55 | 6.15 | |
Marital status | |||
Unmarried | 637 | 71.17 | |
Married | 246 | 27.49 | |
Widowed/divorced | 12 | 1.34 | |
Annual family income (¥) | |||
Less than ¥ 50,000 |
251 | 28.04 | |
¥ 50,000–120,000 |
402 | 44.92 | |
More than ¥ 120,000 |
242 | 27.04 | |
Ever received breast health education | |||
Yes | 346 | 38.66 | |
No | 549 | 61.34 |
Variables | Number (percent) | ||||
Never | Sometimes | Half of times | Most of times | Always | |
BSE | 310 (34.6%) | 451 (50.4%) | 37 (4.1%) | 57 (6.4%) | 40 (4.5%) |
CBE | 533 (59.6%) | 118 (13.2%) | 30 (3.3%) | 157 (17.5%) | 57 (6.4%) |
Mammography | 744 (83.1%) | 52 (5.8%) | 31 (3.5%) | 52 (5.8%) | 16 (1.8%) |
BSE, breast self-examination; CBE, clinical breast examination.
In the exploratory factor analysis, Kaiser-Meyer-Olkin (KMO) = 0.815, Bartlett’s sphere test
statistic = 7765.908, p
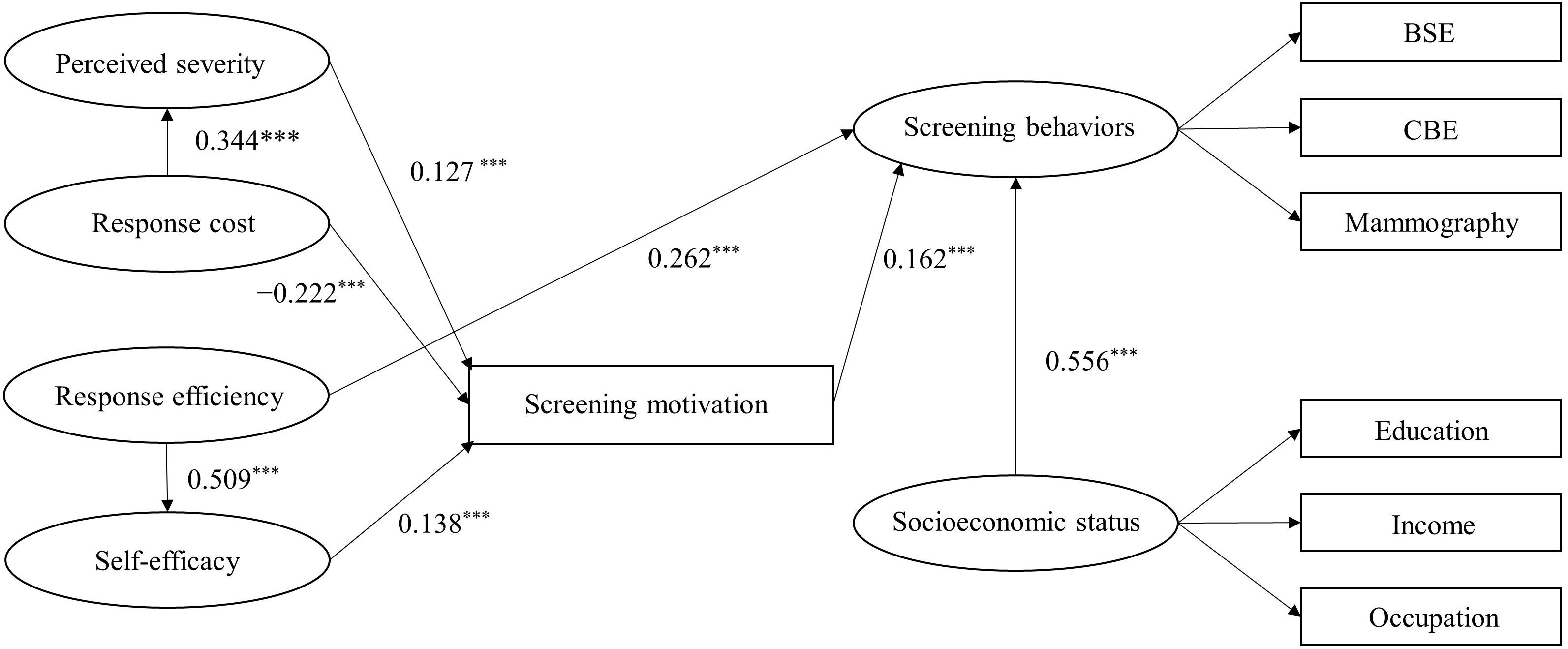
Modified model with standardized path coefficients. BSE, breast
self-examination; CBE, clinical breast examination. *** p
The modified model showed that perceived severity (
As shown in Table 3, response cost indirectly affected screening motivation
through perceived severity (
Model paths | Standardized direct effects | 95% CI | Standardized indirect effects | 95% CI | ||
LLCI | ULCI | LLCI | ULCI | |||
Response cost |
0.344 | 0.412 | 0.717 | |||
Response efficiency |
0.509 | 0.255 | 0.386 | |||
Response cost |
−0.222 | −0.256 | −0.113 | 0.044 | 0.013 | 0.063 |
Response efficiency |
0.070 | 0.021 | 0.066 | |||
Perceived severity |
0.127 | 0.024 | 0.100 | |||
Self-efficacy |
0.138 | 0.063 | 0.197 | |||
Response cost |
−0.029 | −0.047 | −0.01 | |||
Response efficiency |
0.262 | 0.097 | 0.231 | 0.011 | 0.003 | 0.015 |
Perceived severity |
0.020 | 0.004 | 0.022 | |||
Self-efficacy |
0.022 | 0.009 | 0.044 | |||
SES |
0.556 | 0.230 | 0.622 | |||
Screening motivation |
0.162 | 0.078 | 0.264 |
CI, confidence interval; LLCI, lower limit of confidence interval; SES, socioeconomic status; ULCI, upper limit of the confidence interval.
Table 4 presents the results of Pearson’s correlation analysis of the variables.
Breast screening behavior was significantly related to response cost (r
= –0.184, p
Variables | X2 | X3 | X4 | X5 | X6 | X7 |
X1 Screening behavior | −0.015 | −0.184** | 0.323** | 0.197** | 0.350** | 0.274** |
X2 Perceived severity | 0.317** | 0.002 | 0.019 | −0.015 | 0.041 | |
X3 Response cost | −0.181** | −0.235** | −0.167** | −0.181** | ||
X4 Response efficiency | 0.499** | 0.169** | 0.210** | |||
X5 Self-efficacy | 0.144** | 0.205** | ||||
X6 SES | 0.141** | |||||
X7 Screening motivation | 1 |
SES, socioeconomic status.
** p
The modified model uses the goodness-of-fit index to evaluate the model as a whole. It is necessary to explain whether the model fits correctly according to multiple fitting indices. The relevant fitting index data for this study are presented in Table 5. It can be seen that the model fitting indices meet the corresponding requirements of the reference standards, and the model and data fit well.
Fit indices | GFI | AGFI | CFI | SRMR | RMSEA | |
Recommended values | ||||||
Result | 3.078 | 0.944 | 0.923 | 0.949 | 0.05 | 0.048 |
AGFI, adjusted goodness-of-fit index; CFI, comparative fit index; GFI, goodness-of-fit index; SRMR, standardized root mean square residual; RMSEA, root mean square error of approximation.
The predictive value of PMT on breast cancer screening behavior in a group of women in eastern China was assessed in this study using structural equation modeling in accordance with the PMT theory. The results from this study will be useful in creating intervention plans that will be successful in encouraging women to get screened for breast cancer.
Our findings demonstrated that women’s motivation to attend breast cancer screening was negatively impacted by response cost, but both self-efficacy and perceived severity had a direct and positive impact on motivation. Numerous investigations have backed up this finding, which is also in line with earlier research. The study carried out by Liu et al. [35] in Tianjin, China, showed that the response cost was negatively related to the motivation to receive cervical cancer vaccine, and perceived severity and self-efficacy had positive effects on the motivation to receive the vaccine.
In the modified model, we cut out the path between response efficiency and motivation because the path fitting degree did not reach the required fitting degree. However, a study in Australia by Tesson et al. [36] showed that response efficacy and breast cancer prevention motivation were independent and significantly related. Their results were limited to clinical prevention, but were sufficient to explain the direct impact of response efficiency on breast cancer prevention motivations. Although our research interrupted the path between response efficiency and screening motivation, the results of the study showed that response efficiency had a significant positive impact on self-efficacy and a direct impact on screening behavior. Description response efficiency still indirectly affects screening motivation, which further confirms that response efficiency is a combination of knowledge and suggestive measures [37, 38]. Our findings were confirmed in a cervical cancer screening study conducted by Kim et al. [39] in Korean American women. Scholar Kim et al. [39] found that the direct impact between recommended measures and inspection behavior was very important. Health knowledge and recommended measures are mediated by self-efficacy between behaviors.
The findings demonstrated a direct and advantageous link between perceived severity and response cost. In accordance with the literature, we gathered a few scholars discussing the relationship between response cost and perceived severity in research on PMT. However, the more serious the disease, the more time and money it takes to cure. Therefore, response cost can help women understand the risk of the disease to a certain extent and affect their perceived severity of breast cancer.
Among the three factors influencing screening behavior, SES had the strongest promotion effect, whereas screening motivation had a relatively weak promotion effect. This suggests that SES is the most important factor of female breast cancer screening. This result was supported by the findings of Frie et al. [40] and You et al. [41]. Frie et al. [40] surveyed women in the Trivandrum region of India to study the determinants of their participation in the breast screening process. They found that education, occupation, and type of house were independently associated with breast screening in women, and showed that women with high SES were more indicated to undergo breast screening. In a study by You et al. [41] on human papillomavirus (HPV) vaccine uptake and willingness to receive HPV vaccination, higher SES had a direct effect on HPV vaccination. Likewise, indicators of socioeconomic status were correlated with the motivation to receive the HPV vaccine.
Regrettably, although the number of breast cancer patients in China is increasing annually, women still lack routine breast cancer screening and know very little about breast cancer. This study discovered that, from a number of angles, SES and knowledge about breast cancer could influence women’s screening practices. Compared to changing SES, it is obvious that the popularization of breast cancer-related knowledge is more convenient and cheaper. Therefore, to strengthen women’s breast screening behavior, it is possible to conduct breast screening science education, such as BSE, CBE, mammography, and other related knowledge. It is necessary to start with screening principles, results, and methods to accelerate women’s awareness of breast screening, increase women’s breast cancer prevention behaviors and reduce breast cancer mortality.
This study has several limitations. Online self-report questionnaires have some restrictions. The decisions made by participants may be influenced by potential biases and social norms. Although our sample size far exceeds the minimum required sample size, the surveyed women were limited to Anhui Province in eastern China. This may limit the universality of our results and cannot reflect women’s attitudes toward breast cancer in developed regions. Finally, in the process of building the PMT model, the traditional method was not strictly followed. We were improved to some extent, bypassing the two processes of threat assessment and response assessment, and directly discussing the factors that affect motivation.
This study demonstrated that PMT can accurately predict breast screening behavior among Eastern Chinese women. In the PMT substructure, response efficiency and screening motivation were directly associated with screening uptake, while perceived severity, response cost, and self-efficacy indirectly affected screening behavior through screening motivations.
SES is an important predictor of the uptake screening of breast cancer. The findings provide valuable data for designing more effective breast cancer screening interventions to promote the screening uptake, reduce unnecessary mortality and improve survival.
BSE, breast self-examination; CBE, clinical breast examination; HBM, health belief model; PMT, protection motivation theory; SES, socioeconomic status; CI, confidence interval; LLCI, lower limit of confidence interval; ULCI, upper limit of the confidence interval; AGFI, adjusted goodness-of-fit index; CFI, comparative fit index; GFI, goodness-of-fit index; SRMR, standardized root mean square residual; RMSEA, root mean square error of approximation; HPV, human papillomavirus.
The datasets used and/or analyzed during the current study are available from the corresponding author on reasonable request.
SZ designed the research study. YG, JY, HW, BL, and SZ performed the research. YG analyzed the data. YG and SZ wrote the manuscript. All authors contributed to editorial changes in the manuscript. All authors read and approved the final manuscript. All authors have participated sufficiently in the work and agreed to be accountable for all aspects of the work.
The study was conducted in accordance with the Declaration of Helsinki. This work was approved by the Ethics Committee of Bengbu Medical College (2021–070). All eligible participants were informed of the study objectives and consent information.
We thank all study participants for their dedication.
This research was supported by the Key Projects of Science Research in Colleges in Anhui Province, China (No. SK2020A0348, SK2018A1074), Anhui Provincial Colleges and Universities Ideological and Political Work Ability Improvement Project (No. sztsjh2019-7-14), the Key Projects of Teaching Research for Colleges and Universities in Anhui Province, China (No. 2020jyxm1174), and Ideological and Political Teaching Team of Medical Imaging Course in Anhui Province, China (No. 2020kcszjxtd54).
The authors declare no conflict of interest.
Publisher’s Note: IMR Press stays neutral with regard to jurisdictional claims in published maps and institutional affiliations.